Rama Shanker
Department of Statistics, Eritrea Institute of Technology, Asmara, Eritrea
Correspondence to: Rama Shanker, Department of Statistics, Eritrea Institute of Technology, Asmara, Eritrea.
Email: |  |
Copyright © 2017 Scientific & Academic Publishing. All Rights Reserved.
This work is licensed under the Creative Commons Attribution International License (CC BY).
http://creativecommons.org/licenses/by/4.0/

Abstract
A one parameter lifetime distribution named “Rama distribution” for modeling lifetime data has been proposed and investigated. Its statistical and mathematical properties including its shape, moments, skewness, kurtosis, hazard rate function, mean residual life function, stochastic ordering, mean deviations, Bonferroni and Lorenz curves, stress-strength reliability have been discussed. The condition under which Rama distribution is over-dispersed, equi-dispersed, and under-dispersed have been studied and compared with other one parameter lifetime distributions. Maximum likelihood estimation and method of moments have been discussed for estimating its parameter. Finally the goodness of fit of the proposed distribution has been discussed with a real lifetime data and the fit has been compared with other one parameter lifetime distributions.
Keywords:
Lifetime distribution, Moments, Hazard rate function, Mean residual life function, Mean deviations, Bonferroni and Lorenz Curves, Stress-strength reliability, Estimation of parameter, Goodness of fit
Cite this paper: Rama Shanker, Rama Distribution and Its Application, International Journal of Statistics and Applications, Vol. 7 No. 1, 2017, pp. 26-35. doi: 10.5923/j.statistics.20170701.04.
1. Introduction
The modeling and analyzing lifetime data are essential in many applied sciences including biomedicine, engineering, insurance and finance, amongst others. The two one parameter lifetimes distribution used in statistics for modeling lifetime data are exponential and Lindley (1958) distributions. Recently, Shanker et al (2015) have comparative study on both exponential and Lindley distributions for modeling lifetime data and observed that there are many situations where these two distributions are not suitable to fit lifetime data due to their shape and other properties. In a search for continuous distribution which gives better fit than