Phil Ligrani1, Danielle McDowell2, Shreyas Lakshmipuram Raghu1, L. Dale Thomas2
1Mechanical and Aerospace Engineering Department, University of Alabama in Huntsville, Huntsville, Alabama, USA
2Industrial & Systems Engineering and Engineering Management Department, University of Alabama in Huntsville, Huntsville, Alabama, USA
Correspondence to: Phil Ligrani, Mechanical and Aerospace Engineering Department, University of Alabama in Huntsville, Huntsville, Alabama, USA.
Email: |  |
Copyright © 2024 The Author(s). Published by Scientific & Academic Publishing.
This work is licensed under the Creative Commons Attribution International License (CC BY).
http://creativecommons.org/licenses/by/4.0/

Abstract
A structural evaluation method employed to provide components with minimal likelihood of failure, and to compensate for design shortcoming is structural margin or SM analysis. The SM approach is advantageous because the approach can potentially reduce the number of physical tests required to characterize a system. Within the present investigation, considered are the consequences of altering reduction factor parameters a and b, and a noise parameter c on structural margin performance parameters, which include yield margin YM, reliability boundary RB, yield stress ratio YSR, margin depth MD, and mean allowable stress MAS. The effects of reduction factors a and b, and the noise parameter c are considered for this analysis as they affect the mechanical strength characteristics of Al 2024-T6. The a and b reduction parameters are used in particular to quantify potential material property degradation of such a component. Three collections of test cases are considered with different values of the a, b, and c parameters, wherein one parameter is varied, while the remaining two are kept constant. Overall, the present results are the first to show that the structural margin performance parameters considered show complex dependencies as each of the a, b, and c parameters is varied independently.
Keywords:
Structural Margin Analysis, Yield Analysis, Reduction Factors, Statistical Noise, Material Property Degradation
Cite this paper: Phil Ligrani, Danielle McDowell, Shreyas Lakshmipuram Raghu, L. Dale Thomas, Structural Margin Statistical Analysis: Effects of Reduction Factors and Statistical Noise on Performance Parameters, International Journal of Statistics and Applications, Vol. 14 No. 3, 2024, pp. 41-46. doi: 10.5923/j.statistics.20241403.01.
1. Introduction
In the design and development of many engineering components, critical failures often occur because of the inadequacy of structural margins and material strength. Design programs typically adopt the Factor of Safety approach (FoS) to address these issues and to provide components with adequate safety characteristics. A FoS value is generally computed based upon an evaluation of the strength characteristics of a particular system or its components. As such, in general, Factor of Safety (FoS) is determined analytically as the ratio of a structure's absolute strength (structural capability) to actual applied load. As such, FoS gives a measure of the reliability of a particular design. Some sources refer to Factor of Safety determined in this manner as a Realized Factor of Safety. Note that NASA Strength and Life Assessment Requirements for Liquid-Fueled Space Propulsion System Engines provide guidelines for appropriate FoS values to compensate for design shortcomings and to provide components with minimal likelihood of failure [1]. Factor of Safety requirements are applicable to a variety of different aerospace components, including ones associated with rocket engines.To develop a FoS, test-to-failure (TTF) programs are additionally often employed to evaluate the structural capabilities of a system or its components [2]. These procedures are employed to gather strength data over a sufficient range of experimental conditions in order to provide a comprehensive assessment of possibility of failure. Test-fail-fix (TFF) procedures are one of many methods employed to troubleshoot complications and eliminate failure modes during the development of engineering components, especially ones which are employed within the aerospace industry [3,4,5]. TFF cycles involve rigorous testing early in the development of a component to reveal and address defects through progressive improvement. While insightful and useful, extensive application of TTF and TFF programs can heavily increase the overall cost and time spent advancing system development.Another structural evaluation method employed to provide components with minimal likelihood of failure, and to compensate for design shortcoming is structural margin analysis [6,7]. In contrast to set FoS values, structural margins or SM’s are expressed as the number of standard deviations present between the average strength and maximum stress applied. According to Lusser [2]. the principle of safety margins to provides increased design reliability and optimized performance by minimizing the potential for stress to exceed strength. He also indicates that, with the SM approach, characteristic variations of applied stress and material strength are used to generate suitable safety margins. According to Raghu et al. [8], many deficiencies are associated with Factor of Safety (FoS) analysis and design tools and approaches. As a consequence, these investigators provide and recommend SM based analysis procedures in order to improve upon more traditional Factor of Safety approaches. Such SM analysis methods aim to reduce structural failures through probabilistic determination of the stress-strain data, as employed with a stress-strength interference technique.The SM approach is advantageous because, rather than relying on the results of extensive TTF and TFF cycles, the approach can potentially reduce the number of physical tests required to characterize a system. This is achieved by employing an elastoplastic finite element model, Markov Chain Monte Carlo simulation, and a Bayesian inference module, which may all be executed prior to required testing. The combined results of these methods enable the calculation of safety margins and a reliability boundary, as outlined in Lusser’s [2] work, in addition to stress strength ratios which serve as a comparison to traditional FoS values. With the SM approach, material property data, which once required iterative physical testing, can now be obtained through probabilistic methods. In addition, when optimized using this approach, failures induced by applied stress exceeding material strength are completely avoided. The method also generates useful data that is predictive of material strength and load responses. Because data variation and dispersion are considered within the analysis, SM analysis can also lead to an improved understanding of the overall uncertainty associated with designs involving additively manufactured components. Use of such a SM tool may also lead to reduced rework cycles, streamlined verification, and long-term affordability.
