Muhammet Bekçi1, Mehmet Yılmaz2
1Sivas Cumhuriyet University, Faculty of Science, Department of Statistics and Computer Sciences, Sivas, Turkey
2Ankara University, Faculty of Science, Department of Statistics, Ankara, Turkey
Correspondence to: Muhammet Bekçi, Sivas Cumhuriyet University, Faculty of Science, Department of Statistics and Computer Sciences, Sivas, Turkey.
Email: |  |
Copyright © 2019 The Author(s). Published by Scientific & Academic Publishing.
This work is licensed under the Creative Commons Attribution International License (CC BY).
http://creativecommons.org/licenses/by/4.0/

Abstract
In this paper, a new method for constructing bivariate distributions with given marginals is proposed, based on a mixing two bivariate distributions. A new bivariate distribution family is introduced by adding an appropriate term with independence class of distribution. During this construction process, the model is not complicated. By choosing a base distribution from the same marginals we derive a new distribution around the independent class. We note that the new distribution has additional parameter which would provide additional flexibility in applications. The joint probability density, joint reliability and reversed hazard rate functions of the new bivariate distribution are obtained. Furthermore, it is established that construction of bivariate distributions by this method allows for some flexibility in the values of Spearman’s correlation coefficient.
Keywords:
Dependence, Bivariate Distribution, Spearman’s Rho, Fréchet Bounds
Cite this paper: Muhammet Bekçi, Mehmet Yılmaz, Construction of Bivariate Distribution by Mixing Positively Dependent and Negatively Dependent Distributions, International Journal of Statistics and Applications, Vol. 9 No. 4, 2019, pp. 122-127. doi: 10.5923/j.statistics.20190904.04.
1. Introduction
Univariate models are insufficient to explain random phenomena. Today, data such as drought, wind speed and rainfall are measured together with the variables that may affect them. With the development of technology, the construction of continuous bivariate distribution functions with given marginals has become an importance. When creating new bivariate distributions, models that can express high correlation are generally tried to be obtained. [2] introduced a method which based on the choice of pairs of order statistics of the marginal distributions. [8] studied on construction of continuous bivariate distributions that possesses the Positive Quadrant Dependence property. [13] introduced a generalization of Farlie-Gumbel-Morgentern (FGM) distribution family. They extend the maximal correlation coefficient for FGM family. Furthermore, [14] introduced bivariate and multivariate generalization of quadratic transmutation distribution family. Proposal of [14] draws our attention in particular. Because the transition from univariate case to bivariate or multivariate cases is not so easy. While in univariate case the real line is the complement of the probabilities, at least in the bivariate case these supplements are on the quadrant. There are some issues to overcome for the case of positive values of the transmutation parameter. However, marginals of this model are univariate transmuted distributions and it cannot detect independency. They proposed quadratic rank transmuted bivariate distribution as
, where
. Here, for
gives the base distribution
. However, if the base distribution is taken from independency case, i.e.,
, then
can be written as in the eq. (2) of [8] follows:
. For
,
cannot meet the conditions (4) and (5) proposed by [8]. Inspired by these studies, the contribution of the article is to propose a simpler but more useful model than the model introduced by [14]. Proposed model also includes both positive and negative values of the parameter as in FGM. Thus, the model gains some flexibility in modeling both positive and negative dependence. Furthermore, proposed model can detect independency. After giving the necessary conditions to construct a new distribution, Spearman's rank correlation coefficient is calculated on two illustrative examples and the usefulness of this family is discussed. Furthermore, some reliability properties are studied for this family.Let
denote the bivariate distribution function of
having continuous marginal cdfs
and
. Also, let
be the distribution family where the respective marginal are F and G. Then, according to the eq. (2) and the condition (3) of [8], we have the function
where
denotes survival function. This function meets the conditions (3)-(5) given by [8]. Hence, first mixture component distribution is
. Similar work of [8] given by [15] introduces some conditions for negatively dependent families. According to the eq. (2.1) and the condition (2.1) of [15], we have
. Except for the condition (2.3) of [15], this function meets the conditions (2.2) and (2.4) given by [15]. Distribution properties for the second mixture component
have not yet been provided. To overcome this issue, we have the following theorem. Theorem 1. Let
be a distribution function belongs to the distribution family
which is differentiable on
and
denote the joint probability density function. Then (i)
is a distribution function,(ii)
is a distribution function if
,
.Proof. (i) Multivariate distribution function must satisfy following properties: (P1)
(P2)
and
. For the simplicity
and 
.Obviously,
.(P3)
. For the simplicity, let
and
. Then
Now, by noting that positively dependence implies
, then we have
. Also, negatively dependence implies both
and
. Hence, we have
(ii) According to [4], Additionally to the properties (P1)-(P3), to determine bivariate distribution uniquely by its marginals bivariate distribution must lie upper and lower Fréchet bounds. Therefore, this idea explains why we need negative dependence for the construction of distribution given in (ii).(P1)
(P2)
and
.
