Mugotitsa B.1, Samba B.2, Mung’atu J.2
1Department of Statistics and Actuarial Sciences, Jomo Kenyatta University of Agriculture and Technology, Nairobi, Kenya
2Department of Mathematics and Statistics, Maseno University, Kisumu, Kenya
Correspondence to: Mugotitsa B., Department of Statistics and Actuarial Sciences, Jomo Kenyatta University of Agriculture and Technology, Nairobi, Kenya.
Email: |  |
Copyright © 2018 The Author(s). Published by Scientific & Academic Publishing.
This work is licensed under the Creative Commons Attribution International License (CC BY).
http://creativecommons.org/licenses/by/4.0/

Abstract
HIV, malaria and anemia in sub-Saharan Africa were associated with problematic pregnancy and infant mortality. Researchers in the medical field have extensively studied the effects of maternal HIV, Malaria and Anemia on neonatal and postnatal mortalities. With interventions such as Prevention of Mother to Child Transmission (PMTCT) of HIV, free insecticide treated mosquito nets to curb Malaria, and Iron Folic Acid Supplements (IFAS) to curb anemia in pregnant mothers, there is need for research to determine whether the interventions have been effective. This study helps to know the extent of success of the interventions over recent years. The outcome of this study will help to improve the quality of antenatal care in Kenya and possibly other developing countries. The Cox proportional hazard model was used together with correlation and regression analysis and test of hypothesis tools, to determine relationships between the levels of effect before and after interventions. Secondary data for the period A (2001 – 2008) and B (2009 – 2015) on infants born to 1325 and 1270 HIV seropositive mothers respectively in Kisumu County - Kenya were extracted and analyzed. HIV status, Malaria (parasitemia or clinical malaria) and anemia (hemoglobin level <8 g/dL) are the key independent repressors to the response ‘infant mortality’. In group A, infant mortality was 145 (sd = 11.21) deaths per 1000 live births (LBs) while in B, it dropped significantly to 106(13.08). Among the 272 HIV positive infants in group A, 42% died while 29% of 278 in group B did not survive. Among the 1053 HIV negative infants in group A, 7% died while 5% of 992 in group B passed on. There was significant decrement in deaths between the periods. This can be attributed to the government interventions.
Keywords:
Cox Proportional Hazard Model, Survival Analysis, Post Neonatal Infant Mortality (PNIM), Prevention of Mother to Child Transmission (PMTCT)
Cite this paper: Mugotitsa B., Samba B., Mung’atu J., Cox-Proportional Hazard Model for Survival Analysis of Newborns among HIV Seropositive, Anaemic and Malaria Infected Pregnant Women in Kisumu County, International Journal of Statistics and Applications, Vol. 8 No. 5, 2018, pp. 219-225. doi: 10.5923/j.statistics.20180805.01.
1. Introduction
Background of StudyChild mortality, commonly on the agenda of public health and international development agencies, has received renewed attention as a part of the United Nation’s Millennium Development Goals (MDG). Approximately 10 million infants and children under five years of age die each year, with large variations in under-five mortality rates, across regions and countries [1, 2, 4].A great deal of these gains achieved through interventions targeted at communicable diseases (diarrhea, respiratory infections, malaria, measles and other immunisable childhood infections). However these health gains were short lived. In the mid 1970’s the worldwide progress not maintained and infant mortality rates raised especially in Africa because disease-oriented vertical programmes were not effective alone. Maternal, environmental, behavioural and socio-economic factors were recognized as additional important determinants of infant survival. According to (UNICEF 1999), the decline in child mortality in Africa has been slower since 1980 than in the 1960s and 1970s. Of the thirty countries with the world’s highest child mortality rates, twenty-seven were in sub-Saharan Africa. The region’s under-five mortality in 1998 were 