I. A. Iwok, N. P. Akpan
Department of Mathematics/Statistics, University of Port-Harcourt, Port-Harcourt, Nigeria
Correspondence to: I. A. Iwok, Department of Mathematics/Statistics, University of Port-Harcourt, Port-Harcourt, Nigeria.
Email: |  |
Copyright © 2016 Scientific & Academic Publishing. All Rights Reserved.
This work is licensed under the Creative Commons Attribution International License (CC BY).
http://creativecommons.org/licenses/by/4.0/

Abstract
This work focused on Bayesian inference of Nigerian 2015 presidential polls. Prior beliefs about the proportion
prior means and variances of those who favour the two most popular political parties were obtained prior to conducting the election. Based on this information, beta priors
distribution that satisfy these beliefs about
were obtained. As Bayesian tradition demands; election was later conducted and data obtained. From here, the posterior distribution that summarizes the beliefs about
was calculated. The posterior means were also calculated and were found to be Bayesian credible. Thus, prior belief of Nigerians was the basis of which the election was won and lost.
Keywords:
Bayesian concept, Prior probability, Posterior probability, Beta priors and Bayesian credible interval
Cite this paper: I. A. Iwok, N. P. Akpan, Bayesian Approach to Electoral Processes, International Journal of Statistics and Applications, Vol. 6 No. 5, 2016, pp. 309-313. doi: 10.5923/j.statistics.20160605.05.
1. Introduction
Election is a vote to select the winner of a position or political office. Election mainly involves politics but sometimes it can be done by appointment and it is a yardstick for going into political offices. Based on this we can define politics as the social relations involving intrigue to gain authority or power. On the process of an election, a candidate of a particular party wins the election by means of winning the vote of the majority of the population. The winner is expected to serve the people for a particular period of time. Throughout the world, elections are used in selecting leaders to pilot the affairs of many nations. Conducting elections is seen as providing legitimacy to elected leaders, as long as they are conducted fairly and with integrity. However, in some countries like Nigeria, they are electoral fraud and potential manipulation of the electoral process. Some party supporters are said to be sharing monetary gifts, bribing officials, using force on individuals, stealing ballot boxes, etc. in other to manipulate and acquire their votes and this have caused uprising concerns among Nigerians. Provoked by these controversies, mathematicians and statisticians have tried to find out a suitable technique to analyze electoral processes following the electoral conducts. Bayesian inference seems to be the appropriate method for handling issues of this nature.
2. Literature Review
In statistics, Bayesian inference is a method of inference in which Bayes’ rule is used to update the probability estimate for a hypothesis as additional evidence. For some cases, exhibiting a Bayesian derivation for a statistical method automatically ensures that the method works as well as any competing method. In the 17th century the term Bayesian was first used by reverend Thomas Bayes’, who proved a special case of what is now called the Bayes’ theorem in his book tittled “Essay towards solving a problem in the doctrine of chances”.Historically, Laplace introduced a general version of the theorem in 1760 and used it to approach problems in celestial mechanics, medical statistics, reliability, and jurisprudence. Early Bayesian inference, which uses uniform priors following Laplace’s principle of insufficient reason, was called “inverse probability” because it infers backwards from observations to parameters, or from effects to causes. In the 20th century, the ideas of Laplace were further developed into two different views giving rise to objective and subjective view in Bayesian practice. In the objective or “non-informative” view, the statistical analysis depends on only the model assumed, the data analyzed and the method assigning the prior, which differs from one objective Bayesian to another. In the subjective or “informative” view, the specification of the prior depends on the belief (that is, propositions on which the analysis is prepared to act), which can summarize information from experts, previous studies etc. Cox (2001) in his work on “Algebra of probable inference” showed that the Bayesian updating follows from several axioms, including two functional equations and a controversial hypothesis of differentiability. However, it was shown by Cox and Hinkley (2003) that the assumption of differentiability or continuity is questionable since the Boolean algebra of statements can only be finite.Wald (2005), in his work in “statistical decision function” of Bayesian probability proved that every admissible statistical procedure is either a Bayesian procedure or a limit of Bayesian procedures. According to him, all phenomena in nature has a tendency of displaying Bayesian tendencies.Karl and David (2005) in their work “critical rationalism”, rejected the alleged rationality of