Mikhailenko I. M. 1, Dragavtsev V. A. 2
1Scientific deputy director, head of lab of informative-measuring systems
2Main scientist of plants ecologic physiology lab., Institute of Agrophysics, St.-Petersburg, 195220, Grazhdansky prospect 14, Russia
Correspondence to: Mikhailenko I. M. , Scientific deputy director, head of lab of informative-measuring systems.
Email: |  |
Copyright © 2012 Scientific & Academic Publishing. All Rights Reserved.
Abstract
20 major tasks, which were determined by V.A. Dragavtsev[6] for future quantitative technologies of eco-genetic improvement of plants productivity and yield are investigate. Authors offer principles of mathematical modelling for each of these tasks.
Keywords:
Genotype-Environment Interaction, Models of Dynamic, Prediction, Design, Interpreting of the Nature of Ecological Reaction, Signs , Levels of Correlations, the Shifts of Dominance in Different Environments
1. Introduction
Further development of the genetic improvement of plants quantitative traits is largely constrained by inadequate theoretical level of all branches of modern genetics. List of unsolved problems in this area today is quite extensive, but common to them is the lack of a quantitative theory of eco-genetic processes, based on its mathematical models. In this case we are not interested by models in general, but only those models that address to specific breeding objectives. Despite the large number of possible solutions of problems, modern cybernetics includes only three classes of problems whose can solute different in appearance and shape the mathematical models. These include: the problems of optimal control by the state of dynamical systems, which use predictive dynamic model (PDM), the problems of the optimal design, which uses both dynamic and static model of design (MD), explicitly contain the parameters that need to be decide based on the operation of the system objectives of the study systems in which the natural experiment is replaced by computer experiment, and which use detailed models, reflection-mental "physics" of phenomena and processes (FM).G. Kekser in his famous work "Kinetic models of development and heredity" has come to the conclusion that the theories, laws, hypotheses are logically equivalent to a model. Often there are two types of models - speculative and heuristic. Recent allow formulate a more or less precise statements, leading to further experiments. In this case, they can be not mathematical models. A genetic model, such as a model of Mendel, predicts thepictures of the hereditary traits segregation. In this model, the genotype is postulated solely on the basis of genetic experiments analysis[1, p.47].A mathematical model of quantitative traits inheritance identify the key processes (essential variables) necessary for predicting of biological systems behaviour at different levels of organization - from molecular to biocenosisal. For a model of natural resources of the biosphere using, there are common variables: segregation, preying-proof, competition, parasitism, the spread of diseases, the impact ofenvironmental factors on the distribution of plants and animals. In modelling these processes are linked by commonmathematical properties of skim: retardation effects, cumulative effects, threshold values, a large number of variables, and their complex interaction.In the papers[2,3,4,5,6,7] introduced the concept of eco-genetic organization of quantitative traits, which allows us to develop a genetic theory by implementing the following guidelines which may be regarded as variants of the researcher-ray problems. Here we are at the same time we point and type of mathematical model:1. Understanding of the mechanisms of the genotype reactions on the limiting factors of environment - can be implemented by the FM;2. Understanding of the mechanisms of a quantitative trait development in ontogeny - can be