Faiq Mohammed Sarhan Al-Zwainy1, Neran Taher Hadhal2
1Department of Civil Engineering, Alnahrain University, Baghdad, Iraq
2Department of Civil Engineering, Baghdad University, Address, Baghdad, Iraq
Correspondence to: Faiq Mohammed Sarhan Al-Zwainy, Department of Civil Engineering, Alnahrain University, Baghdad, Iraq.
Email: |  |
Copyright © 2016 Scientific & Academic Publishing. All Rights Reserved.
This work is licensed under the Creative Commons Attribution International License (CC BY).
http://creativecommons.org/licenses/by/4.0/

Abstract
Due to lack of data and information in feasible study of the construction projects, therefore the predicating of the construction cost is very difficult. The objective if in this study is development a mathematical model for predicting the cost of the communication towers projects. Multifactor linear regression technique is developed and used for predication of the cost of communication towers projects in Iraq. Seven effectiveness factors are used for cost forecasting by MLR model, they involve Security Conditions, Tower Types, Experience of Contractor, Foundation Types, Tower High, Main Cable and Site Area. It was found that Multifactor linear regression has the flexibility to foretell the cost with a excellent degree of accuracy 90.1%, mean absolute percentage error 9.891% and coefficient of correlation (R) was 98.6%.
Keywords:
Communication Tower Projects, Cost Estimation, MLR Model, SPSS
Cite this paper: Faiq Mohammed Sarhan Al-Zwainy, Neran Taher Hadhal, Building a Mathematical Model for Predicting the Cost of the Communication Towers Projects Using Multifactor Linear Regression Technique, International Journal of Construction Engineering and Management , Vol. 5 No. 1, 2016, pp. 25-29. doi: 10.5923/j.ijcem.20160501.03.
1. Introduction
Cost prediction remains a difficult and complex problem, in spite that, the researchers were still studying and trying different approaches and methodologies to solve it. Preparation of a construction cost estimate for any project is a very complex process. Process of construction cost estimation contains many variable factors. Every variable has to be correctly estimated based on proper study, past experience and research to calculate total project cost of construction [1]. The researcher believed the Prediction of the cost estimation of communication towers projects is a vital mission in the management of Iraqi construction sector. The scope of this research is to build and evaluate an analytical model which may be used to estimate the budget of towers projects, by utilizing Multifactor Linear Regression Technique (MLRT), through the following steps:● Identification of MLR model variables, which have an effect on the cost of communication towers projects in Iraq.● Build an inclusive tool for parametric cost estimation utilizing the MLR as a favorable predicting model.● Develop a numerical equation to calculate the cost of communication towers projects.● Investigation the verification and validation of the mathematical model developed.● Determine the degree of accuracy for the numerical equation and clarifying the amount of the correlation between predication costs and actual cost.● Investigation and assess of the proposed Multifactor Linear Regression models.
2. Research Methodology
Research methodology has adopted to involve the following:● Literature Survey: is to review the cost estimation and application multifactor linear regression technique in communication project. The concept of the cost estimation and regression analysis, benefits, structures, and applications of regression analysis in project management field, Which include the review of literatures involving references, thesis, papers, books and web-site relating to the subject of research especially which are related to construction industry. ● Field Work: This involved data description and selection of the variables, then development of Multifactor Linear Regression (MLR) Model. And validity of the MLR Model.
