Oyenuga I. F.1, Oyekunle J. O.2, Agbona A. A.2
1Department of Mathematics and Statistics, The Polytechnic, Ibadan, Nigeria
2Department of Statistics, Federal Polytechnic, Ede, Nigeria
Correspondence to: Oyenuga I. F., Department of Mathematics and Statistics, The Polytechnic, Ibadan, Nigeria.
Email: |  |
Copyright © 2019 The Author(s). Published by Scientific & Academic Publishing.
This work is licensed under the Creative Commons Attribution International License (CC BY).
http://creativecommons.org/licenses/by/4.0/

Abstract
An exchange rate is the value of a country’s currency in relation to another country or economic zone. Most exchange rates are free-floating and will rise or fall based on supply and demand in the market. Various countries use different mechanisms to keep their currency stable by identifying an exchange rate regime that best suits their economy. Monetary policy has always been seen as a fundamental instrument over the years for the attainment of macroeconomic stability which is often seen as a prerequisite to achieving sustainable growth of output. The main aim of this paper is to model the exchange rate of Naira against four other major currencies using ARIMA model. The currencies modelled were Dollar, Pounds Sterling, Euro and Swiss Franc. The annual time series data used for the study were extracted from 2018 Central Bank of Nigeria, Statistical Bulletin between 1999 to 2017. The results show that Dollar, Pounds, Euro and Swiss franz time series data were satisfactorily stationary after the second difference and followed ARIMA (1 2 1), (2 2 1) (2 2 1) and (2 2 2) respectively with corresponding minimum AIC of 51.122, 55.370, 71.027 and 73.819. The errors derived from the models were random, normally distributed and no presence of error correlation which indicates that the residuals are white noise. The fitted models are used to make forecasts for four years and the results indicate perpetual increase in the exchange rate.
Keywords:
ARIMA, Modeling, Exchange Rate, Monetary Policy, AIC, White noise
Cite this paper: Oyenuga I. F., Oyekunle J. O., Agbona A. A., Modeling the Exchange Rate of the Nigeria Naira to Some other Major Currencies, International Journal of Statistics and Applications, Vol. 9 No. 3, 2019, pp. 67-73. doi: 10.5923/j.statistics.20190903.01.
1. Introduction
Exchange rate policy has been identified as one of the endogenous factors that can affect the economic performance of a nation [1]. Exchange rate is the price of one country’s currency in relation to another country. It is the price in which one currency is exchange for another. It measures the domestic worth of an economy; in terms of the currencies of most industrialized countries such as United State of America Dollars, British Pound Sterling, German Duetsche Mark, Swiss Franc, French Frank, Italian Lira and Canadian Dollar [2].In Nigeria, the management of exchange rate is carried out by the Central Bank of Nigeria. Following the adoption of the structural adjustment program policy in 1986, the country has moved from a pegged or rigid exchange rate regime to a more flexible regime [3]. In practice, no exchange rate is ‘clean or pure float’, that is a situation where the exchange rate is left completely to be determined by market forces of demand and supply but rather the prevailing system is the managed float whereby the monetary authorities intervene periodically in the foreign exchange market of a country in order to attain some strategic objectives [4].The inconsistency in policies and lack of continuity in exchange rate policies aggregated unstable nature of the naira rate [5]. [6,7] noted that despite various efforts by the government to maintain a stable rate, the naira has depreciated throughout the 80’s to date.Most researchers have done a great research on forecasting of exchange rate of developed and developing countries using different approaches. The approach might vary in either fundamental or technical approach. [8] used a technical approach to forecast Nigeria naira to US Dollar using Seasonal ARIMA model for the period of 2004 to 2011 and was stable in 2008. His good work expatiates on that seasonal difference once produced a series SDNDER with slightly positive trend but still within discernible stationarity. [9] conducted a research on forecasting exchange rate between Ghana Cedis and US Dollar using Time Series analysis for the period January 1994 to December 2010. Their findings reveal that predicted rates were consistent with the depreciating trend of the observed series and ARIMA (1 1 1) was found to be the best to such series and a forecast for two years were made from January 2011 to December 2012 and reveals that a depreciation of Ghana Cedi’s against the US Dollar was found.[10] examined the volatility of the Naira/USD and Naira/UK Pound Sterling exchange rates in Nigeria using the GARCH model. They use monthly exchange rates spanning the period 2006-2010. According to them, the main objective of an exchange rate policy is to determine an appropriate exchange rate and ensure its stability over the years, efforts put by the Nigerian Government to achieve this have not yielded positive results. They thus sought to build a forecasting model that would adequately capture the volatility of Nigeria Exchange rate return series using GARCH model and the outcome of their research was to assist the government to manage the exposure of the exchange rate volatility in short run and inform investors on future behavior of exchange rates thus helping them in decision making.