exponential and Lindley distributions, Shanker (2015 a, 2015 b, 2016 a, 2016 b, 2016 c) has introduced one parameter lifetime distributions namely Akash, Shanker, Aradhana, Amarendra and Sujatha distributions. Each of these lifetime distributions has advantages and disadvantages over one another due to its shape, hazard rate function and mean residual life function. Still there are many situations where these distributions are not suitable for modeling lifetime data from theoretical or applied point of view. Therefore, an attempt has been made in this paper to obtain a new distribution which is flexible than these lifetime distributions for modeling lifetime data in reliability and in terms of its hazard rate shapes. The new one parameter lifetime distribution is based on a two- component mixture of an exponential distribution having scale parameter
and a gamma distribution having shape parameter 4 and scale parameter
with their mixing proportion
. The probability density function (p.d.f.) of a new one parameter lifetime distribution can be introduced as | (1.1) |
We would call this distribution, “Rama distribution”. This distribution can be easily expressed as a mixture of exponential
and gamma
with mixing proportion
. We have
where
.The corresponding cumulative distribution function (c.d.f.) of (1.1) can be obtained as  | (1.2) |
The graph of the p.d.f. and the c.d.f. of Rama distribution for varying values of
are shown in figures 1 and 2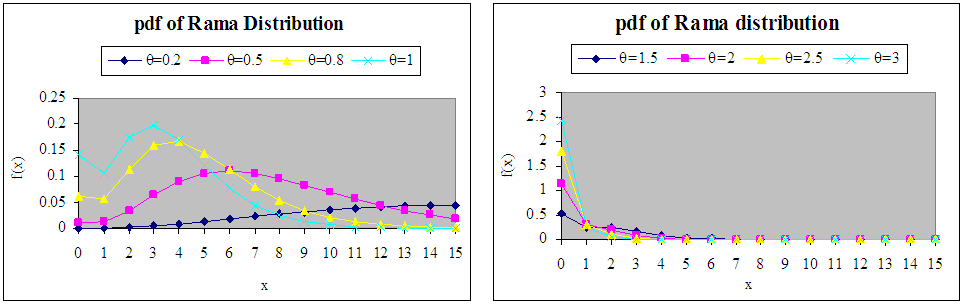 | Figure 1. Graph of the pdf of Rama distribution for varying values of the parameter θ |
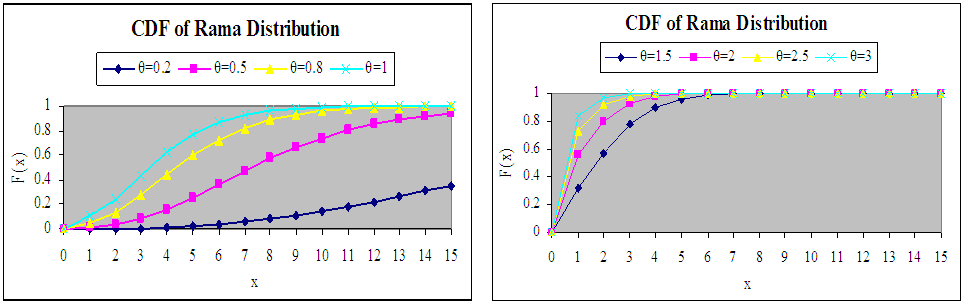 | Figure 2. Graph of the cdf of Rama distribution for varying values of the parameter θ |
2. Moments and Moments Based Measures
The moment generating function of Rama distribution (1.1) can be obtained as
Thus the
th moment about origin of Rama distribution (1.1) can be obtained as
The first four moments about origin of Rama distribution (1.1) are as follows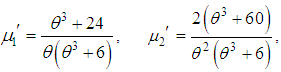
Using relationship between moments about mean and moments about origin, central moments of Rama distribution (1.1) are obtained as
The coefficient of variation
, coefficient of skewness
, coefficient of kurtosis
and index of dispersion
of Rama distribution (1.1) are thus obtained as