173 per 1000 live births (UNICEF, 2000) compared to the minimum goal of 70/1000 internationally adopted in the 1990 World Summit for Children [2, 4].Although enormous literature exists on child mortality, evidence on why infant and child mortality rates remain high in many sub-Saharan African countries despite action plans and interventions made is still scanty. As the world enters into the 21st century, debate on childhood mortality remains a big issue for developing countries. Their commitment is reflected in their desire to reduce the level of child mortality by two- thirds of their 1990 levels by the year 2015, as expressed in the Millennium Development Goals (MDG). To achieve this goal, it is imperative to attempt and determine what factors contribute to the high levels of child mortality in developing countries, and Kenya, in particular.HIV transmission from mother to child during pregnancy, labour and delivery, or breastfeeding is known as perinatal transmission and is the most common route of HIV infection in children [6]. When HIV is diagnosed before or during pregnancy, perinatal transmission can be reduced to less than 1% if appropriate medical treatment is given, the virus becomes undetectable, and breastfeeding is avoided. Since the mid-1990s, HIV testing and preventive interventions have resulted in more than a 90% decline in the number of children prenatally infected with HIV in the United States (CDC 2012).Childhood Mortality in KenyaInfant mortality rate is the number of infants dying before reaching one year of age, per 1,000 live births in a given year [5]. Although accurate information on cause of death is lacking, the leading cause of under-five mortality in Kenya is pneumonia, malaria, measles and diarrheal disease, which were estimated to have been responsible for some 60 percent of disease burden in the region [4, 8] Kenya experienced dramatically fall in child mortality in the late 1940’s and early 1960’s. Until around 1980, the under -five mortality rate fell at an annual rate of about 4 percent per annum. This rate of decline slowed in the early 1980s to about 2 per cent per annum. Data from the 1998 Kenya Demographic and Health Survey (NCPD, 1989) show that, far from declining, the under-five mortality rate increased by 25 percent from the late 1980s to the mid-1990s. The recent Kenya Demographic and Health Survey (CBS, 2004) shows that under- five mortality rate is 115 deaths per 1,000 live births (see Table 1).Table 1. Levels and Trends of Childhood Mortality in Kenya 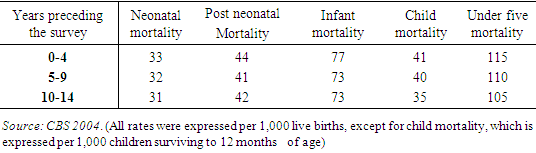 |
| |
|
The table above shows the infant and under-five mortality rates for each of the three five-year periods preceding the 1998 KDHS and the 2003 KDHS. The use of rates for five-year periods conceals any year-to-year fluctuations in early childhood mortality. For the most recent five-year period preceding the survey, infant mortality is 77 deaths per 1,000 live births, and under-five mortality is 115 deaths per 1,000 live births. This means that one in every nine children born in Kenya dies before attaining his or her fifth birthday. This pattern shows that 29% of deaths under-age five occur during the neonatal period while 38% occur during the post-neonatal period.The perinatal period commences at the 22 completed weeks (154 days) of gestation (the time when the birth weight is normally 500g), and ends seven completed days after birth. The neonatal period commences at birth and ends 28 completed days after birth. Neonatal deaths (deaths among live births during the first 28 completed days of life) may be subdivided into early neonatal deaths, occurring during the first seven days of life, and late neonatal deaths, occurring after the seventh day but before 28 completed days of life [11].
2. Materials and Methods
