Michael L. Bukwimba
Research Scholar in the Department of Statistics at Acharya Nagarjuna University, Nagar India, And he is working for the Institute of Finance Management, Dar es Salaam, United Republic of Tanzania
Correspondence to: Michael L. Bukwimba, Research Scholar in the Department of Statistics at Acharya Nagarjuna University, Nagar India, And he is working for the Institute of Finance Management, Dar es Salaam, United Republic of Tanzania.
Email: |  |
Copyright © 2015 Scientific & Academic Publishing. All Rights Reserved.
Abstract
Insurance companies, financial institutions and any other business firms should conduct what we call self evaluation on whether they are playing within the risk free boundaries by applying the random walk technique in determining the extreme points. This paper will concentrate on evaluating the memory less timeTat which the company is assumed to reach the highest return, or at which the company will achieve weak minimum return. At either point, it is said to be unsafe for the profit oriented firms to operate. The extreme value theory is highly employed in Actuarial Industry particularly in financial risk management when the company or firm wants to set out the risk free demarcations to operate or play around, and in the situations where the Company wants to conduct self performance evaluation, making forecast over a period of time and making any Economical based decisions. The author believe that, there is a more and detailed information contained in the text that follows, and it is his sincere hope that this paper will increase motivation of researchers interested in a more broadly based risk management in finance.
Keywords:
Random Walk, Financial risk Assessment, Stopping Times, Memory less Time, Peaks and Dips
Cite this paper: Michael L. Bukwimba, Extreme Value Theory in Financial Risk Management: The Random Walk Approach, International Journal of Probability and Statistics , Vol. 4 No. 1, 2015, pp. 32-35. doi: 10.5923/j.ijps.20150401.03.
1. Introduction
The extreme value theory is a blend of an enormous variety of applications involving natural phenomena such as rainfall, floods, wind gusts, Air pollution, corrosion etc (Chang Hsien-kuo).Historically, work on extreme value problem may be dated back to as early as 1709 when Nicolas Bernoulli discussed the mean largest distance from the origin when points lie at random on a straight line of length . [see Gumbel, 1958]In the history of finance, risk management has been identified as one of the most important field of interest to financial and risk Managers in the 20th century, or rather as among the three major areas of interest following the Markowitz portfolio theory and the Black-Sholes-Merton option pricing theory (Cairns A, 2004). In recent years, we have noticed evidences all over the world the huge development of the field of financial risk management which resulted from the global financial crisis that emerged in 2008 which intensified the need of risk management among financial institutions and insurance companies (TANG Qihe).The Extreme value theory (EVT) holds promise for advancing assessment and management of extreme financial risks. Recent literature suggests that the application of Extreme value Theory generally results in more precise estimates of extreme quantiles and tail probabilities of financial asset returns (Embrechts P. et al, 1999).In regard to this paper, the author will discuss concisely the application of Extreme value theory in the field of financial risk management which is the foremost objective for financial institutions and insurance companies by the help of the Random Walk concept.
1.1. Extreme Value Theory
Extreme value theory (EVT) is a tool used to determine probabilities (Risks) associated with extreme events. It is used by Investors in situations where there is/expected to occur higher stress on investment portfolios. The EVT is also used to model the behavior of tips (Maxima) and or dips (Minima) in a series of asset returns etc.VaR approach was the standard measure of financial risks and other risks such as Industrial risk management etc. Basically, it used to measure the expected loss over a period of time for known distribution of for known probability and under normal market conditions. (Longuin, 1999).Recently, Portfolio managers, Investors, Risk managers, Claim managers etc, have become more concerned over occurrences under “Extreme market conditions”.This paper is organized as follows: Section 2 introduces the risk management and approaches to minimize financial risks, section 3 describes the Random walk movement as a result of extremes, and section 4 concluding.
2. Risk Management
Risk management is the process of Identification, Assessment and Decision making over the risks facing an Organization. It can be Formal process which involves Procedures, Quantitative and Qualitative assessments, or it can be Informal (Peter Moles, 2013).