The condition under which Rama distribution is over-dispersed, equi-dispersed, and under-dispersed has been discussed along with condition under which Akash, Shanker, Aradhana, Amarendra Sujatha, Lindley and exponential distributions are over-dispersed, equi-dispersed, and under-dispersed and are presented in table 1.Table 1. Over-dispersion, equi-dispersion and under-dispersion of Rama, Akash, Shanker, Aradhana, Amarendra, Sujatha, Lindley and exponential distributions for varying values of their parameter 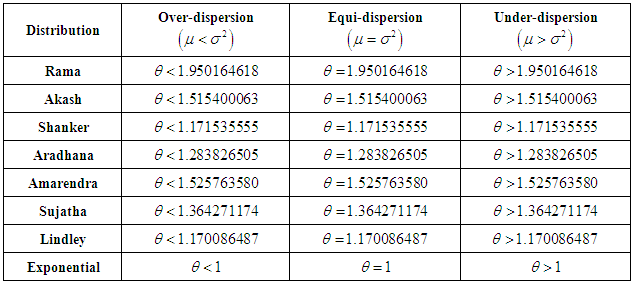 |
| |
|
3. Hazard Rate Function and Mean Residual Life Function
Let
and
be the p.d.f. and c.d.f of a continuous random variable. The hazard rate function
(also known as the failure rate function) and the mean residual life function
of
are respectively defined as  | (3.1) |
and | (3.2) |
The corresponding hazard rate function,
and the mean residual life function,
of the Rama distribution are thus obtained as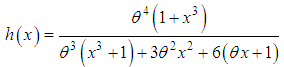 | (3.3) |
and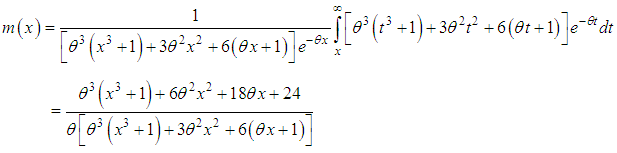 | (3.4) |
It can be easily verified that
and
. The graphs of the hazard rate function and mean residual life function of Rama distribution for varying values of the parameter are shown in figures 3 and 4.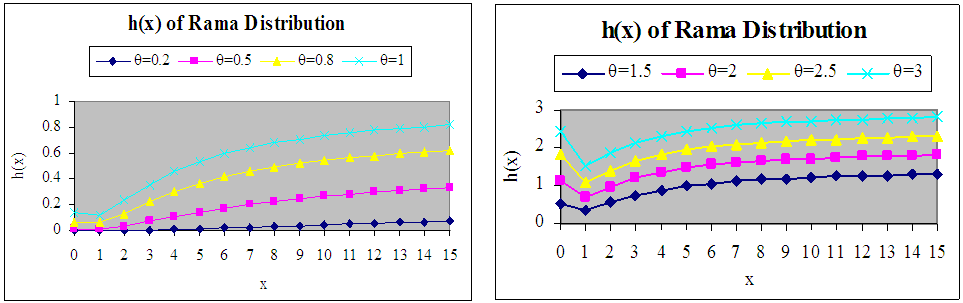 | Figure 3. Graphs of hazard rate function of Rama distribution for different values of parameter |
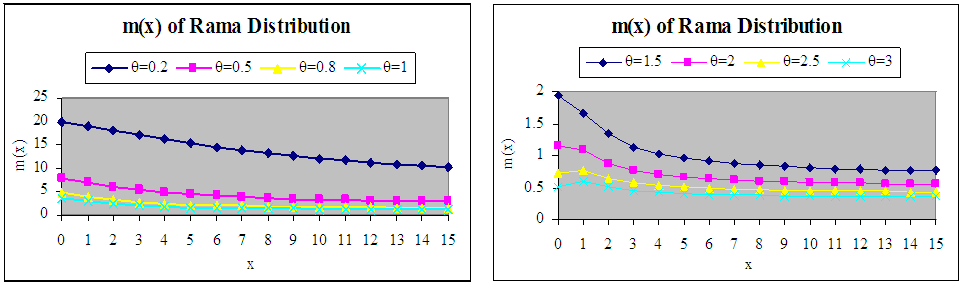 | Figure 4. Graphs of mean residual life function of Rama distribution for different values of parameter |
4. Stochastic Orderings
Stochastic ordering of positive continuous random variables is an important tool for judging their comparative behavior. A random variable
is said to be smaller than a random variable
in the (i) stochastic order
if
for all
(ii) hazard rate order
if
for all
(iii) mean residual life order
if
for all
(iv) likelihood ratio order
if
decreases in
.The following results due to Shaked and Shanthikumar (1994) are well known for establishing stochastic ordering of distributions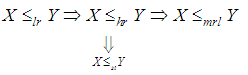 | (4.1) |
The Rama distribution is ordered with respect to the strongest ‘likelihood ratio’ ordering as shown in the following theorem:Theorem: Let
Rama distribution
and
Rama distribution
. If
, then
and hence
and
Proof: We have
Now
This gives
Thus for
,
. This means that
and hence
,
and
.