2. Structural Margin Analysis
The first step of structural margin or SM analysis involves an elastoplastic finite element model to calculate the nominal parameters for Al 2024-T6. A simple 1D rod is used as the finite element. Incremental load application formulas are applied to characterize the response of the rod under uniaxial tensile loads. The resulting parameters include Young’s modulus E, yield stress Sy, strength Su, and fracture strain ϵu, where the first two of these parameters are the most useful for SM analysis. Considering the development and design of modern engineering components and systems, potential material property degradation is an important consideration. With the present analysis approach, such degradation is quantified by incorporating reduction parameters, a and b, as these are applied to Young’s modulus E and yield stress Sy. The use of these parameters is illustrated by the equations which are given by | (1) |
 | (2) |
where E* and Sy* are initial values, and E and Sy are values corrected by incorporation of the reduction parameters. Additionally, increased data variation is applied using the noise parameter, c, to model and simulate the scatter and uncertainties that are associated with physically obtained data. Based on given values for a, b, and c and data for the estimated properties of Al 2024-T6, a modified stress-strain curve is generated, and a Markov Chain Monte Carlo (MCMC) simulation is initiated. The purpose of the MCMC simulation is to obtain normal distributions of the reduction parameters a and b. Additional discussion of methods employed to determine statistical distributions of these parameters is provided by Wang [9] and Smith [10].A Bayesian inference module is then applied to determine distributions of Young’s modulus E and yield stress Sy. Employed within these modules is a numerical procedure called MCMC or Markov Chain Monte Carlo simulation [9,10,11]. Key ingredients for associated analysis steps are the dependence of yield strength Su on Sy, E, and fracture strain ϵu, and the dependence of allowable stress S on the same parameters, Sy, E, and ϵu. With these results in hand, additional distributions for the allowable stress S and yield strength Su are developed and compared to provide stress-strength interference information. The combined results of these techniques further enable the evaluation of performance metrics within the SM analysis. The allowable stress S for the structural margin based design is given by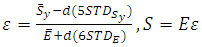 | (3) |
Distributions of allowable stress S and yield stress Sy are required in order to determine magnitudes of the reliability boundary RB and the yield margin YM. As described by Lusser [2], the reliability boundary RB for a component is the stress level which must be managed with complete reliability. This factor is equivalent to the average stress level plus six standard deviations relative to the average value. The yield margin YM is also based on Lusser’s [2] method of determining structural margins. This quantity is calculated using the average yield stress minus five standard deviations to address unreliability associated with data variation. Within the equations which follow, bar notation is representative of mean values, d is a correction factor based on sample size, and STD represents the standard deviation. With this approach, RB and YM are then determined using  | (4) |
and | (5) |
respectively. Within these equations,
is the mean allowable stress or MAS, and
is the mean yield stress. To address potential failures and characterize the interference of stress and strength distributions, useful is a margin depth MD variable. Also relevant is the yield stress ratio YSR, which is expressed as the ratio of the mean yield stress to the mean allowable stress MAS. MD and YSR are then given by | (6) |
and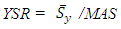 | (7) |