implemented by the FM;3. Understanding of the mechanisms and prediction of “reactions norms” and homeostasis of productivity under different environmental conditions - can be implemented by the FM;4. Understanding of the mechanisms of the dominance “shifts” for quantitative characters in different environments - can be implemented by the FM;5. Understanding of the nature of ecologically dependent heterosis, and prediction of its appearance in ecological or competitive limiting of growth processes - can be implemented through the PDM;6. Understanding of the nature of the "shifts" and predicting changes of signs and levels of genotypic, genetic and environmental correlations - can be implemented through the PDM;7. Control of the amplitude of genetic variability of quantitative character in the population by changing external constraints - can be realized through the PDM;8. Managing by the number of genes, which determine the level and genetic variability of trait in the population by changing the limiting factors - can be realized through PDM;9. The choice of characters by which to conduct selection in specific environmental conditions - can be implemented by MD;10. Designing the ideal background characters (with zero genetic variance) to identify in real-time genotypes by phenotypes - can be realized by the MD;11. Understanding of the transgressions mechanisms and prediction of their occurrence in specific hybrid populations - may be implemented through the PDM and MD;12. Understanding of the mechanisms and predicting the effects of genotype-environment interaction (GEI) - can be implemented through the PDM;13. Understanding of the nature of pleiotropy forquantitative traits and prognosis of “breaking" pleiotropic complexes in different environmental conditions - can be realized by means of PDM;14. Creation of new principles of parental pairs selection for solving breeding tasks - may be implemented through the MP;15. Development of the theory and practice of improved seeds production - may be implemented through the MD;16. Creating a common science-based technologies of breeding for productivity with using of artificial climate systems for the study of adaptive traits of genotypes in different phases of development and at different lim-factors, - can be realized by the MD and PDM;17. Assessing one of the three basic characteristics: adaptive property of varieties, the dynamics of the limiting factors and genetic parameters of populations by the other two - could be implemented through the PDM;18. Prediction of Hayman’s graphs configurations and Heyman’s parameters rank options without diallel crosses - can be implemented through the PDM;19. Establishment of a quantitative theory of plantontogenesis on the basis of ecology- genetic control - may be implemented through the PDM;20. Genetic inventory of plant populations - can be realized by PDM.Thus, of the 20 tasks listed 12 tasks based on the use of prognostic dynamic models (PDM), 4 the problem - by using models of design (MD) and the same number of tasks based on the research of the physical model (FM). Obviously, from the viewpoint of the authors of the concept of the most urgent tasks, which use predictive dynamic models that largely corresponds to the very essence of the concept of «eco- genetic response - a quantitative character».Any of the above problems involves the construction offorecast changes of quantitative traits in response to changing of the environment’s parameters. In this large majority of quantitative traits are states of the production process. Therefore, the new model on the concept of "genotype - environment" interaction will be largely the further development of methods for modelling theproduction process, which should include all the main features and characteristics of a particular geno- and phenotype.
2. Conceptual Schema of Model
