F. B. Adebola1, N. A. Adegoke1, Ridwan A. Sanusi2
1Department of Statistics, Federal University of Technology Akure, Nigeria
2Department of Mathematics and Statistics, King Fahd University of Petroleum and Minerals, Saudi Arabia
Correspondence to: N. A. Adegoke, Department of Statistics, Federal University of Technology Akure, Nigeria.
Email: |  |
Copyright © 2015 Scientific & Academic Publishing. All Rights Reserved.
Abstract
This paper examines a class of regression estimator with cum-dual ratio estimator as intercept for estimating the mean of the study variable Y using auxiliary variable X. We obtained the bias and the mean square error of the proposed estimator, also, the asymptotically optimum estimator (AOE) was obtained along with its mean square error. Theoretical and numerical validation of the proposed estimator were done to show it’s superiority over the usual simple random sampling estimator and ratio estimator, product estimator, cum-dual ratio and product estimator. It was found that the estimator while performed better than other competing estimators, performed in almost the same way as the usual regression estimator when compared with the usual simple random estimator for estimating the average sleeping hours of undergraduate students of the department of statistics, Federal University of Technology Akure, Nigeria.
Keywords:
Difference estimator, Auxiliary variable, Cum-Dual ratio estimator, Bias, Mean square error, Efficiency
Cite this paper: F. B. Adebola, N. A. Adegoke, Ridwan A. Sanusi, A Class of Regression Estimator with Cum-Dual Ratio Estimator as Intercept, International Journal of Probability and Statistics , Vol. 4 No. 2, 2015, pp. 42-50. doi: 10.5923/j.ijps.20150402.02.
1. Introduction
Ratio estimation has gained relevance in estimation theory because of its improved precision in estimating the population parameters. It has been widely applied in agriculture to estimate the mean yield of crops in a certain area and in forestry, to estimate with high precision, the mean number of trees or crops in a forest or plantation. Other areas of relevance include economics and Population studies to estimate the ratio of income to family size. Utilizing Information from high resolution satellite data, [1] examined the possibilities of different forms of auxiliary information derived from remote sensing data in two-phase sampling design and suggested an appropriate estimator that would be of broad interest and applications by proposing a new class of regression-cum estimators in two-phase sampling. He found it to be more efficient than the usual regression and ratio estimators. A class of product-cum-dual to product estimators was proposed by [2] for estimating finite population mean of the study variate. The use of auxiliary information at the estimation stage to increase the efficiency of the study variable was proposed by [3]. He used supplementary information on an auxiliary variable X positively correlated with Y to develop the ratio estimator to estimate the population mean or total of the study variable Y. The ratio-estimator is always more efficient than the normal SRS when the relationship between the study variable Y and the auxiliary variable X is linear through the origin, and Y is proportional to X [4]. Product estimator was proposed by [5]. [6] suggested the use of ratio estimator
when
and unbiased estimator
when
where
and
are coefficient of variation of y, coefficient of variation of x and correlation between y and x respectively. A lots of work have been done using auxiliary information. A ratio-cum-dual to ratio estimator was proposed for finite population mean. It was shown that the proposed estimator is more efficient than the simple mean estimator, usual ratio estimator and dual to ratio estimator under certain given conditions [7]. [8] proposed a modified ratio-cum-product estimator of finite population mean of the study variate Y using known correlation coefficient between two auxiliary characters X1 and X2, while [9] proposed a ratio-cum-product estimator of finite population mean using information on coefficient of variation and coefficient of kurtosis of auxiliary variate and showed that the proposed estimator is more efficient than the sample mean estimator, usual ratio and product estimators and estimators proposed by [10] under certain given conditions. Moreover, two exponential ratio estimators of population mean in simple random sampling without replacement were shown to be more efficient than the regression estimator and some existing estimators under review based on their biases, mean squared errors and also by using analytical and numerical results (at optimal conditions) for comparison [11]. Also, [12] suggested a ratio-cum product estimator of a finite population mean using information on the coefficient of variation and the coefficient of kurtosis of auxiliary variate in stratified random sampling.Suppose that simple random sampling without replacement (SRSWOR) of n units is drawn from a population of N units to estimate the population mean
