Rasaki Olawale Olanrewaju1, Anthony Gichuhi Waititu2, Nafiu Lukman Abiodun3
1Pan African University, Institute for Basic Sciences, Technology and Innovation (PAUSTI), Kenya
2Department of Mathematical Sciences, Jomo Kenyatta University of Agriculture and Technology, Kenya
3Department of Mathematics and Statistics, Kabale University, Kabale, Uganda
Correspondence to: Rasaki Olawale Olanrewaju, Pan African University, Institute for Basic Sciences, Technology and Innovation (PAUSTI), Kenya.
Email: |  |
Copyright © 2021 The Author(s). Published by Scientific & Academic Publishing.
This work is licensed under the Creative Commons Attribution International License (CC BY).
http://creativecommons.org/licenses/by/4.0/

Abstract
This paper describes regime-switching, full range of shape changing distributions (multimodalities), and cycles traits that were characterized by time-varying series via Weibull distributional noise for time series with fluctuations and long-memory. We developed and established a Weibull Mixture Autoregressive model of k-regimes via
with Expectation-Maximization (EM) algorithm adopted as parameter estimation technique. The ergodic process for the
model was ascertained via the maximized derivation of the absolute value of the subtraction of its likelihood from its expected likelihood.
Keywords:
Expectation-Maximization, k-regimes, Mixture Autoregressive model, Regime-switching, Weibull Distribution
Cite this paper: Rasaki Olawale Olanrewaju, Anthony Gichuhi Waititu, Nafiu Lukman Abiodun, On the Estimation of k-Regimes Switching of Mixture Autoregressive Model via Weibull Distributional Random Noise, International Journal of Probability and Statistics , Vol. 10 No. 1, 2021, pp. 1-8. doi: 10.5923/j.ijps.20211001.01.
1. Introduction
Most economic and financial time series possesses traits such as regime shifting, outburst, outliers and change point like behavior that have be addressed by some non-linear time series models, such as Autoregressive Moving Average (ARMA), Self-Exciting Threshold Auto-Regressive (SETAR) and Autoregressive Conditional Heteroscedasticity (GARCH), and its variants. Problems of overall-stationarity, Conditional Heteroscedasticity, excessive skewness and kurtosis, non-linearity violation, full range of shape changing predictive distributions (multimodality) and ability to handle cycles are yet to be addressed fully in modeling fluctuating time series data [9], [2]. Financial, climate and economic time series are often driven by unimodal innovation series. This often implies a unimodal marginal and/or a unimodal conditional distribution for the time series itself. In reality, many financial, climate and economic time series exhibits multimodality either in the marginal or the conditional distribution. However, this article will be adopting a more robust marginal distributional of Weibull distributional form in the build-up of specifying the Mixture Autoregressive model denoted by
. The Weibull marginal distribution for autoregressive regimes-switching model will be adopted as a substitute for widely used Gaussian marginal distribution because of its ability to capture and model contaminated series characterized with traits such as skewness, kurtosis, k-regimes, outburst, outliers and change point like behavior [6], [5]. The
with Gaussian distributional form of the random noise lacks the ability to fully capture and represent distortion caused by contaminated series with above said traits. Therefore, this paper develops, ascertains and estimates the Mixture Autoregressive of k-regimes with Weibull marginal distributional form, denoted by
.