The most important step in the construction of any mathematical model of a complex system of course, applies a living organism, is the selection of significant variables in the system, describing its complete state. Despite the difficulties of selecting substantially variables in biological systems, and here it is expedient to adhere to the principle of the system-wide. It lies in the fact that these variables should be makes for the purpose based on them, directly or through auxiliary variables must be calculated or assessed optimality criteria used for the solution of specific problems. Therefore does not make sense to talk about a set of essential variables, until the final statement of the specific tasks of forecasting and management. Yet restrict the most common approach to choosing the type and structure of the model and will start from the adoption of modular structure, developed by the authors of the concept.Distinguish the main features of the simulated processes and phenomena: most of the quantitative traits are the main parameters of state (hereinafter simply states), the other less common of characters of a resultant outputs, and are combinations of states (often paired),а lot of dynamic and static characteristics of external factors: the randomness, the existence of the inverse effect on the plants themselves (competition), the intensity and duration of exposure, the magnitude and the moments of minima and maxima of the action (strikes), the presence of the phases of ontogeny and their influence on the dynamic of characteristics, availability of the spectrums of genes that control quantitative traits, the number of which depends on environmental factors, and due to which there are significant changes in the dynamics of quantitative traits.Fig. 1 shows the conceptual scheme of the model, which defines the dual effect of environmental factors, directly on the evolution of quantitative traits (signal communication) and in the spectra of genes - the parametric context by which it is possible to significantly affect the distribution of the contributions of states in the module quantitative traits, and thus the final result. In addition, there is reflected the inverse effect on the environment the plants themselves, which is especially noticeable in the soil.According to this scheme to construct a mathematical model of «genotype-environment» interaction, we must address the following main tasks: modelling the evolution of states and outputs of the modules of quantitative traits, simulation of the change of the spectra of genes in the genotype or the state of genetic-physiological systems, depending on the perturbation of the environment and their influence on the parameters of the model of the evolution of states, an imitation of changes in equations of environmental factors.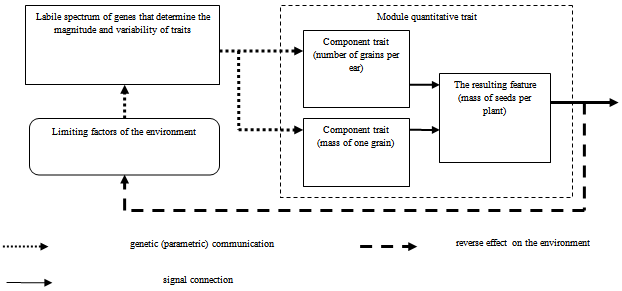 | Figure 1. Conceptual diagram of the model of interaction "genotype-environment" |
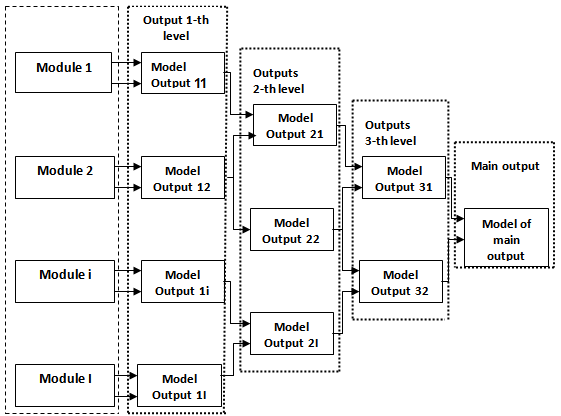 | Figure 2. The modular structure of a mathematical model |
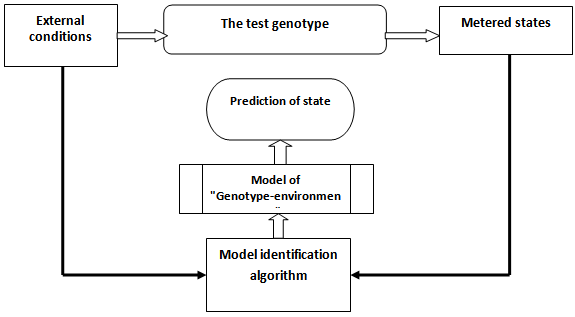 | Figure 3. Block diagram of model identification, "Genotype-environment" |
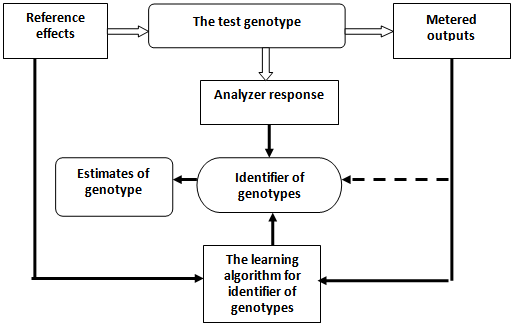 | Figure 4. Block diagram of the identification genotype by the reference effect |
It is helpful to place a large number of genes in the spectrums, which determine the evolution of quantitative traits in the modules, to simulate the sevengenetic-physiological systems: attraction - providing a period of plastic substances filling pumping out with crowbars, and leaves in the ear; micro-distribution attraction matter between grain and chaff have spiked, husk and kernel in sunflower, etc.; adaptation (resistance to stressors on the climatic and chemical parameters of the environment); polygenic resistance; susceptibility (response) to the doses of soil nutrition, tolerance to the density; genetic variability of ontogenetic phases.