Several studies which have been conducted on infant and child mortality in Kenya use census or survey data. All of these studies, including the four Demographic and Health Surveys [11, 15] conducted so far, use indirect methods like the Trussell’s technique to estimate the child mortality. Some of these studies have also employed multivariate linear and logistic regression to identify the determinants of infant child mortality. However, Ordinary Least Squares (OLS) or binary dependent variable regression models cannot handle very well the aspect of child mortality because of the occurrence of the transition event being the dependent variable. OLS, for example is inadequate for survival data because of censoring (and truncation), time varying covariates and structural modeling [11]. Binary dependent regression models can to some extend take care of both the censoring and structural modeling problem (not the time varying covariates) but there is a risk of losing a lot of information in particular about when someone faced the hazard since the dependent variable is mis-measured.This study focused on confirming whether there is significant difference in infant mortality rate/the survival rate of newborn babies and babies up to the age of one year, that can be associated with various government interventions [6, 8, 13]. This was done among pregnant women who had HIV, Malaria and anaemia during the pregnancy period.This study contributes to methodological innovation in infant and child mortality studies in Kenya by introducing survival analysis into child mortality modeling. Duration models are the most suited for such analysis because they account for problems like right-censoring, and structural modeling which traditional econometric techniques cannot handle adequately.Study Site and Screening ProceduresData on births and survival of infants from pregnant mothers attending antenatal care (ANC) in 3 hospitals in Kisumu County (Jaramogi Oginga Odinga Teaching and Referral Hospital (JOOTRH), Port Florence Community Hospital and Chulaimbo Hospital) was studied and extracted with the aid of research assistants. Data was sectioned in two blocks. Recorded cases between year 2001 to 2008 and cases within 2009 and 2015. Records of pregnant HIV-seropositive mothers who consistently attended antenatal care (ANC) clinic throughout the pregnancy period and a year after birth, or death of infant, where included in the study.Post-neonatal infant mortality PNIM was a death occurring between 29 and 364 days of delivery. HIV-positive infants were infants of HIV seropositive mothers who had _2 consecutive positive PCR tests with the first positive PCR test at age _4 months; HIV-negative infants were infants of HIV-seropositive mothers who had 2 consecutive negative PCR tests and for whom the PCR test at the last visit was negative. Infants for whom we had insufficient PCR data to determine their status were excluded. Malaria in infants was defined as the presence of asexual stage parasites in thick smears, independent of the presence or absence of clinical signs and symptoms and independent of species. Moderate to severe anemia among infants was defined as a hemoglobin level <8 g/dL. Only cases with complete data where considered for the study. This therefore was not 100% sample from the hospitals. Analysis was carried by use of Cox proportional hazard model and computations done by a computer program called R.The variables were selected on the basis of dependent and independent cases. The dependent variable was the number of infant deaths while the independent variables are the prevalence of HIV, Malaria and anemia among pregnant mothers.Figure 1 below shows the country statistics as plotted using the World Bank data.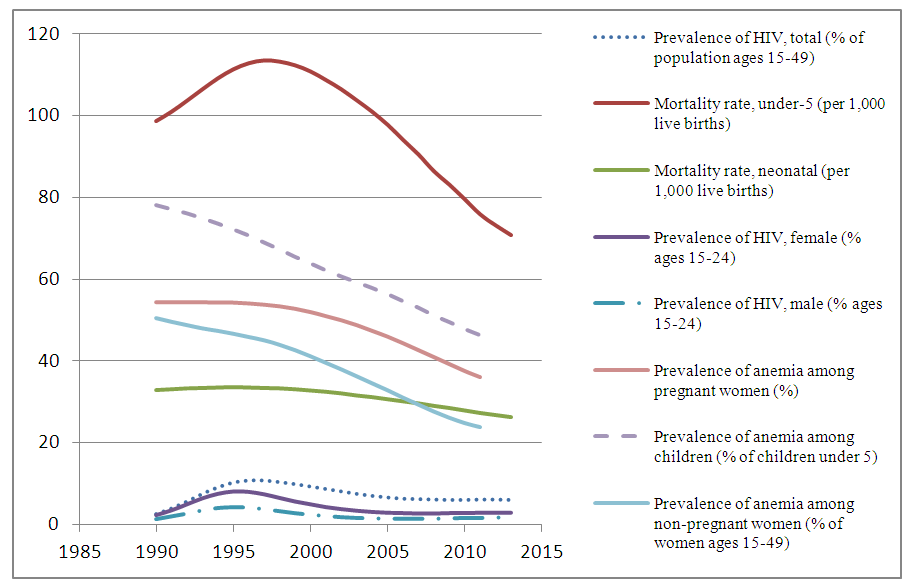 | Figure 1. Chart on Prevalence of HIV in male and female in comparison with mortality rates. (Source: World Bank) |
Theoretical ModelThe study employs survival analysis, whose main concepts are the hazard function and the survivor function. The aim is to estimate the probability of a child dying within the next day after surviving for t months as a result of environmental factors, among others. We focus on children that were born alive and model their mortality probabilities until reaching age 1. Duration models were used to specify this mortality.The survivor function denotes the probability that a child survives up to a particular age. The function is obtained from what is known in the survival analysis literature as the failure function F(t). | (2.1) |
