Luís G. N. Martins1, Michel B. Stefanello1, Gervásio A. Degrazia1, Lidiane Buligon2, Luca Mortarini3, Lilian P. Moor1, Debora R. Roberti1, Felipe D. Costa4, Franciano S. Puhales1, Domenico Anfossi3
1Departamento de Física, Universidade Federal de Santa Maria, Santa Maria, Brasil
2Departamento de Matemática, Universidade Federal de Santa Maria, Santa Maria, Brasil
3Institute of Atmospheric Sciences and Climate (CNR/ISAC), Unit of Turin, Turin, Italy
4Programa de Pós-Graduação em Engenharia, Universidade Federal do Pampa, Campus Alegrete, Alegrete, Brasil
Correspondence to: Gervásio A. Degrazia, Departamento de Física, Universidade Federal de Santa Maria, Santa Maria, Brasil.
Email: |  |
Copyright © 2016 Scientific & Academic Publishing. All Rights Reserved.
This work is licensed under the Creative Commons Attribution International License (CC BY).
http://creativecommons.org/licenses/by/4.0/

Abstract
The following study employs two different autocorrelation functions (ACF) to obtain the principal parameters that describe the behavior of the low-wind meandering phenomenon. The looping parameter (m) and the meandering time scale (T*) were estimated from the fit of 828 experimental ACF evaluated from measurements accomplished at the Santa Maria micrometeorological site (Santa Maria, south of Brazil). The results show that both meandering ACFs appropriately fit the negative lobes and the oscillatory behavior observed in experimental meandering ACFs. The T* parameter, obtained from the distinct ACF formulations, agrees in most of the analyzed cases. However, substantial differences between the values of the m parameter, obtained by the two formulations, are observed. This difference is more evident for higher values of m. This highlights the distinct functional form that describes the turbulence in the meandering ACF.
Keywords:
Wind meandering, Autocorrelation functions, Low wind
Cite this paper: Luís G. N. Martins, Michel B. Stefanello, Gervásio A. Degrazia, Lidiane Buligon, Luca Mortarini, Lilian P. Moor, Debora R. Roberti, Felipe D. Costa, Franciano S. Puhales, Domenico Anfossi, Comparing Meandering Parameters from the Distinct Autocorrelation Functions, American Journal of Environmental Engineering, Vol. 6 No. 4A, 2016, pp. 116-118. doi: 10.5923/s.ajee.201601.17.
1. Introduction
The velocity autocorrelation functions are important statistical quantities to describe atmospheric movements. Such functions can be used to calculate the dispersion parameters associated to the turbulent diffusion modeling studies in the planetary boundary layer (PBL). Based on the Taylor Statistical Diffusion Theory, the following formula has been proposed by Frenkiel [1] and [2] to represent the autocorrelation functions describing the connected states between turbulent and non-turbulent (submeso motions e.g. [3]) movements.  | (1) |
This functional form is composed of the product of classical exponential function, that represents the autocorrelation function for a fully developed turbulence, by the cosine function that describe the larger scale movements, such as the wind meandering, associated to the observed low frequency horizontal wind oscillation.Recently, [4] proposed a new mathematical formulation to represent turbulent and submeso motions. This formulation is similar to those of Frenkiel and can be described as: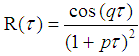 | (2) |
Differently from Eq. (1), Eq. (2) contains a binomial function to represent the fully developed turbulence [5]. The cosine function allows the reproduction of the negative lobes observed in the autocorrelation functions ([6]; [4]). Both mathematical formulations are hybrid, since that they are described in term of
, the Lagrangian integral time scale for a fully developed turbulence, and m the loop parameter, which controls the meandering oscillation frequency associated to the horizontal wind. The m parameter controls the negative lobe absolute value in the autocorrelation functions and as a consequence defines the meandering phenomenon intensity [6]. Following [2] and [7] p and q are defined by the relations: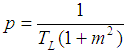 | (3) |
where T* is the meandering period. The ratio between Eq. (4) and Eq. (3) yields:  | (4) |
In this study, employing observational wind data and the Eqs. (1) and (2), we evaluate the principal parameters that characterize the meandering phenomenon. Specifically, the magnitudes of mu,v,T and
(u and v are the horizontal and lateral turbulent velocity components and T is the temperature), are calculated. Furthermore, the looping parameters and the meandering periods derived from Eq. (1) are compared with those obtained from Eq. (2).