3. The Modular Structure of the Model
Fig.2 shows the structure of a mathematical model that contains the basic modules and components of quantitative traits outputs, forming several layers.In accordance with the above structural scheme, we give the canonical form of model, which includes a block of core modules that define the evolution of the continuum states (the component characters) (which include biomass, the mass of commodities or items of plant, linear dimensions, etc.):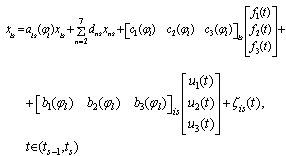 | (1) |
Counting of states (number of fruits, grains in the ear, ears, stems, internodes, and others)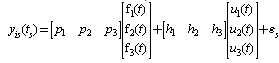 | (2) |
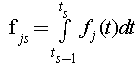 | (3) |
And, in fact, the unit outputs | (4) |
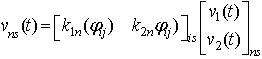 | (5) |
Model of quantitative traits in vector-matrix form is as follows | (6) |
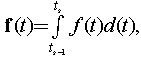 | (7) |
 | (8) |
In models (1) - (8), the following notation: i = 1, I; n = 1, N indexes modules, l-the index of the state of genetic-physiological system, denoted by φ; s-indices phenophases; a11(φij )-a22(φij), Ais (φij)-dynamic parameters of the models of the modules perturbed by the corresponding genetic and physiological systems; d11-d22, Dis-parameters of cross-linking of modules; f1, f2, f3, F-uncontrollable external factors (climate factors); u1, u2, u3, U-managed by external factors (nutrient); c11 (φij)-C23 (φij), Cis (φij) - parameters that define the system sensitivity to external uncontrollable impact and perturbed by the relevant genetic -physiological systems; b11(φij)-b22(φij), Bis (φij) - parameters that define the system sensitivity to external control action and angers the relevant genetic and physiological systems; vi-outs of the first level; vn-outs subsequent levels; k1i, k2i; kni, kn2;-parameters and matrices of increasing dimension, the unit output modules; XN-total state vector; ζ1, ζ2; Ζ-random perturbations of states (noise quantitative traits) is a Gaussian process with zero mean and covariance θ1, θ2; Θ. In the above model, there are two main sources of uncertainty (information noise): direct dynamic random perturbations of the external environment, stimulating the covariances states of any phenotype; covariances of the model parameters, including both genetic variation and inaccuracy in the parameters for individual phenotypes.Applying the above model, the operation of mathematical expectation and then selecting from it centered component, can cause the model to two dynamic blocks of sufficient statistics: the expectation of the vector of states and the covariance matrix of states.
4. State Model of Genetic-Physiological Systems
Due to the fact that the ecology-genetic approach involves instead of "genetic analysis" study of the characteristics of genetic-physiological systems through which possibly improve the species, it does not make sense to model the dynamics of a large number of genetic spectrums, and immediately assess the result of the influence these systems on the status of output modules through the parameters of basic units, as indicated in the diagram Fig. 1. However, unlike the signal to the external environment on the condition of the basic modules, which is important only the magnitude and duration of exposure, here are informative, form and time of the annex of exposure.We introduce the shape parameters of external influence (the limiting factor), which for convenience we combine the vectors Πl, where l = 1,7 - indices of genetic-physiological systems. The choice of an adequate set of parameters forms for each condition (informative factors) is precisely the subject of study genetic-physiological systems. After identifying a set of parameters is possible to construct model state of genetic-physiological systems.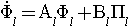 | (9) |
 | (10) |
Фl - a vector of state parameters l-th genetic-physiological system; Αl - the dynamic matrix; Βl-matrix parameters influence the form of external influence on the state of a l-th genetic-physiological systems Pl - the vector of parameters of the n-th module model of qualitative features perturbed by l-th genetic-physiological system, Dnl- matrix of connection parameters n-th module of qualitative features of the model and condition of l-th genetic-physiological system.We distinguish the possible parameters of the state basic genetic-physiological systems in order:-attractions, providing a period of plastic substances filling pumping out stems and leaves in the ear - weight of stem and spike (economic and non-economic parts of the plant) in the content of carbohydrates and protein in the commodity and non-market units;-microdistribution of attracted matter between grain and chaff for cereals, husk and kernel in sunflower, etc. - weight of grain and non-cereal part of the ear (chaff, awns, etc.);-adaptation (resistance to stressors on climate and chemical parameters of the environment) - the degree of slowing of the growth processes under the influence of environmental parameters, speed and time of restoring the normal dynamic of growth processes;-polygenic steadiness - resistance of plants to pathogens, the development of plant stability mechanisms;susceptibility (response) to the elements of soil nutrition - the parameters of the sensitivity of the productivity; tolerance to density - the parameters of sensitivity of productive indicators to the density of plants.Variability of periods of ontogenesis - the parameters covariance of between phases periods to environmental factors.