3. Selection Variables
In this study, the researcher used quantitative and qualitative approaches to collection data, as shown following:1) The qualitative approach: in this approach, interviews and the questionnaire were used to investigation and determine the main factors affecting the cost of communication towers projects in Iraq. Most effective factors can be shown in Table 1. And, the detail of this approach was explained in manuscript (Al-Zwainy and Hadhal, 2015) [1].2) The quantitative approach: was used to gather the real data from resources (Asiacell and Zain companies) by filling a form for each communication towers project, which contains the input factors and the actual cost for each project as output. Multifactor Linear Regression model require a lot of historical data. All of this communication towers projects were done between 2013 and 2014 in Iraq. The projects information was gathered from direct watching and reports of contractors and companies. This method faces a great difficulty nowadays because of the unsecured status of the country (Iraq), and the shortage in documentation. In spite of these obstacles, the researcher succeeded in gathering well trusted data for more than 45 communication projects through the companies’ visits and reading the concerned sheets, contract documents and reports of projects.Table 1. Effective Factors the Cost of Communication Towers Projects in Iraq 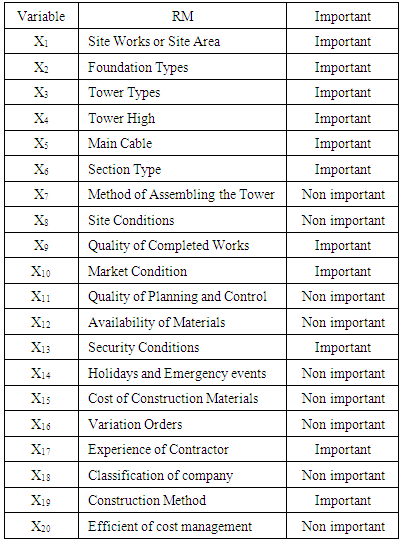 |
| |
|
These variables are represented fell into two classifications, subjective and objective variables. All variable can be analyzed so as to find the optimum approach to illustration for the variable within the modeling process, as shown in Table 2.Table 2. Classification of variables 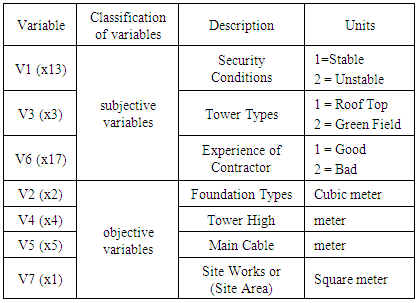 |
| |
|
Objective variables content four variables, and may be measured utilizing the unit of measurement such as High of Tower (V4) and Length of Main Cable (V5) were measured in length meter. While the second variable is Foundation Type (V2) measured in cubic meter, and the variable offsite Area (V7) can be measured by square meter.Subjective variables content three variables, and could be measured depend on the coding system, such as, security conditions (V1) will be classifies to security conditions and non-security conditions and specify them the value 1 and 2, respectively. Also the third variable Tower Type (V3) specifies the nature of tower as green filed or roof tower. It assigns them the values of 1 and 2 respectively. Latterly, the sixth variable Experience of Contractor (V6) defines an index which accounts for Experience of Contractor with two types good, and poor. It specifies them the values of 1 and 2 respectively.
4. Development of Multifactor Linear Regression (MLR) Model
A lot of many subscriptions and books described the multiple linear regressions technique. The researcher founded the text by (Al-Zwainy et al., 2013) [2] and (Smith, 1999) [3] could be particularly useful because it is simply and understand. In this study, multifactor linear regression can be used as an optimum forecasting model and very strong mathematical tool that allows the engineers and researchers to find the engineering relationship between dependent variables (e.g. actual cost) and independent variables (objective and subjective variables) (e.g. Foundation type, tower type, etc.) It can tentatively formulate (Johnson and Bhattacharyya, 2006) [4]: | (1) |