5. Mean Deviations
The amount of scatter in a population is measured to some extent by the totality of deviations usually from mean and median. These are known as the mean deviation about the mean and the mean deviation about the median and are defined as
and
, respectively, where
and
. The measures
and
can be calculated using the relationships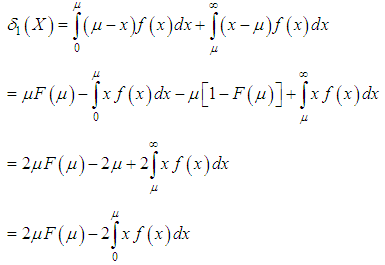 | (5.1) |
and 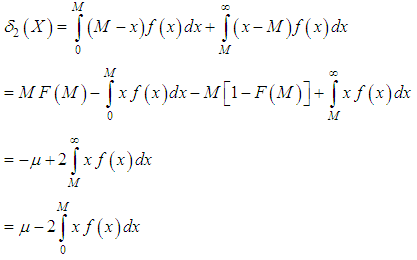 | (5.2) |
Using p.d.f. (1.1) and its mean, we get | (5.3) |
 | (5.4) |
Using expressions from (5.1), (5.2), (5.3), and (5.4), the mean deviation about mean,
and the mean deviation about median,
of Rama distribution are obtained as | (5.5) |
 | (5.6) |
6. Bonferroni and Lorenz Curves
The Bonferroni and Lorenz curves (Bonferroni, 1930) and Bonferroni and Gini indices have applications not only in economics to study income and poverty, but also in other fields like reliability, demography, insurance and medicine. The Bonferroni and Lorenz curves are defined as | (6.1) |
and  | (6.2) |
respectively or equivalently 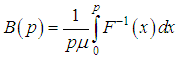 | (6.3) |
and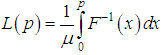 | (6.4) |
respectively, where
and
.The Bonferroni and Gini indices are thus defined as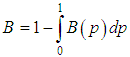 | (6.5) |
and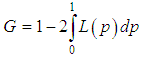 | (6.6) |
respectively.Using p.d.f. (1.1), we get  | (6.7) |
Now using equation (6.7) in (6.1) and (6.2), we get  | (6.8) |
and  | (6.9) |
Now using equations (6.8) and (6.9) in (6.5) and (6.6), the Bonferroni and Gini indices are obtained as | (6.10) |
 | (6.11) |
7. Stress-Strength Reliability
The stress- strength reliability describes the life of a component which has random strength
that is subjected to a random stress
. When the stress applied to it exceeds the strength, the component fails instantly and the component will function satisfactorily till
. Therefore,
is a measure of component reliability and in statistical literature it is known as stress-strength parameter. It has wide applications in almost all areas of knowledge especially in engineering such as structures, deterioration of rocket motors, static fatigue of ceramic components, aging of concrete pressure vessels etc.Let
and
are independent strength and stress random variables having Rama distribution (1.1) with parameter
and
, respectively. Then, the stress-strength reliability
of Rama distribution can be obtained as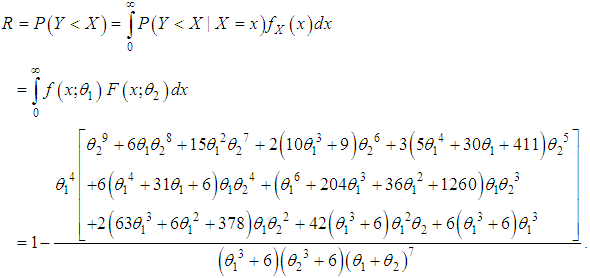
8. Estimation of Parameter
8.1. Maximum Likelihood Estimation (MLE)
Let
be a random sample from Rama distribution (1.1). The likelihood function,
of (1.1) is given by
The natural log likelihood function is thus obtained as
Now
where
is the sample mean.The MLE
of
is the solution of the equation
and so it can be obtained by solving the following fourth degree polynomial equation  | (8.1.1) |
8.2. Method of Moment Estimation (MOME)
Equating the population mean of the Rama distribution to the corresponding sample mean, MOME
of
is the same as given by equation (8.1.1).