Obviously,
.(P3)
.
Negatively dependence implies
. Hence, we have
However, if X and Y are positively dependent,
can not bigger than Fréchet lower bound. Therefore, the assumption of negative dependence is needed. To show this situation, we assume
. Then we have
As it can be seen that positivity of the above statement depends on
.According to Theorem 1, first mixture component distribution can be positively, negatively dependent or independent. But the second component distribution must be negatively dependent or independent. Thus, for the base distribution
, in order to be same structure for both mixture components, the random variables X and Y must be negatively dependent or independent. After this motivation, we can now propose the mixing of these two distributions as follows: Let T and V be negatively dependent (or independent) continuous random variables. Then their joint distribution function denoted as
belongs to the distribution family
where F and G denote respectively marginals of T and V. Let
and
respectively denote the distribution functions of
and
having the same marginals as H. The distribution functions of
and
are respectively defined by | (1) |
and | (2) |
where
denotes survival function of
i.e.,
. As can be seen immediately from equations (1) and (2),
and
are positively dependent random pairs, and
and
are negatively dependent random pairs.According to Therorem 1, we can define a new pairs of random variables X and Y as below:
Hence, the distribution of
obtained by mixing (1) and (2) which is given by | (3) |
By letting
, where
, eq. (3) can be rewritten as | (4) |
indicates
i.e., independence of X and Y,
indicates that X and Y negatively dependent, and
indicates positive dependence between X and Y. Note that X and Y are independent from each other, F indicates well-known bivariate distribution which is Farlie-Gumbel-Morgenstern distribution (see, [3] and [5]).We need the survival and probability density function for subsequent discussions. These functions are respectively given by
and
where
.
2. Reversed Hazard Rate of the New Family of Bivariate Distribution
The bivariate reversed hazard is defined by [1] as
. Analogously to the hazard gradient by [7], [11] defined the bivariate reversed hazard rate as follows:
, where
Reversed hazard rate gradients of
given by eq. (4) are as follows:
Accordingly, after some simplifications, bivariate reversed hazard rate can be given by 
3. Lower and Upper Bounds on Spearman’s Rho Measure for the New Family of Bivariate Distribution
This section deals with obtaining bounds for the bivariate distribution family given by the eq. (4). According to [6] and [4], for any bivariate distribution belonging to
contains Fréchet a lower bound and an upper bound. These bounds are respectively defined as  | (5) |
 | (6) |
For
, Spearman’s rho can be expressed as | (7) |
(see, [12]). The coefficient of Spearman’s rho for the new family can be obtained by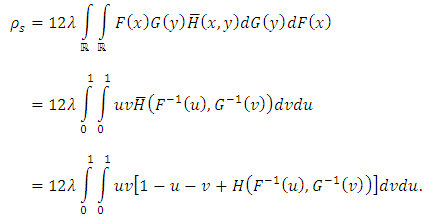 | (8) |
Hence, by using the eq. (5) for
, we have the lower bound as
To obtain the upper bound, we use the eq. (6), then
According to sign of
, we achieve the bounds as below:
We have two example to illustrate this family.Example 1. The Farlie-Gumbel-Morgenstern (FGM) family of bivariate distributions are given by
, for
. By taking
, the distribution
is given by
where
and
. Hence,
. Since
. One can conclude that this family model weak dependence as FGM does.Example 2. The bivariate Gumbel- Exponential (BGE) distribution is given by
, for
. The distribution
is given by
, where
and
. According to [9], the Spearman’s rho coefficient of BGE distribution is
, where
is the exponential integral function. After some algebraic manipulation,
can be obtained as
We calculate approximates values of
and
by using Maple with respect to some values of
. Tabulated values are given as Table 1 below:Table 1. Approximate Values of Spearman's Rho for BGE and F 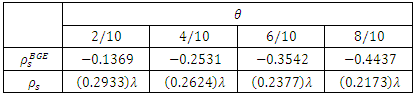 |
| |
|
For the proposed bivariate distribution families, it is generally expected to model a stronger correlation structure between two random variables. The distribution derived from the base of FGM can only reach the correlation structure that FGM can model. But, unlike in Example 1, as can be seen from the Table 1, it can be said that F can model negative dependence slightly better than BGE in small theta values. Then, we can choose this distribution as an alternative to BGE to provide a correlation structure between random variables.