respectively. These quantities are useful in regard to the development and use of interference diagrams which present yield strength and allowable stress. An overlap of these distributions, when presented as they vary with dimensional magnitudes of yield strength and allowable stress, indicates the potential for failure. Such a potential is also indicated by magnitudes of MD which are less than 1.0. When MD is equal to 1.0, an overlap between the stress and strength distributions is not observed, which means that the potential for failure is much less. Magnitudes of yield margin YM and RB are then useful as they are employed to determine MD values. As mentioned, reliability boundary RB is the stress level a component must be able to manage with complete reliability.An example of such an interference diagram is shown in Figure 1. Presented are probability density function distributions of allowable stress S and yield strength Su, which are provided for respective reduction parameters a and b of 75 and 100 percent, and noise c value of 5 percent. These values of the a, b, and c parameters are employed because they are representative of aerospace application environments [8]. Within Figure 1, probability density function distributions of allowable stress and yield strength do not overlap, which indicates a low potential for component failure. As such, the associated MD value is 1.0, which is based upon YM=1.0772 and RB=1.0772. The resulting yield stress ratio YSR value is then 1.1265.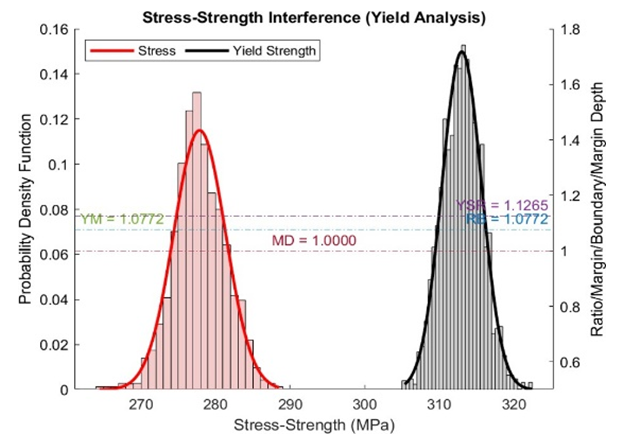 | Figure 1. Interference diagram with probability density function distributions of allowable stress S and yield strength Su for respective reduction parameters a and b of 75 and 100 percent, and noise c value of 5 percent |
3. Present Investigation
The present work considers the effects of reduction factors a and b, and added noise, as quantified with the c parameter, on the mechanical strength characteristics of Al 2024-T6. Note that variations of the noise parameter c models and simulates the scatter and uncertainties that are associated with physically obtained data. Of particular focus are the consequences of altering these parameters on yield margin YM, reliability boundary RB, yield stress ratio YSR, margin depth MD, and mean allowable stress MAS. As discussed, all of these parameters are related to values and variations of Young’s modulus E, yield stress Sy, strength Su, and fracture strain ϵu. Changing the a, b, and c parameters thus alters stress-strain relationships, and the performance parameters associated with SM analysis. Three collections of test cases are considered, wherein one parameter is varied, while the remaining two are kept constant. For the first test case set, a is set equal to 100, 95, 85, and 75 percent, b is maintained constant at 100 percent, and c is maintained constant at 5 percent. For the second test case set, a is maintained constant at 100 percent, b is set equal to 100, 95, 90, and 85 percent, and c is maintained constant at 5 percent. For the third test case set, a is maintained constant at 100 percent, b is maintained constant at 100 percent, and c is set equal to 15, 10, 7, and 5 percent.The present assessment of the influences of the a, b, and c parameters on SM performance metrics highlights the effectiveness and utility of this analysis approach in regard to determination of the probability of mechanical failure. As such, the present results provide insights into the physical effects of noise parameter c and reduction parameters a and b on the mechanical stress and strain characteristics of an Al 2024-T6 component. Information on consequences of changes to Young’s modulus E and yield stress Sy are thus also provided, as they influence mechanical structure stress and strain characteristics, as these are quantified using yield margin YM, reliability boundary RB, yield stress ratio YSR, margin depth MD, and mean allowable stress MAS.
