L. Tetteh-Ahinakwa, F. T. Oduro
Department of Mathematics, Kwame Nkrumah University of Science and Technology, Kumasi, Ghana
Correspondence to: L. Tetteh-Ahinakwa, Department of Mathematics, Kwame Nkrumah University of Science and Technology, Kumasi, Ghana.
Email: |  |
Copyright © 2018 The Author(s). Published by Scientific & Academic Publishing.
This work is licensed under the Creative Commons Attribution International License (CC BY).
http://creativecommons.org/licenses/by/4.0/

Abstract
This paper assesses the factors that contribute to infant mortality, with malaria as the central focus, at the Komfao Anokye Teaching Hospital (KATH), Kumasi - Ghana from 2010 to March 2015 using the logistic regression model. Data was taken from the registers at the Research and Department Unity of KATH. The logistic regression model was used to determine the impact of the independent variables; Duration of stay in hospital, Age of Infant, Infant Weight Level and Diagnosis presented simultaneously to predict membership of the Outcome of Infant (dead or alive). Findings show that duration of stay in hospital contributes significantly to infant mortality at the KATH. Malaria did not contribute significantly to the outcome.
Keywords:
Infant mortality, Logistic regression, Diagnosis, Outcome
Cite this paper: L. Tetteh-Ahinakwa, F. T. Oduro, Logistic Regression Model on Infant Mortality at the Komfo Anokye Teaching Hospital, Kumasi - Ghana, International Journal of Probability and Statistics , Vol. 7 No. 3, 2018, pp. 63-66. doi: 10.5923/j.ijps.20180703.01.
1. Introduction
The intent of every hospital facility in the world is to cure disease in order to save lives. Anyone attending or admitted at a hospital is hopeful of being discharged in total health. Thus, the loss of an infant at the hospital is expected to be very minimal if not entirely prevented. However, this is not always the case. A lot of infants lose their lives in hospitals for various reasons: known and unknown. Infant mortality is defined as the death of a child before reaching one year of age per 1,000 live births in a given year [1]. There are wide- range reasons why an infant may lose his life in the hospital with some of them largely preventable. In a research to identify the factors which are associated with infant deaths in a typical rural community in South-western Nigeria with mid-year population of 3,308, infant mortality was found to be 68.2 per 1,000 live births. First birth order and old mothers (34 years) at time of death were associated with significantly higher risk for mortality in the village (p=0.004). Information on births and deaths were collected for five consecutive years (1993-1997) by trained Village Health Workers (VHW) and Traditional Birth Attendants (TBA). The commonest cause of death was due to complications of low birth weight due to infant mortality [2]. A research with an objective to determine the association of different feeding patterns for infants (exclusive breastfeeding, predominant breastfeeding, partial breastfeeding and no breastfeeding) with mortality and hospital admissions during the first half of infancy [3], found out that non-breastfed infants had a higher risk of dying when compared with those who had been predominantly breastfed (Hazard Ratio (HR) = 10.5; 95% Confidence Interval (CI) = 5.0–22.0; P < 0.001) as did partially breastfed infants (HR = 2.46; 95% CI = 1.44–4.18; P = 0.001). Their research was based on a secondary analysis of data from a multicentre randomized controlled trial on immunization-linked vitamin A supplementation. In Mozambique, clinical malaria incidence in infants aged 1– < 6 months was substantial (320/1,000 child-years at risk in 2003–2004 and 146/1,000 child-years at risk in 2004–2005). Although malaria in infants aged under six months represented less than 20% of the total outpatient