of the study variable Y. All the sample units are observed for the variables Y and X. Let
where
denotes the set of the observation for the study variable Y and X. Let the sample means
be unbiased of the population means of the auxiliary variable
and study variable
based on the n observations.The usual ratio estimator of
is given as
and the usual regression estimator is given as
where
and
is the estimate slope of regression coefficient of Y and X. [13] obtained dual to ratio-cum estimator given as
, where
is the un-sampled auxiliary variable in X given as
The use of auxiliary information in sample surveys was extensively discussed in well-known classical text books such as [14], [15], [16], [17] and [18] among others. Recent developments in ratio and product methods of estimation along with their variety of modified forms are lucidly described in detail by [19].In this paper, we proposed a class of difference estimator with dual to ratio cum as the slope of the estimator instead of
also,
was used instead of x in the usual regression estimator. The proposed estimator is used to estimate the average sleeping hours of undergraduate students of the department of statistics, Federal University of Technology Akure, Nigeria.The organization of this article is as follows: In Section 2, we provide the conceptual framework of the proposed class of estimator. We derived its bias, Mean Squared Error (MSE) and the resulting optimum value of the MSE, with their rigorous proofs up to order one. In section 3, we compared the MSE of the proposed estimator
with the MSE of
under Simple Random Sampling Scheme, in Section 4, we provide the numerical validation of the proposed estimator by using data on the ages and hours of sleeping by the undergraduate students of the Department of Statistics Federal University of Technology Akure, Ondo State, Nigeria. Finally, Section 5 provides the conclusion of our findings.
2. The Proposed Class of Estimator
For estimating population mean
we have proposed a class of difference estimator with dual to Ratio cum as the intercept given as
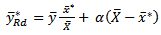 | (1) |
Where α is a suitably chosen scalar. The bias and mean square error (MSE) of
to the first order approximation is obtained by substituting
into equation (1), hence, equation (1) becomes, 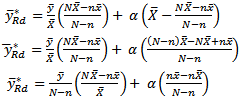 | (2) |
We write,
This implies that
Respectively. Hence, equation (2) becomes,
Where 
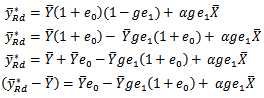 | (3) |
By taking the expectation of equation (3) we have | (4) |
But, 
and
Hence, equation (4) becomes,
Recall, 

The Mean Square Error of the estimator given as
is obtained by squaring both sides of equation (3) and taking the expectation. We have
Ignoring the higher powers of error greater than or equal to 3, we have. | (5) |
Take the expectation of (5) we have 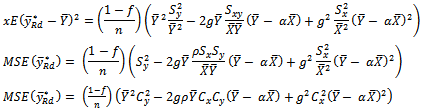 | (6) |
Where
and
The optimum value of the
is given as  | (7) |
Set equation (7) to zero; we have 
Where
and
Thus, the resulting OPTIMUM MSE of
is given as
By substituting
in equation (6)
Where 
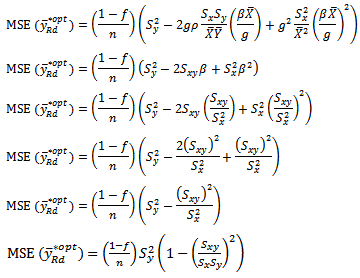 | (7) |
Equation (7) shows that the
is the same as the MSE Regression estimator.Remark The Bias of
is the same as Bias of the dual ratio estimator
and when
becomes
of dual to ratio estimator
proposed by [13].The bias of
is given as
The
is given as  | (7a) |
3. Efficiency Comparisons
In this section, we compared the MSE of the proposed estimator
with the MSE of
under Simple Random Sampling Scheme given as, 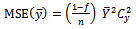 | (8) |
From equations (5) and (8), the proposed estimator is better than that the usual estimator
if,
That is,
This holds if and only if,
Or
The Range of α under which the proposed estimator
is more efficient than
is given as,
We also compared the proposed estimator
with the usual ratio estimator
The MSE of the
is given as
It is found that the proposed estimator
will be more efficient than the usual ratio estimator
if
That is,
This holds if the following two conditions are satisfied
Or
This condition holds if
and
or
and 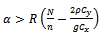
We also compared the proposed estimator
with the usual product estimator
The MSE of the
is given as
It is found that the proposed estimator