2. Literature Review
Regime-switching generalization via Mixture Autoregressive (MAR) model was propounded by [10] to relax all these mentioned stylized properties of outburst, outliers and change-point like behavior characterized linear and non-linear time series model but yet to be addressed by ARMA, ARIMAX, SETAR and GARCH. Shortly afterwards, [1], In his work “Prediction with Mixture Autoregressive models” deduced Mixture Autoregressive (MAR) models have the attractive property that the shape of the conditional distribution of a forecast depends on the recent history of the process. It was also ascertained by [1] that if the original MAR model is a mixture of normal distributions, then, the multi-step distributions are also mixtures of normal distributions. A pth ordered model and explicitly expressed dimensional stationary distribution giving a mixture of Normal distribution with constant mixing weights via a general formulation for a univariate nonlinear autoregressive model was presented by [6]. They presented an illustration via an empirical example of interest rates of ten (10) years. In advancement to [6], [5] proposed a novel nonlinear Vector Autoregressive (VAR) otherwise called Gaussian Mixture Vector autoregressive (GMVAR) model. They developed and explained its asymptotic theory of maximum likelihood whose usefulness is vital when dealing with bivariate time series settings. [3] adopted Student’s t- distribution as the error term for mixture autoregressive model via. Their intention was catering for meet inadequate ergodicity and stationary properties that had been a problem by Gaussian error term. Furthermore, [4] improved and examined Finite Mixture (FM) model accompanied with flexibility of classification of two parts of distributions based on scale mixtures of normal (TP-SMN) constitutive members. They claimed that the family make room for robust estimation of FM models development with the ability to capture and absolve asymmetric and symmetric, and heavy and fat-tailed distributions. They further maintained that TP-SMN provides an alternative family member to scale mingling of skewed normal (SMSN) family and vital traits of well-hierarchical expression of the family to obtain ML estimates of the model coefficients via an EM parameter estimation technique.From the available and reviewed literature, it was glaring that the
has not been subjected to any of the candidates of the Extreme Valued Distributions (EVDs). In support of the need to subject the MAR model to any of the EVDs in order to capture fluctuation caused by extreme values, this article will be adopting the Weibull as the marginal distribution (random noise) for the MAR model as well as the extreme valued distributional form. The mean and variance of the multimodal conditional distribution for the
will be ascertained. The Expectation-Maximization (EM) estimation technique will be adopted via E-step and M-step leading to a system of equation and Newton-Raphson iterative technique for estimating AR coefficients, and standard errors attached to each regime. As well as the Ergodic Process for the model.
3. Detailed Description of the Gamma Mixture Autoregressive Model
Normal mixture transitional distribution (GMTD) models for conditional Normal distribution was firstly introduced by [7], it was splinted-out and viewed as a finite (countable) mixture distribution by [11] as;  | (1) |
where
is whole mixture regime-switching probability density function of identically distributed function and
are the probability density functions which may depend on certain parameters; mixing weight or weighted probability
for
.A k-component of Mixture Autoregressive (MAR) model was defined by [9], [11] and [8] to be  | (2) |
Extending the k-component of MAR model in (2) to Weibull Mixture Auto-Regressive
gives | (3) |
where;
Otherwise,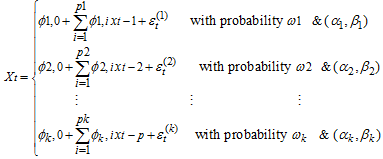 | (4) |
such that the model is denoted by
, where
is the conditional cumulative distribution function of
given the immediate past information, evaluated at
,
,
, for
,
. For mixing weights (weighted probabilities)
,
, for
.
is the Cumulative Distribution Function of the standard Weibull, where
, with mean
and variance
.
3.1. The Conditional mean and Variance for WMAR Model
The conditional mean and variance for WMA model of
given the immediate past information is as follow: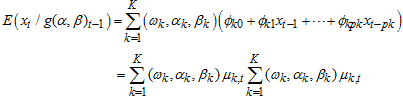 | (5) |
since,
Since the mean of Weibull is
, | (6) |
which depends on immediate past values of the time series and
is the PDF of Weibull.