2. Results and Discussion
In this section, we analyze low-wind speed data to evaluate experimental autocorrelation functions. These experimental curves, presenting negative lobes, are fitted by mathematical formulations provided by the Eqs. (1) and (2). Therefore, Eqs. (1) and (2) are mathematical representations that reproduce the observed meandering curves and are used to obtain the loop parameters and the meandering periods. The low-wind speed dataset employed in this analysis were measured in a micrometeorological station (UFSM, Rio Grande do Sul, Brazil) between November 2013 and December 2014. Observations of wind speed and temperature were sampled by a sonic anemometer installed at 3 m in a tower at a frequency of 10Hz. Figures 1-3 show peculiar patterns of the comparison among the velocity and temperature ACFs calculated on the Santa Maria low wind speed dataset (continuous line) and the associated best fits (dotted and dashed lines) calculated from the ACFs (Eqs. (1) and (2) respectively). 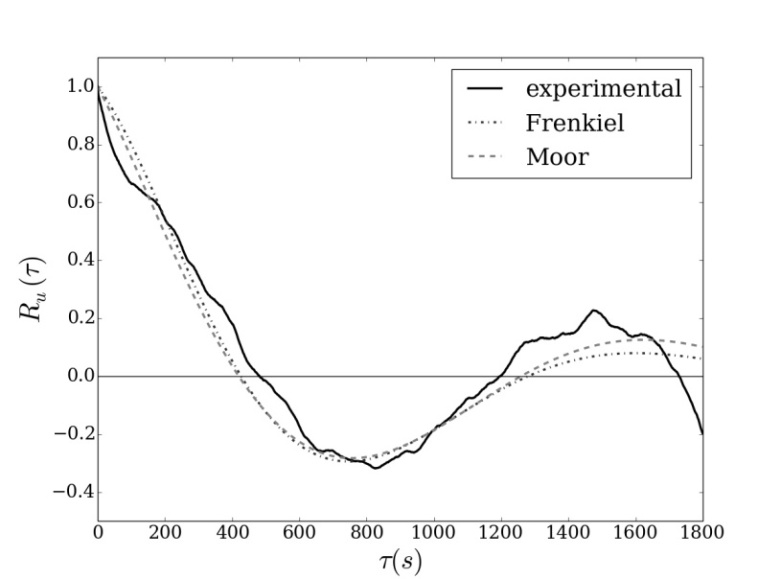 | Figure 1. Comparison between the ACF estimated on the Santa Maria dataset (continuous line) and the associated best fits (dotted and dashed lines) for the u component, evaluated from Eq.(1) and from Eq. (2) on April 25 of 2014, 00:00LT;  |
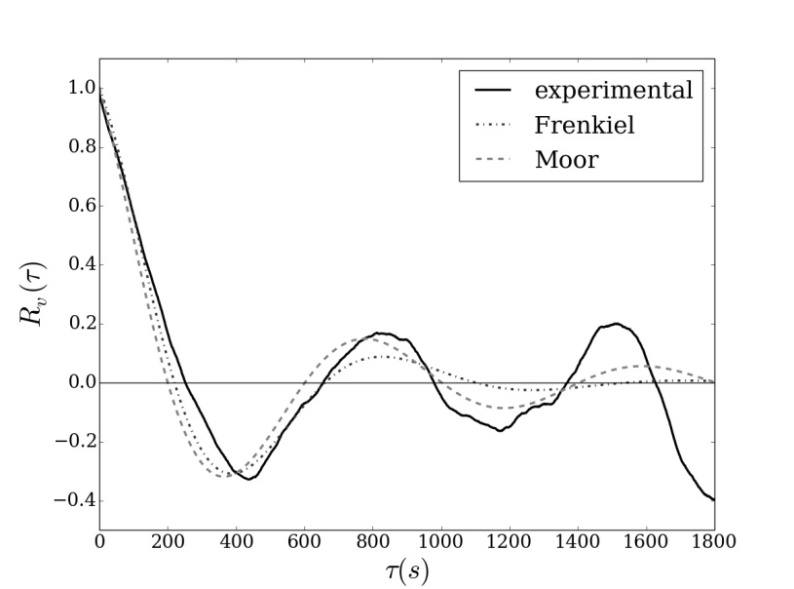 | Figure 2. As in Fig. 1, for the v component |
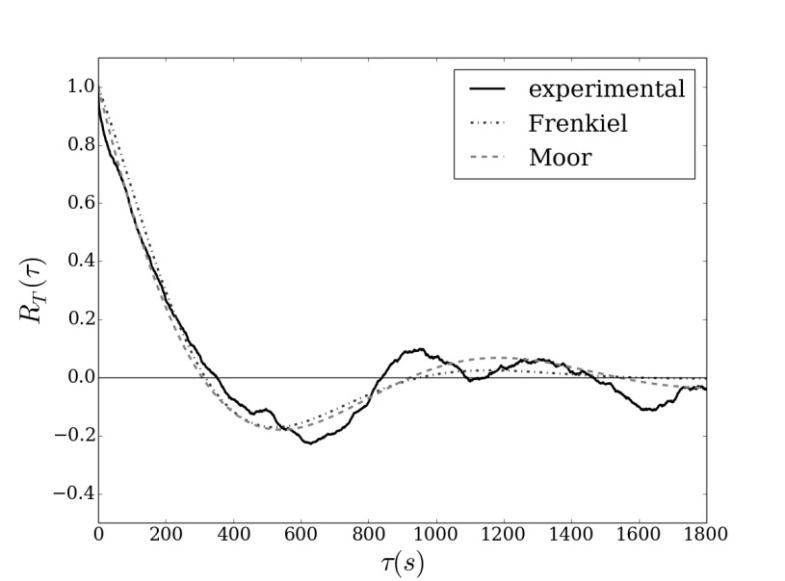 | Figure 3. As in Fig. 1, for the temperature |
Following [6], all the ACFs analyzed in this study were evaluated from one-hour time series having the mean horizontal speed less than 1.5 m s-1 and looping parameters