will be more efficient than the usual ratio estimator
if 
That is,
This holds if the following two conditions are satisfied
Or
This condition holds if
and
or
and 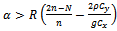
We also compared the MSE of the proposed estimator with MSE of dual product estimator
proposed by [20]. The MSE
proposed by [20] (1980) is given as
It is found that the proposed estimator
will be more efficient than that of [20] estimator
if
That is
That is,
This holds if,
This condition holds if
and
or
and
The range of α under which the proposed estimator
iS more efficient than
is
Lastly, we compared MSE of the proposed estimator
with that of dual to ratio estimator
proposed [13] given in equation (7a). The proposed estimator will be more efficient than
if
That is,
This holds if
Or
This condition holds if
Therefore, the range of α under which the proposed estimator
is more efficient than dual ratio estimator
Thus it seems from the above that the proposed estimator
may be made better than the usual estimator, ratio estimator, product estimator, dual to product estimator
and the dual to ratio estimator
if the given conditions are satisfied.Comparison of ‘AOE’ to 
is more efficient than the other existing estimators
the ratio estimator
the product estimator
, the dual to ratio estimator
and the dual to product estimator
since
Hence, we conclude that the proposed class of estimator
is more efficient than other estimator in case of its optimality.
4. Numerical Validation
To illustrate the efficiency of the proposed estimator over the other estimators
Data on the ages and hours of sleeping by the undergraduate students of the Department of Statistics Federal University of Technology Akure, Ondo State, Nigeria. A sample of 150 out of 461 students of the department was obtained using simple random sampling without replacement. The information on the age of the students was used as auxiliary information to increase the precision of the estimate of the average sleeping hours. The estimate of the average hours of sleeping of the students were obtained and also the 95% confidence intervals of the average hours of sleeping were obtained for the proposed estimator and the other estimators. Table 1., gives the estimates of the average sleeping hours and the 95% confidence Interval. As shown in Table 1.0, the proposed estimator performed better than the other estimators, the width of the confidence interval of the proposed estimator is smallest than the other competing estimators.Table 1. Average Sleeping Hours and 95% confidence intervals for Different Estimators for the undergraduate Students of Department of Statistics, Federals University of Technology Akure. Nigeria 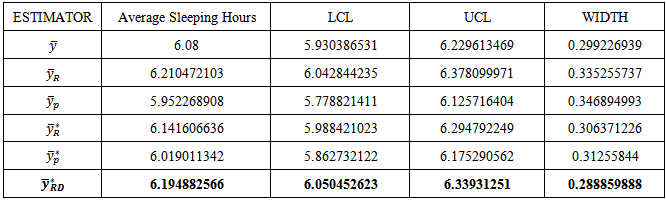 |
| |
|
The proposed estimator performed the same way as the regression estimator when compared with the usual simple random sampling. The average Sleeping Hours and 95% confidence intervals for the proposed estimator and the regression estimator is given below, the two estimators have the same width.To examine the gain in the efficiency of the proposed estimator
over the estimator
we obtained the percentage relative efficiency of different estimator of
with respect to the usual unbiased estmator
in Table 2. The proposed estimator
performed better than the other estimators
and perfoirmed exactly the same way as regression estimator.Table 2. Average Sleeping Hours and 95% confidence intervals for the proposed estimators and regression estimators for the undergraduate Students of Department of Statistics, Federals University of Technology Akure. Nigeria  |
| |
|
Table 3. The percentage relative efficiency of different estimator of with respect to the usual unbiased estimator  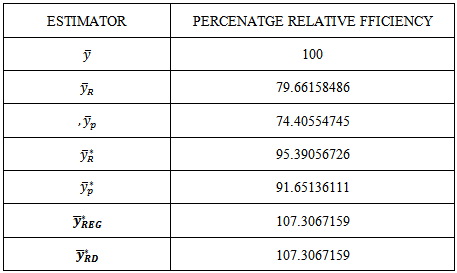 |
| |
|
5. Conclusions
We have proposed a class of regression estimator with cum-dual ratio estimator as intercept for estimating the mean of the study variable Y using auxiliary variable X as in equation (1) and obtained ‘AOE’ for the proposed estimator. Theoretically, we have demonstrated that proposed estimator is always more efficient than other under the effective ranges of
and its optimum values. Table 1. shows that the proposed estimator performed better than the other estimators as the width of the confidence interval of the proposed estimator is smallest than the other competing estimators. The percentage relative efficiency of different estimator of
with respects to the usual unbiased estimator
in Table 2. shows that the proposed estimator
performed better than the other estimators
and performed exactly the same way as regression estimator. Hence, it is preferred to use the proposed class of estimator in practice.