So,  | (7) |
But,
for
(immediate past values of the time series that depends on
the present values). | (8) |
Therefore, 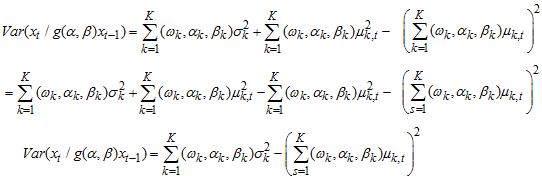 | (9) |
The expression
is a positive (non-negative) and would be tantamount to zero if and only if the each regime mean is equal to each other, that is,
. The expression satisfies the non-negativity property of variance, so the variance for WMAR Model is non-negative.Since the mean and variance of Weibull PDF are;
respectivelyAlternatively,
can be rewritten as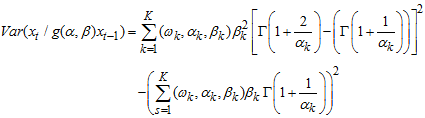 | (10) |
3.2. Parameter Estimation for WMAR via EM Algorithm
Adopting the Expectation-Maximization (EM) algorithm Let
;
;
;
;
for
.Furthermore, let “S” be the unobserved random variable where
is a
dimensional vector such that 
whose component is
For
, that is,
Let
be the parameter space.Given
, the Weibull distribution of the complete data
is given by 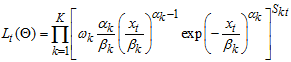 | (11) |
Allowing
to be the conditional log-likelihood function at time
. The log-likelihood is then
. Let
be the joint conditional log-likelihood function for large sample size (n) that makes the effect of
trifling. So, the maximizing the conditional log-likelihood function
gives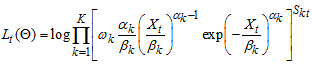 | (12) |
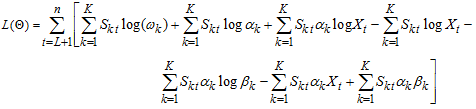 | (13) |
Where
First-order derivatives of
with respect to each of the parameter gives, | (14) |
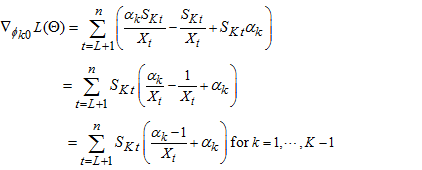 | (15) |
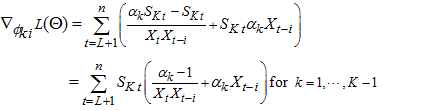 | (16) |
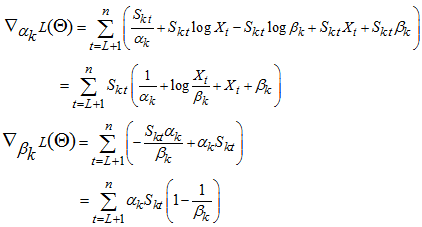 | (17) |
For
Second-order derivatives of
with respect to each of the parameter gives; Let the function
be a function of a random variable at time
and counter 
So,  | (18) |
 | (19) |
 | (20) |
 | (21) |
 | (22) |
 | (23) |
 | (24) |
 | (25) |
 | (26) |
 | (27) |
 | (28) |
Employing the EM algorithm procedure for estimating
, the parameter space via the
in equation (13).The first step from the acronym EM algorithm, that is the E-step, suppose the parameter space
is available, then the missing values for the unobserved data
is then replaced by impose means imposed on each parameter on the observed data X. Allowing
to be the imposed mean of
, then
is individual transition probability of the imposed mean all over the totality of the transition probability imposed expectation (that is, Bayes’ theorem)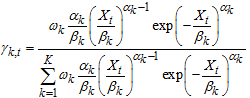 | (29) |
For
The second case (M-step) where the missing data S is assumed to be guessed and to be replaced by their imposed means on the parameters. The estimates of the parameters of the parameter space,
. The estimates of the parameters
can be obtained via
by subtracting
from
to give,  | (30) |
Such that
for that runs from
that could also be estimated via a system of equations (that is, the estimates of the parameters are then obtained by iterating these two steps until convergence) or alternatively via Newton-Raphson iterative procedure of all the parameter space at once.  | (31) |
3.3. Ergodic Process for WMAR Model
Strictly stationary and ergodic process WMAR model would be ascertained via | (32) |