5. Imitation of External Disturbances
Of the three above-mentioned problems of modelling we need to consider the principles of imitation externalinfluences. Without solving this problem is not as rapid predicting the status of agricultural crops, and the solution design and objectives of planning on technology. Under the operational forecasts, projections are understood to proactively from one week to 3 to 4 month period. In view of the local task and the limited information capacity, we do not use models of atmospheric physics, and each of the climatic factors are considered as random vectors observed process. Results of forecasting model, can cover area bounded by the size of a farm field or a farm.However, even this simplified approach faces a serious problem of choosing an adequate mathematical model of the dynamics of individual climatic factors, which would be sufficient to solve the problem prognostic properties. So study the dynamics of the monthly fluctuations of each factor in a few years have shown that a single a priori model, even very precisely tuned to their measured value has property of regularity and posterior projections derived from them do not meet the specified standards of accuracy and reliability.This has led to resort to special adaptive algorithms for variable structure. The basis of these algorithms is the representation of changes of weather factors as complex poly scenarios a dynamic process in which each of the dominant scenarios is a random event and is given by its model with a priory unknown parameters. Then in the task of identifying the process is how to identify the number of dominant scenarios for each forecast period, the parametric-ray view of a priori probability of their occurrence, as well as to refine these probabilities and parameters in real time.In this representation of processes model of each of the three above-mentioned climatic optical factors for any given period can be represented as follows: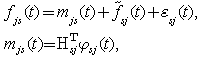 | (11) |
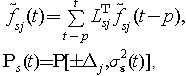 | (12) |
where - a deterministic basis for s-th scenario, the j-th climatic factor, which represents the expectation of the process - centred component of the scenario, represented by an autoregressive process with a memory depth p, ε(t)- error simulation (noise model) is accepted as a Gaussian random process with zero mean and residual variance σ2ε; Hsj, Lsj -the vectors of model parameters of deterministic basis and centred part of the scenario - φsj(t)- the basic functions of time a determinate basis scenario; Ps(t) - a priori probability of occurrence of scenarios was determined by the sphere of permissible errors of prediction Preliminary study of the processes of change of temperature j = 1 to separate months of growing season (May-September) revealed the following possible scenarios:linear trend of s = 1
quadratic spline s = 2
harmonic number s = 3
For estimating the parameters of the matrix forms of external influence Pl used in the block modelling of the genetic and physiological systems, Monte Carlo simulated scenario processes together with the probabilities of change scenarios that can first be unified (out scenario) processes of change of external disturbances,
and from them the estimation of parameters forms (elements of the vectors Πl).
6. Issues Model of Identification of Genotypes by Phenotypes
Of all the 20 above-mentioned problems of greatest interest to the models of researchers, geneticists, is the problem of identifying of genotypes by observations of phenotypes. This problem is directly joined to a common task of identification and mathematical model. Sense of such connections is that without enough impossible reliably identify the model reliable identification of the genotype as identified by the model.What is the difference between these two acts of identification? To clarify them in Fig. 3,4,5 are shown block diagrams of these procedures.Identification of the mathematical model is to estimate all parameters and correcting model structure, if you can not get reliable estimates and regularity in terms of forecasting accuracy for current structure. For parameter estimation using arbitrary changes in external factors and reaction to them investigated genotype, which is reflected in the dynamics of the state vector. Effectiveness evaluation verified for accuracy of forecasting.In contrast, model identification, the task of identifying the genotype is essentially a pattern recognition problem and its solution is possible to use multiple methods andtechniques. Depending on the conditions of their implementation, these methods can be divided into two large groups. In the first group of methods that can be called deterministic, use standard impact for the studied plants. The composition of these Impact contain all forms of options included in the vectors Πl models (9), (10) genetic-physiological systems. Naturally, the set of reference actions we located causeway and a set of standard responses in the modules of quantitative traits, which allow the identification of genotypes. It is clear that such methods of identification require the specialized phytothrone protected from the effects of uncontrolled environmental factors, as well as the database is a set of standard responses of different genotypes.Closer to the real conditions of breeding are stochastic methods of recognition, where all the external factors are uncontrollable and may vary randomly-formed way. Here is an important condition of observables, whereby constructed a posteriori laws of probability distributions of quantitative traits. Availability of such information allows us to apply Bayesian classification methods, or method of maximum likelihood[9,10]. But the implementation methods of the second group requires a large amount of prior information about the state of genotypes and phenotypes and imposes strict requirements to store all the information of the breeding process, which means a large number of variants and replications. All this information must be combined into a knowledge bases of breeders, which is not currently the case.