4. Analytical Statistical Results
Examples which show characteristics of SM analysis metrics when the reduction parameters, a and b, and noise c are varied are illustrated by the data presented within Figures 2, 3, and 4. Each of these figures shows variations of mean allowable stress MAS (part a), yield margin YM (part b), yield stress ratio YSR (part b), reliability boundary RB (part c), and margin depth MD (part c). Within Figure 2, these data are provided as a is set equal to 100, 95, 85, and 75 percent, b is maintained constant at 100 percent, and c is maintained constant at 5 percent. Within Figure 3, these data are provided as a is maintained constant at 100 percent, b is set equal to 100, 95, 90, and 85 percent, and c is maintained constant at 5 percent. Within Figure 4, these data are provided as a is maintained constant at 100 percent, b is maintained constant at 100 percent, and c is set equal to 15, 10, 7, and 5 percent.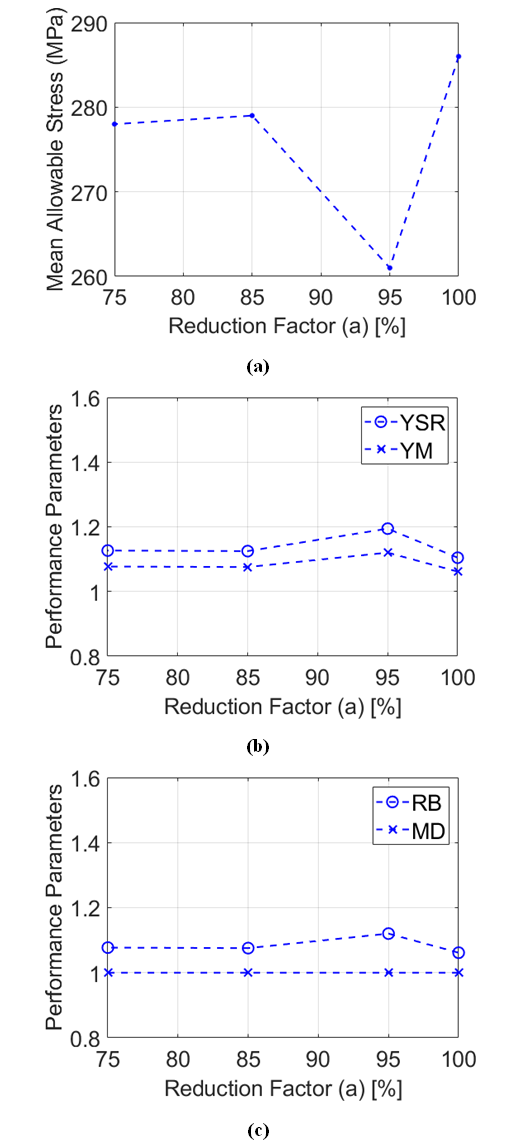 | Figure 2. Variations of mean allowable stress MAS (part a), yield margin YM (part b), yield stress ratio YSR (part b), reliability boundary RB (part c), and margin depth MD (part c) as a is set equal to 100, 95, 85, and 75 percent, b is maintained constant at 100 percent, and c is maintained constant at 5 percent |
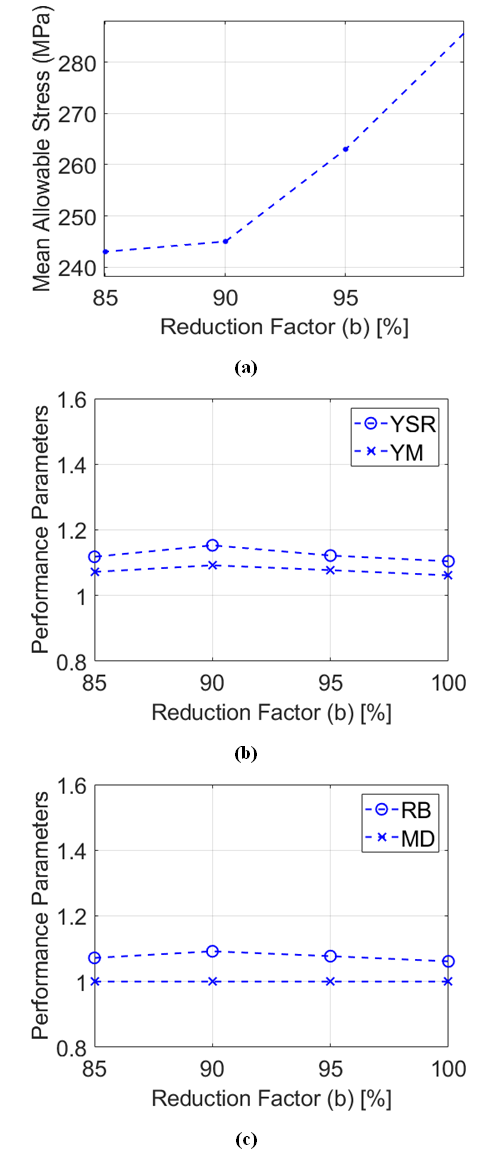 | Figure 3. Variations of mean allowable stress MAS (part a), yield margin YM (part b), yield stress ratio YSR (part b), reliability boundary RB (part c), and margin depth MD (part c) as a is maintained constant at 100 percent, b is set equal to 100, 95, 90, and 85 percent, and c is maintained constant at 5 percent |
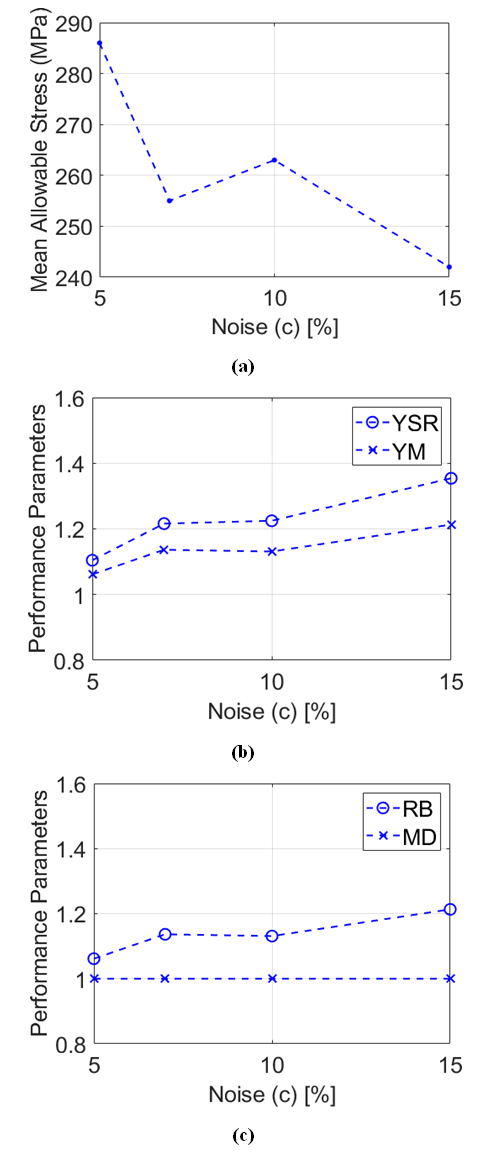 | Figure 4. Variations of mean allowable stress MAS (part a), yield margin YM (part b), yield stress ratio YSR (part b), reliability boundary RB (part c), and margin depth MD (part c) as a is maintained constant at 100 percent, b is maintained constant at 100 percent, and c is set equal to 15, 10, 7, and 5 percent |
