Festus Kehinde Ojo, Damilare Oluwole Akande, Abiodun Idris Azeez, Gabriel Ayobamidele Oyeola
Department of Electronic and Electrical Engineering, Ladoke Akintola University of Technology, Ogbomosho, Nigeria
Correspondence to: Festus Kehinde Ojo, Department of Electronic and Electrical Engineering, Ladoke Akintola University of Technology, Ogbomosho, Nigeria.
Email: |  |
Copyright © 2015 Scientific & Academic Publishing. All Rights Reserved.
Abstract
The recent developments in wireless industries have led to a remarkable increase in spectrum demand. Literatures have shown that the scarcity of radio spectrum is mainly due to the inefficiency of traditional static spectrum allocation policies and the available spectrums are also underutilized. Cognitive Radio offers a solution by utilizing the spectrum holes that represent the potential opportunities for non-interfering use of spectrum. The reduction of this scarcity is however achieved by improving the spectrum sensing performance. A typical type of spectrum sensing task is cooperative spectrum techniques. The main concept behind cooperative spectrum sensing is to enhance the sensing performance by exploiting the spatial diversity in the observations of spatially located cognitive radio (CR) users by cooperation. CR users can share their sensing information for making a combined decision more accurate than the individual decisions. This paper presents cooperation method using Centralized Cooperative Spectrum Sensing (CCSS) and Decentralized Cooperative Spectrum Sensing (DCSS) based on the probability of true detection (Pd) and the probability of false detection (Pf). The Pd being important to the primary user as it indicates their protection level from secondary users just as the (Pf) is important to the secondary users as it indicates their usage of an unoccupied channel. The simulation results shows that the robust and reliable detection of primary user (PU) is possible even at very low SNR and increases proportionately with the number of secondary user to a certain level which is of crucial importance in cooperative sensing techniques in cognitive radio.
Keywords:
Cognitive radio, Spectrum sensing, Probability of true detection, Probability of false detection
Cite this paper: Festus Kehinde Ojo, Damilare Oluwole Akande, Abiodun Idris Azeez, Gabriel Ayobamidele Oyeola, Investigation of Interference in Cognitive Radio Using Cooperative Sensing Techniques, International Journal of Networks and Communications, Vol. 5 No. 3, 2015, pp. 41-45. doi: 10.5923/j.ijnc.20150503.01.
1. Introduction
Over the years, it has been observed that the scarcity of radio spectrum is mainly due to the inefficiency of traditional static spectrum allocation policies. This issue leaves little or no spectrum for future demands; Spectrum scarcity has thus become increasingly serious leading to intensified attention [1, 2]. Cognitive radio network (CRN) has emerged as a promising technology to communicate efficiently (FCC, 2002). A typical CRN is comprises of two types of users: primary users (PUs) and secondary users (SUs). PUs are authorized to utilize licensed bands/channels whenever they have demands. In contrast, SUs are not licensed users, but they are allowed by PUs to temporarily access channels without harmful interference to the PUs through dynamic spectrum access (DSA).If the interference from SUs to PUs is dominant and destructive, SUs have to restraint from transmission. This concept of cognitive radio enables coexistence of the legal user (primary user) and cognitive users (secondary users), [3]. This distinct feature of CRNs raises an essential and challenging question, i.e how to accurately estimate or predict interference from SUs to PUs. Spectrum sensing (SS) plays a crucial role in the implementation of the cognitive radio technology, it provides the ability for secondary users (SUs) to detect the unused spectrum and sharing it without harmful interference to primary users (PUs). Spectrum sensing techniques can be classified into two main categories: Local sensing or Primary detection, and Cooperative sensing. In local Sensing each cognitive radio users must independently have the capability to determine the presence or absence of the primary user in a certain spectrum [5]. This method although is easy in terms of computation and Implementation, thus they are sensitive to model uncertainty, fading and shadowing. Research shows that there are several proposed methods for local SS such as matched filtering, Waveform-Based Sensing, Cyclo-stationarity Based Sensing and Energy Detector-Based Sensing. For Cooperative sensing technique, information from multiple cognitive radio users are appropriately incorporated for PUs detection [3, 5]. This approach enhances the accuracy and reliability of the PUs detection and it is robust to fading, shadowing and model uncertainties. In this paper, Cooperative spectrum sensing technique is being considered particularly an optimal fusion strategy based centralized and decentralized spectrum sensing schemes are investigated and analyzed. In Centralized Cooperative Spectrum Sensing (CCSS) a central node collects hard or soft sensing information from cognitive radio users, identifies the available spectrum, and broadcasts this information to other cognitive radio users or directly controls the cognitive radio traffic. Where in Decentralized Cooperative Spectrum Sensing (DCSS) cognitive nodes share information through local communications, in-order to make their own decisions as to which part of the spectrum can be used [4, 5].The Rest of this research paper is organized as: Section 2 represents cooperative spectrum sensing techniques, Section 3 represents the steps for evaluating cooperative sensing, and Section 4 presents conclusions.