2.1. Approaches for Decision Making
After the risk has been identified on its nature and type, assessment has taken place i.e how big the risk is, the probability to occur and when it is going to happen, then, the decision makers of the Organization or Firm may opt on the following three generic approaches:• Hedging: Eliminate risk by selling into the market place through cash or spot market transactions or through Forward, Future and Swap contracts.• Diversification: This reduces risk by combining risks which are not perfectly correlated to form a portfolio.• Insurance: Risks are transferred from the buyer (Policyholder) to the seller i.e Insurer (Peter Moles, 2013).
3. Intuition of the Random Walk
It is essential for Insurance Companies, Financial Institutions and any profit oriented firm to conduct an evaluation as to when the company is expected to earn a maximum returns or highest profit together with the question how much is that maximum return value.On the other hand, it would be of important interest for the company to know when it is expected to be ruined so that the required but optimal measures can be adopted against the crisis and also to rescue from loss of potential shareholders and against bad reputation to customers. The company may also be interested to learn whether it will remain liquid forever i.e what happens to the company’s returns at
, and ultimately what will be the distribution of the value of
so that it can ensure the capital of their shareholders as well as to ensure continuous dividend payments and other benefits to its customers.This paper tried to explore some mathematical techniques which will be helpful in computing the time at which the company will be at the highest peak (maximum returns) or the least dip (minimum returns), as well as when
.The returns will be mainly expressed in discrete time (Random Walk) and then studying a variety of stopping times using the strong Markov property. The cycles which are generated by stopping times iterates are assumed to be independent and identically distributed.Let us now introduce the memory less time
with distribution
where is smaller than (but close to 1 so that
is large). Since we are interested with the maximum and minimum before
, these will be determined by the last peak stopping time
and the last dip stopping time
before time
respectively.Now, by distribution
Where
and
are independent stopping times, similarly
Where
denotes the sum of independent random variables, and that
and
is the value at the highest peak stopping time and the value at the least dip stopping time respectively and they are said to be independent random variables.
3.1. Random Walk
The idea of Radom Walk originated from considering a completely a drunk person who walks with no sense of direction. So she /he may move forward with equal probability that she/he moves backwards. A similar notion is found in financial environment or financial markets where the returns from a single asset have found to be mutually independent and identically distributed.By definition: If we let
be a sequence of independent and identically distributed
random variables (Returns), and that
to be a stochastic process, then
Where
Again let us also consider the following situationIf
are independent and identically distributed (i.i.d) random variables and let us fix some set A such that
i.e the first time any member of
to be contained in set A. also we know that
Then
The geometric Random walk model implies that the future is independent of the past and therefore it is not possible to predict the future deviation trends.
3.2. Stopping Times
Stopping time is a random variable
which takes place in units of time such that
is a function of
for all
. Examples is like the first time returns of the company reach a certain peak, and or the first time a share price reaches a certain level after dropping by a specified amount.Let us now consider the iterates of a stopping time
such that, if we define
be the n-th iterate, and let
be defined by
, then we can say that
Thus, in general
In this case a set of independent random stopping times are generated i.e
. In other words we can say that
for
are the peaks such that the returns reaches before falling, and therefore 
in terms of their magnitudes.But our major interest is on the last peak before time
Let,
Be the counting process associated to the iterates of stopping times
, then
, for all
Where
becomes the last time before memory less time
of which we are interested in.
3.3. Random Walk Stopping Times and the Strong Markov Property
A stopping time is a random variable taking values in the time – set including possibly the value of
such that
is a deterministic function of
for all
.The Markov property states that: For any
the future process
is independent of the past process
if we know the present
, and thus this remains true if we replace the deterministic
by a random stopping time
.Strong Markov property: Suppose
is a time – homogeneous Markov chain. Let
be a stopping time, then conditional on
and
, the future process
is independent of the past process
and has the same law as the original process started from
.
