Peeyush Misra, R. Karan Singh
Department of Statistics, Lucknow University, Lucknow, India
Correspondence to: Peeyush Misra, Department of Statistics, Lucknow University, Lucknow, India.
Email: |  |
Copyright © 2015 Scientific & Academic Publishing. All Rights Reserved.
Abstract
For estimating finite population variance using information on single auxiliary variable in the form of mean and variance both, the Ratio-Product-Difference (RPD) type estimators are proposed. The generalized cases of these estimators leading to the classes of estimators are also proposed. The bias and mean square error (MSE) of the proposed estimators are found. Theoretical comparisons with the traditional estimator are supported by a numerical example. By this comparison it is shown that the proposed estimators are more efficient than the traditional one.
Keywords:
Auxiliary Variable, Taylor’s Series Expansion, Bias, Mean Square Error (MSE) and Efficiency
Cite this paper: Peeyush Misra, R. Karan Singh, Estimators for Finite Population Variance Using Mean and Variance of Auxiliary Variable, International Journal of Probability and Statistics , Vol. 4 No. 1, 2015, pp. 1-11. doi: 10.5923/j.ijps.20150401.01.
1. Introduction
In sampling theory, auxiliary information is used widely at both the stages of selection and estimation. At the estimation stage, auxiliary information is used by formulating various types of estimators of different population parameters with a view of getting increased efficiency and are available in plenty in the literature.Let U = (1, 2, . . . , N) be a finite population of N units with Y being the study variable taking the value
for the unit i of U and X being the auxiliary variable taking the value
for the unit i of the population, i = 1, 2, . . . , N.Let
be the population of mean of Y and
be the population mean of X. Also, let
,
and
For a simple random sample of size n drawn from U with the sample observations
,
, . . . ,
on
and
,
, . . . ,
on
, let
and
be the sample means of y-values and x-values respectively.
2. The Suggested Estimators
We know that the finite population variance
of the study variable is | (2.1) |
From (2.1) it is natural to get an estimator of
if we replace
and
by their some estimators. In particular if
is estimated by
and
is estimated by
, we get the estimator of
as follows | (2.2) |
and its generalized estimator as 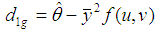 | (2.3) |
where
and
and f (u , v) satisfying the validity conditions of Taylor’s series expansion is a bounded function of (u , v) such that f(1, 1) = 1.Also if
is estimated by
and
is estimated by
, we get another estimator of
as follows | (2.4) |
and its generalized estimator as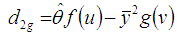 | (2.5) |
where
and
, f(u) and g (v) both are bounded functions in u and v respectively such that
= 1 at the point u = 1 and
= 1 at the point v = 1 and both are satisfying the regularity conditions for the validity of Taylor’s series expansion having first two derivatives with respect to u and v respectively to be bounded.
3. Bias and Mean Squared Error of Suggested Estimators
(a) Bias and Mean Square Error of Suggested Estimator
Let
,
,
and
,For simplicity, it is assumed that the population size N is large enough as compared to the sample size n so that finite population correction terms may be ignored. Now | (3.1) |
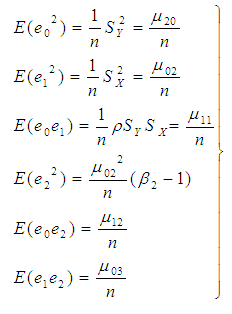 | (3.2) |
and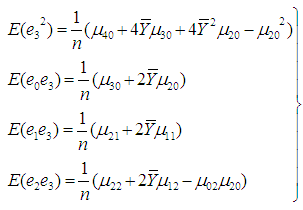 | (3.3) |
