Aladeniyi O. B., Bodunwa O. K., Sonde M.
Department of Statistics, Federal University of Technology, Akure, Nigeria
Correspondence to: Bodunwa O. K., Department of Statistics, Federal University of Technology, Akure, Nigeria.
Email: |  |
Copyright © 2017 Scientific & Academic Publishing. All Rights Reserved.
This work is licensed under the Creative Commons Attribution International License (CC BY).
http://creativecommons.org/licenses/by/4.0/

Abstract
This study involves statistical analysis of reported cases of sexually transmitted diseases, in the State Specialist Hospital, Akure, Ondo State, Nigeria. The data were collected from January 2013 – December, 2014. The research studied incidence of sexually transmitted diseases and survival among different age groups and gender and factors which influence the incidence in the target population. Descriptive statistics were used in the presentation of the data; logistic regression was also used to establish a model that can predict the survival of patients with sexually transmitted diseases based on their age and gender, the duration of hospital stay and type of sexually transmitted disease. The type of sexually transmitted disease and age were found to be significant in predicting survival. Other models were also fitted with logistic regression to determine the individual contribution of the independent variables to survival. Chi-square test was used to determine the association between age, gender, disease type and survival outcome of sexually transmitted diseases (STDs). The result showed that there is no relationship between the age and survival, while there is a significant relationship between sex and survival. Also there is a significant relationship between the type of sexually transmitted disease and the survival. It was discovered that the male gender has a greater chance of surviving any one of the diseases regardless of age.
Keywords:
Logistic regression, Survival, Sexually transmitted disease
Cite this paper: Aladeniyi O. B., Bodunwa O. K., Sonde M., Statistical Analysis of Reported Cases of Sexually Transmitted Diseases, International Journal of Statistics and Applications, Vol. 7 No. 3, 2017, pp. 186-191. doi: 10.5923/j.statistics.20170703.04.
1. Introduction
Sexually Transmitted Diseases (STDs) refers to a set of clinical infections in which a mode of transmission is through sexual contact, and in which at least, one partner is infected. Many of these infections spread predominantly through sexual intercourse, but in some others, sexual contact may play a less predominant or uncertain role. Most STDs are not, however spread through casual contact, vectors or formites.Sexually transmitted diseases (STDs) are recognized as a major public health problem in most of the industrialized world. The World Health Organization (WHO) estimates that, in the mid-1990s, 30 million curable sexually transmitted infections (syphilis, gonorrhea, Chlamydia and trichomoniasis) occurred every year in North America and Western Europe with an additional 18 million cases in Eastern Europe and Central Asia. These counts do not include incurable Sexually transmitted diseases (STDs) such as genital herpes and Human Papilloma Virus (HPV) infections, for which no up-to-date estimates have been derived by the WHO. Approximately 74, 000 new HIV infections are estimated to have occurred in 1997 in North America and Western Europe. Centre for disease Control and prevention (Sexually transmitted diseases Surveillance – 2011).Although some increases in incidence are documented, it is unclear, how much of this upward trend is due to improvements in case ascertainment and surveillance or to actual increase in STD incidence. Most developed countries have seen dramatic declines in the incidence of Syphilis and gonorrhea since World War II. Some eastern European countries nevertheless have recently experienced increase in these two STDs.STDs deserves attention, not only because of the high prevalence, but also because they frequently go undetected and untreated and can result in serious reproductive morbidity and mortality. Compared with the extensive efforts devoted to research and intervention on HIV and AIDS, very little attention has been paid to other STDs. Hence there is a need to increase awareness of, at least, one central aspect of most common curable STDs – their incidence. Recent findings shows that some STDs acts as a cofactor or facilitator for HIV transmission, arguing that research on STDs other than HIV and AIDS can also contribute to better insights into HIV infections. Sexually transmitted diseases are responsible for a variety of health problems and can have especially serious consequences for adolescents and young adults. CDC-STD Surveillance (2011).TransmissionThe mode of transmission varies among the different sexually transmitted diseases. Some bacteria or virus are found in vaginal secretions or semen (e.g. gonorrhea), while others are shed from the skin of and around the genitals (e.g. HSV and HPV). Infections typically occur during sexual intercourse or when the genitals come into close contact. Infections may also occur during oral sex. It may also be transmitted during non-censenting sex acts such as rape or molestation.The transmission of STDs is more efficient from men to women than from women to men. For example, with just one unprotected sexual encounter with an infected partner, a woman is twice as likely to acquire gonorrhea or Chlamydia. In addition, different STDs have different rates of transmission. For example, with one unprotected sexual intercourse, a woman has 1 percent chance of acquiring HIV, 30 percent chance of acquiring herpes and 50 percent chance of contracting gonorrhea if her partner is infected. Macdonald