Of particular interest are the complicated variations of mean allowable stress MAS with parameter a shown in Figure 2a. For example, data within this figure show that the mean allowable stress experiences a minimum at an a value of 95%. Such variations are present because different values of the reduction parameter a directly alter the behavior of the plastic region of the stress-strain characteristics of the material, and because of the complex dependence of allowable stress S upon Young’s modulus E, yield stress Sy, and fracture strain ϵu. Within Figure 3a, mean allowable stress MAS data consistently increase as parameter b increases. This behavior is a result of the direct application of the b reduction parameter to yield stress Sy, and the resulting relatively simple dependence of allowable stress S upon Young’s modulus E, yield stress Sy, and fracture strain ϵu with such an arrangement. Such MAS variations are also tied to increasing values of yield stress Sy, as Young’s modulus E remains approximately constant.Variations of mean allowable stress MAS with noise parameter c, shown in Figure 4a, also show relatively complex variations. Such characteristics illustrate the intricate influences of noise parameter c on allowable stress S, especially with a local MAS minimum value for a c value of 7%, and a local MAS maximum value for a c value of 10%. Overall, mean allowable stress MAS data values generally decrease as the noise level becomes larger. As a consequence, an increase in the YSR value with increasing noise level is also observed in Figure 4b. Note that yield margin YM and reliability boundary RB also generally become larger with increasing noise level in Figures 4b and 4c, respectively.Figures 2b, 2c, 3b, and 3c show that yield margin YM, reliability boundary RB, and yield stress ratio YSR have only small variations as reduction parameters a and b are altered. Local maximum values for all of these quantities are evident for an a parameter value of 95%. Lowest values of all of these parameters are present for a b parameter value of 100%. The associated YSR deviations are of course directly tied to corresponding variations of the mean allowable stress MAS shown in Figures 2a and 3a. Overall, the MD data shown in Figures 2c, 3c, and 4c show that the MD value is consistently equal to 1.0 for all experimental conditions. This means that YM and RB are also equivalent in value. Such behavior indicates that interference between allowable stress and yield strength is not present, and that the associated designs do not have the potential to fail as a result of excessive stress levels.