2. Cooperative Spectrum Sensing
In cooperative spectrum sensing, information from multiple cognitive radio users are appropriately incorporated for PU detection. This method enhances the accuracy and reliability of the PU detection and it is robust to fading, shadowing and model uncertainties.
2.1. Centralized Cooperative Spectrum Sensing (CCSS)
In this case, the cognitive radio network is provided by a fusion center or a Base Station (BS) which can be used for decision making in the spectrum sensing mode. The following steps are required to carry out CCSS:Step1: Let the number of SUs senses the channel for N consecutive time and observe a complex baseband-equivalent signal be: | (1) |
Step 2: If z in (2) is the baseband-equivalent signal transmitted by the PUs to the kth SU over a noisy, flat-fading and time-invariant channel, thenStep 3: The base station collects the information through the noisy channels and the received signal is given as thus: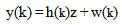 | (2) |
where
is a Circularly Symmetric Complex Gaussian Noise (CSCGN), h(k) is a CSCG channel gain, i.e.
(representing Rayleigh fading between the PUs and the kth SUs, Assumed that z, h(k) and w(k) are independent.At the kth SUs, an energy detector is used to make a local decision u (k) = y(k) (xk) which is then transmitted to the FC for the central decision making. However, two different types of decision can be distinguished: soft and hard. In soft decision the kth SU sends its energy directly to FC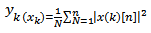 | (3) |
and in hard decision u(k) is 0 unless the energy exceeds a threshold, | (4) |
Where I is the indicator function. Soft decisions are transmitted to the FC and in contrast to previous studies which SU-FC channels are assumed to be perfect control, here, an Additive White Gaussian Noise (AWGN) channel n(k) is considered between each SUs and the FC. Therefore, the received signal at the FC from kth SUs is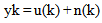 | (5) |
where u(k) is the energy of the signal. Hence, the evidence available to the FC to make the global decision at time i is the set of SU-FC channel outputs 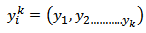 | (6) |
2.2. Decentralized Cooperative Spectrum Sensing (DCSS)
In DCSS, there is no FC to collect all the information and make a unanimous decision. However, some of the SU’s can share their information with each other to make the decision processing more reliable. A DCSS algorithm is proposed which improves the reliability of decisions made by the SU’s.Assume G = (V, E) is a model on the SU’s where each node is a SU, also
if and only if there is a communication link between node I and j. Assume that these links are independent Binary Symmetric Channels (BSC) with cross probability
which means if u is the input and y is the output then
Note that interference from primary user is modeled as noise.The proposed DCSS step is as follows.Step 1: At the first iteration of the algorithm (nodes are assumed to be synchronized), every node senses the channel and based on the received energy makes a hard decision.Step 2: Let the jth node decision be uj(1) (j = 0, 1,…,K - 1).Step 3: Then every node sends its decision (uj(1)) to its neighbors, assume the output of theBinary Symmetric Channels (BSCs) be yjt(1) where
this completes the first iteration.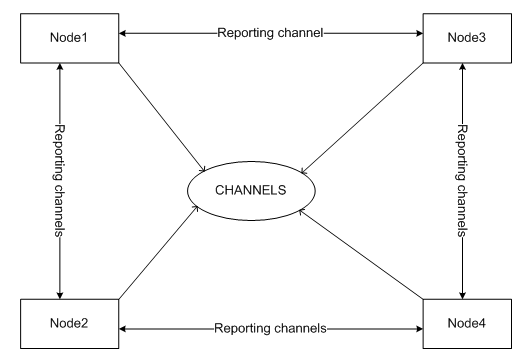 | Figure 1. DCSS System Model |
In the second iteration, every node makes a new decision (uj(2)) based on the data that it had, (uj(1)), and the data that it received from the neighbors,
At this point SUj’s decision is based on its own information and the 1-neighbor 1 information. This process is being continued till the point that every node has the whole information. N.B i is a k-neighbor of j if and only if the shortest path from j to i is of length k [5].