and David (2003).Several studies on STDs have been evaluating sexual behaviors for quite a while. Following HIV pandemic from 1980-1990s, the focus on sexual behaviors evaluation intensified. The researchers have been investigating sexual behaviors in a variety of context often asking the same questions for various purposes. Some authors evaluated sexual behaviors in relation with STDs in order to assess individuals’ risk of acquiring STDs. Others concentrated on specific groups to describe and identify high and low risk population. Kennedy and ZephantaMtaturu (2006). However, statistics on STDs show no sign of abating the ever increasing number of STDs and deaths due to the fact that HIV/AIDS are common now, particularly in developing countries. As the time goes on, the trend of sexually transmitted diseases is becoming a big problem among the youth. Kennedy and ZephantaMtaturu (2006).Prevalence estimates suggests that young people aged 15-24 years acquire half of all new STDs and that 1 in 4 sexually active adolescent females have an STD, such as Chlamydia or HPV. Compared with other adults, sexually active adolescent aged 15-19 years and young adults aged 20-24 are at higher risk of acquiring STDs for a combination of behavioral, biological and cultural reasons. For some STDs, such as Chlamydia, adolescent females may have increased susceptibility to infection because of increased cervical ectopy. The higher prevalence of STDs among adolescents also may reflect multiple barriers to accessing quality STD prevention services, including lack of health insurance or ability to pay, lack of transportation, discomfort with facilities and services designed for adults and concerns about confidentiality. CDC (2011).According to Ayo et.al. (2013), Nigeria has a fast growing population and is confronted with numerous health challenges. With a population of more than 150 million, the country’s population is young; therefore, the future of the country rests to a greater extent, on how successful, its youth have a transition to a healthy and productive adulthood. Adebowale (2013) et al in a research work on statistical modeling of social risk factors for sexually transmitted diseases among female youths in Nigeria argues that, STDs remain a major public health challenge because of their health consequences, several complications especially among women who excessively bear long term consequences. It was also stated that the prevalence of STDs among Nigerian female youths is 17 percent, arguing that STDs causes infertility in women and increases the risk of transmission of HIV/AIDS. Adopting a multi-staged probability sampling to select respondents among women of child bearing age (15-49 years), the study used data from records of ICF Macro Calverton, in conjunction with National Population Commission, Nigeria in 2008.In light of this, this paper investigated the effect of the incidence of sexually transmitted diseases and survival among different age groups and gender in the target population using the age and gender of the patient, duration of hospital stay and type of sexually transmitted disease as independent variable. Three diseases were considered namely: Staphylococcus, Urinary Tract Infection (UTI) and Retroviral disease (RVD).
2. Methodology
Descriptive Statistics was used to show the frequency distribution in percent of demographic variables of people infected with Sexually Transmitted Diseases and also to show the distribution of the diseases across the population of study. The descriptive statistics was carried out with the aid of Microsoft Excel Package.The Logistic regression model was to determine the factors contributing to the survival of a patient having any of the sexually transmitted diseases, and the Chi-square test was used to determine the association existing between age, gender and disease type and the survival outcome of STDs. The Alkaike Information Criterion (AIC) was also used to determine which of the Logistic regression models has the best fit and to assess how the model behaves as the number independent variables increases.The Logit and Odd ratio model is given as | (1) |
Where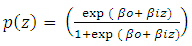 | (2) |
And the Chi-square model is given as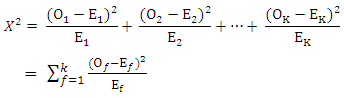 | (3) |
Where Of is the observed frequencyEf is the expected frequency
3. Data Collection and Presentation
In order to gather relevant data and information for this research work, the secondary source of data collection was adopted. This data was obtained from case notes and hospital health record documents of 200 reported cases of the specified diseases for a period of 24 months starting from January 2013 – December, 2014 of the Health information department, State Specialist Hospital, Akure, Ondo State, Nigeria. The data used for this analysis shows the survival outcome of three (3) sexually transmitted diseases namely: Staphylococcus, Urinary Tract Infection and Retroviral Disease. Data was analyzed using the R Programming Language. Logistic Regression and Chi-square tests were utilized. A p-value of 0.05 was considered statistically significant. A description of the variables is presented in Tables 1 to 3 and fig 1.Table 1. Distribution of age among people infected persons 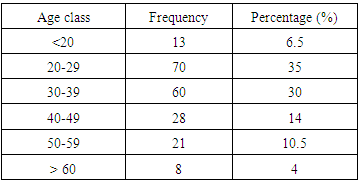 |
| |
|
Table 2. Gender Distributions 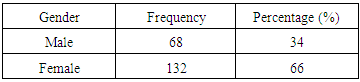 |
| |
|
Table 3. Distribution of the three diseases across gender among infected persons 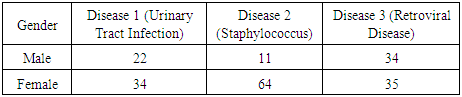 |
| |
|
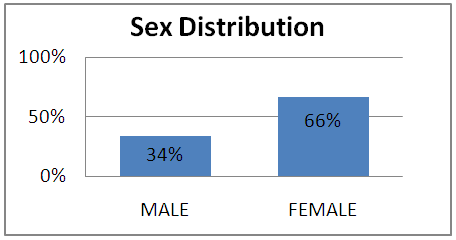 | Figure 1. Graphical Representation of Gender Distribution |
LOGISTIC REGRESSION ANALYSISThe Logistic Regression analysis was performed with the aid of R Programming Language version 3.1.2.