visits, infants with malaria were admitted in a significantly higher proportion than children aged 1–4 years Also, malaria infections were common in Ghanaian infants less than 6 months of age (13.6% in new-borns; 1.5–9.7% in those aged from 2–26 weeks), although clinical malaria symptoms were rare or uncommon [4].Ethnic specific socio-cultural practices may be salient to infant mortality differentials in Ghana [5]. The bivariate results using data on 3298 recent births from the 1998 Ghana Demographic and Health Survey indicated significant ethnic differences in the risk of infant data. Thus, the observed ethnic differences in infant mortality mainly reflect socio-economic disparities among groups rather than intrinsic cultural norms. A spatial analysis was carried out to identify factors related to geographic differences in infant mortality risk in Mali by linking data from two spatially structured databases: the Demographic and Health Surveys of 1995–1996 and the Mapping Malaria Risk in Africa database for Mali. The analysis was carried out by fitting a Bayesian hierarchical geo-statistical logistic model to infant mortality risk, by Markov Chain Monte Carlo simulation. It confirmed that mother’s education, birth order and interval, infant’s sex, residence, and mother’s age at infant’s birth had a strong impact on infant mortality risk in Mali. [6].A pooled analysis of studies assessed the effect of not breastfeeding on the risk of death due to infectious diseases [7]. Results showed that protection provided by breastmilk declined steadily with age during infancy (pooled odds ratios: 5.8 (95% CI 3.4-9.8) for infants aged <2 months, 4.1 (2.7-6.4) for 2- to 3-month-olds, 2.6 (1.6-3.9) for 4- to 5-month-olds, 1.8 (1.2-2.8) for 6- to 8-month-olds, and 1.4 (0.8-2.6) for 9-11-month-olds). In the first 6 months of life, protection against diarrhoea was substantially greater (odds ratio 6.1 (4.1-9.0)) than against deaths due to acute respiratory infections (2.4 (1.6-3.5)). However, for infants aged 6-11 months, similar levels of protection were observed (1.9 (1.2-3.1) and 2.5 (1.4-4.6), respectively). A variety of data sources and methods were used to assess levels of infant mortality and their trend over time in one Central Asian republic, Kyrgyzstan, between 1980 and 2010 [8]. An abrupt halt to an already established decline in infant mortality was observed to occur during the decade following the break-up of the Soviet Union, contradicting the official statistics based on vital registration. Infants of Central Asian ethnicity and those born in rural areas were also considerably more at risk of mortality than suggested by the official sources. In South Africa, a research conducted indicated that an increase in the number of post-neonatal infant deaths over time likely to be associated with HIV/AIDS at all age under 1 year is high. The aim was to identify any trends over recent time and to examine these trends for HIV-associated and non-HIV associated causes of mortality. There was a peak in HIV-related deaths, centred at 2-3 months of age rising monotonically over time. This was an indicator of paediatric AIDS in South African population [9]. A research on the impact of three key pollutants: carbon monoxide (CO), particulate matter less than 10 microns in diameter (PM10), and ozone (O3) on infant mortality rates using data from California for the 1990s showed that exposure to high level of ambient carbon monoxide does increase the infant mortality rate. The data come from a period in which CO levels in California were on average about two-thirds below the national ambient air quality standards established by the Environmental Protection Agency [10]. Overall, the estimated impact of the reduction in CO during this period—about 1,000 fewer infant fatalities—was a cut in the infant mortality rate of about 5 percent.
