Ogoke U. P.1, Nduka E. C.1, Soyinka A. T.2
1Department of Mathematics and Statistics, University of Port Harcourt, Nigeria
2Department of Research and Training, Federal Neuro-Psychiatric Hospital Aro, Nigeria
Correspondence to: Ogoke U. P., Department of Mathematics and Statistics, University of Port Harcourt, Nigeria.
Email: |  |
Copyright © 2016 Scientific & Academic Publishing. All Rights Reserved.
This work is licensed under the Creative Commons Attribution International License (CC BY).
http://creativecommons.org/licenses/by/4.0/

Abstract
In this paper, we estimate the missing values in the outcome of an experiment that involved four different types of poultry birds fed over a period of eighteen months in four feed weight replicates per month. The outcome which is the number of eggs produced monthly by the birds is stochastically dependent on two inputs (feed weight and months). The study assumed a cubic polynomial interaction that follows a binomial series expansion between the inputs. A regression equation was used to link the outcome and the input interaction. The regression coefficients of the link function was determined by the method of ordinary least square via multiple imputation technique while replacing the missing values with the available outcomes in turn. So for each imputation, there is a corresponding likelihood value obtained as estimate of the missing data from the regression equation. The probability of the chi-square goodness of fit was then used to determine the maximum likelihood value of the missing data by plotting the graph of the obtained chi-square density against the available outcomes.
Keywords:
Missing values, Cubic interactions, Multiple imputations, Chi-Square density
Cite this paper: Ogoke U. P., Nduka E. C., Soyinka A. T., A Chi-Squared approach to Obtaining Missing Values on Egg Production, International Journal of Statistics and Applications, Vol. 6 No. 6, 2016, pp. 386-390. doi: 10.5923/j.statistics.20160606.07.
1. Introduction
Missing data is an important aspect of statistical science that involves the unavoidable absence of an information as a result of factors which includes no response from the respondent due to acclaimed privacy violation, dropout of respondent in the middle of a research procedure and so making further information not available and finally as a result of initial improper capturing of critical statistics thus making such data inappropriate as a secondary source. However due to the fact that missing data reduce the representativeness of the sample and can therefore distort inferences about the population because majority of the statistical tools requires a complete data set for analysis; then there is need to prevent missing data or to properly estimate missing data before proceeding for further analysis. In situation where missing data are likely unavoidable, the researcher is advised to use statistical methods like statistical design and sampling techniques that allow for robustness in case of missing values. Hence the ultimate aim is to develop properly modeled statistical methods to estimate the missing values so that research work will not die prematurely. There are two major methods of estimating missing data in statistical science which are multiple imputations (MI) and maximum likelihood (ML) methods. Recently, modification of multiple imputations via local least square imputation (LLS) and Bayesian principal component analysis (BPCA) have been commonly used along with some form of neural network approach. Though MI and ML has been extensively used over years for estimating missing data, the precision and accuracy of the obtained estimate via sufficient statistics and standard error computation is still sketchy and difficult. Hence in this study, we bridged the advantages of MI and ML to obtain likelihood estimates of a missing data and confirm the most appropriate maximum likelihood estimate via chi square density approach (Aguilar (2003) [1], Allison (2006) [2], Stoop et al (2010) [3]).
