Ganesh Gopal Masti Jayaram
Infosys Quality Engineering, Infosys Limited, City, USA
Correspondence to: Ganesh Gopal Masti Jayaram, Infosys Quality Engineering, Infosys Limited, City, USA.
Email: |  |
Copyright © 2024 The Author(s). Published by Scientific & Academic Publishing.
This work is licensed under the Creative Commons Attribution International License (CC BY).
http://creativecommons.org/licenses/by/4.0/

Abstract
Organizations across industries are increasingly recognizing the critical importance of data quality for IT success, particularly in today’s complex enterprise environments. Poor data quality, often exacerbated by siloed systems, hinders growth and prevents organizations from unlocking valuable insights. This article will cover how data quality directly impacts business decisions, how poor data can lead to missed opportunities for revenue growth, and how consistent, accurate data unlocks strategic value across the enterprise. A sample implementation from a large-scale organization will showcase how incorporating data quality checks can transform siloed data into a strategic asset, with a demonstration of how these improvements support better decision-making.
Keywords:
Enterprise Data Quality, Strategic Data Transformation, Data Quality Framework
Cite this paper: Ganesh Gopal Masti Jayaram, Data Quality - A Prerequisite for Organizational Success, Software Engineering, Vol. 11 No. 3, 2024, pp. 35-40. doi: 10.5923/j.se.20241103.01.
1. Introduction
The customer landscape is rapidly evolving, where physical experiences are converging with virtual spaces, the real is meeting e-commerce, and live events are now accessible on demand. This shift means that customers are going digital, and for organizations, “going digital” involves much more than technology—it signifies a profound transformation where human relationships are increasingly digitized. Why is this important? In this evolving landscape, organizations must build the capability to engage with customers wherever they are and through any channel they prefer. Customer expectations have risen, demanding brands to be accessible, responsive, and well-informed in every interaction. However, effectively meeting these expectations hinges on one key factor, high-quality data. Data quality becomes the bedrock for sustaining these human relationships, ensuring organizations have accurate, consistent, timely insights to engage customers meaningfully. Therefore, the key to successfully digitizing these human relationships lies in the reliability of trusted data.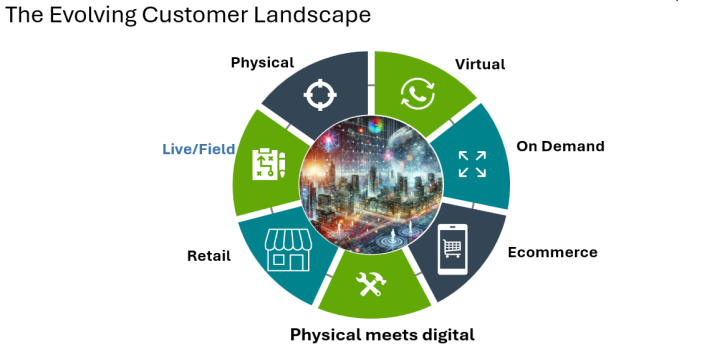 | Figure 1. The Evolving Customer Landscape |
2. Literature Review
As customer interactions increasingly migrate to digital platforms, organizations face the dual challenge of meeting heightened customer expectations and managing complex data ecosystems. According to Gartner (2023), 91% of organizations recognize the importance of delivering consistent customer experiences across multiple touchpoints. However, achieving this requires more than digital technologies; it necessitates integrating data from diverse sources to create a cohesive understanding of customer needs [1].High-quality data is a critical enabler of effective customer engagement. Poor data quality has been cited as a significant barrier to digital transformation, with McKinsey & Company (2022) noting that organizations with unreliable data systems experience 30% higher operational inefficiencies [2]. Furthermore, bad data can erode customer trust, leading to misaligned messaging, inaccurate recommendations, and failed interactions [3]. Data quality is multifaceted, encompassing accuracy, consistency, timeliness, and completeness [4]. Accurate data ensures that customer information is correct and actionable, while consistency ensures uniformity across