[11] forecasting exchange rate between Naira and US Dollar using time domain model. The study used non seasonal ARIMA model for the period January 1994 to December 2011. The result revealed that the model ARIMA (1 2 1) best fit the data.[12] examined the naira exchange rate in relation to four other major currencies. The impact of exogenous variables in modeling volatility was considered using both the GARCH (1 1) and its asymmetric variants. The results of the fitted models show that some of the parameters were significant and that volatility is quite persistent while the results of the asymmetric model indicate different impacts for both negative and positive shocks. This shows superior forecasting performance to the symmetric GARCH.[13] investigated the strength of Nigeria Naira to US Dollar using Box Jenkins approach, data spans the period 1972 to 2014. The best model that explains the series was found within the confidence limits and this suggests that if positive measure is not taken, the value of naira will continue to depreciate.[14] presented an empirical study of modelling and forecasting time series data of official exchange rate of Nigeria using Box-Jenkins ARIMA methodology. The result of the analysis revealed that the best model for forecasting Dollar to Naira is ARIMA (0 1 0) obtained through the use of the function auto.arima.[15] model and forecast the naira to US Dollar exchange rate over the period 1960 to 2017. The best model that fit the series is ARIMA (1 1 1) which is used to forecast. The result shows that naira will continue to depreciate and it is recommended for Central Bank of Nigeria to devalue naira in order to not only restore exchange rate stability but also promoting foreign capital inflows.[16] presented modeling the exchange rate volatility of Naira against Euro, Pound Sterling, Dollar and West African Unit of Account (WAUA) in Nigeria using symmetric and asymmetric GARCH models in the presence of Gaussian and Non-Gaussian errors. The results show that symmetric GARCH (1 1) model captured all volatility clustering with evidence of shock persistence in the four exchange rate return series. The asymmetric EGARCH (1 1) and TGARCH (1 1) models produced responses and leverages effects in the four exchange rates log return series suggesting that positive shocks produces more volatility in Nigerian foreign exchange market than positive shocks of the same magnitude.
2. Material and Methods
The data used for the study was extracted from Central Bank of Nigeria (CBN), Statistical Bulletin between 1997 and 2016 spanning twenty year on annual cross Exchange rate of the naira to four other major currencies namely: US Dollar, British Pound Sterling, Euro and Swiss Franc.A. Time Series Modelsi. Autoregressive Process (AR)A time series Xt is said to follow an autoregressive process of order P i.e AR(P), if it is a weighted sum of the past P values plus a random shock so that.
The value at time t depends linearly on the last p values and the model looks like a regression model hence, the term autoregression. Using the backward shift operator B such that
the AR(P) model may be re-written as
Hence
where
ii. Moving Average Process (MA)A process is said to be a moving average process of order q. MA (q) if it is a weighted sum of the last random shocks, that is
Using the backward shift operator B, it may be written as
Hence,
where,
iii. Autoregressive Moving Average Processes (ARMA)This is a mixed Autoregressive Moving Average model with p, AR terms and q, MA terms, that is ARMA (p,q). This is denoted by:
where
are polynomials in B of finite order p,q respectively.iv. Autoregressive Integrated Moving Average Process (ARIMA)If the original data series is differenced the ‘d’ times before fitting an ARMA (p,q) process, then the model for the original differenced series is said to be an ARIMA (p. d, q) process. The I stands for integrated factor and denotes the number of difference taken.It is generalized as
ARIMA models focus on how to predict the conditional mean of future values based on current and past data.B. Model Selection CriteriaThe Alkaike Information Criterion (AIC) (1973) is used for model selection. The criterion says to select the model that minimizes:
Where
if the model contains an intercept or a constant term and
if otherwiseThe addition of the
serves as a ‘penalty function’ thus ensuring the selection of a parsimonious model.k is the number of parameters in the model. The value of k yielding the minimum AIC specifies the best model. The lower the AIC value, the better the model fit. AIC balances the error of the fit against the number of the parameters.C. Box-Jenkins MethodologyBox-Jenkins (1973) developed a practical approach to build ARIMA model, which is best fit a given time series and also satisfy the parsimony principle. Their concept has fundamental importance on the area of time series analysis and forecasting. The Box-Jenkins methodology does not assume any particular pattern in historical data of the series being forecasted. Rather, it uses a three step iterative approach of model identification, parameter estimation and diagnostic checking to determine the best parsimonious model from general class of ARIMA models.