2. Methodology
This study uses the Logistic regression model to assess the factors that contribute to infant mortality at the Komfo Anokye Teaching Hospital. Data was obtained from the Research and Department Unit of the hospital. The SPSS version 17 is used for this analysis.Logistic regression is a linear model for the logit transformation of a binomial parameter [11]. Logistic regression models are Generalized Linear Models (GLMs) with binomial random component and logit link. The logit is the natural parameter of the binomial distribution. Thus, the logit link is its canonical link function. It is the most important model for categorical response data and used increasingly in a wide variety of applications. Early uses were in biomedical studies but the past 20 years have also seen much use in social science research and marketing. It has also become a popular tool in business application.For a binary response variable Y and an explanatory variable X, let
The logistic regression model is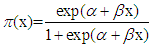 | (1) |
Equivalently, the log odds, called the logit, has a linear relationship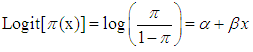 | (2) |
Like ordinary regression, logistic regression extends to models with multiple explanatory variables. For instance, the model for
at values
of p predictors is | (3) |
The alternative formula directly specifying
is | (4) |
3. Results
Infants admitted during the study period were a total of 587. There were 378 infant deaths. Figure 1 shows a pie chart of the distribution of infant mortality for 2010-2015 (March). The highest infant mortality of 91 deaths (24.07%) was recorded in 2013 and the lowest of 25 (6.61%) was recorded in 2012.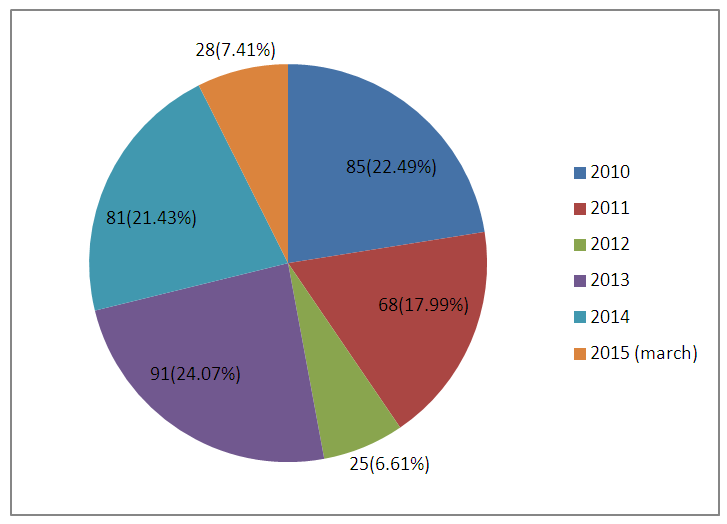 | Figure 1. Pie chart showing the distribution of infant mortality at KATH for 2010 – 2015 (March) |
3.1. Model containing Factors that Affect Infant Outcome at KATH
SPSS statistical package version 17 is used in modelling the factors that contribute to infant mortality at the Komfo Anokye Teaching Hospital. Out of the 587 cases, only 466 (79.4%) cases were included in the analysis. 121 cases (20.6%) were missing.A direct binary logistic regression is performed to assess the impact of age, duration of stay in hospital, diagnosis and weight on the likelihood of infant on admission at Komfo Anokye Teaching Hospital dying. The response variable is the outcome of admission. The outcome and diagnosis are categorical variables. Their categories are as follows;
The model containing all the predictor variables (PVs) is statistically significant with chi-square value of 15.463 with p-value of 0.004 for Omnibus Test of Model Coefficients and chi-square value of 7.537 with p-value of 0.480 for Hosmer and Lemeshow Test. The Cox & Small R2 of 0.033 and the Nagelkerke R2 of 0.045 indicate that between 3.3% and 4.5% of the variation in the response variable is explained by the predictor variables (PVs).The binary logistic regression model is given as:
Where,a = diagnosisb = weightc = durationd = age PV are predictorsThe model predicts the likelihood of an infant on admission at the KATH of dying of malaria.Table 1a. Analysis of parameter estimates for factors that affect infant mortality 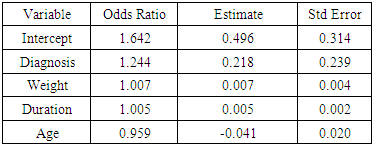 |
| |
|
Table 1b. Analysis of parameter estimates for factors that affect infant mortality 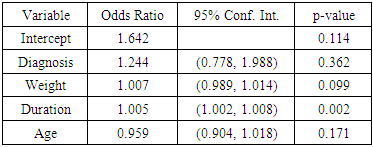 |
| |
|
From tables 1a and 1b, the only significant predictor variable is duration of stay. Thus, the binary logistic regression model for significant predictors then becomes:
Hence,
The odds ratio of 1.005 indicates that the duration of stay is 1.005 more likely to contribute to infants on admission dying than surviving. The positive coefficient of 0.005 indicates that the higher the duration of stay the higher the likelihood of infants on admission dying.