Where T is the length of a completed spell which implies that the Survivor function is given as:- | (2.2) |
There is a one-to-one relationship between specifications for the hazard rate and the survivor function.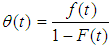 | (2.3) |
This, after mathematical manipulation gives,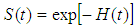 | (2.4) |
Where, | (2.5) |
Is the integrated hazard function.The important result is that, whatever functional form is chosen for (t), one can derive S(t) and F(t) from it (and also f(t) and H(t)), and vice versa. In the context of child mortality, the hazard rate is often referred to as mortality rate [10]. The mortality rate at age t can be interpreted as the intensity at which a child dies at this age, given that the child survived until age t.To check robustness, two models were implemented for the length of time t that a child survives. These were a parametric (Weibull model) and a semi- parametric model (the Cox proportional hazard model).Weibull Model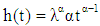 | (2.6) |
Introducing the covariates, the model becomes: | (2.7) |
Cox Model | (2.8) |
Where (h(t,…)) denotes the resultant hazard, given the values of the m covariates for the respective case (X1, X2,…,Xm) and the respective survival time. The term (h0 (t)) is called the baseline hazard and it is the hazard for the respective individual when all independent variable values were equal to zero. The model can be linearized by dividing by both sides of the equation 2.8 by (h0 (t)) and then taking the natural logarithm of both sides.The hazard function h(t/X) was estimated using Maximum Likelihood estimation. The likelihood function is given as: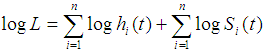 | (2.9) |
The log of h(t)S(t) is the individual contribution of the likelihood function intended to be maximized.Differences in proportions were analysed using the Chi-square test. This is the sum of (0-E)² /E = χ² . According to the R output, χ² = 2.1347+3.8733=6.0069, d.f= 4 and P<0.50.Differences in means were compared using Student’s t test;This was run in the R programme and the results obtained as follows: (t-test for equality of means)t-value= 1.73, d.f= 8, 2-tail sig= 0.125 and SE of d.f= 6.017.Since the table value > Calculated value, we accept that there is significance decrease in infant mortality as a result of interventions by the government.Differences in medians were compared using nonparametric tests. Crude mortality rates were calculated using survival tables that were computed in the R programme using survival analysis commands.Cox regression analysis was used to calculate hazard ratios (HRs) to assess the rate of PNIM among infants with placental malaria and infant HIV infection. This was done using a model that allowed both time-fixed and time-varying variables. Variables examined were those associated with infant mortality and included low birth weight (LBW) [6]. Placental malaria was retained in all models because it was one of the main exposures of interest. Time varying variables included infant malaria parasitemia and hemoglobin levels <8 g/dL.Models were stratified by infant HIV status because of significant interactions between infant HIV status and other variables of interest; variables that were significant in the main model were maintained in the stratified models to allow comparison.All tests were 2-sided, and P < .05 was considered to be statistically significant. Analysis was done using R for Windows.
3. Results and Discussions
Since the primary interest of this study was to investigate the extent of success of the government interventions over recent years by use of analysis of probability of child mortality, a multivariate model of the child life duration was specified. Table 2 shows a summary of findings from the study.Table 2. Summary of Study Findings 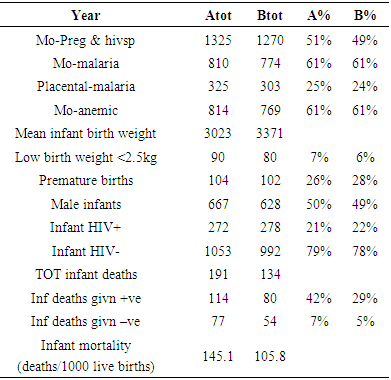 |
| |
|