Where:● i = 1,……,n ● xi1 and xi2 are the values of the input variables for the i the experimental run● Yi is the corresponding response ● ei the error components are assumed to be independent normal variables with mean zero and variance σ2● β0, β1, ….,βp the regression parameters are unknown and so is σ2In the field of scientific research there is much statistical software. But in Iraq, the most common software is Statistical Package for the Social Sciences (SPSS). The developers of the (SPSS) made every effort to make the software easy to use. Also, the researcher believed that SPSS is one among the analytics software's are estimate with confidently what will happen next and will be create smarter choices, improve outcomes and solve complex problems. In this study, the researcher used SPSS Version. 24 to development of the multifactor linear regression model and statistical analysis results as shown in Table 3., Table 4., and Table 4.Questionnaire forms were completion and distribution, then analyzing those statistically according statistical lows. Summary of statistical analysis can be seen in Table 3.The quantity (R), called the linear coefficient of correlation, measures the direction and the strength of a linear relationship between two variables. And the determination coefficient (R2) represents the percent of the data that is the nearest to the line of best fit. Table 4 show results of the correlation test, where the value of coefficient of correlation equal to 99.2%. The positive value means the correlation between a dependent variable (actual cost) and an independent variable is very strong. Also, the Coefficient of determination equal to 98.4% means that 98.4% of the total variation in dependent variable (actual cost) (y) will be explained with the linear relationship between xi and y. The other 1.6% of total variation in y remains unexplained.Table 5. shows the regression variables, regression coefficients and statistical tests of the P-values of coefficients.Regression analysis generates an equation to explain the mathematic relationship between one or a lot of predictor variables and also the response variable. SPSS software labels the standardized regression coefficients as “Beta” and unstandardized coefficients are labeled “B”. Coefficient Standardization is sometimes done to answer the question of that of the independent variables have a greater impact on the dependent variable in a multiple correlation analysis, once the variables are measured in numerous units of measurement. Key of the coefficients understanding is slopes, and they are sometimes called slope coefficients. Can be concluded from this table, independent variables (foundation type, tower type, height of the tower, the main cable and site location ) were significant from a statistical standpoint, according to T-test (at the P-values P≤ 0.05). But the independent variables (security conditions and the Experience of the contractor) did not have a significant impact on the multiple regression models, according to T-test.The researcher believed a regression models as a powerful tool will be utilized to estimate the cost of communication tower project from multi variables, and it allowing to prediction the cost of projects in future depending on the information about past or present projects. Values of unstandardized coefficients in Table 5 can be used to build the multivariable linear regression, as shown the following eqution. | (2) |
Table 3. Descriptive Statistics 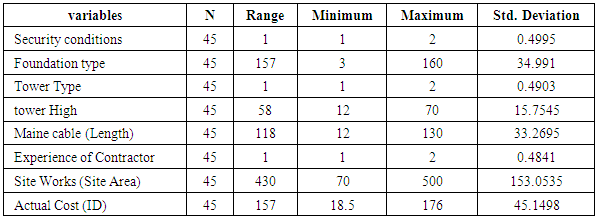 |
| |
|
Table 4. Results of the Correlation  |
| |
|
Table 5. Regression Coefficients Values 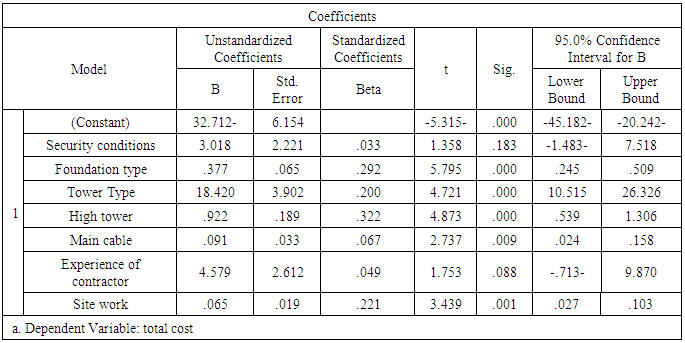 |
| |
|
5. Validity of the Multiple Linear Regression MLR Model
The purpose of the validation is to provide a statistically reliable model. In this study, twelve new documents for communication tower projects were gathering as shown in Table 6. These documents weren't included in model developed, but utilized for validation and verification of this model. Actual cost of communication tower projects and the predicted values (by suggested estimation equation) were presented in Table 7.Table 6. Information of the New Communication Tower Projects 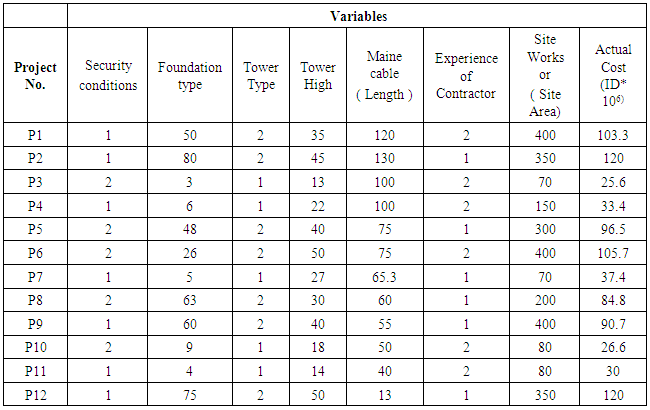 |
| |
|
Table 7. Actual Cost and the Predicted Cost 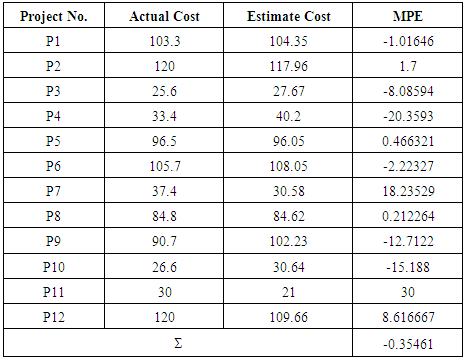 |
| |
|
In order to prove the validity of the model developed, the researcher used the coefficient of determination to explain the linear relationship between projected cost and actual cost, where the coefficient of determination equal to 97.32%, as shown in Figure 1 which means that model showing the excellent homogeneous with actual observations.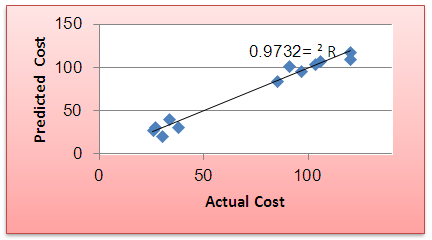 | Figure 1. Validation MLR Model |