systems. Timely data ensures that insights are relevant when decisions are made, and completeness ensures no critical information is missing [5]. The humanization of digital customer engagement hinges on trusted data. Harvard Business Review (2022) highlights that good data is crucial for AI-driven personalization, enabling organizations to tailor interactions, predict customer needs, and build long-term relationships. AI tools such as chatbots, recommendation systems, and predictive analytics depend heavily on accurate and structured data to function effectively and deliver value [6]. For instance, AI-driven tools like chatbots and recommendation engines rely heavily on clean, structured data to function effectively. Conversely, poor data quality limits the capabilities of these systems, resulting in customer dissatisfaction and revenue loss [7]. Improving data quality requires robust data governance frameworks, regular audits, and advanced tools for data validation and cleansing. As the digital landscape continues to evolve, the reliance on high-quality data will only grow. IDC (2023) predicts that by 2025, 70% of customer interactions will be influenced by AI-powered systems, underscoring the critical need for reliable data [8]. Organizations that prioritize data quality today will be better positioned to meet future challenges, maintaining meaningful connections in an increasingly digital world. Building on these insights, this article introduces the DATA SMARTS methodology—a targeted approach to tackling data quality challenges by harnessing the 5V's—Volume, Velocity, Variety, Veracity, and Value. By emphasizing standardization, robust governance, and continuous validation, DATA SMARTS transforms data into a strategic asset, enabling organizations to drive revenue growth and make informed decisions.
2.1. The Cost of Poor Data Quality on Business Agility and Growth
Poor data quality significantly undermines an organization’s ability to make sound business decisions, drive revenue growth, and create strategic value. Inaccurate or inconsistent data obstructs the flow of reliable insights, ultimately hampering the agility that businesses need to adapt and thrive in today’s fast-paced environment. When decision-makers rely on flawed data, they risk pursuing ineffective strategies, misallocating resources, and missing revenue opportunities. For instance, unreliable customer data can lead to misdirected marketing efforts, inflating costs without delivering meaningful engagement. Additionally, data inconsistencies across systems create operational inefficiencies, heightening the risk of errors and slowing down critical processes. Beyond operational setbacks, the broader impact of poor data quality is felt in diminished strategic positioning. Without trustworthy data, organizations lose their ability to extract valuable insights, which erodes the foundation for informed, impactful decision-making.
3. Understanding the 5 Vs of Big Data: The Foundation for High-Quality Data
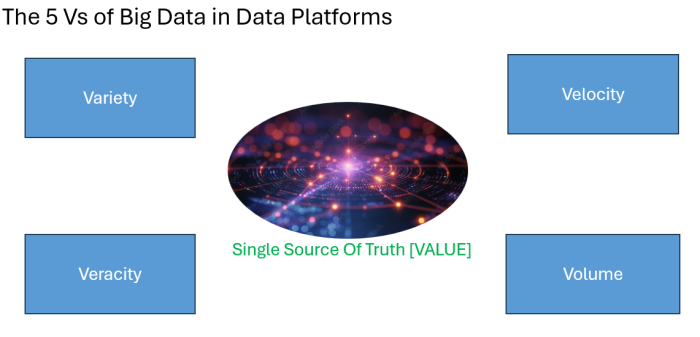 | Figure 2. The 5V’s of Big Data |
In the realm of big data, understanding the 5 V’s—Volume, Velocity, Variety, Veracity, and Value—is essential for building a robust data strategy. These dimensions not only define the unique characteristics of big data but also emphasize the importance of data quality in deriving meaningful insights.
3.1. Volume
The sheer volume of data available today is unprecedented. With data generated from countless sources—social media, transactions, IoT devices, and more—organizations are faced with managing massive quantities of information. Handling large volumes effectively requires scalable storage solutions and efficient processing capabilities, making it vital to filter out irrelevant data and maintain high-quality datasets for reliable insights.