3. Analysis and Results
The time series data was subjected to stationary test using Augumented Dickey-Fuller test after plotting the time plot graph to observe the behavior of the series. Data was analyzed using Statistical Package for Social Science (SPSS) version 20.Table 3.1. Exchange rate of Nigeria Naira to other major currencies from 1999 to 2017 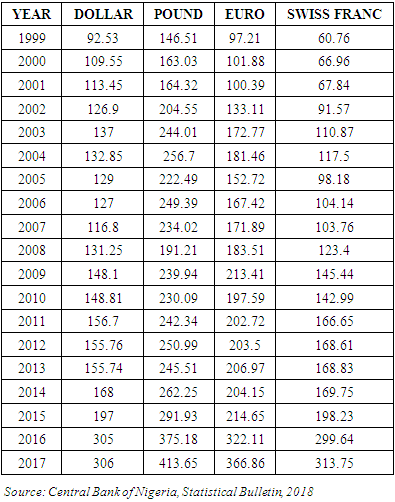 |
| |
|
The time plot shows that the time series are not stationary since it shows upward movement (trend). This indicates that the mean of exchange rate in Nigeria is changing with unstable variance of time series data.Table 3.2. Augumented Dickey-Fuller (ADF) test for Dollar and Pounds 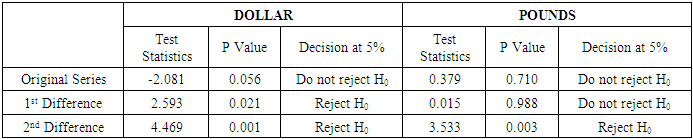 |
| |
|
Table 3.3. Augumented Dickey-Fuller (ADF) test for Euro and Swiss Franz 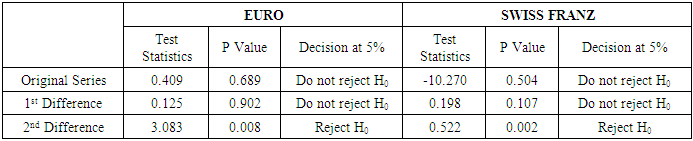 |
| |
|
Table 3.2 and 3.3 shows the result of ADF test for unit root of Nigeria Naira to Dollar, Pounds, Euro and Swiss franz. The results indicate that the time series data are stationary after the second difference I (2) except in the case of Dollar that was marginally stationary at first difference but satisfactorily stationary at the second difference. Therefore, dollar time series data is both integrated at both I (1) and I (2) to compute various ARIMA models and the best model is based on minimum AIC, SE and Log likelihood.A. Model IdentificationSince the Dollar time series data is marginally stationary after the first difference and highly stationary after the second difference, therefore, the tentative ARIMA models considered are (0 1 1), (1 1 0), (1 1 1), (1 1 2), (2 1 1), (2 1 2), (0 2 1), (1 2 0), (1 2 1), (2 2 0), (0 2 2), (1 2 2), (2 2 1) and (2 2 2). The corresponding Akaike Information Criterion (AIC), Standard Error (SE) and Log likelihood are respectively tabulated below.Table 3.4. Tentative ARIMA models for Dollar 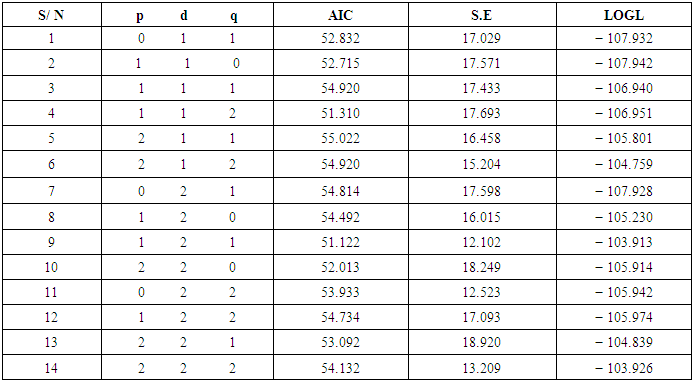 |
| |
|
Table 3.4, shows various ARIMA models for Dollar. Using the model selection criteria, that is, AIC and SE. ARIMA (1 2 1) is the best model because it has the smallest AIC and SE.Since the Pounds, Euro and Swiss Franz time series data are stationary after the second difference, therefore, the tentative ARIMA models considered are (0 2 1), (1 2 0), (1 2 1), (2 2 0), (0 2 2), (1 2 2), (2 2 1) and (2 2 2). The corresponding Akaike Information Criterion (AIC), Standard Error (SE) and Log likelihood are respectively tabulated below.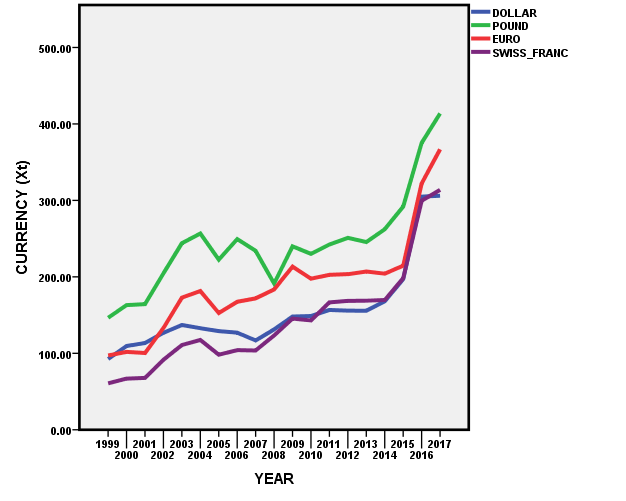 | Figure 1. Time Plot of Observed Values of all the major currency |