3. Results and Discussion
The performance metrics used include Signal to Noise ratio (SNR) and their mean sensing time. These are based on the energy detection method as this has been the most studied model. However, the results obtained are being modified for use in cooperative sensing techniques using CCSS and DCSS. CCSS techniques are compared under their probabilities of true detection and probabilities of false alarm i.e. probability of false detection. The results of the simulation for the probability of true detection, and for the probability of false detection in the centralized cooperative spectrum sensing (CCSS), and decentralized cooperative spectrum sensing (DCSS) for some number of users are presented in Figure 2. It can be seen from the plot that as the secondary users (k) increases to a certain number, the probability of true detection also increases; this means that the chances of detecting the presence of primary users is ultimately higher as the number of secondary user who are cooperating increases. Also, as the signal-to-noise ratio (dB) increases, the probability of true detection also increases in proportional to the secondary users.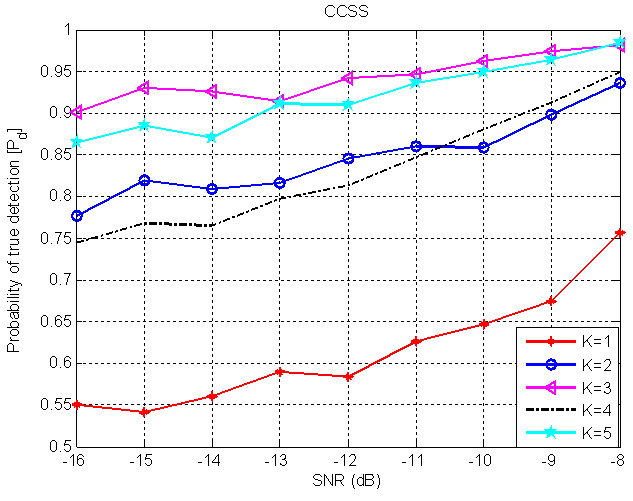 | Figure 2. Probability of true detection [pd] against SNR (dB) using CCSS |
Figure 3 shows that as the number of secondary users (k) increases, the probability of false detection decreases; this means that the chances of having a false detection of the presence of primary users is lower as the number of secondary user cooperation increases. however, since spectrum sensing is the most important aspect of cognitive radio network, accurate decision must be made in detecting the presence of PUs in the spectrum so as to ultimately reduce the effect of interference in the channel.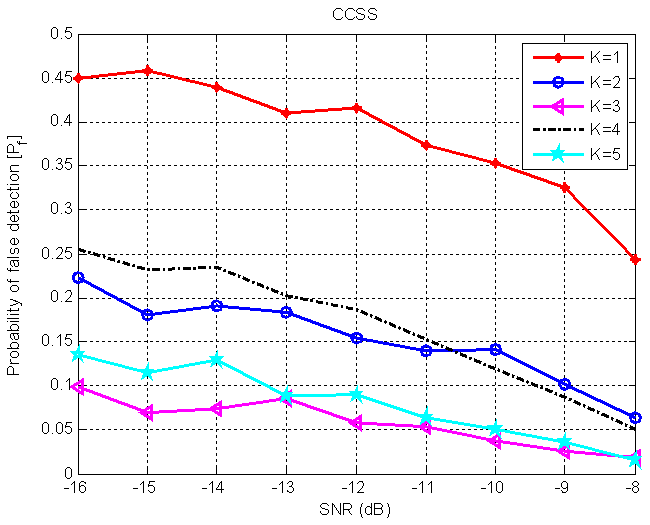 | Figure 3. Probability of false detection [pf] against SNR using CCSS |
Figure 4 and Figure 5 shows the plot of probability of false detection [pf] against SNR (dB) using DCSS for user one and user two respectively. The performance of the system can be improved by increasing the number of iterations performed for SU1. However in SU2 the performance is improved in the second iteration and decreased a bit for high SNRs in the third iteration. The reason is that; SU2, has gathered all the relevant information in the second iteration and the third is just adding more randomness in the decision which reduces the performance. Therefore it is necessary that nodes run the iteration not more than required to gather all the information needed.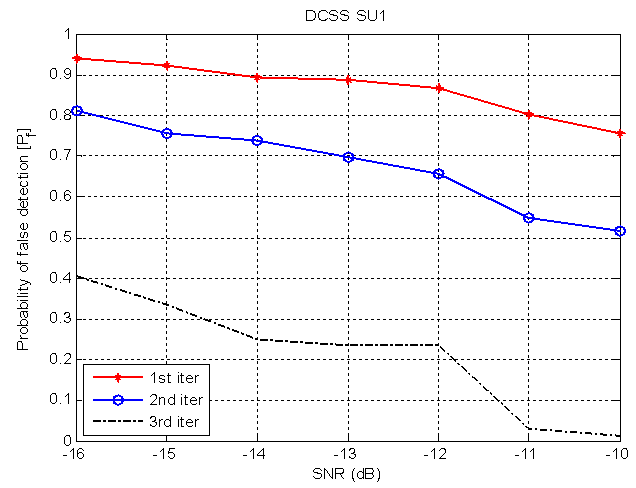 | Figure 4. Probability of false detection [pf] against SNR using DCSS for user one |