References
[1] | B. K. Handique, “A Class of Regression-Cum-Ratio Estimators in Two-Phase Sampling for Utilizing Information From High Resolution Satellite Data,” ISPRS Ann. Photogramm. Remote Sens. Spat. Inf. Sci., vol. I–4, pp. 71–76, Jul. 2012. |
[2] | S. Choudhury and B. K. Singh, “A Class of Product-cum-dual to Product Estimators of the Population Mean in Survey Sampling Using Auxiliary Information,” Asian J Math Stat, vol. 6, 2012. |
[3] | W. G. Cochran, “The estimation of the yields of cereal experiments by sampling for the ratio of grain to total produce,” J. Agric. Sci., vol. 30, no. 02, pp. 262–275, 1940. |
[4] | S. Choudhury and B. K. Singh, “An efficient class of dual to product-cum-dual to ratio estimators of finite population mean in sample surveys,” Glob. J. Sci. Front. Res., vol. 12, no. 3-F, 2012. |
[5] | D. S. Robson, “Applications of multivariate polykays to the theory of unbiased ratio-type estimation,” J. Am. Stat. Assoc., vol. 52, no. 280, pp. 511–522, 1957. |
[6] | M. N. Murthy, “Product method of estimation,” Sankhyā Indian J. Stat. Ser. A, pp. 69–74, 1964. |
[7] | B. Sharma and R. Tailor, “A new ratio-cum-dual to ratio estimator of finite population mean in simple random sampling,” Glob. J. Sci. Front. Res., vol. 10, no. 1, 2010. |
[8] | H. P. Singh, “Estimation of finite population mean using known correlation coefficient between auxiliary characters,” Statistica, vol. 65, no. 4, pp. 407–418, 2005. |
[9] | R. Tailor and B. K. Sharma, “A modified ratio-cum-product estimator of finite population mean using known coefficient of variation and coefficient of kurtosis,” Stat. Transition-new Ser., vol. 10, p. 1, 2009. |
[10] | L. N. Upadhyaya and H. P. Singh, “Use of transformed auxiliary variable in estimating the finite population mean,” Biometrical J., vol. 41, no. 5, pp. 627–636, 1999. |
[11] | E. J. Ekpenyong and E. I. Enang, “Efficient Exponential Ratio Estimator for Estimating the Population Mean in Simple Random Sampling.” |
[12] | R. Tailor, B. Sharma, and J.-M. Kim, “A generalized ratio-cum-product estimator of finite population mean in stratified random sampling,” Commun Korea Stat Soc, vol. 18, no. 1, pp. 111–118, 2011. |
[13] | T. Srivenkataramana, “A dual to ratio estimator in sample surveys,” Biometrika, vol. 67, no. 1, pp. 199–204, 1980. |
[14] | W. G. Cochran, “Sampling techniques.,” 1977. |
[15] | P. V Sukhatme and B. V Sukhatme, “Sampling theory of surveys with applications,” 1970. |
[16] | P. V Sukhatme, B. V Sukhatme, S. Sukhatme, and C. Asok, “Sampling theory of surveys with applications.,” 1984. |
[17] | M. N. Murthy, “Sampling theory and methods.,” Sampl. theory methods., 1967. |
[18] | F. Yates, “Sampling methods for censuses and surveys.,” Griffin Books Stat., 1960. |
[19] | S. Singh, Advanced Sampling Theory With Applications: How Michael Selected Amy, vol. 2. Springer Science & Business Media, 2003. |
[20] | S. Bandyopadhyay, “Improved ratio and product estimators,” Sankhya Ser. C, vol. 42, no. 2, pp. 45–49, 1980. |