Bayesianism of using Bayes’ rule to make epistemological inference. According to this view, a rational interpretation of Bayesian inference would see it merely as a probabilistic version of falsification, rejecting the belief, commonly held by Bayesians, that high likelihood achieved by a series of Bayesian update would prove the hypothesis beyond any reasonable doubt, or even with likelihood greater than zero. Steel (2014) described the need of Bayesian methods in time series. He employed the Markov chain Monte Carlo methods to solve problems of some complex time series models amendable to Bayesian analysis. The result showed that fractionally integrated series can be modeled by the Bayesian Approach.Efstathios et al (2007) investigated the usefulness, of Decision trees, Neural Networks and Bayesian belief Networks in the identification of fraudulent financial statements. The input vector was composed of ratios derived from financial statements. Three data mining techniques in detecting fraudulent financial statement were used. The result obtained agreed with prior results indicating that published financial statement data contains falsification indicator. It was also found that a relatively small list of financial ratios largely determined the classification results. The obtained models were capable of achieving considerable classification accuracies.Chen (2016) constructed a valid and rigorous fraudulent financial statement detection model. Companies which experienced both fraudulent and non-fraudulent financial statements between the years 2002 and 2013 were used as research objects. The work consisted of two stages. The first stage consisted of two decision tree algorithms, including the classification and regression trees (CART) and the chi-squared automatic interaction detector (CHAD) being applied in the selection of major variables. The second stage combined CART, CHAD, Artificial neural network and Bayesian belief network to construct fraudulent financial statement detection models. The result showed that the detection performance of the CHAD-CART model was the most effective, with an overall accuracy of 87.97%.Smith (2008) used participatory systems analysis to construct system models of the operating environment for fire management in conservation reserves in North Queensland, Australia. The study aimed at identifying stumbling blocks to the adaptive management of five and to test whether it could be done using participatory methods and systems modeling tool called Bayesian belief networks (BBN). The results indicated that the participatory system analysis approach provides a co-learning environment that captures the collective knowledge of the factors influencing planning, implementing, monitoring and receiving outcomes; thus allowing critical success factors (CSF) that influence the success of adaptive management to be identified. The BBN provided the scaffolding for piecing together Bayesian knowledge, allowing managers to structure complex problems and conduct dynamic sensitivity and scenario analysis to identify where intervention or investment can significantly improve the practice of adaptive management within a natural resource management (NRM).Wolfe et al (2012) examined semantic coherence and internal inconsistency fallacies in conditional probability estimation. The problems reflected five distinct relationships between two sets with special case of overlapping sets. It was discovered that inconsistency occurs when the constellation of estimate does not conform to Bayes theorem. Semantic coherence was found to occur when constellation of estimates is consistent with the depicted relationship among sets. Fuzzy-trace theory was applied and it was predicted that people have difficulty with over-lappy sets and subsets because they required class-inclusion reasoning. It was also discovered that identically, mutually exclusive and independent sets yielded superior performance than subsets and overlapping sets. Also, there was a strong correlation between inconsistency in conditional probability estimation and conjunction fallacies of joint probability estimation, suggesting that similar fallacies reasoning process produce these errors.Keneth (2014) used the least informative priors to study the validity of the gubernatorial elections in Ghana. The least informative priors was used to give a description of a class of prior in which the goal is to minimize the amount of subjective informative content and to use a prior that is determined solely by the model and the observed data. The result of the work supported the decisions of the actual elections and according to him; the data was able to speak for itself. Stephen (2014) used Jeffrey’s priors in Bayesian time series to forecast the voting strength in his local government elections in Nigeria. The method was an attempt to establish a least information prior that is invariant to transformations. In his results, the forecasts generated were found to give minimum errors when compared with other existing methods. However, the use of Jeffrey’s priors was found to work well for a single parameter while multi-parameter situations were found to have some detrimental effects.