Where,
are likelihoods and expectations of likelihoods respectively.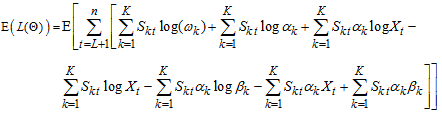 | (33) |
From Jensen’s inequality,
for every positively integrable X.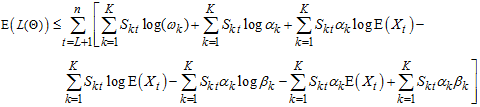 | (34) |
But, 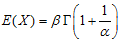
 | (35) |
So,  | (36) |
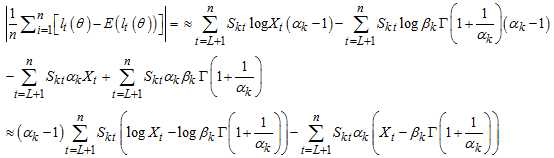 | (37) |
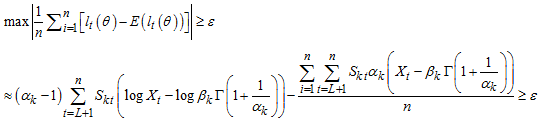 | (38) |
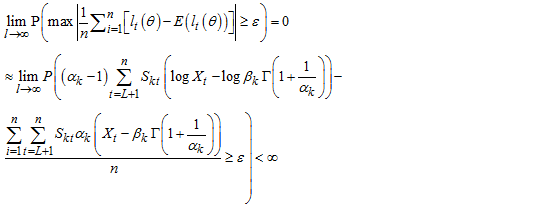 | (39) |
4. Conclusions
The proposed and formulated model of
being one of the candidates models for extreme valued mixture autoregressive was designed in order to capture, and correct stylized properties; entire outrange of switching-patterns with Weibull marginal distribution(multimodalities), change points like behavior, regime-switching (capable of handling recurring periodical sequence), and time-varying volatilities (conditional means-variances). The model was also formulated to absolve heavy-tailed, long-memory and non-Gaussian MAR model characterized by positive and strictly count (discrete) valued random noises (error terms) series.
ACKNOWLEDGEMENTS
We wish to thank the reviewers.
References
[1] | G.N. Boshankov, “Prediction with Mixture Autoregressive Models” Research Report No.6, 2006, Probability and Statistics Group School of Mathematics. The University of Manchester, 2006. |
[2] | G. Behrooz, S.M.N. Abu Hassan, and S. Tamat, “Application of the Threshold Model for Modelling and Forecasting of Ex change Rate in Selected ASEAN Countries” Sains Malaysiana, Vol.43(10), pp. 1609-1622, 2014. |
[3] | M. Meitz, D. Preve, and P. Saikkonen, “A mixture autoregres sive model based on Student’s t- distribution”, 2018. |
[4] | M. Maleki, and A. R. Nematollahi, “Autoregressive Models with Mixture of Scale Mixtures of Gaussian Innovations” Iran Journal of Science and Technology: Transactions A Science. Vol. 41(4), pp. 1099–1107, 2017. https://doi.org/10.1007/s40995-017-0237-6. |
[5] | L. Kalliovirta, M. Meitz, and P. Saikkonen,” A Guassian Mix ture Vector Autoregressive”. Journal of econometrics, Vol. 192, pp. 485-498, 2016. |
[6] | L. Kalliovirta, M. Meitz, and P. Saikkonen, “A Guassian Mix ture autoregresive model for univariate series” Journal of Time Series Analysis, Vol. 36, pp. 247-266, 2012. |
[7] | M. Li and M. Raftery, “Modeling flat stretches, burst and outli ers in time series using Mixture Transition Distribution Mod els” J.Am Statist. Ass., Vol. 91, pp. 1504-1514, 1966. |
[8] | N. Nguyen, “Hidden Markov Model for Stock Trading” Inter national Journal Financial Studies, Vol 6 (36), 2018. Doi:10.3390/ijfs6020036. |
[9] | C. S. Wong, and W. K. Li, “On a Mixture Autoregressive Mod el” Journal of Royal Statistics Society. B 62, pp. 95–115, 2000. |
[10] | C. S. Wong, W. S. Chan, and P. L. Kam,” A Student t-mixture autoregressive Model with applications to heavy-tailed financial data” Singapore Economic Review Conference, pp. 1–10, 2009. |
[11] | C. S. Wong, Statistical inference for some nonlinear time series models, Ph.D thesis, University of Hong Kong, Hong Kong, 1998. |