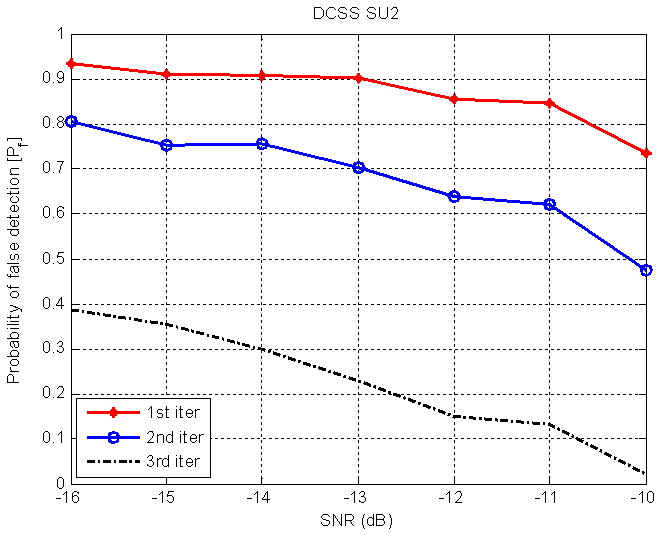 | Figure 5. Probability of false detection [pf] against SNR using DCSS for user two |
4. Conclusions
Cooperative spectrum sensing methods are of great importance in cognitive radio because they are robust against impairments in wireless communication systems, they improve the coordination and cooperation between SUs. Consequently, cooperative sensing techniques enhance the accuracy and reliability of the spectrum sensing in cognitive radio network. The simulation results showed that the robust and reliable detection of PUs is possible even at very low SNR’s which is of crucial importance in cognitive radio. A comparison between the performances of the Energy detection models in CCSS indicates that capturing the dependencies in the PU’s activities at different times can significantly improve the performance of the system. It is clear that using the CCSS, the detection of the PUs is possible even at very low SNR’s. In addition, by increasing the number of SUs the probability of wrong detection was decreased. This is expected, because having more SUs provides more information at FC and accordingly, more reliable decisions can be made, but as the number of secondary user increase to certain level it is understood that the interference in the channel increases thereby causing enormous problem in detecting the PUs. The results also showed how the flexibility and lack of need of the centralized fusion center in DCSS can be compromised with the reliability and accuracy of spectrum sensing in CCSS. However, in both case (CCSS, DCSS) the performance is improved by the increase of the number of SUs. Therefore, careful considerations must be made in the design of cognitive radio by making an appropriate trade-off between available resources and goals.
References
[1] | Zeng, F. Li, C. Tian, Z. (2010) Distributed compressive spectrum sensing in coopertive multihop cognitive network, IEEE Journal of Selected Topics in Signal Processing. |
[2] | Mitola J, and Maguire, G.Q. (1990) “Cognitive radios: makingsoftware radios morepersonal,” IEEE Personal Communication, vol.6, no.4, pp.1318. |
[3] | Mansi Subhedar and Gajanan Birajdar (2011). “Spectrum Sensing Techniques in Cognitive Radio Networks A Survey”, International Journal of Next – Generation Networks, Vol.3.No.2. |
[4] | Mishra, S.M., Sahai, A., and Brodersen, R.W. (2006) “Coperative sensing among cognitive radios,” in proc.IEE ICC.pp.1658-1663. Istanbul, Turkey. |
[5] | Noorshams N., Malboubi M., and Bahai A., (2010) Centralized Decentralized Cooperative Spectrum Sensing in Cognitive Radio Networks: a novel Approach, IEEE 11th international workshop on signal processing advances in wireless communications (SPAWC), Vol.1-5. |