Let us consider the proposed estimator
defined in (2.2)
or | (3.4) |
Taking expectation on both sides of (3.4), the bias in
to the order
is given byBias
Using values of the expectations given from (3.1) to (3.3), we have | (3.5) |
Now squaring (3.4) on both sides and then taking expectation, the mean square error of
to the first degree of approximation is given by
or  | (3.6) |
For minimizing (3.6) in two unknowns
and
, the two normal equations after differentiating (3.6) partially with respect to
and
are 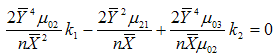 | (3.7) |
 | (3.8) |
Solving (3.7) and (3.8) for
and
, we get the minimizing optimum values to be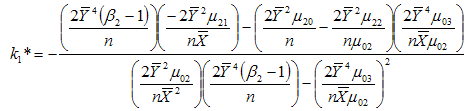 | (3.9) |
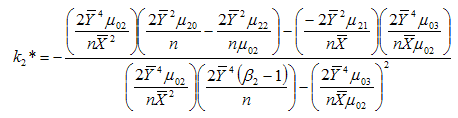 | (3.10) |
which when substituted in (3.6) gives the minimum value of mean square error of the estimator
as | (3.11) |
(b) Bias and Mean Square Error of Suggested Estimator
For f1 and f2 being the first order partial derivatives of
with respect to u and v respectively at the point (1,1), that is
expanding
in (2.3) in third order Taylor’s series about point (1, 1), we have
where f1 and f2 are already defined; f11, f22 and f12 are the second order partial derivatives given by
and u* = 1 + h ( u- 1) , v *= 1 + h ( v-1) , 0 < h < 1.or 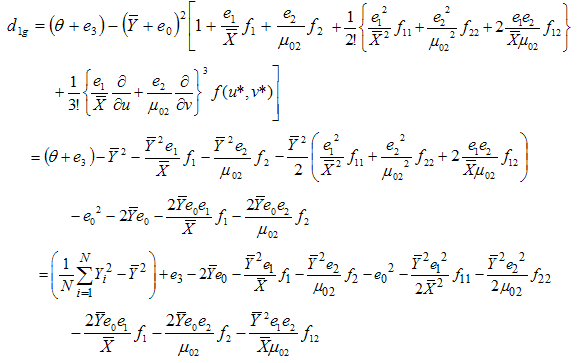
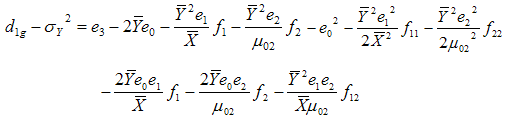 | (3.12) |
Taking expectation on both sides of (3.12) and using values of the expectations given from (3.1) to (3.3), the bias in
to the order
is given by
 | (3.13) |
Now squaring (3.12) on both sides and then taking expectation, the mean square error of
to the first degree of approximation is given by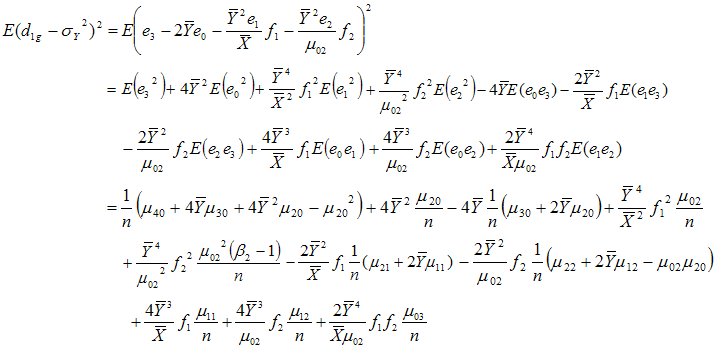
 | (3.14) |
For minimizing (3.14) in two unknowns f1 and f2 , the two normal equations after differentiating (3.14) partially with respect to f1 and f2 are | (3.15) |
 | (3.16) |
Solving (3.15) and (3.16) for f1 and f2, we get the minimizing optimum values to be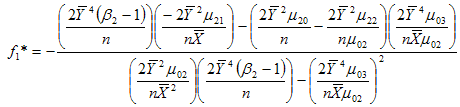 | (3.17) |
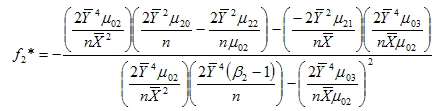 | (3.18) |
which when substituted in (3.14) gives the minimum value of mean square error of the estimator
as | (3.19) |
(c) Bias and Mean Square Error of Suggested Estimator
Let us consider the proposed estimator
defined in (2.4)
or | (3.20) |
Taking expectation on both sides of (3.20), the bias in
up to terms of order
is given by
Using values of the expectations given from (3.1) to (3.3), we have | (3.21) |
Now squaring (3.20) on both sides and then taking expectation, the mean square error to the first degree of approximation is
or | (3.22) |
For minimizing (3.22) in two unknowns
and
, the two normal equations after differentiating (3.22) partially with respect to
and
are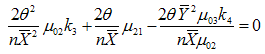 | (3.23) |
 | (3.24) |
Solving (3.23) and (3.24) for
and
, we get the minimizing optimum values to be 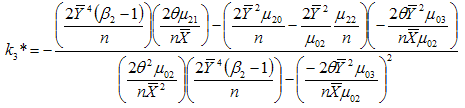 | (3.25) |
and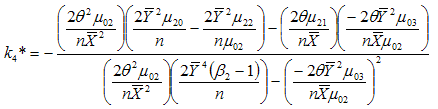 | (3.26) |
which when substituted in (3.22) gives the minimum value of mean square error of the estimator to be | (3.27) |
(d) Bias and Mean Square Error of Suggested Estimator
For
,
and
to be first, second and third order derivatives of
at the point u = 1, u* = 1 + h1 (u-1), 0 < h1 < 1 , also
,
and
to be first, second and third order derivatives of
at the point v = 1 and v* = 1 + h2 (v-1), 0 < h2 < 1, expanding
and
in
in third order Taylor’s series, we have