3.2. Velocity
Velocity refers to the speed at which data is generated, captured, and processed. In fast-paced environments, data must be processed in real-time or near-real-time to enable agile decision-making. High-quality data at this speed requires efficient pipelines and robust systems to ensure that data remains accurate and usable as it flows through the organization.
3.3. Variety
Variety encompasses the different forms data can take—from structured data like databases to unstructured formats such as text, video, and audio. Managing this diversity is challenging, as each type of data presents unique quality considerations. Consistent data quality across varied data types ensures that the organization can draw accurate insights from any data source.
3.4. Veracity
Veracity is the measure of data’s accuracy and reliability. With data coming from multiple sources, it’s crucial to assess its quality and consistency to avoid misleading insights. Veracity is often the hardest to achieve but is essential for maintaining the integrity of data-driven decisions. Without high veracity, data may become “dirty,” leading to skewed analytics and flawed strategies.
3.5. Value
Finally, deriving Value is the aim of enterprises. Transforming raw information into actionable insights that support business objectives. High-quality data ensures that the insights derived hold genuine value, empowering organizations to make informed decisions that drive growth, improve customer experience, and create strategic advantages. To fully leverage the 5V’s of big data—Volume, Velocity, Variety, Veracity, and Value—organizations should first adopt a strategic approach to taming these dimensions. This involves implementing scalable storage solutions and robust processing pipelines to handle large volumes of data efficiently while filtering out irrelevant information. Real-time data pipelines and validation systems are critical for maintaining data accuracy at high speeds, enabling agile decision-making. For diverse data types, standardization frameworks and tailored quality benchmarks ensure consistent insights across structured and unstructured data sources. Rigorous validation processes, regular audits, and governance practices are essential to uphold data integrity and minimize inaccuracies. Ultimately, organizations should focus on deriving actionable value from their data by aligning it with strategic goals, ensuring that high-quality data translates into meaningful business outcomes such as revenue growth, operational efficiency, and customer satisfaction. The 5Vs of big data collectively underscore the importance of data quality as a foundation for effective analytics. By prioritizing these dimensions, organizations can not only manage their data more effectively but also unlock their full potential to drive innovation and achieve strategic success.
4. The Value of a Single Source of Truth: Enhancing the 5V’s of Big Data for Strategic Advantage
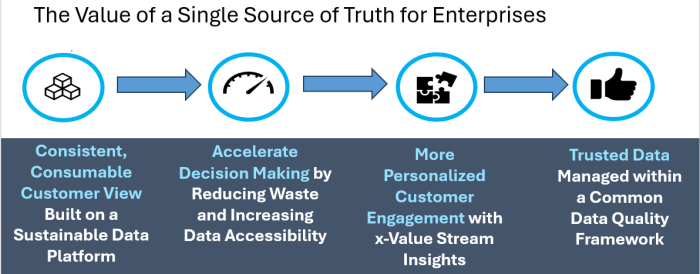 | Figure 3. The The Power of a Single Source of Truth |
To fully leverage the 5V’s of big data, organizations must establish a single source of truth - a unified, reliable data foundation that eliminates silos, enhances data integrity, and enables seamless collaboration for strategic growth. A single source of truth (SSOT) brings profound value to organizations, addressing the 5V’s of big data—Volume, Velocity, Variety, Veracity, and Value—by creating a unified, reliable view of data across the enterprise. To better understand how organizations can establish this foundation, let’s explore a mechanism for building an effective SSOT. To achieve an effective SSOT, organizations must adopt robust mechanisms to unify and manage their data ecosystem. These mechanisms include.
4.1. Data Governance Frameworks
Begin by establishing clear policies, standards, and processes to ensure data quality, security, and compliance. A strong governance framework lays the foundation for reliable and consistent data management, which is critical to building an SSOT.
4.2. Master Data Management (MDM)
Next, create a central repository for critical data elements to standardize definitions, eliminate duplication, and maintain consistency. MDM ensures that foundational data is accurate and serves as the cornerstone of the SSOT.