5. Summary and Conclusions
The present study considers structural margin or SM analysis, which is a structural evaluation method employed to provide components with minimal likelihood of failure, and to compensate for design shortcomings. The SM approach is advantageous because the approach can potentially reduce the number of physical tests required to characterize a system. The present work is focussed on the effects of reduction factors a and b, and added noise, as quantified with the c parameter, on the mechanical strength characteristics of Al 2024-T6. Here, reduction parameters, a and b are used to quantify potential material property degradation of the associated component. Of particular interest are the consequences of altering these parameters on structural margin performance parameters, which include yield margin YM, reliability boundary RB, yield stress ratio YSR, margin depth MD, and mean allowable stress MAS. Three collections of test cases are considered with different values of the a, b, and c parameters, wherein one parameter is varied, while the remaining two are kept constant. Overall, the structural margin performance parameters considered show complex dependencies as each of the a, b, and c parameters is varied independently. As such, the results presented within the present paper are the first within published literature which are determined using structural margin analysis to illustrate this dependence. Also provided are insights into the physical effects of these parameters on the mechanical stress and strain characteristics of an Al 2024-T6 component. The resulting assessment of the influences of the a, b, and c parameters on structural margin performance metrics highlights the effectiveness and utility of this analysis approach in regard to determination of the probability of mechanical failure. Note that the present analysis approach, as employed for structural margin performance analysis, is also applicable to other technical performance quantifications, including ones associated with electrical system power production variation with demand, and pointing accuracy capability variations with required accuracy.
ACKNOWLEDGEMENTS
Associate Professor Gang Wang is acknowledged for his guidance in regard to several aspects of the structural margin analysis techniques presented within the present paper.
References
[1] | National Aeronautics and Space Administration, “Strength and Life Assessment Requirements for Liquid-Fueled Space Propulsion System Engines,” NASA-STD-5012B, 2016. |
[2] | R., Lusser, “Reliability Through Safety Margins,” Redstone Arsenal, Huntsville, Alabama, USA, 1958. |
[3] | G., Havskjold, “Developing Innovative Products on Budget and On Schedule--Part 1: Identifying and Measuring Cost Drivers Correlates Technical Uncertainty with Rework Cycles,” 45th AIAA/ASME/SAE/ASEE Joint Propulsion Conference & Exhibit, 2009. https://doi.org/10.2514/6.2009-5436. |
[4] | D. E., Aldrich, “Study to Accelerate Development by Test of a Rocket Engine,” Rocketdyne Report R-8099, NASA-18734, 1969. |
[5] | C., Joyner, J., Laurie, D., Levack, E., Zapata, “Pros, Cons, and Alternates to Weight Based Cost Estimating,” 47th AIAA/ASME/SAE/ASEE Joint Propulsion Conference and Exhibit, 2011. https://doi.org/10.2514/6.2011-5502. |
[6] | B., Jena, “Limit State Design and Factor of Safety: An Overview,” Recent Developments in Sustainable Infrastructure, Vol. 75, pp. 201-211, 2021. https://doi.org/10.1007/978-981-15-4577-1_16. |
[7] | S. S., Afshari, C., Zhao, X., Zhuang, X., Liang, “Deep Learning-Based Methods in Structural Reliability Analysis: A Review,” Measurement Science and Technology, Vol. 34, 2023. https://doi.org/10.1088/1361-6501/acc602. |
[8] | S. L., Raghu, S. B., Rawlins, T., Neal, L. D., Thomas, “Demonstration of a Reliability-Driven Framework for Rocket Engine Development, Test, and Verification,” AIAA SciTech Forum, 2024. |
[9] | G. Wang, “Beam Damage Uncertainty Quantification using Guided Lamb Wave Responses,” Journal of Intelligent Material Systems and Structures, Vol. 29, No. 3, pp. 323–334, 2018. https://doi: 10.1177/1045389X17704911. |
[10] | R. C. Smith, “Uncertainty Quantification: Theory, Implementation, and Applications,” Society for Industrial and Applied Mathematics (SIAM), 2014. |
[11] | H. Rappel, L. A. A. Beex, J. S. Hale, S. P. A. Bordas, “Bayesian Inference for the Stochastic Identification of Elastoplastic Material Parameters: Introduction, Misconceptions and Insights,” arXiv e-prints, arXiv: 1606.02422, 2016. https://doi: 10.48550/arXiv.1606.02422. |