9. Goodness of Fit
The goodness of fit of Rama distribution has been conducted with several lifetime data from biomedical science and engineering and in majority of data sets the goodness of fit of Rama distribution has been found to be quite satisfactory. In this section, the goodness of fit of one parameter Rama distribution has been discussed with a real lifetime data set and fit has been compared with one parameter Akash, Shanker, Aradhana, Amarendra, Sujatha, Lindley and exponential distributions. The following data set has been considered for the goodness of fit of Rama distribution. The data set is the strength data of glass of the aircraft window reported by Fuller et al (1994)
In order to compare lifetime distributions,
, AIC (Akaike Information Criterion) and K-S Statistics ( Kolmogorov-Smirnov Statistics) for the above data set have been computed. The formulae for computing AIC and K-S Statistics are as follows:
, where
= the number of parameters,
= the sample size and
is the empirical distribution function. The best distribution is the distribution which corresponds to lower values of
, AIC, and K-S statistics. The MLE
of
, S.E
, standard error of
,
, AIC and K-S Statistic of the fitted distributions are presented in the following table 2.Table 2. MLE’s, - 2ln L, AIC and K-S Statistics of the fitted distributions of data set 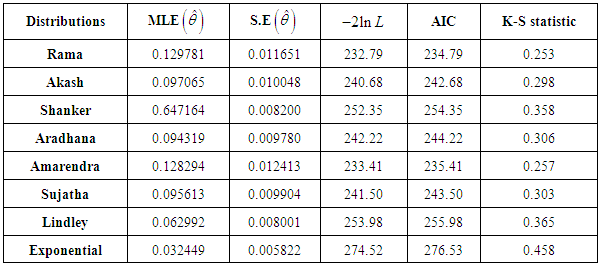 |
| |
|
It can be easily seen from above table that the Rama distribution gives better fit than Akash, Shanker, Aradhana, Amarendra, Sujatha, Lindley and exponential distributions and hence can be considered an important lifetime distribution for modeling lifetime data.
10. Concluding Remarks
A one parameter lifetime distribution named, “Rama distribution” has been proposed. Its statistical properties including shape, moments, skewness, kurtosis, hazard rate function, mean residual life function, stochastic ordering, mean deviations, Bonferroni and Lorenz curves, stress-strength reliability have been discussed. The condition under which Rama distribution is over-dispersed, equi-dispersed, and under-dispersed are presented along other one parameter lifetime distributions. Maximum likelihood estimation and method of moments have been discussed for estimating its parameter. Finally, the goodness of fit test using K-S Statistics (Kolmogorov-Smirnov Statistics) for a real lifetime data set has been presented and the fit has been compared with other one parameter lifetime distributions.
ACKNOWLEDGEMENTS
The author is grateful to the editor- in - chief and the anonymous reviewer for helpful comments which improved the quality of the paper.
References
[1] | Bonferroni, C.E. (1930): Elementi di Statistca generale, Seeber, Firenze. |
[2] | Fuller, E.J., Frieman, S., Quinn, J., Quinn, G., and Carter, W. (1994): Fracture mechanics approach to the design of glass aircraft windows: A case study, SPIE Proc 2286, 419-430. |
[3] | Lindley, D.V. (1958): Fiducial distributions and Bayes’ theorem, Journal of the Royal Statistical Society, Series B, 20, 102- 107. |
[4] | Shaked, M. and Shanthikumar, J.G. (1994): Stochastic Orders and Their Applications, Academic Press, New York. |
[5] | Shanker, R., Hagos, F, and Sujatha, S. (2015): On modeling of Lifetimes data using exponential and Lindley distributions, Biometrics & Biostatistics International Journal, 2 (5), 1-9. |
[6] | Shanker, R. (2015 a): Akash distribution and its Applications, International Journal of Probability and Statistics, 4(3), 65 - 75. |
[7] | Shanker, R. (2015 b): Shanker distribution and its Applications, International Journal of Statistics and Applications, 5(6), 338 – 348. |
[8] | Shanker, R. (2016 a): Amarendra distribution and its Applications, American Journal of Mathematics and Statistics, 6(1), 44 – 56. |
[9] | Shanker, R. (2016 b): Aradhana distribution and its Applications, International Journal of Statistics and Applications, 6(1), 23 – 34. |
[10] | Shanker, R. (2016 c): Sujatha distribution and its Applications, Statistics in Transition-new series, 17(3), 1 – 20. |