3.4. Stopping Times Path into Cycles
Let us also consider another random walk
By tossing a fair coin, let
where
and
, also let us define
be the first cycle to contain
and that
for which
And that
are independent and identically distributed random cycles produced by stopping times iterates such that;
3.5. Peaks and Dips of Cycles
In this section therefore, we will concentrate on peaks and dips of various cycles formed during the process, particularly the last peak and dip before success time
, that is before the time at which the company attains the maximum value and or the weak minimum value.Let
be the time for the first peaki.e
it follows that
for and
Similarly,Let
be the time for the first dipi.e
and hence
for
and
And therefore we can say that
is the k-th time
value becomes strictly larger than all previous values defined by
Similarly we can also say that
is the k-th time
takes smaller value than or equal to all previous ones, defined by
Thus, the corresponding counting processes are
For this reason we can say that,
Where
and
are the last peak and dip before hitting time
respectively.Now, from section 1.4, we know that
and
; again let us think the situation when
and define
then
After several observations of tosses of a biased coin, assume now that we got the cycle that contain success. Let us now define the following notations.
be the last peak before success time 
be the last dip before success time
Also, let
and
be the values of the last peak and dip before success time
respectively. And since
and
they have nothing in common (they are independent), then 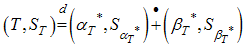
4. Conclusions
The extreme value theory is highly employed in Actuarial industry, particularly in the situations where the Company wants to conduct self performance assessment, identifying investment risks, financial risk assessment, making forecast over a period of time and making any Economical based decisions.Returns evaluation or financial risk management and time at which the firm is expected to achieve the maximum/minimum returns (profit/loss) are fundamental tasks to the company and to all profit oriented firms (Risk/Claim managers) as this will help them to identify their risk free operating limits in the sense that; it is too risky for the company to operate at extreme points. For managerial purposes therefore, returns evaluation / assessment has a greater importance to managers and other decision makers to know their risk free operating boundaries.Lastly, since the computations involve strong mathematical models, we clearly need computer software to be able to reach the targeted goals as it is realy convolution to get solution by hand.
References
[1] | Acerbi, C., Nordio, C., & Sirtori, C. (2001). Expected shortfall as a tool for financial risk management. arXiv preprint cond-mat/0102304. |
[2] | Bai, L.J, Jakeman, A.J, and McAleer, M. (1992). On the use of extreme value distributions for predicting the upper percentiles of environmental quality data, Mathematics and Computers in simulation, 33, 483-488. |
[3] | Balakrishnan, N., and Chan, P.S. (1992c). Extended tables of means, variances and covariances of order statistics from the extreme value distribution for sample sizes up to 30, Report, department of Mathematics and Statistics, McMaster University, Hamilton, Canada. |
[4] | Cairns A. (2004) Interest Rate Models: An Introduction |
[5] | Embrechts P., Resmick S.I. and Gennady S. (1999), Extreme value Theory as a Risk Management Tool. North American Actuarial Journal. 3(2). |
[6] | Francis X. Diebold, Til Schuermann, John D. Stroughair (2000), “Pitfalls and opportunities in the use of extreme Value Theory in Risk Management”, The Journal of Risk Finance. 1(2):30-35. |
[7] | London R.R, Mckean H.P, Rogers L.C.G, Williams D. (1982). “A Martingale Approach to some Wiener-Hopf problems”. I. sminaire de probabilits de Strasbourg. 16:41-67. |
[8] | Manfred G., Evis Këllzi (2006), “An application of extreme Value Theory for measuring Financial Risk”. Computational Economics. 27(2-3):207-228. |
[9] | McNeil, A. J. (1999). Extreme value theory for risk managers. Departement Mathematik ETH Zentrum. |
[10] | Peter Moles (2013), Financial Risk Management. |
[11] | Rogers L.C.G, Willium D. (1982), “Time-substitutional based on fluctuating additive function (Wiener-Hopf factorization for infinitesimal generators). Sminaire de probabilits de Strasbourg. 14:332-342. |
[12] | WILLIAMS D. (1991), Some Aspects of Wiener-Hopf Factorization, Phil.Trans. R. lond. A 335, 593-608. |