. Eq. (4) provides the meandering characteristic time scale (meandering period). From this equation values of the magnitude of T* were obtained. Figure 4 shows the scatter plots of T* calculated from Eq. (1) (x-axis) and Eq. (2) (y-axis). It can be seen that there is a very good agreement between the magnitudes of T* obtained from the two mathematical formulations. This behavior is evidenced by the fact that most of the T* values are situated on the line of perfect agreement. From Eq. (5) the loop parameter m can be estimated. Figure 5 exhibits the scatter plot of the m values obtained from Eq. (1) (x-axis) and Eq. (2) (y-axis). Looking at this scatter plot, it is evident that the loop parameter estimated from Eq. (2) presents greater magnitudes than the one provided by Eq. (1). Furthermore, it is important to note that the Figure 4 present a linear relation between the m values calculated from Eqs. (1) and (2). 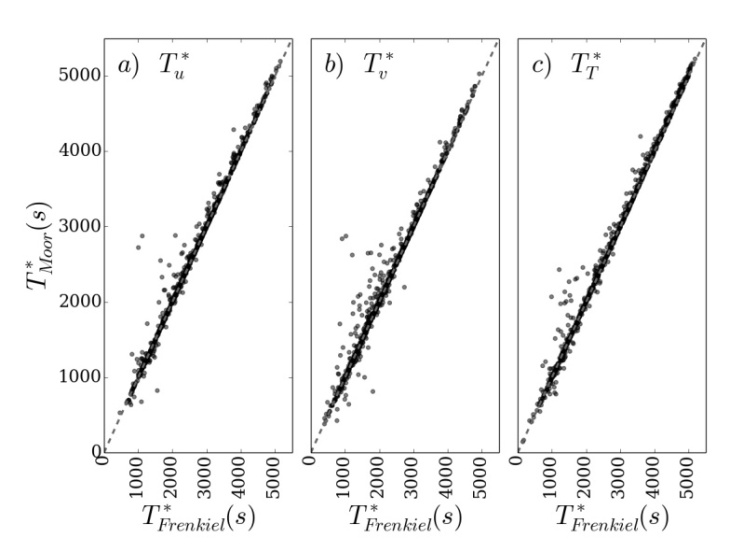 | Figure 4. Meandering period for all low wind dataset |
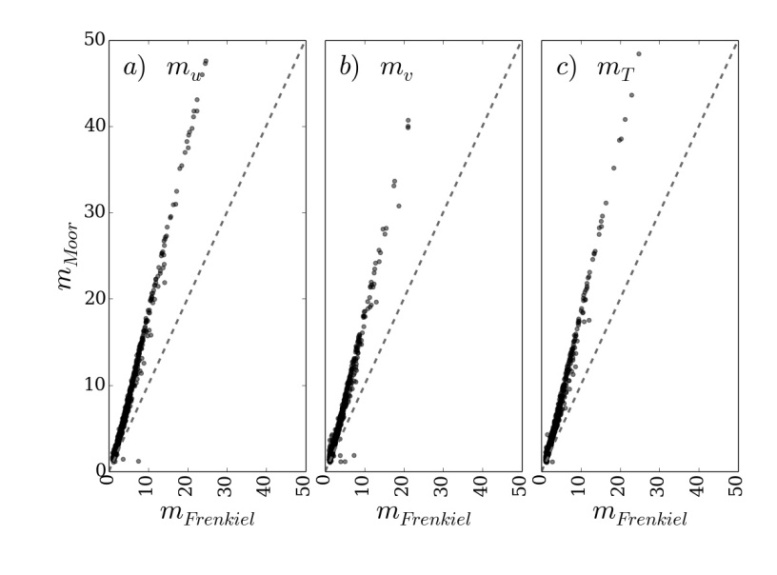 | Figure 5. Loop parameter (m) for all low wind dataset |
The higher values of m, calculated from Eq. (2), suggest that the binomial formula contained in this equation reproduces for longer time the oscillatory effects associated to the meandering motions. This means that the values of the p parameter from the fitting of Eq. (2) are smaller than the ones obtained from Eq. (1). These differences evidence the distinct functional formulation that represents the turbulent component of the low-wind ACF. The present analysis shows that both ACFs can be employed to obtain meandering parameters from wind and temperature observational data.
3. Conclusions