A. The General (multivariate) ModelThis hypothesis sought to determine the extent to which the likelihood of reporting survival is different across the independent variables. The table below gives the outcome of the logistic regression model fitted using sex, age, diseases, duration of hospital stay as independent variables while the dependent variable is the outcome survival (using 0 for death and 1 for alive) after admission.Note: Disease 1 represents Urinary Tract Infection, disease 2 represents Staphylococcus and disease 3 represents retroviral disease (RVD) Sex 1 represents the male gender while sex 2 represents the female genderH01: probability of being alive is equal to probability of dyingH11: there is difference in their probabilitiesESTIMATION
(Dispersion parameter for binomial family taken to be 1)Null deviance: 258.98 on 199 degrees of freedomResidual deviance: 201.79 on 194 degrees of freedomAIC: 213.79Number of Fisher Scoring iterations: 4The exponential gives the odd ratio (using odd ratio 1 to indicate that the event is equally likely in both groups or for all values of an independent variable).a1. Odds Ratios
a2. The 95% Confidence interval for the general model
b. This is to test the assumption that the survival outcome of persons of average age is equally likely in all cases. Simple Logistic Regression output for survival outcome of persons as a function of age.
(Dispersion parameter for binomial family taken to be 1)Null deviance: 258.98 on 199 degrees of freedomResidual deviance: 247.60 on 198 degrees of freedomAIC: 251.6Number of Fisher Scoring iterations: 4b1.
C. This is to test the assumption that males are at a greater risk of not surviving regardless of the effects of age and duration of stay at the hospitalc1. Model output for survival of males regardless of effect of age
c2.
c3. Model output for survival of males regardless of duration
(Dispersion parameter for binomial family taken to be 1)Null deviance: 258.98 on 199 degrees of freedomResidual deviance: 254.56 on 197 degrees of freedomAIC: 260.56Number of Fisher Scoring iterations: 4c4.
D. This is to test the assumption that regardless of the disease type, there is equal chance of survival for infected persons.d1. Model output for survival of infected persons regardless of disease type
(Dispersion parameter for binomial family taken to be 1)Null deviance: 258.98 on 199 degrees of freedomResidual deviance: 210.18 on 197 degrees of freedomAIC: 216.18Number of Fisher Scoring iterations: 4d2.