4.3. Data Integration Platforms
Implement tools to integrate data from disparate sources across the organization. This step ensures that all relevant data is connected, harmonized, and accessible within a cohesive framework.
4.4. Metadata Management
Leverage metadata to provide context to the integrated data. By categorizing and annotating data effectively, metadata management enables users to search, retrieve, and interpret information with ease.
4.5. Cloud-Based Data Warehousing
Finally, utilize scalable cloud infrastructure to store and manage data efficiently. Cloud solutions allow for real-time access to data, ensure flexibility, and support seamless integration with other tools without the need for physical centralization.By leveraging these mechanisms, organizations can establish a robust Single Source of Truth (SSOT), creating a reliable foundation for data accuracy, streamlined operations, and strategic decision-making, ultimately driving meaningful outcomes and sustained competitive advantage.
5. Case Study- Enhancing Partner Program Performance Through High-Quality Data
Building on the foundation of a robust Single Source of Truth, let’s explore how high-quality data can drive tangible results in real-world scenarios. One such example is enhancing the performance of partner programs—collaborative initiatives between organizations and their external partners (such as resellers, distributors, or service providers) aimed at driving mutual growth, expanding market reach, and delivering value to end customers. In a partner program enhancement for a tech company, implementing high-quality data practices allowed the organization to gain better insights and drive strategic engagement with its partners. Previously, partner data was fragmented across sales, training, and support systems, creating inconsistencies and limiting visibility into partner performance and needs. Partners were having significant difficulties locating products specific to their needs that could help them achieve growth. The partners spent 50% more time searching for these products and additionally, they faced a significant loss in revenue attribution for commissions on sales, further straining their relationship with the organization and driving them to consider switching to other providers for their hardware and software needs. By setting up a centralized data quality framework, the company unified partner data across all touchpoints, ensuring that every department accessed a single, accurate view of each partner's activity, certifications, and performance metrics. This consistent, high-quality data enabled the partner management team to identify patterns, such as partners with high training engagement but low sales, and proactively offer targeted support. Additionally, the program could now track key performance indicators (KPIs) in real-time, allowing the company to identify high-potential partners early and provide tailored resources to accelerate their growth. Sales teams used these insights to collaborate with partners more effectively, recommending specific products and solutions aligned with each partner’s market focus and strengths. Crucially, the organization was able to accurately attribute sales back to partners for precise commission payouts, while simultaneously avoiding duplicate payouts by having a clearer understanding of customer ownership and transaction history, further enhancing operational efficiency and partner trust. The results of this improvement were substantial. Partner satisfaction increased by 25% due to more personalized engagement, while partner-driven revenue grew by 30%, as the company’s data-driven approach to partner management led to more targeted strategies and stronger partnerships. This example demonstrates how high-quality data can elevate a partner program, transforming fragmented insights into actionable intelligence that drives both partner success and organizational growth.