3. Methodology
3.1. The Bayesian Basic Concept
In non-Bayesian Statistics, the density function
of a random sample
is assumed known while the parameter
is fixed but unknown. However, in Bayesian Statistics, the reverse is the case. The parameter
with possible values
is assumed to be a random variable while the random variable
with possible values
becomes fixed and depend on
If
is a random variable; then it has a probability distribution.
3.2. Prior Probability Distribution
The prior distribution of a parameter
is a probability function that expresses the degree of belief about the value of
prior to observing a sample of a random variable
whose distribution function depends on
Given the prior density
and the conditional density of the elements of a sample
the joint density of the sample and parameter is | (1) |
The marginal density of the sample values is | (2) |
3.3. Posterior Distribution
The posterior density for
is the conditional density of
given the sample values. Thus,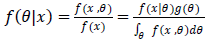 | (3) |
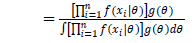 | (4) |
3.4. Bayesian Binomial Proportion
For a binomial process, | (5) |
Now, if
is held fixed and
varies over its possible values, we have the likelihood function, | (6) |
From Bayes’ posterior distribution theorem, | (7) |
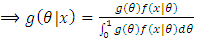 | (8) |
3.5. Beta Prior
In Bayesian analysis, possible choices of priors are available. In this work, however, we choose to entertain the Beta prior because of its features and relevance.Let
be the Beta prior density used for
so that | (9) |
From equation (6), (7) and (9), | (10) |
The constant
and
does not have significant effect on the distribution and so can be ignored. Thus, we have the Bayes theorem for posterior distribution to be, | (11) |
Observantly, equation (11) is a Beta distribution with parameters
and
Replacing the proportionality sign with equality in equation (11); we have | (12) |
Thus, the Beta distribution is the conjugate family of the binomial distribution.
3.6. Prior Mean and Variance
Let
be the prior mean and
the prior standard deviation.For
distribution, the prior belief about the mean of the proportion can thus be expressed as | (13) |
and the prior belief about the standard deviation of the proportion is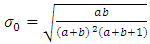 | (14) |
From equation (13),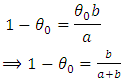 | (15) |
Substituting (15) in (14), we have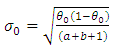 | (16) |
3.7. The Posterior Mean
The posterior mean
Thus, when
is 
 | (17) |
3.8. The Posterior Variance
The posterior variance
For 
 | (18) |
3.9. Bayesian Credible Interval for 
The
credible region for
is approximately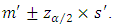 | (19) |
4. Data Analysis
4.1. Data Collection
The actual data used for this work is the 2015 election results of the two strong contending political parties in Nigeria. The parties are the All Progressive Congress (APC) and the Peoples Democratic Party. Other remaining parties are not included because they are not popular.For the Bayesian approach to be applied, the data has to be collected at two stages. The first stage involves obtaining the values of
prior to conducting the 2015 elections in Nigeria. The values of
at this stage represented the proportion of Nigerians who supported the ruling party (PDP) and the opposition party (APC) at the National, State and the Local Government level before (prior) the election was conducted. The values of these prior beliefs were computed from the pilot test figures conducted and published by Nigerian Media Association (NMA). The summary of the prior beliefs
the prior means
and the prior variances
for the two outstanding parties are presented in table 1 below.Table 1. Prior Parameters for APC and PDP |
| |
|
This first stage provides information for the Beta priors
that will be used to obtain the posterior distribution
The second stage involves the actual data obtained after the election has been conducted. The actual data is used with the
to obtain the
that summarizes the belief about 
4.2. Analysis
We shall apply the Bayesian approach to the two political parties (APC and PDP) separately.