We present a comparison between two mathematical formulations that describe the negative lobes associated to the observed meandering ACF. The analysis shows that both mathematical expressions appropriately fit the experimental data. The oscillatory behavior presents in the meandering motion are equally well described by both representations. Thus, the meandering time scale can be estimated independently of the ACF formulation chosen. On the other hand, the m parameter is larger for the case in which the turbulence movement is represented by the binomial function. This last decreases more slowly than the exponential function.
ACKNOWLEDGEMENTS
The authors would like to thank the CNPq and Capes for partial financial support of this work.
References
[1] | Frenkiel, F.N., (1953) Turbulent diffusion: mean concentration distribution in a flow feld of homogeneous turbulence Adv. Appl. Mech., 3, pp. 61–107. |
[2] | Murgatroyd, R., (1969). Estimations from geostrophic trajectories of horizontal diffusivity in the mid-latitude troposphere and lower stratosphere. Q J R Meteorol Soc 95:40–62. |
[3] | Mahrt, L., (2014). Stably stratified atmospheric boundary layers. Annu Rev Fluid Mech 46:23–45. |
[4] | Moor, L., Degrazia, G., Stefanello, M., Mortarini, L., Acevedo, O., Maldaner, S., Szinvelski, C. Roberti, D., Buligon, L., Anfossi, D., (2015). Proposal of a new autocorrelation function in low wind speed conditions, Physica A: Statistical Mechanics and its Applications 438 286–292. |
[5] | Phillips, P., Panofsky, H. A., (1982). A re-examination of lateral dispersion from continuous sources. Atmospheric Environment, 16(8), 1851–1859. |
[6] | Anfossi, D., Oett, D., Degrazia, G., Goulart, A., (2005). An analysis of sonic anemometer observations in low-wind speed conditions. Boundary-Layer Meteorol 114:179–203. |
[7] | Mortarini L., Anfossi, D., (2015). Proposal of an empirical velocity spectrum formula in low-wind speed conditions. Q J R Meteorol Soc 141:85–97. |