Lilian P. Moor1, 2, Lidiane Buligon3, Jonas da Costa Carvalho4, Gervásio Annes Degrazia1, Michel Stefanello1, Otávio Acevedo1, Luca Mortarini5
1Programa de Pós-Graduação em Física, Universidade Federal de Santa Maria, Santa Maria, RS, Brazil
2Instituto Federal Farroupilha, Jaguari, RS, Brazil
3Departamento de Matemática, Universidade Federal de Santa Maria, Santa Maria, RS, Brazil
4Faculdade de Meteorologia, Universidade Federal de Pelotas, Pelotas, RS, Brazil
5Institute of Atmospheric Sciences and Climate (ISAC) of the Italian National Research Council (CNR), Unit of Turin, Italy
Correspondence to: Lilian P. Moor, Programa de Pós-Graduação em Física, Universidade Federal de Santa Maria, Santa Maria, RS, Brazil.
Email: |  |
Copyright © 2016 Scientific & Academic Publishing. All Rights Reserved.
This work is licensed under the Creative Commons Attribution International License (CC BY).
http://creativecommons.org/licenses/by/4.0/

Abstract
In this study we present a simulation of the meandering of contaminant dispersion in a low wind stable boundary-layer. The horizontal wind meandering parameters are obtained from data were measured in Brazilian Amazon region. These new observational parameters are employed in the Iterative Langevin Solution for Low Wind (ILS-LW) model. The results are compared with the experimental data of Idaho National Engineering Laboratory (INEL). The simulated concentrations agree with the values presented and discussed in the literature.
Keywords:
Contaminant Dispersion, Horizontal Wind Meandering, Lagrangian Dispersion Model
Cite this paper: Lilian P. Moor, Lidiane Buligon, Jonas da Costa Carvalho, Gervásio Annes Degrazia, Michel Stefanello, Otávio Acevedo, Luca Mortarini, Meandering of Contaminant Dispersion, American Journal of Environmental Engineering, Vol. 6 No. 4A, 2016, pp. 46-49. doi: 10.5923/s.ajee.201601.07.
1. Introduction
The dispersion of contaminants in low wind speed conditions in the Stable Boundary Layer (SBL) represents a difficult physical problem as the occurrence of meandering winds do not allow traditional dispersion models to simulate adequately the contaminant concentration. Thus, it is essential to develop new parameterizations that describe such processes as they are observed in the atmosphere [1, 2]. In situations in which the wind mean velocity is small, the horizontal wind direction is subject to large variations. Therefore, it is not possible to determine a preferential direction [3, 4]. In these conditions, the large horizontal wind oscillations of low frequency, called meandering, spread the contaminants over a rather wide angular sector, which reinforces the horizontal transport of contaminant [1, 5]. Consequently, the meandering phenomenon needs to be incorporated in the simulations of the contaminant dispersion [6, 7]. In this particular case, the Lagrangian models are considered the more indicated, because they describe appropriately the dispersion process during the presence of the meandering. Furthermore, in these models it is possible to incorporate, in a more simple way, temporal and spatial variations in turbulence properties in relation to other techniques, such as similarity or gradient-transfer theory [8, 9]. In this study we employ the model ILS-LW [8] to simulate the enhanced meandering dispersion. The meandering loop parameter
and meandering period
used in this simulation are obtained from observational data measured in Brazilian Amazon Region [4].The performance of the ILS-LW model using the meandering parameters proposed by Moor et al. [4] is validated considering the values of ground-level concentrations measured during the experiments of the Idaho National Engineering Laboratory (INEL) [10]. In addition, we present a statistical analysis to evaluate the simulation with new meandering parameters in comparison with the observed concentrations [10]. In Section 2 we present the Langevin model, in Section 3 the meandering parameters employed in the simulations and in Section 4 the results are discussed.
2. Lagrangian Stochastic Particle Model
Lagrangian stochastic particle models are based on Langevin equation [11], where the particle turbulent velocity is given by the combination between a deterministic term
and a stochastic term
The velocity and the position of each particle, in each time, are obtained from the numerical integration of the equations: 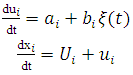 | (1) |
where
is the turbulent velocity,
is the displacement,
is the mean wind velocity. The method applied solves the Langevin equation (Eq. (1)), in a semi-analytical manner, using the method of successive approximations, or Picard’s Iterative Method [8].
3. Experimental Physical Parameters of the Horizontal Wind Meandering
[4] presented a new autocorrelation function to study horizontal wind meandering. From a dataset measured in Brazilian Amazon region, they obtained an experimental fitting that allows to calculate the meandering time scale and the loop parameter magnitudes for low wind speed in a nocturnal stable layer (Table 1).Table 1. Meandering period and loop parameter average values for the u and v components by [4]  |
| |
|
The autocorrelation function proposed in [4] considers cases in which turbulence coexists with low-frequency motion associated to the meandering phenomenon. The expression is provided by | (2) |
Equation (2) can also be written in a different manner, namely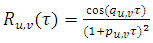 | (3) |
with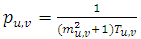 | (4) |
and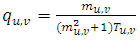 | (5) |
are hybrid quantities described in terms of the time scale for a fully developed turbulence
and a non-dimensional quantity that controls the meandering oscillation frequency
associated to large oscillation of the horizontal wind [1, 12, 13]. This autocorrelation function generates a negative lobe for the horizontal wind components attributed to the meander [1, 4].