4.2.1. All Progressive Congress
Applying equations (13) and (16) to the values of the prior means and variances, we have | (20) |
 | (21) |
From equation (20),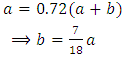 | (22) |
From equation (2) we also have 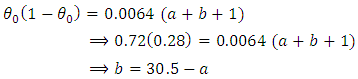 | (23) |
Equating equations (22) and (23) we have,
Substituting
in equation (23) gives
Hence, the prior distribution is
4.2.1.1. The Posterior DistributionFor the posterior distribution,
where
is the number of Nigerians who voted for APC.
where
is the total number of vote.Hence, the posterior distribution is
Then, the posterior mean is
and the posterior variance is
4.2.1.2. Credibility of the Posterior MeanThe Bayesian credible interval,
is
Testing for the credibility of the posterior mean
where
is the Bayesian estimate of the posterior mean and
Since
lies inside the credible interval
the null hypothesis cannot be rejected. Hence, the posterior mean is Bayesian credible.
4.2.2. Peoples Democratic Party
 | (24) |
 | (25) |
From equations (24) and (25), we have | (26) |
and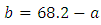 | (27) |
Equating equations (26) and (27) we obtain,
Substituting
in equation (27) gives
Hence, the prior distribution is
4.2.2.1. The Posterior Distribution
Hence, the posterior distribution is
Now,
and
4.2.2.2. Credibility of the Posterior MeanThe Bayesian credible interval,
is
Testing for the credibility of the posterior mean
where
is the Bayesian estimate of the posterior mean and
Since
lies inside the credible interval
the null hypothesis cannot be rejected. Hence, the posterior mean is Bayesian credible.
5. Discussion and Conclusions
The use of Bayesian inference in analysis seems not to be much in practical use. With this work, we have seen that Bayesian inference can be applied just like any other statistical inference. Since the posterior mean is Bayesian credible, the prior belief of the people is the basis of which the election was won and lost. Though with high prior variance, the prior belief for APC reflected the highest value which on the course of the analysis produced the minimized posterior Beta parameters. The posterior distribution has summarized the prior belief about
and is therefore not surprising that APC won the election.
References
[1] | Chen, S. (2016). Detection of fraudulent financial statements using the hybrid data mining approach. Springer Plus 2016; 5: 89. DOI: 10.1186|540064-016-1707-6. |
[2] | Cox, K.R. (2001). Algebra of probable inference. Journal of Agebra and Probability. Vol. 2, issue 4, pp. 28-35. ISBN 0-07-331257-1. |
[3] | Cox, H.A. and Hinkley, D.V. (2003). Journal of theoretical Statististics. Vol. 7. pp. 41-50. ISBN 0-05-443214-1 |
[4] | Efstathios K., Spathis, C. and Manolopoulos Y. (2007). Data mining technique for the detection of fraudulent financial statements. Expert systems with applications 32(2007)995-1003. www.sciencedirect.com. |
[5] | Karl, P. and David, M. (2005). Critical Rationalism, the Logic of Scientific Discovery. 2nd Edition, Routledge. pp. 57. ISBN: 0-415-27843-0. |
[6] | Keneth, E.H. (2014). Bayesian concept on election matters. A case study of Ghana’s gubernatorial election. International Journal of Political Scienc, Vol.3, No. 8, pp. 72-81. ISBN: 0-322-41267-7. |
[7] | Steel, M. (2014). Application of Bayesian Principle in Time Series Analysis. Journal of Economic and Statistics. Vol. 30; 14 – 22. |
[8] | Stephen, K.S. (2014). Bayesian approach to time series using Jeffrey’s. International Journal of Physical Science. Vol. 9; No. 12. pp. 61-69. ISBN: 0-1427-32411-6. |
[9] | Smith, C., Feldehof, L. and Bosch, O.J. (2008). Adaptive management: making it happen through participatory systems analysis. System research and behavioural science. Vol. 24, Issue 6, pp. 567-587. DOI:10.1002|sress.835. |
[10] | Wald, K. (2005). On Statistical Decision Functions. Journal of Mathematical Functions. Vol. 9, issue 3, pp. 21-30. ISBN: 0-02441224-1. |
[11] | Wolfe, C. F., Fisher, C.R. and Reymand V.F. (2012). Journal of Behavioural Decision Making. John Wiley and Sons limited. DOI: 10.1002|bdm.1756. |