Table 3.5. Tentative ARIMA Models for Pounds, Euro and Swiss Franz 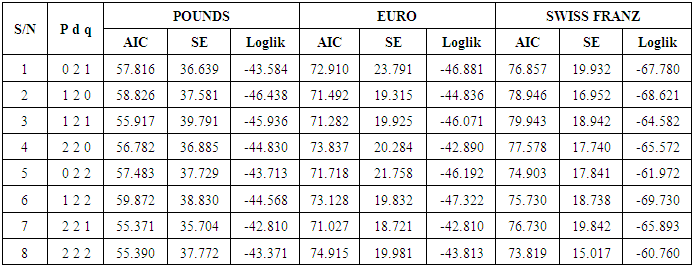 |
| |
|
Table 3.5, shows various ARIMA models for Pounds, Euro and Swiss Franz. Using the model selection criteria, AIC and SE, ARIMA (2 2 1), (2 2 1) and (2 2 2) are the best models for Pounds, Euro and Swiss Franz respectively.B. Parameters EstimationTable 3.6. ARIMA Models with their respective estimates for the four major currencies 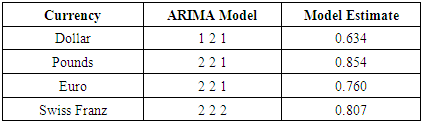 |
| |
|
The table 3.6 above shows the model estimations, where the parameters estimate conform to the stationary conditions since all the model estimates are < 1.C. Diagnostic checkingTable 3.7. The Ljung Box Test 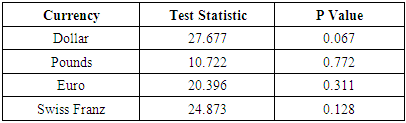 |
| |
|
Table 3.7 above shows the results for testing the residual white noise in optimal model. The errors derived from the models were normally distributed, random and no presence of errors correlation since the P values > 0.05. Therefore, the residuals of ARIMA models satisfied the characteristics of white noise process. D. Models FittingThe fitted model for the currencies can be written as follows:Dollar having ARIMA (1 2 1)
Open the brackets
Transforming the back operator
Make
the subject of the formular
Pounds and Euro having ARIMA (2 2 1), therefore the model can be written as follows
Open the brackets, multiply
by the LHS and
by the RHS
Transforming the back operator and make
the subject of the formular
Swiss franz that follows ARIMA (2 2 2), the model can be written as
Open the brackets, multiply
by the LHS and
by the RHS
Transforming the back operator and make
the subject of the formular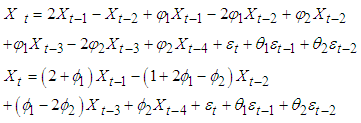
Table 3.8. Forecasts of Exchange rate for 4 years 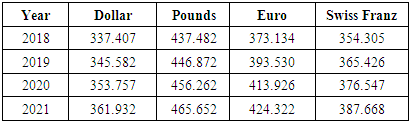 |
| |
|
The result in table 3.8 shows upward movement in the forecasts of naira from 2018 to 2021 from the models obtained. This means that naira will continue to depreciate unless an adequate measure is taken by the Central bank of Nigeria to prevent it.