4. Results and Discussions
In the simulations the mean values of the loop parameter
and the meandering period
(Table 1) were employed in the ILS-LW model [8]. The results are compared with concentrations measured data in the Idaho National Engineering Laboratory (INEL) [10]. Furthermore, the concentrations data from our simulation are compared with the simulation performed by [8]. Table 2 shows the comparisons between the simulated concentration data and observed concentrations data.Figure 1 represents the scatter diagram between observed and predicted ground-level concentrations. The scatter over the central line shows that new parameterization to the meandering loop parameter and the meandering period inserted in this model reproduces satisfactorily the experimental data in low wind speed conditions. Furthermore, the concentrations simulated values are similar with those obtained by [8].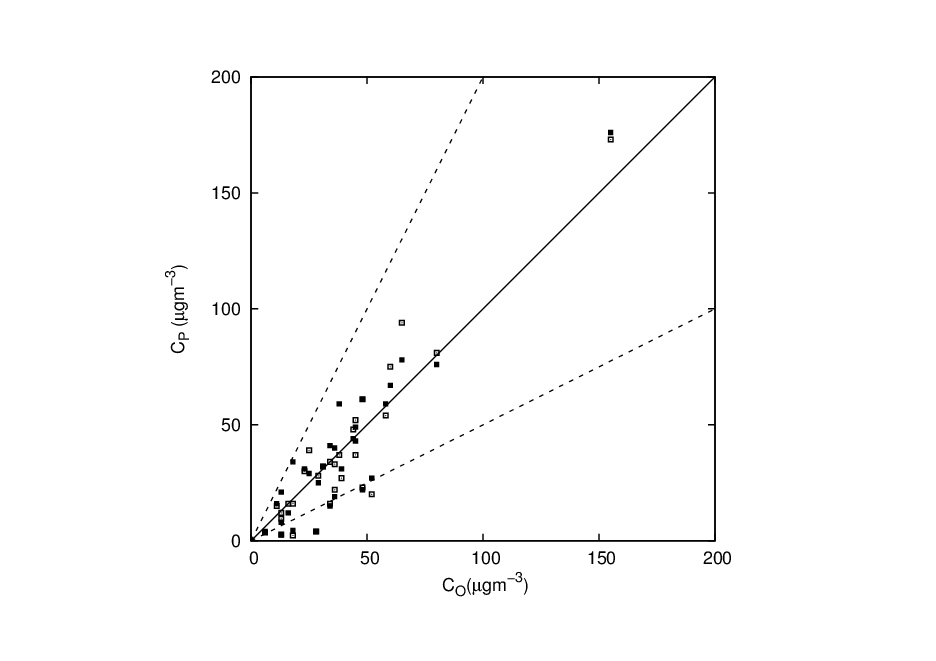 | Figure 1. Scatter diagram between predicted (Cp) and observed ground-level concentration (Co) for the INEL experiment. The solid squares represent the concentrations by [8] and the open square the new parameterization proposed by [4] |
These results show the importance of considering the parameters related to meandering in the simulations of the contaminant dispersion. The data between two dashed lines are in a factor of 2 (0.5 ≤ Co/Cp ≤ 2) with Cp the predicted concentration and Co the observed ground-level concentration for the INEL experiment.Table 2. Comparison between observed concentration and measured during the INEL experiment and predicted values from [8] and [4] 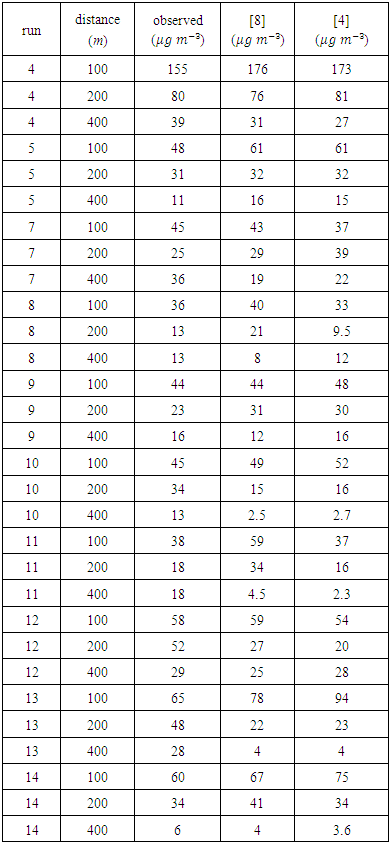 |
| |
|
Table 3 presents an analysis based on statistical indices [14]. The small positive values of statistical index FB, suggest that the models underestimate the mean observed concentrations. The FS indicates that the dispersion of the mean simulated concentrations overestimates the experimental data. Other indices, such as NMSE and FA2, are similar for both cases and it is an indication of a good agreement between models and observations. Furthermore, the correlation coefficient R, positive and near one, indicate that there is a good correlation between the observed and simulated values.Table 3. Statistical evaluation of the proposed simulation and [8] model with observed values 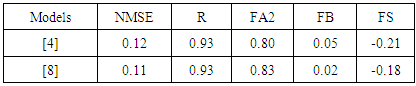 |
| |
|
5. Conclusions
In the low wind stable boundary layer, the contaminant dispersion is directly influenced by the meandering phenomenon, which is characterized by low frequency horizontal oscillations of horizontal wind. Therefore, the results of simulations accomplished during low wind speed periods depend of the meandering parameters inserted in the dispersion models. For the meandering dispersion we employ the meandering period and the loop parameter obtained from experimental data measured in Brazilian Amazon region in the ILS-LW model to simulate dispersion of contaminant. The concentrations obtained with the new observational meandering parameters were compared with the concentration measured data in the Idaho National Engineering Laboratory (INEL). The results presented and discussed are in agreement with experimental values found in the literature.