CHI-SQUARE ANALYSIS: The Chi - Square analysis was performed with the aid of R Programming Language version 3.1.2.E. Relationship between sex and outcome of survival: Output on relationship between sex and outcome of survival
Pearson's Chi-squared test with Yates' continuity correctionX-squared = 2.1635, df = 1, p-value = 0.1413F. Relationship between age and outcome of survival Chi-square output on relationship between age and outcome of survivalPearson's Chi-squared test X-squared = 54.2463, df = 49, p-value = 0.2813G. Relationship between duration and outcome of survivalOutput on relationship between duration and outcome of survival
Pearson's Chi-squared testX-squared = 14.3923, df = 17, p-value = 0.6392H. Relationship between disease and outcome of survival Output on relationship between disease and outcome of survival
Pearson's Chi-squared testX-squared = 47.2756, df = 2, p-value = 5.423e-11I. Sex-disease Interaction Output on Sex-disease Interaction
Pearson's Chi-squared testX-squared = 49.1989, df = 5, p-value = 2.021e-09
4. Results and Discussion
The table above shows the distribution of age among infected individuals. The age group 20–29 has the largest percentage (35%) and the age group 60 and above has the least percentage of (4%). It is also shows that the females gender have frequency and percentage of infected persons of 66% than the male gender which have a percentage of 34%. The table also shows that in the distribution of the three diseases across gender among infected persons, staphylococcus has a higher incidence with the female gender having the highest occurrence.From the logistic regression model, the general model Model: Log (odds that survival = 1) = 0.72061 - 0.01740(Sex2) -0.03426(Age) - 0.05584(duration) + 2.71739(disease 2) + 1.79718(disease 3) + єShows that when all independent variables are equal to zero, the log odds of survival = 0.72061, at 0.05 level of significance, age, disease 2 and disease 3 are significant in the model.Since the intercept βo is greater than zero (0), we reject the null hypothesis and we conclude that the probability of being alive is not equal to the probability of dying.Also, for every one unit change in the independent variables, the log odds of survival changes by the coefficient of the independent variables.The Logistic regression output for survival of persons of average age shows that the intercept βo is significantly greater than zero (0). This implies that the odds of survival outcome for persons of average age are not equally likely in all cases.Considering the survival of the male gender regardless of age, the p-value for sex is 0.30784, which is not significant at 0.05. This shows that the male gender has a greater chance of survival regardless of age. Also, considering the survival of the male gender regardless of the duration spent on admission at the state hospital, the p-value is 0.1704, which is also not significant at 0.05. Hence, the male gender has a greater chance of survival regardless of the duration spent at the hospital. Considering the survival outcome based on the type of disease, the p-value for the diseases are 7.79e-10 and 4.36e-05. This shows that irrespective of the disease type, there is equal chance of survival of the diseases.Looking at the chi-square relationship test, for relationship between sex and outcome of survival, the p-value is 0.1413, which shows that there is no significant relationship between gender and outcome of survival. Also, looking at the relationship between age and outcome of survival, the p-value is 0.2813, showing that there is no significant relationship between the 2. Relationship between duration of stay in the hospital and outcome of survival gives a p-value of 0.6392 which also implies no significant relationship. Considering disease and outcome of survival, the p – value of 5.423e-11 shows a significant relationship between the two variables. Also, the analysis has shown a sex-disease interaction to be significant in the outcome of survival of the diseases.Several findings were deduced from this study. Based on the data analysed, it could be seen that the male gender have a greater chance of surviving any sexually transmitted disease. The male gender also has a lower chance of getting infected with any sexually transmitted disease. The various sexually transmitted diseases examined have equal effect on the survival outcome, that is, each of them can lead to death of an infected person and can also be survived. Age and duration of stay at the hospital is not significant in determining whether the patient will survive the disease or not.
5. Recommendations
The result of this study shows that female is at greater risk of contracting and dying of sexually transmitted disease because their anatomy is more prone to infection. Therefore, constant examination of the females is recommended, so as to detect any infection early enough before it becomes a threat. Women should be encouraged to avoid unprotected sex to avoid the risk of contracting sexually transmitted disease.Furthermore, since the diseases examined have equal chance of being survived, it is recommended that they should all be treated with equal priority as soon they are detected in a person. It is also recommended that people infected with sexually transmitted diseases should seek early treatment in order to increase their chance of survival.
References
[1] | Ayo Adebowale Stephen, Musibau T. Adeniyi F.F. and OdunayoAkinyeni J. (2013). Adebowale et al. Statistical modeling of social risk factor for sexually transmitted diseases among female youths in Nigeria. Department of epidemiology, medical statistics and environmental health, college of medicine, University of Ibadan. |
[2] | Centre for disease Control and prevention (Sexually transmitted diseases Surveillance – 2011), U.S department of health and human services, Atlanta. |
[3] | Kennedy D, Mwanbete and ZephantaMtaturu (2006). Article on knowledge of sexually transmitted diseases among secondary school students in Dar es Salam, Tanzania:Afri. Health Sci. (2006) Sep: 6(3): PP 165-169. |
[4] | Dass, H K (1988). Advance Engineering Mathematics. 19th Edition, S.Chand& Co. Limited. |
[5] | Mac Donald, Noni E. and David M. Patrick (2003). Sexually transmitted diseases syndromes in Principles and practice of pediatric infectious diseases, second edition, New York: churchhill Livingstone. |
[6] | Rohan Palmer. An introduction to Logistic Regression in R. |