4. Conclusions
In this study, we proposed a new bivariate distribution using a base distribution from the negative dependency class which is in
. Thus, this new distribution can reveal both negative dependence, positive dependence and independence between the random variables X and Y. The upper and lower bounds show that the values of the correlation coefficient for this family lies in the interval
. Besides, as a result of illustrative examples, it can be said that distributions can be derived for pairs of random variables with higher correlations considering some base distributions. For further discussion, focusing on the negative dependence condition on
, a new distribution family can be derived with any distribution function from
.
ACKNOWLEDGEMENTS
We thank anonymous referees for their valuable suggestions and constructive comments which helped us in revising the paper in the present form.
References
[1] | Bismi, G., Bivariate Burr Distributions, unpublished PhD Thesis, Cochin University of Science and Technology, 2005. |
[2] | Dolati A. and Ubeda-Flores M., Constructing Copulas by Means of Pairs of Order Statistics, Kybernetika, 45-6, (2009), 992-1002. |
[3] | Farlie D., The Performance of Some Correlation Coefficients for a General Bivariate Distribution, Biometrika, 47-3/4, (1960), 307-323. |
[4] | Fréchet M., Sur Les Tableaux de Corrélation Dont Les marges Sont Donnees, Annales de l’Université de Lyon, Sciences 4, (1951), 13–84. |
[5] | Gumbel E. J., Bivariate Exponential Distributions, Journal of the American Statistical Association, 55-292, (1960), 698-707. |
[6] | Hoeffding W., Masstabinvariante Korrelationstheorie, Schriften des Mathematischen Instituts und Instituts fur Angewandte Mathematik der Universitat Berlin, 5, (1940), 181-233. |
[7] | Johnson N. L. and Kotz S., A Vector Multivariate Hazard Rate, Journal of Multivariate Analysis, 5-1, (1975), 53-66. |
[8] | Lai C. D. and Xie M., A New Family of Positive Quadrant Dependent Bivariate Distributions, Statistics and probability letters, 46-4, (2000), 359-364. |
[9] | Yela P.J. and Cuevas T.J.R., Estimating the Gumbel-Barnett Copula Parameter of Dependence, Revista Colombiana de Estadística, 41-1, (2018), 53-73. |
[10] | Richard E.B. and Proschan F., Statistical Theory of Reliability and Life Testing: Probability Models, Holt, Rinehart and Winston, 1975. |
[11] | Roy D., A Characterization of Model Approach for Generating Bivariate Life Distributions Using Reversed Hazard Rates, Journal of Japan Statistical Society, 32-2, (2002), 239–245. |
[12] | Schweizer B. and Wolff E., On Nonparametric Measures of Dependence for Random Variables, The Annals of Statistics, 9-4, (1981), 879-885. |
[13] | Bairamov I. Kotz S. and Bekci M., New generalized Farlie-Gumbel- Morgenstern distributions and concomitants of order statistics. Journal of Applied Statistics, 28-5, (2001), 521-536. |
[14] | Bourguignon M. Ghosh I. and Cordeiro G. M., General results for the transmuted family of distributions and new models. Journal of Probability and Statistics. Vol. 2016, Article ID 7208425, 12 pages, http://dx.doi.org/10.1155/2016/7208425. |
[15] | Han Kwang-Hee., A new family of negative quadrant dependent bivariate distributions with continuous marginal, Journal of the Chungcheong Mathematical Society, 24-4, (2011), 795-805. |