Two popular methods of analysing the effect of explanatory variables on the hazard rate are the proportional hazard model and the accelerated failure time. The former is based on a semi-parametric model. This is a flexible method of estimation since the baseline hazard is estimated non-parametrically and eliminates the risk of corrupting the estimated hazard parameters while the effect of the covariates takes a particular functional form.Secondary data for the period A(2001 – 2008) and B(2009 – 2015) on infants born to 1325 and 1270 HIV seropositive mothers respectively in Kisumu County - Kenya were extracted and analysed. HIV status, Malaria (parasitemia or clinical malaria) and anaemia (haemoglobin level <8 g/dL) are the key independent regressors to the response ‘infant mortality’.On free insecticide treated mosquito nets to curb MalariaMothers who presented cases of malaria infection did not change significantly between the two time periods. Incidentally, approximately the same percentages (61%) of women who had malaria also had anaemia. Placental malaria insignificantly (t-test: p>0.05) decreased by 1% between period A and B. Birth weight increased slightly from 3kg to 3.3kg on average.According to the analysis, it is evident that the issuance of free insecticide treated mosquito nets to curb Malaria in Pregnant women and infants as a measure of curbing PNIM, the intervention has very little positive influence so far to the overall rate of PNIM.On Prevention of Mother to Child Transmission of HIVAmong the 272 HIV positive infants in group A, 42% died while 29% of 278 in group B did not survive. Among the 1053 HIV negative infants in group A, 7% died while 5% of 992 in group B passed on. There was significant decrement in deaths between the periods. This can be attributed to above mentioned government intervention. This means that the program of PMTCT is a success as seen from the results. A chi-square test confirmed the hypothesis with a p-value (P < .001).In this study population, placental malaria and infant parasitemia were not risk factors for PNIM among infants of HIV-seropositive mothers. On Distribution of Iron Folic Acid Supplements (IFAS) to Curb Anaemia during PregnancyInfants born to mothers with anaemia have been reported to have a higher risk of infant anaemia. It was therefore determined that the observed association between infant anaemia and PNIM could partially be explained by the effect of being born to mothers who had anaemia during pregnancy, which, was also associated with PNIM, although the direction of the association differed between HIV-negative and HIV-infected infants. Removal of placental malaria from the models did not substantially change the HR between PNIM and infant parasitemia or anaemia (AHR, 0.36 [95% CI, 0.10–1.23] and AHR, 5.06 [95% CI, 1.99–12.90] for parasitemia and anemia, respectively, in HIV-negative infants; AHR, 0.34 [95% CI, 0.08–1.44] and AHR, 1.02 [95% CI, 0.30–3.42] for parasitemia and anaemia, respectively, in HIV-positive infants). However, the prevention of infant anaemia may decrease PNIM among HIV negative infants of HIV-seropositive mothers.Table 3. Infant mortality by malaria and timing to death for the two periods A and B 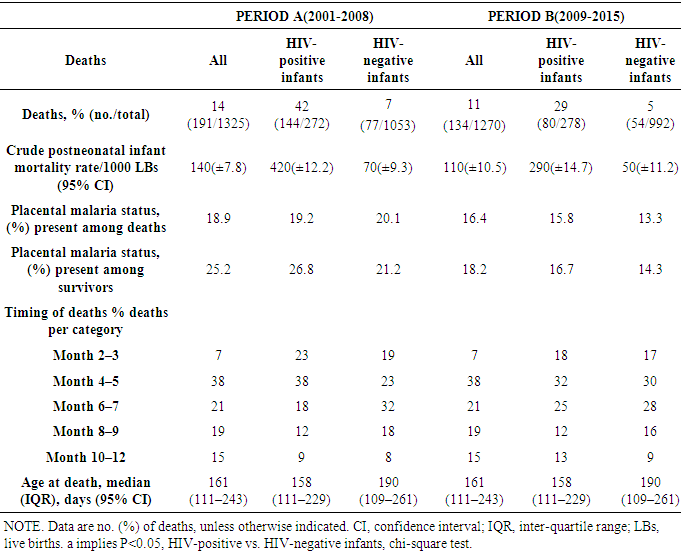 |
| |
|
Table 4. Risk factors for post neonatal infant mortality by Infant HIV-status among infants of HIV-seropositive women, Kisumu, Kenya, 2001-2015 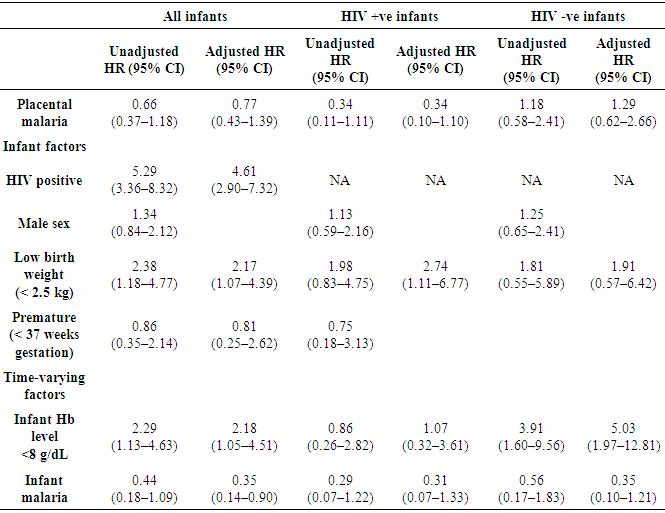 |
| |
|
From the table above, multivariate Cox regression analysis among HIV-negative infants, PNIM was associated with infant anaemia (adjusted hazard ratio [AHR], 4.73 [95% CI, 1.23–11.65]) but not with placental malaria (AHR, 1.12 [95% CI, 0.59–2.75]) or infant malaria (AHR, 0.38 [95% CI, 0.15–1.18]). Among HIV-positive infants, neither placental malaria (AHR, 0.37 [95% CI, 0.13–1.08]) nor infant malaria (AHR, 0.43 [95% CI, 0.02–1.43]) or anaemia (AHR, 1.11 [95% CI, 0.37–3.71]) was significantly associated with PNIM.With the high rate of PNIM due to mothers having anaemia, it was however noted that the rate of anaemic pregnancy recorded over the years under study had reduced as a result of the government intervention of distribution of IFAS to curb anaemia in pregnant women.
