Hoa Tran
Department of Computer Information Sciences, Fordham University, 10458, Bronx, NY
Correspondence to: Hoa Tran , Department of Computer Information Sciences, Fordham University, 10458, Bronx, NY.
Email: |  |
Copyright © 2012 Scientific & Academic Publishing. All Rights Reserved.
Abstract
The probability of ruin plays a crucial role in determining the Network Security Risk. The stable rate, initial stable values, together with ruin time, ruin function will be discussed in the directions of mapping to the Security Systems. As the stable rate becomes larger, the observation is the probability of ruin will be smaller. Random walk, Brownian motion and the connection with Network Security Risk also will be addressed. People can use the model to determine the implementation structure of the Network Security Systems to prevent intrusions.
Keywords:
Probability of Ruin, Time of Ruin, Ruin Function, Stable Rate, Random Walk, Brownian Motion
Cite this paper:
Hoa Tran , "Aspect in Network Security Risk: A System Ruin Problem", International Journal of Networks and Communications, Vol. 1 No. 1, 2011, pp. 23-25. doi: 10.5923/j.ijnc.20110101.05.
1. Introduction
Since Dassios[5], Harrison[7] and Paulsen[15], several models for Risk Analysis had been established, but there are no connections to the CAPM, Sharpe[16]. As was pointed out by Dassios[5], these processes should provide a standard theory for studying applications in insurance risk theory. The Poisson arrival condition will be relaxed to a general renewal arrival process, Karatzas[10]. The service payment model will follow the replacement model in Gihman[6]. The analysis of integral representation will be given by Moriconi[14], the properties of which were subsequently investigated by Lintner[11]. In this paper, relevant facts that affect the probability of ruin can be mapped to Network Security System so that people can determine the stability of Network Systems. The models for the corruption of an event can be viewed as the stage of Network Security Risk, using the parameters to determine its stability.
2. Stochastic Risk Model
Let
be a stochastic process with stationary, independent increments, finite variance, and
. We call this as gain process. Given a positive y of initial stable values and a positive stable rate
, we define the stable process by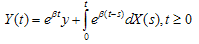 | (2.1) |
Let
be a Poisson process with arrival rate λ, and let
be independent and identically distributed random variables with distribution F. Let c be a finiteconstant, take
Let
Call T the time of ruin and r(.) the probability of ruin, then | (2.2) |
Assume that the process X is defined on a probability space and has stationary, independent increments with E[X(t)] =
and Var[X(t)]=
, where
and
.Let
, then
Theorem:We have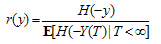 | (2.3) |
where H is the distribution function of
.The proof of the theorem had been done properly in Harrison[7].
3. Stable Rate Determines System Stability
As the stable rate
increases, the ruin function r(.) will get smaller. This fact can say a lot about the way to control stability of systems. The chance of being intruded will be smaller if the stable rate is raised or higher.For the characteristic function of X(t) we have
Where
From[6], the representation can be | (3.1) |
where G is a probability distribution in R.Suppose The exponential function is
then  | (3.2) |
Invert H then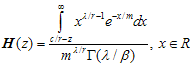 | (3.3) |
with is the Gamma function 

The probability of ruin is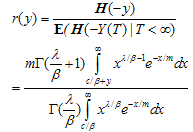 | (3.4) |
Suppose now that
(m > 0),with F (x) = 1 for x > 0. We obtain
Similarly, take the inversion, we have
4. Some Problems in Network Security Risk
From (2.1) and (2.2), we can remodel the parameters as components in Network such as traffic channels, systems, encryption processes. The probability of ruin in this environment will represent for the stability and risk of network security components.Theorem 4.1 Assume
= 0. Let
and consider the equation | (4.1) |
Where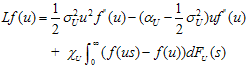 | (4.2) |
Let y (u) and z(u) be solutions of (4.1) with
and
respectively, and such that 0 < y (u), z (u) < 1. Assume:(B1) if
> 0, then
Otherwise it is sufficient that
(B2)
then
Proof. Let X (u) = exp{
}, then since k is real,
and
is the solution of (4.1) with
. For some constant c,
Since by
. Then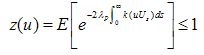
5. Conclusions
In this paper, ideas of mapping to Network Security Risk had been presented for the important applications of System Security Risk so that companies can use to evaluate the System Security stability. The applications of Stochastic Ruin Models will be useful for System Intrusions, to prevent attacks. By using appropriate parameters for the environment, the models for Network Security Risk play important role for Homeland Security, to make decisions for kinds of secure system implementations. Further investigations on the applications on the particular devices for Network Security systems will be studied in the future.
References
[1] | M. Avellaneda, A. Levy and A. Paras, “Pricing and Hedging Derivative Securities in markets with Uncertain Volatilities,” Appl. Math. Finance 2 (1995), 73-88 |
[2] | M. Avellaneda and A. Paras, “Dynamical Hedging Strategies for Derivatives Securities in the Presence for Large Transaction Costs,” Appl. Math. Finance 1, (1994), 165-193 |
[3] | P. Bickel and K. Doksum, Mathematical Statistics, vol. 1, 2nd edition. Prentice Hall, New Jersey, 2001 |
[4] | P. Billingsley, Probability and Measure, 3rd edition. Wiley, New York 1995 |
[5] | A. Dassios and P. Embrechts, “Martingales and Insurance Risk,” Comm. Statist. – Stochastic Model 5 (1989) 181-217 |
[6] | I. Gihman and A. Skorohod, Introduction to the Theory of Random Processes. Saunder, Philadelphia, PA, 1969 |
[7] | J. M. Harrison, “Ruin Problems with Compounding Assets,” Stochastic Process. Appl. 5 (1977) 67-79 |
[8] | J. Hull, Options, Futures and Other Derivatives, 5th edition. Prentice Hall, New Jersey, 2003 |
[9] | N. L. Jacobs and H. R. Petit, Investments. Irwin, Homewood, IL, 1984 |
[10] | I. Karatzas and S. E. Shreve, Brownian Motions and Stochastic Calculus. Springer, New York, 1988 |
[11] | J. Lintner, “The Valuation of Risk Assets and the Selection of Risky Investments in Stock Portfolios and Capital Budgets,” Review of Economics and Statistics 47, no. 1, (February 1965) 13-37 |
[12] | H. McKean, Stochastic Integrals. Academic Press, 1969 |
[13] | R. Merton, “Option Pricing when the underlying stock returns and are discontinous,” J. Financ. Econom. (1976), 125-144 |
[14] | F. Moriconi, “The submartingale assumption in risk theory,” Insur. Math. Econom. 5 (1986) 295-304 |
[15] | J. Paulsen, “Risk Theory in a stochastic economic environment,” Stochastic Process. Appl. 46 (1993), 327-363 |
[16] | W. Sharpe, “Capital Asset Prices: A Theory of Market Equilibrum under Conditions of Risk,” Journal of Finance 19, no. 3 (September 1964), 425-442 |