5.1 The DATA SMARTS Approach: Tackling Data Quality Challenges for Revenue Growth
To enhance partner program performance, we approached data quality through DATA SMARTS—a structured methodology tailored to standardize and enhance data consistency, accuracy, and reliability within the partner program. We approached data quality through DATA SMARTS—a structured strategy to address challenges and drive revenue growth. This approach emphasizes early discovery and cleansing of data, aligning end-to-end validation, and continuously tracking data quality. By adopting unified processes, setting clear data rules, and implementing robust governance, we ensure data accuracy and reliability. Continuous improvement, risk reduction, and stakeholder engagement transform data quality from a technical task into a business asset that delivers measurable insights and value, supporting strategic goals and enhancing decision-making across the organization.The DATA SMARTS methodology offers actionable insights by emphasizing early discovery to identify and cleanse inaccurate data, ensuring a reliable foundation for analytics. It incorporates end-to-end validation processes to maintain data consistency throughout its lifecycle and establishes standardized governance frameworks to enforce data quality rules across teams. Continuous monitoring and improvement are central, leveraging automation and audits to sustain high standards. By directly linking data quality to measurable business outcomes like increased revenue and improved decision-making, the methodology transforms data quality from a back-end task into a strategic enabler. Stakeholder engagement is critical, fostering alignment between data initiatives and organizational goals to ensure long-term success.Continuous improvement, risk reduction, and stakeholder engagement transform data quality from a technical task into a business asset that delivers measurable insights and value, supporting strategic goals and enhancing decision-making across the organization.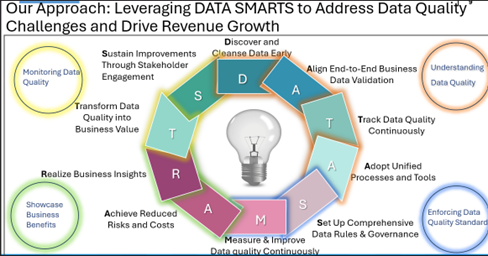 | Figure 4. The DATA SMARTS Framework for Addressing Data Quality Challenges and Driving Revenue Growth |
In summary, the DATA SMARTS approach to data quality focuses on four key areas: Understanding Data Quality by recognizing its impact on decision-making and strategic goals; Enforcing Data Quality Standards through consistent rules, processes, and governance to ensure accuracy and reliability; Showcasing Business Benefits by connecting data quality to clear, measurable outcomes that drive value; and Monitoring Data Quality Continuously to support ongoing improvement and maintain high standards. This comprehensive approach transforms data quality from a back-end task into a strategic enabler of business growth and agility.
5.2. The Turning Point: Harnessing the 5V’s to Transform Partner Program with Robust Data Governance
Recognizing the need for a robust solution, we embarked on a transformative journey, leveraging the principles of the 5V’s of Big Data to establish a foundation for quality and consistency. Velocity (Connect)The first step was to establish connections with diverse data sources, such as Salesforce APIs, Teradata, and Hadoop, to enable the seamless flow of raw data into a centralized system. Using tools like Databricks, we built real-time pipelines to process and validate incoming data. These pipelines not only ensured the efficient flow of data but also centralized it in a secure repository on the cloud, creating a single access point for all incoming information. This allowed us to quickly identify and address data inconsistencies while preparing it for further refinement.Volume (Store & Refine)With data centralized and flowing efficiently, the next step was to organize and store it in platforms such as SQL Server and Snowflake. Snowflake was chosen for its ability to scale seamlessly and support both structured and semi-structured data, enabling efficient querying and processing. This process consolidated over 5 million inaccurate partner profiles into a unified system. By applying an initial set of governance rules, we reduced redundancies, eliminated inaccuracies, and refined the raw data into a structured and reliable form, ensuring it was ready for downstream processing and analysis.Variety (Transform & Model)With centralized data in place, we focused on transforming and modeling it to meet the specific requirements of the partner program. Using tools like Azure Data Factory and SSIS, we standardized and harmonized data from multiple sources—such as sales platforms, CRM systems, and financial tools—into a unified, structured format. While SSIS handled critical ETL tasks, Azure Data Factory provided flexibility in harmonizing diverse data types across cloud and on-premises systems.Veracity (Unify & Validate)To ensure trust and accuracy in the data, we built mechanisms to validate its quality and enforce governance policies. By implementing strict validation rules in SQL Server Management Studio (SSMS) and leveraging automated checks, we identified and eliminated duplicates and errors. These steps ensured that the data adhered to compliance standards and became a true Single Source of Truth (SSOT) for the organization. These tools consolidated partner records into a centralized system, reducing redundancies and inaccuracies.Value (Analyze & Predict)With refined, accurate, and compliant data, we derived actionable insights to drive measurable outcomes. Tools like Power BI and advanced Excel analytics enabled us to predict rebate payouts, resolve sales attribution issues, and support downstream systems like commissions and reporting. This data-driven approach enhanced partner collaboration, optimized program performance, and unlocked significant business value. 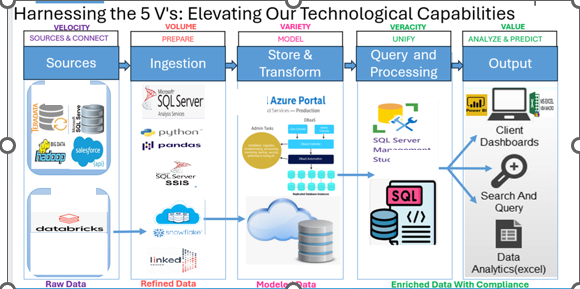 | Figure 5. Leveraging the 5V’s of Big Data to Elevate Partner Program Performance Through Robust Data Governance |
6. The Transformation: Turning Data Challenges into Strategic Opportunities
Through a comprehensive transformation, the company established a robust governance framework grounded in the principles of the 5Vs of Big Data. This ensured data accuracy, consistency, and usability across the organization. Comprehensive processes and automated reporting systems were implemented to proactively identify and resolve missing sales orders and address data quality incidents. These data quality improvements stabilized all downstream systems, including reporting, and commissions. Accurate partner profiles now enable reliable rebate predictions and precise sales attributions, empowering better decision-making and operational efficiency. This transformation not only addressed immediate data challenges but also set a strong foundation for sustained success. By embracing robust governance practices and leveraging the 5 V’s, the organization turned its partner data into a strategic asset, unlocking long-term value for both the company and its partners.
7. Conclusions
In conclusion, championing data quality is more than an operational necessity; it is a strategic enabler. By ensuring reliable systems and fostering accurate, consistent data, IT teams empower organizations to make informed decisions, drive innovation, and respond swiftly to market dynamics. This transformation not only strengthens the company’s operational foundation but also amplifies its strategic value, positioning it for sustained growth, competitive advantage, and long-term success in an increasingly data-driven world.
ACKNOWLEDGEMENTS
I would like to acknowledge the insights and contributions from Gartner, McKinsey & Company, Harvard Business Review, and IDC, which provided foundational perspectives that guided this paper. Additionally, I deeply appreciate the opportunities provided by my clients and employer, whose real-world challenges and collaborative engagements have significantly influenced the development of the DATA SMARTS framework to address data quality. Their support has been instrumental in showcasing how data quality serves as a cornerstone for effective decision-making, innovation, and strategic growth. I also appreciate the continuous efforts of industry leaders and researchers in advancing frameworks that address data quality as a cornerstone of effective decision-making and innovation, which have greatly inspired and guided me in writing this article.
References
[1] | Gartner. (2023). Data Quality: Why It Matters and How to Achieve It. https://www.gartner.com/en/data-analytics/topics/data-quality. |
[2] | McKinsey & Company. (2018). Unlocking success in digital transformations. https://www.mckinsey.com/capabilities/people-and-organizational-performance/our-insights/unlocking- success-in-digital-transformations. |
[3] | Forbes. (2021). Flying Blind: How Bad Data Undermines Business. https://www.forbes.com/sites/forbestechcouncil/2021/10/14/flying-blind-how-bad-data-undermines-business/. |
[4] | Redman, T. C. (2008). Data Driven: Profiting from Your Most Important Business Asset. Harvard Business Review Press. |
[5] | Hevo Data. (2022). What is Data Quality Management? Best Practices & Techniques https://hevodata.com/learn/what-is-data-quality-management/. |
[6] | Harvard Business Review. (2022). Customer Experience in the Age of AI. https://hbr.org/2022/03/customer-experience-in-the-age-of-ai. |
[7] | Monte Carlo Data. (2022). 5 Examples Of Bad Data Quality. https://www.montecarlodata.com/blog-bad-data-quality-examples/. |
[8] | IDC FutureScape: Worldwide Future of Customer Experience 2023 Predictions. https://www.idc.com/getdoc.jsp?containerId=US48543222. |