4. Conclusions
The aim of the study was to assess the factors that contribute to infant mortality with malaria as the central focus, at the Komfao Anokye Teaching Hospital (KATH).From the analysis, malaria does not have any significant effect on the outcome of infant on admission at the Komfo Anokye Teaching Hospital. The only variable affecting the outcome is duration of stay at the hospital. Duration of stay results in a 0.5% greater likelihood of infants on admission dying than living. The variable accounted between 3.3% and 4.5% of the variations in the outcome.
ACKNOWLEDGEMENTS
We wish to express our sincere gratitude to everyone that contributed in making this research a success.
References
[1] | The World Bank IBRD – www.data.worldbank.org/indicator/SP.DYN.IMRT.IN. |
[2] | Lawoyin, T. O. (2001). Risk factors for infant mortality in a rural community in Nigeria. doi: 10.1177/146642400112100213 Pespectives in Public Health June vol. 121 no. 2 114-118. |
[3] | Bahl, R., Frost, C., Kirkwood, B. R., Edmond, K., Martines, J., Bhandari, N., Arthur, P. (2005). Infant feeding pattern and risks of death and hospitalization in the first half of infancy: multi centre cohort study ISSN 0042-9686 Bull World Health Organ vol.83 n.6 Genebra Jun. http://dx.doi.org/10.1590/S0042-96862005000600009. |
[4] | D’Alessandro, U., Ubben, D., Hammed, K., Cessay, S. J., Okebe, J., Taal, M., Lama, E. K., Moussa, K., Koivogui, L., Nahum, A., Bojang, K., Sonko, A. A. J., Lalya, H. F., Brabin, B. (2012), “Malaria in infants aged less than six months- is it an are of unmet medical need?” Malaria Journal 2012, 11:400 doi:10.1186/1475-2875-11-400. |
[5] | Gyimah, Stephen Obeng (2002), "Ethnicity and Infant Mortality in Sub-Saharan Africa: The Case of Ghana," PSC Discussion Papers Series: V ol. 16: Iss. 10, Article1Sika-Bright, Solomon (2009) Socio-Cultural factors influencing current trends of infant feeding practices in Cape Coast Metropolis. |
[6] | Gemperli, A., Vonatsou, P., Kleinschmid, I., Bagayako, M., Lengeler, C., Smith, T. (2002). Spatial Patterns of Infant Mortality in Mali: The Effect of Malaria Endemicity. Vol. 159, No. 1 DOI: 10.1093/aje/kwh001 (Received for publication August 30, 2002; accepted for publication May 23, 2003). |
[7] | Victoria, G. (2000). Effect of breastfeeding on infant and child mortality due to infectious diseases in less developed countries: a pooled analysis. Lancet (British edition) 2000 Vol. 355 No. 9202 pp. 451-455 ISSN 0140-6736 DOI 10.1016/S0140-6736(00)82011-5 Rec. No: 20002013560. |
[8] | Guillot, M., Lim, S.J., Torgashavel, L., Denisenko, M. (2013). Infant mortality in Kyrgyzstan before and after the break-up of the Soviet Union. Population Studies; 67(3):335-52. |
[9] | Bourme, D. E., Thompson, M. L., Brody, L. L., Cotton, M., Draper, B., Laubscher, R., Abdullah, M. F., Myers, J. E (2009). Emergence of a peak in early infant mortality due to HIV/AIDS in South Africa Volume 23–Issue1 p 101-106 doi: 10.1097/QAD.0b013e32831c54bd. |
[10] | Currie, J., Neidell, M. (2005). Air Pollution and Infant Health: What Can We Learn from California's Recent Experience? The Quarterly Journal of Economics (2005)120(3): 1003-1030. doi: 10.1093/qje/120.3.1003. |
[11] | Agresti, A. (2007). An Introduction to Categorical Data Analysis. Second Edition, John Wiley and Sons; Hoboken, New Jersey. |