S. O. Adeyemo1, F. N. Nwobi2
1Department of Mathematics and Statistics, Federal Polytechnic, Nekede, Owerri, Imo State, Nigeria
2Department of Statistics, Imo State University, Owerri, Imo State, 460222, Nigeria
Correspondence to: S. O. Adeyemo, Department of Mathematics and Statistics, Federal Polytechnic, Nekede, Owerri, Imo State, Nigeria.
Email: |  |
Copyright © 2014 Scientific & Academic Publishing. All Rights Reserved.
Abstract
Estimable functions of the parameters are characterized in terms of generalized inverses. The concept of estimability is applied to data from a designed experiment on varietal trials. We demonstrate in this note that this technique of solving the normal equations is equivalent to the nearest neighbour method for the analysis of unbalanced randomized design.
Keywords:
BLUE, Estimable Functions, Estimability, Generalized Inverses, Less than Full Rank, Linear Combination, Linear Models
Cite this paper: S. O. Adeyemo, F. N. Nwobi, A Note on Estimability in Linear Models, International Journal of Statistics and Applications, Vol. 4 No. 4, 2014, pp. 212-216. doi: 10.5923/j.statistics.20140404.06.
1. Introduction
Linear models are generally of the form | (1) |
(where y is an
observation vector, X is an
design matrix of fixed constants having rank r
,
is an
vector of unknown parameters,
is an
vector of unknown random errors having zero means) and
. The Ordinary Least Square (OLS) solution of (1) is
, a unique solution. In practice, not all linear models of the form in (1) are of full rank. When X is not of full rank, then
is singular and the normal equations
do not have a unique solution. However, there are various approaches of obtaining the inverse of singular matrices, for which the row echelon form given by Elswick et al (1991), Moore Penrose and the generalized inverse, Searle (1977) are popular in the literature. The generalized inverse is the approach we apply in this paper.
2. Form of Estimability
With X less than full rank and
singular, i.e.
, there is an infinite number of solutions of
to the normal equations. Attention is therefore directed not to the solutions themselves but to linear functions of their elements. Consider a linear function
of the parameters in
, where
is a known vector. This linear function is defined as being an estimable function if there exists some linear combinations of the observations
whose expected value is
, i.e. if there exists a vector
such that the expected value of
is
, then
is said to be estimable. Consider the following theorem given in Graybill, 1976:Theorem 1. (Graybill, 1976)Assuming a linear model in (1),
is an estimable function if and only if there exist an
vector such that
Proof. If there exist a vector such that
, then,
.Only if: Conversely, if
is estimable, then,
Thus
In addition, Elswick et al (1991) argues that if X is of full rank,
exists and the rows of
matrix
serve as the necessary set of vectors because 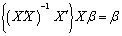
3. Illustration
We demonstrate this discussion by considering the data from a study to compare classical and nearest neighbour methods in the analysis of varietal trials (See, e.g. Nwobi, 2000). In the experiment, nine (9) different varieties of cassava crop were tried, six at a time over a maximum of five years in such a way that these varieties were not replicated equally. The model (without interaction) is given by  | (3) |
where
is the yield from the jth trial of the ith variety,
is the general mean,
is the effect of the ith variety,
is the random error associated with
.Equation (3) is written in matrix form as  | (4) |
Based on the model in (2), the parameter vector
is given by
The components of the model (4) are
from where we obtain
A generalized inverse of
written as
such that
see, e.g. Searle (1977) is
with
and
. The function
is estimable for any given values to the
. With this we obtain the solution to the normal equation as
Therefore, the Best Linear Unbiased Estimator (BLUE) of
is
To see if
where
is estimable, we write the parameter as
where, in this case, we define
, a
matrix;
is of dimension
, so that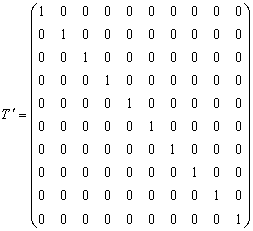
Since
,
is not estimable. However, considering
, this function may be written for
and
as
where
so that
. This implies that
is estimable. Similarly, since there are 9 (nine) parameters, taking two (contrast) at a time gives
estimable functions
Therefore,
. Thus, we can say that a linear combination of estimable functions is estimable.
4. Conclusions
We have shown that for any arbitrary vector
,
is estimable with BLUE
. The solution of the normal equation,
, confirms that this approach is equivalent to the Nearest Neighbour method of analysis of designed experiments. Both methods agree on the selection of varieties though the value of these estimates are not unique due to the application of generalized inverses. Furthermore, we verified that the linear combination of estimable functions is estimable.
References
[1] | Elswick, R.K., Gennings, C., Chinchili, V.M., Dawson, K.S. A Simple Approach for Finding Estimable Functions in Linear Model. The American Statistician, Vol 45, No 1. Feb 1991. pp. 51-53. 1991. |
[2] | Searle, S. Linear Models. Wiley, New York. (1977). |
[3] | Graybill, F.A. Theory and Applications of the Linear Model. Belmont, C.A: Wadsworth. (1976). |
[4] | Nwobi, F.N. A Comparison of Classical and Nearest Neighbour Methods in the Analysis of Varietal Trials. Journal of Sustainable Agriculture and Environment. Vol. 2 No.1. pp.62-67. (2000). |