4. Conclusions
The main objective of the study was successfully met since we determined that there was some significant improvement in infant mortality between the two time brackets 2001-2008 and 2009-2015.However, Malaria’s effect had not diminished. It is concluded that the government effort to provide insect treated mosquito nets has not significantly reduced malaria infection among HIV – seropositive pregnant women.There was significant decrease however in the rate of HIV Transmission of Mother to Child across the years thus reducing the rates of PNIM as a result of HIV transmission.The rate of PNIM as a result of mothers suffering from anaemia during pregnancy also reduced significantly during the time of study.
ACKNOWLEDGEMENTS
I acknowledge the County Government of Kisumu for approving the study protocol by way of research permit. I also acknowledge JOOTR Hospital, Port Florence Community Hospital and Chulaimbo Sub – County Hospital for allowing us to collect and use their data.
References
[1] | Abdul H, Mohammad H, & Md-Musa K (2013) Cox’s Proportional Hazard Model Analysis of Child Survival in Bangladesh, Asian Journal of Social Sciences & Humanities, vol.2 pp 608-618. |
[2] | CDC, (2012) HIV among Pregnant Mothers, Infants, and Children in the United States. Webpage http://www.cdc.gov/hiv/pdf/risk_WIC.pdf [accessed 1/03/2015]. |
[3] | Baker, R. (1999) Differential in Child Mortality in Malawi, Social Networks Project Working Papers, No. 3, University of Pennsylvania, USA. |
[4] | Central Bureau of Statistics (CBS) [Kenya], Ministry of Health (MOH) [Kenya], and ORC Macro (2004) Kenya Demographic and Health Survey. |
[5] | Corse, D. (1972) “Regression Models and Life-tables”, Journal of the Royal Statistical Society, Series B, 74: 187-220. |
[6] | De-Gita, S. (1996) The Socio-economic Differentials of Infant and Child Mortality in Kenya: Evidence from Kenya Demographic and Health Survey (KDHS) 1993 data. Journal of Population Studies and Research. Vol.7 pp (18-64). |
[7] | Esponazilo, M. (2002) Infant Mortality and its Underlying Determinants in Rural Malawi, Journal of medical Sciences. Vol 345. |
[8] | Geda, A., Jong, N, & Kimenyi, M. (2001) Determinants of Poverty in Kenya: Household-Level Analysis Nairobi. KIPPRA Working Paper No. 6. |
[9] | Hill, K., Bicego G., & Mahy M. (2000) Childhood Mortality in Kenya: An Examination of Trends and Determinants in the Late 1980s to Mid-1990s, Macro International Report, Maryland, USA. |
[10] | Jacoby, H., & Wang, L. (2003) Environmental Determinants of Child Mortality in Rural China: A Competing Risks Approach, Journal of Health Science, vol 9. |
[11] | Jada, L. (1992) Determinants of Levels and Differentials of Early Childhood Mortality in Kenya Based on Kenya Demographic and Health Survey (KDHS) 1989, Journal of Scientific Publishers, vol 6, pp (23-49). |
[12] | Kamau, N. (1998) Child survival determinants in the arid and semi-arid lands: A study of Machakos, Kilifi and Taita-Taveta districts, Journal of demography and related studies, vol 13. |
[13] | Kimalu, P., Nafula, N., Manda, D., Mwabu, G., & Kimenyi, S. (2012) A Situational Analysis of Poverty in Kenya. KIPPRA Working Paper No. 6, Nairobi: KIPPRA. |
[14] | Lindskog, U., Carstensen, N., Larsson, Y., & Gebre-Medhin. (1988) Childhood Mortality in Relation to Nutritional Status and Water Supply-a Prospective Study from Rural Malawi, Journal of Action Pediatrics Stand 77: 260-268. |
[15] | Mosley, W., & Chen, L. (1984) An Analytical Framework for the Study of Child Survival in Developing Countries, Journal of Population and Development Review 10: 25-45. |
[16] | Murray, C., & Lopez, A (1996) The Global Burden of Disease: A Comprehensive Assessment of Mortality from Diseases, Injuries and Risk Factors in 1990 and projected to 2020. Journal of International Diseases and Cure, vol 17, pp (44-78). |