ACKNOWLEDGEMENTS
The authors would like to thank the CNPq (Conselho Nacional de Desenvolvimento Científico e Tecnológico) and Capes (Coordenação de Aperfeiçoamento de Pessoal de Nível Superior) for partial financial support of this work.
References
[1] | Anfossi, D., Oettl, D., Degrazia, G. A. and Goulart, A., 2005, An Analysis of Sonic Anemometer observations in Low wind speed conditions. Boundary- Layer meteorology, 114(1), 179-203. |
[2] | Degrazia, G. A., Goulart, A., Costa Carvalho, J., Szinvelski, C. R. P., Buligon, L. and Ucker Timm, A., 2008, Turbulence dissipation rate derivation for meandering occurrences in a stable planetary boundary layer, Atmospheric Chemistry and Physics, vol. 8, 1713–1721. |
[3] | Mortarini, L., Anfossi, D., 2015, Proposal of an empirical velocity spectrum formula in low-wind speed conditions, Q. J. R. Meteorol. Soc., vol. 141, pp. 85-97. |
[4] | Moor, L. P., Degrazia, G. A., Stefanello, M. B., Mortarini, L., Acevedo, O. C., Maldaner, S., Szinvelski, C. R. P., Roberti, D. R., Buligon, L. and Anfossi, D., 2015, Proposal of a new autocorrelation function in low wind speed conditions, Physica A: Statistical Mechanics and its Applications, vol. 292, 438-286. |
[5] | Goulart, A. G. O., Degrazia, G. A., Acevedo, O. C. and Anfossi, D., 2007, Theoretical considerations of meandering winds in simplified conditions, Boundary- Layer meteorology, 125(2), pp. 279-287. |
[6] | Anfossi D, Alessandrini S, Trini Castelli S, Ferrero E, Oettl D, Degrazia G., 2006, Tracer dispersion simulation in low-wind speed conditions with a new 2-D Langevin equation system, Atmos. Environ. vol. 40, 7234–7245. |
[7] | Anfossi D, Tinarelli G, Trini Castelli S, Ferrero E, Oettl D, Degrazia G, Mortarini L., 2010,Well mixed condition verification in windy and low-wind speed conditions, Int. J. Environ. Pollut. vol. 40, 49–61. |
[8] | Carvalho, J. C. and De Vilhena, M. T. M. B., 2005, Pollutant dispersion simulation for low wind speed condition by the ILS method, Atmospheric Environment, 39(34), 6282-6288. |
[9] | Carvalho, J. C., Vilhena, M. T. M. B., Degrazia, G. A. and Sallet, M., 2013, A General Lagrangian Approach to Simulate Pollutant Dispersion in Atmosphere for Low-wind Condition, American Journal of Environmental Engineering, 3(1), 8-12. |
[10] | Sagendorf, F. J. and Dickson, C. R., 1976, Difusion under low wind speed, inversion conditions, In Third symposium on atmospheric turbulence, difusion, and air quality, Preprints. |
[11] | Rodean, H.C., 1996 Stochastic Lagrangian. Models of Turbulent Diffusion, American. Meteorological Society, Boston, 84 pp. |
[12] | Frenkiel, F. N., 1953, Turbulence diffusion: Mean Concentration Distribution in a Flow field of homogeneous turbulence. Advances in applied mechanics, Academic Press, vol. 3, p. 61-107. |
[13] | Murgatroyd, R.J., 1969, Estimations from geostrophic trajectories of horizontal diffusivity in the mid-latitude troposphere and lower stratosphere, Quart. J. Roy. Meteorological Soc., 95, 40–62. |
[14] | Hanna, S. R., 1989, Confidence limits for air quality model evaluations as estimed by bootstrap and backife resampling methods, Atmos. Environ., vol. 23, 1385–1398. |