4. Conclusions
The results of the analysis show that the time series data of all the currencies became stationary at second difference except for Dollar who was slightly stationary at first difference but satisfactorily stationary at the second difference. The diagnostic checking also confirmed that ARIMA (1 2 1), ARIMA (2 2 1), ARIMA (2 2 1) and ARIMA (2 2 2) are appropriate for Dollar, Pound, Euro and Swiss Franz respectively based on the minimum SE, Log likelihood and AIC. The residual of the model is white noise. The optimal models obtained are used to make forecasts from 2018 to 2021 which indicate perpetual increase in the exchange rate.
References
[1] | Jameela, O. Y (2010). Exchange rate changes and output performance in Nigeria. Pakistan Journal of Social Sciences. 7(5):380-387. |
[2] | Akpan, E. O and Atan, A (2012). Effects of Exchange rates movements on economic growth in Nigeria. Journal of Applied Statistics. 2(2):80-98. |
[3] | Azeez, B. A., Kolapo, F. T and Ajayi, L. B (2012). Effect of exchange rate volatility on macroeconomic performance in Nigeria in Nigeria. Interdisciplinary Journal of Contemporary Research in Business. 4(1):149-155. |
[4] | Mordi, C. N (2006). Challenges of exchange rate volatility in economic management in Nigeria. CBN Bulletin. 30(5):17-25. |
[5] | Gbosi, A. N (2005). Money, monetary policy and economy. Port Harcourt: Sodek. |
[6] | Augustine, C. O (2015). Exchange Rate Fluctuation, Oil prices and Economic Performance: Empirical Evidence from Nigeria. International Journal of Energy Economy and Policy. 5(2):502-506. |
[7] | Aliyu, S. R (2011). Impact of Oil Price Shock and Exchange Rate Volatility on Economic growth in Nigeria: An Empirical Investigation. Research Journal of International Studies. 11:103-120. |
[8] | Ette, H. E (2012). Forecasting Nigeria Naira-US Dollar exchange rate by a seasonal ARIMA model. American Journal of Scientific Research. 59:71-78. |
[9] | Appiah, S. T and Adetunde, I. A (2011). Forecasting exchange rate between the Ghana Cedi and US Dollar using time series analysis. Current Research Journal of Economic Theory. 3(2):76-83. |
[10] | Awoyemi, C and Alagbe, S (2011). Empirical modelling of Nigerian exchange rate volatility. Mathematical Theory and Modelling. 1(3):8-15. |
[11] | Onasanya, O. K. and Adeniji, O. E. (2013). Forecasting of exchange rate between Naira and US Dollar using time domain model. International Journal of Development and Economic Sustainability. 1(1):45-55. |
[12] | David, A. K and Augustine, A. A (2018). Modelling volatility dynamics of foreign exchange rates in Nigeria: Application of Univariate GARCH models. Journal of Scientific and Engineering Research. 5(11):50-62. |
[13] | Mohammed, M. B and Abdulmuahymin, S. A (2016). Modelling the exchange ability of Nigeria Naira currency with respect to US Dollar. International Journal of Scientific and Engineering Research. 7(7):86-104. |
[14] | Ajao, I. O., Obafemi, O. S and Bolarinwa, I. A (2017). Modelling Dollar-Naira exchange rate in Nigeria. Nigeria Statistical Society, Edited Conference Proceedings. 1(1):191-198. |
[15] | Thabani, N (2018). Modelling and forecasting Naira/USD exchange rate in Nigeria: a Box-Jenkins ARIMA approach. Munich Personal RePEc Achive MPRA. Paper No:88622: 1-36. |
[16] | David, R. O., Dikko, H. G and Gulumbe, S. U (2016). Modelling volatility of the exchange rate of the naira to major currencies. CBN Journal of Applied Statistics. 7(2):159-18. |
[17] | Box, G.E.P and Jenkins, M, (1976). Time Series analysis forecasting and control. Holden –Day Inc. |
[18] | Chris Chatfield (2001). Time Series Forecasting. Chapman and Hall CRC, London. |