or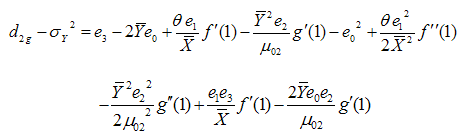 | (3.28) |
Taking expectation on both sides of (3.28) and using values of the expectations given from (3.1) to (3.3), the bias in 
to the order
is given by | (3.29) |
Now squaring (3.28) on both sides and then taking expectation, the mean square error of
to the first degree of approximation is given by
Using values of the expectations given from (3.1) to (3.3), we have
or 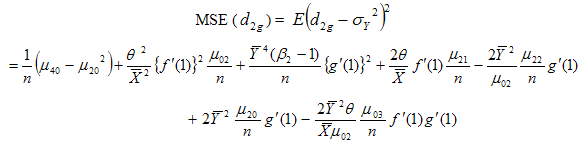 | (3.30) |
For minimizing (3.30) in two unknowns
and
, the two normal equations after differentiating (3.30) partially with respect to
and
are | (3.31) |
and | (3.32) |
Solving (3.31) and (3.32) for
and
, we get the minimizing optimum values to be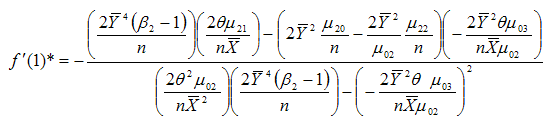 | (3.33) |
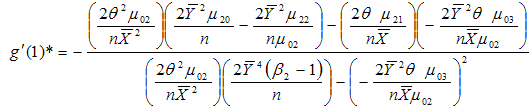 | (3.34) |
which when substituted in (3.30) gives the minimum value of mean square error as | (3.35) |
4. Efficiency Comparison with the Traditional Estimator
As we know that the mean square error of usual conventional unbiased estimator
of population variance
is
and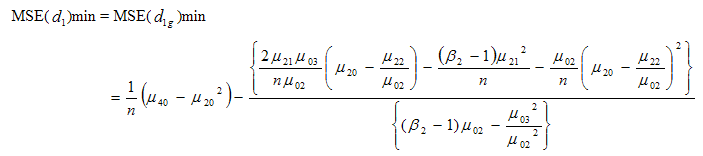 | (4.1) |
and 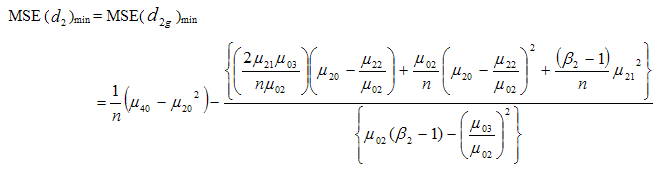 | (4.2) |
Comparative studies regarding their efficiency over the usual conventional unbiased estimator are carried out with the help of a numerical illustration.Considering the data given in Cochran (1977) dealing with Paralytic Polio Cases ‘Placebo’ (Y) group, Paralytic Polio Cases in not inoculated group (X), computations of required values of
have been done and comparisons are made for a simple random sample of size n. For the data considered, we have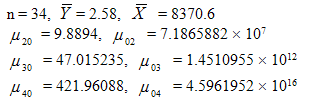
Using above values, we haveMean Square Error of usual conventional unbiased estimator = 9.534136695 and
Hence the percent relative efficiency (PRE) of the proposed estimators
,
,
, and
over the usual conventional estimator are given by
showing that the proposed estimators
,
,
, and
are more efficient with highly significant percent relative efficiency over the usual conventional unbiased estimator of the population variance.
5. Conclusions
We have derived new sampling estimators of population variance using auxiliary information in the form of mean and variance both, the bias and mean square error equations are obtained. Using these equations, MSE of proposed estimators are compared with the traditional estimator in theory and shown that the proposed estimators have smaller MSE than the traditional one.
ACKNOWLEDGEMENTS
The authors are thankful to the referees and the Editor- in- chief for providing valuable suggestions regarding improvement of the paper.
References
[1] | Cochran, W. C. (1977) – ‘Sampling Techniques’, 3rd Edition, John Wiley and Sons, New York. |
[2] | Das, A. K. and Tripathi, T. P. (1978) – Use of auxiliary information in estimating the finite population variance. Sankhya, Vol. 40, Series C, 139-148. |
[3] | Singh, R. K., Zaidi, S. M. H. and Rizvi, S. A. H (1995) – Estimation of finite population variance using auxiliary information, Journ. of Statistical Studies, Vol. 15, 17-28. |
[4] | Singh, R. K., Zaidi, S. M. H. and Rizvi, S. A. H.(1996) – Some estimators of finite population variance using information on two auxiliary variables., Microelectron, Reliab., Vol. 36, No. 5, 667-670. |
[5] | Srivastava, S. K. and Jha, J. J. H. S. (1980) – A class of estimators using auxiliary information for estimating finite population variance, Sankhya, Vol. 40, Series C, 87-96. |