2. Model Assumption
Let the number of eggs produced
be dependent on the input factors feed weight
and months
. Then
is a function of the interaction between feed weight
and months
that is | (1) |
Assuming that both the feed weight
and months
has different levels of cubic, quadratic, linear and constant interaction effect on the birds then by binomial expansion | (2) |
we obtain the general stochastic relationship 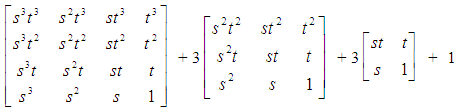 | (3) |
which result into the general regression equation  | (4) |
The matrix of the regression coefficients from (4) is  | (5) |
For the period of eighteen months, the regression model is thus  | (6) |
The design matrix for the feed weights (s) and months (t) interaction is given by the rectangular matrix (18 x 16) equation (7).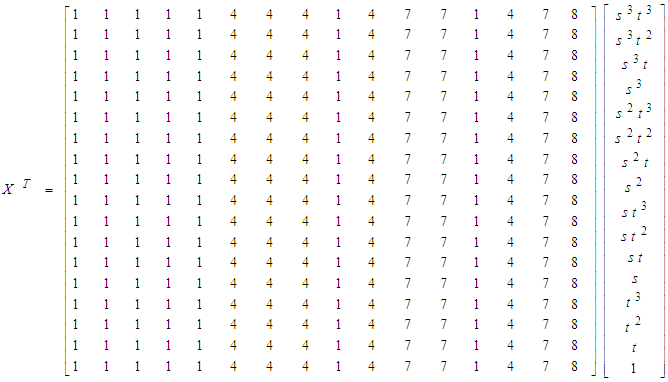 | (7) |
3. Application
The matrix of the egg produced for each month by the different categories of birds along with its missing spots is given below in equation (8)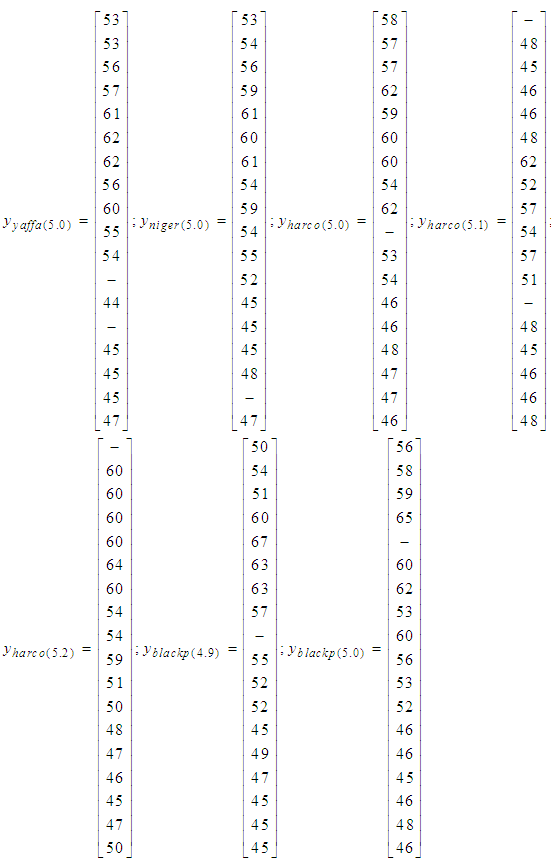 | (8) |
4. Methodology
Missing values Estimation stepsa. Substitutes the missing values in turn that is one after the other with each of the available outcome
.b. At each substitution obtain regression coefficients
and estimate the missing value
.c. For each available outcomes
denoted as
there is corresponding equivalent value estimated for the missing values (-) denoted as
.d. Recall the chi square goodness of fit. Then evaluate
based on the null hypothesis
. That is we obtain the observed value
that has the maximum chi-square probability of not been significantly different from the missing value estimates. e. Then the Maximum Likelihood Estimate for the missing value is located at the point where the graph of
against
and
is minimum or at the point where the density of the chi square calculated against
and
at specified degree of freedom k is maximum.
5. Data Analysis and Result
1. Estimating the missing value in the column
with the feed weight of
(column 6) in equation (8).
 | Table 1. |
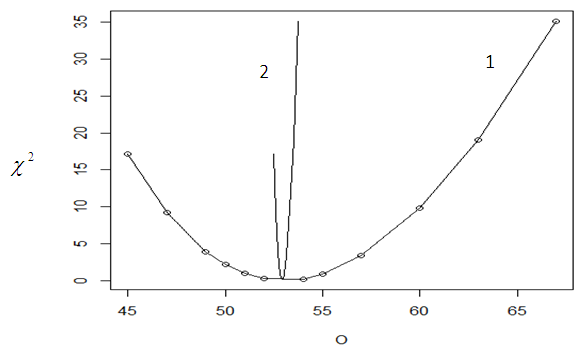 | Figure 1. Graph of Chi-square value against the observed O {1} and the expected value {2} |
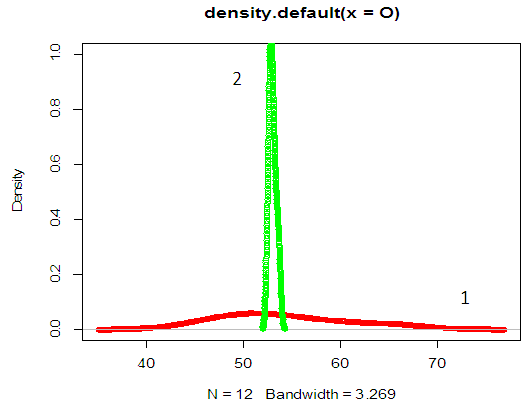 | Figure 2. Graph of probability density against observed O {1} and the expected values {2} |
The value of the missing spot is 52.2. Estimating the missing value in the column
with the feed weight of
(column 1) in equation (8).