7. Conclusions
The basic principles of modelling the interaction"genotype-environment", included in the joint use of a dynamic model of the production process, and seven models of genetic-physiological systems: attraction providing a period of plastic substances filling pumping out with crowbars, and leaves in the ear; micro-distribution attraction matter between grain and chaff have spiked, husk and kernel in sunflower, etc.; adaptation (resistance to stressors on the climatic and chemical parameters of the environment); polygenic resistance; susceptibility (response) to the doses of soil nutrition, tolerance to the density; genetic variability of ontogenetic phases. In this model the production process is complemented by multi-unit output interface modules for each phase of ontogenesis, each of which is connected to the inputs to the outputs of the model of the production process and the genetic-physiological systems, which are modelling by a change of the spectrums of a huge number of genes and their influence on the quantitative characters, depending on the limiting factors of environment. In accordance with the principles of the proposed methods for estimating substantiated parameters of models of productivity and the state of genetic-physiological systems and identification of genotypes by their phenotypes.
References
[1] | Kekser G. Kinetic models of development and heredity. Symp. Modeling in biology. MM: PCL, 1963. p. 42-64. |
[2] | Glazko VI, Glazko GV Russian-English-UkrainianDictionary of applied genetics, DNA technology and bioinformatics. Kyiv: Nora-Print, 2000. pp. 215-216. |
[3] | Dragavtsev VA Tsilke RA, Reuter B., et al Genetics of productivity characters of spring wheat in western Siberia. Novosibirsk: SOAN 1984. p.121. |
[4] | Dragavtsev VA Litun PP, Skele, NM, Nechiporenko NN Model of eco-genetic control of quantitative traits of plants / Dokl. Academy Sci. USSR, 1984. T. 274. № 3. pp. 720-723. |
[5] | Dragavtsev V. A. Integration of Biodiersity and Genom Technology for Crop Improvement // National Institute of Agrobiological Resources. Japan. Tsucuba. 2000. pp. 93-95. |
[6] | Dragavtsev V.A. Algorithms of eco-genetic survey of thegenefund and methods of creating the varieties of crop plants for yield, resistance and quality. St. Petersburg. VIR. 2002. p.41. |
[7] | Chesnokov YV, Pochepnya NV, A. Berner, U. Lovasser, Goncharova EA, Dragavtsev VA Eco-genetic organization of quantitative traits of plants and mapping of loci determining agronomically important characters in wheat / / Dokl. of the Academy of Sciences (RAS). 2008. T. 418. № 5. pp. 1-4. |
[8] | Glazko VI, Glazko GV Explanatory dictionary of terms for general and molecular biology, general and applied genetics, plant breeding, DNA technology and bioinformatics. MM: ECC "Akademkniga, 2008. MM: Medkniga, 2008. T. 2. p.308. |
[9] | Sage EP, Mels G. Identification of control systems. Moscow: Nauka, 1974. 280 pp. |
[10] | Modern methods of identification systems, Ed. P. Eykhoffa. Moscow: Mir, 1983. p. 384 |