The researcher used the same statistics measures adopted by (Al-Suhaily et al., 2015, Al-Zwainy, 2014, and Al-Zwainy et al., 2012) [5-7] to determine the average accuracy of the this model, as shown in the Table 8 below, where the Mean Absolute Percentage Error (MAPE) equal to 9.891% that mean the Average Accuracy (AA) of this model equal to 90.1% is excellent.Table 8. Statistical Measures Results for Regression model 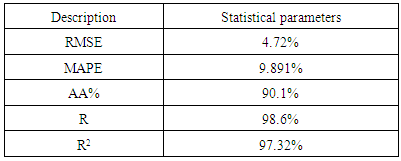 |
| |
|
6. Conclusions
Traditional of estimation methods suffer from the major disadvantages of the lack of precision and uncertainty, but a regression analysis offer several advantages over traditional methods for the prediction of the communication projects cost. The conclusions drawn from the above study were summarized below:● Multifactor linear regression has the ability to estimate the cost of communication towers Projects, one an optimum forecasting model Developed as a comprehensive tool for parametric cost estimation with high degree of accuracy with 90.1%.● Coefficient of determination using to explain the linear relationship between projected cost and actual cost with 97.32%● The developed model is useful for researchers and stakeholders because it provides easy tool to predict the cost of construction Projects.● The developed model on preparing the historical data equal to 45 past project as historical data to be modelled and determining the relation between output and input variables. Seven influencing factors are used for cost forecasting by Multifactor linear regression model, they include Security Conditions, Tower Types, Experience of Contractor, Foundation Types, Tower High, Main Cable and Site Area.
7. Proposals for Future Studies
The researcher here introduces a number of proposals to be investigated as future studies and as follows:● Repeating the same study by using neural network technique and making a comparison between the results to show which method is more adequate for use in construction management.● Developing several MLR models to demonstrate the ways in which different types of civil engineering problems ensure the successful development and application of this technology to project management problems.
References
[1] | Al-Zwainy, F. M. S. and Hadhal, N. T. (2015). “Investigation and Evaluation of the Cost Estimation Methods of Iraqi Communication Projects” International Journal of Engineering and Management Research, IJEMR, Vol. 5, No. 6, pp. 41-48.. |
[2] | Al-Zwainy, F. M. S., Abdulmajeed, M. H. and Aljumaily, H. (2013). “Using Multivariable Linear Regression Technique for Modeling Productivity Construction in Iraq” Open Journal of Civil Engineering, OJCE, Vol. 3, No. 3, pp. 127-135. doi:. |
[3] | Smith, S. D., (1999) “Earthmoving Productivity Using Linear Regression Techniques,” Journal of Construction Engineering and. Management, Vol. 125, No. 3, pp. 133-141. doi:10.1061/(ASCE)0733-9364(1999)125:3(133). |
[4] | Johnson, R. A., Bhattacharyya G. K., (2006). Statistics: Principiles and Methods, 5ed, John Wiley and Sons, Inc., USA. |
[5] | Al-Suhaily, R. H., Saco, Z. M., Alzwainy, F. M. S., (2015). Project Management and Artificial Neural Networks: Fundamental and Application, LAP LAMBERT Academic Publishing, Germany. |
[6] | Al-Zwainy, F. M. S. (2014). “Development of the Mathematical Model for Predicating the Construction Productivity in Iraq Using the Artificial Neural Perceptron Network” Journal of Engineering and Development, Vol. 18, No. 2, pp. 1-21. |
[7] | Al-Zwainy, F. M. S., Hatem, A. R. and Huda, F. I., (2012). “Using Artificial Neural Network for Finishing Works for Floors with Marble,” ARPN Journal of Engineering and Applied Sciences, Asian Research Publishing Network (ARPN) Vol. 7, No. 6, 2012, pp. 714-722. http://www.arpnjournals.com/jeas/research_papers/rp_2012/jeas_0612_714.pdf. |