 | Table 2. |
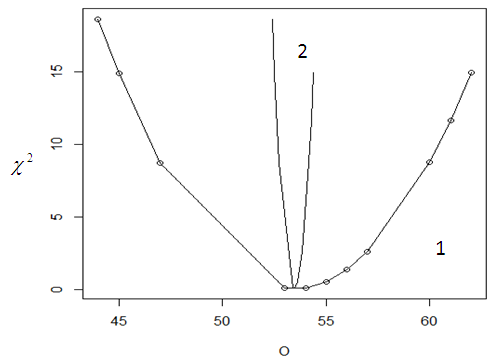 | Figure 3. Graph of Chi-square value against the observed O {1} and the expected value {2} |
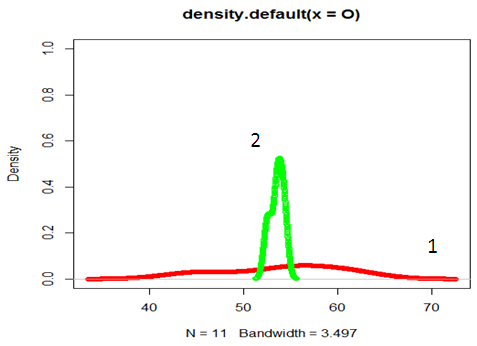 | Figure 4. Graph of probability density against observed O{1} and the expected values {2} |
The value of the missing spots is 54 a piece. Extending the procedure to other columns in equation 8 the estimate of the missing values are
;
;
;
;
. See appendix for the r program.
6. Conclusions and Recommendations
The chi square approach to estimating likelihood estimate of missing value(s) in data sets via multiple imputations and obtaining its maximum likelihood estimate via area under a chi square density is an approach that is easy to apply in practice. The approach is applicable to any factorial experiment where levels of each factors as well as its interactive factor levels are well defined. The missing data can then be estimated from other available outcomes with similar factor-level interaction. The approach can also be used to estimate missing data as a result of subject that drops out from a particular or joint intervention(s) in medical research.
References
[1] | R.S. Aguilar, “Missing value estimation methods for DNA microarrays,” Statistics and Genomics Seminar and Reading group, 2003. |
[2] | P.D. Allison, “Modern Methods for Missing Data Statistical,” Horizons LLC, 2006. |
[3] | I. Stoop, J. Billiet, A. Koch, and R. Fitzgerald, “Improving survey response lessons learned from European social survey,” John wiley ISBN 0-470-51669-0, 2010. |
[4] | E. Craig, “Applied Missing Data Analysis,” Guilford Press New York, 2010. |
[5] | J.L. Roderick and D. Rubin, “Statistical Analysis with Missing Data” John Wiley & Sons, Inc: Hoboken 2002. |
[6] | D. J. Fogarty, 2006. “Multiple imputation as a missing data approach to reject inference on consumer credit scoring,” Interstat. URL, 2006. |
[7] | A. Dempster, N. Liard and D. Rubin, “Maximum likelihood from incomplete data via the EM algorithm (with discussion)” Journal of Royal Statistcal. Society B39, 1977, pp 1-38. |
[8] | K. Lakshminarayan, S. Harp, and T. Samad, “Imputation of missing data in industrial databases,” Applied Intelligence 11 (3), 1999, 259 -275. |
[9] | D.B. Rubin, “Multiple imputation for Nonresponse in Surveys” New York: John Wiley and Sons, 1987. |
[10] | J.L. Schafer and J.W. Graham, “Missing Data: Our View of the State of the Art” Psychological Methods, 2002. |