Fred Nimoh 1, Enoch Kwame Tham-Agyekum 2, Samuel Ayisu 1
1Department of Agricultural Economics, Agribusiness and Extension, KNUST-Kumasi
2Department of Agricultural Extension, University of Ghana, Legon-Accra
Correspondence to: Enoch Kwame Tham-Agyekum , Department of Agricultural Extension, University of Ghana, Legon-Accra.
Email: |  |
Copyright © 2012 Scientific & Academic Publishing. All Rights Reserved.
Abstract
In order to empirically assess the factors causing the default of credit repayment among maize farmers, a study of this nature becomes imperative. The Asante Akim North District of the Ashanti Region was selected because, maize farmers in the District had benefitted from the Special Maize Project (SMP). The target population was all 400 maize farmers. A total sample size of 60 maize farmers was selected using the systematic sampling for every 5th person from the sampling frame. Frequencies, percentages and the probit model were used to analyse the data. Results of the probit model revealed that age, household size, rent, status of farmer and output significantly influence credit default. About 87% of the farmers consider the SMP idea as a good step by MOFA. The study recommends that farmers from different areas should also be included in the project.
Keywords:
Ashanti Region, Credit, Default, Factors, Maize Farmers
1. Introduction
To attain agricultural development, every government must consider agricultural credit as an important policy. The basic principle of reform in agricultural finance is to maintain and repair instead of to destroy or take apart the whole system. Agricultural finance is therefore vital to the achievement of agricultural policy objectives, and should not be regarded and treated as a general finance system. There is a general acceptance of the important role of farm credit and a wide appreciation by most government of the need for credit.The price of maize has not been stable for the past four years in the country. In 2003-2005, prices rose due to the high demand and therefore the shortage of the commodity in the country. This could be attributed to the immense drought that was experienced during the year 2005 (Cudjoe, 2007). As a result of the high demand and therefore increasing price of maize, the government had to import maize for local consumption. It was then realised by the Ministry of Food and Agriculture to rather encourage farmers to produce maize locally by funding the farmers with the money used for the importation. Therefore the need to intervene in the production of maize at the district levels was made by introducing the Ministry of Food and Agriculture (MOFA) Special Maize Project (SMP). In commencing the SMP in the year 2005, four hundred (400) farmers were therefore given credit in the form of inputs amounting GH¢76.3 per farmer to help them in the initial production of maize (Obaatanpa) for the minor rain season. A top-up money was added to the input credit to round the total credit to one hundred Ghana Cedis (GH¢100).For the past three years the SMP has been going on in the Asante Akim North District and other districts in the country. The project is now in its third phase with the hope that the beneficiaries (maize farmers) will improve in their credit repayment. Evidence from the SMP office indicates that many of the farmers are defaulting. Out of the total GH¢40,000 given to the 400 farmers, only GH¢4,287 (10.7%) has been paid as of 26/07/07 with an outstanding GH¢35,713 left unpaid. The payment of credit given to farmers by governments usually receives less attention than it deserves. A credit is said to be defaulted if any of the terms of repayment is not met. Default in its simple sense is non-adherence to agreed terms. With the high default rate (89.3%), it is therefore envisaged that the project which is helping the maize farmers and the country at large might not be sustainable in the future as the money is not recovered for it to be recycled for the benefits of other farmers. Credit to the maize farmers has not worked smoothly owing to loan delinquency which is now a serious problem to the Ministry. This farmer-credit situation can be hypothesized as one of the reasons for the high rate of default among the maize farmers and other food crop farmers. However, in order to empirically assess the factors causing the default among the maize farmers, a study of this nature becomes imperative.
2. Research Questions
In view of the above issues, the general research question raised in this study is; what are the contributing factors to credit default among the maize farmers?The specific questions raised are:1. Are the socio-economic characteristics of the maize farmers contributing to the credit default?2. What are the reasons for credit default?3. What are the farmers’ opinions of farmers about the SMP?4. What are the other sources of credit available to the maize farmers? The study therefore seeks to find out the factors that influence credit default and then make policy recommendations based on the results to help streamline the operations of similar government projects.
3. Research Methodology
The Asante Akim North District of the Ashanti Region was selected as the study area for this study. This was because, maize farmers in the District had benefitted from the Special Maize Project (SMP). The target population was all maize farmers who were beneficiaries of the SMP in the Asante Akim North District for the year 2005. The 400 farmer beneficiaries were the population for the study. The 400 beneficiaries were stratified into males and females from the sampling frame (i.e. the list of all the farmers and their location). Fifteen percent of each group was chosen from the total percentage of each group. Systematic sampling was used by selecting every 5th person in male group and every 5th person in the female group from the sampling frame. A total sample size of 60 maize farmers comprising 38 male farmers and 22 female farmers from the population of 254 males and 146 females was used. Primary data was collected by the use of structured questionnaires and interviews. Secondary data was also collected from published and unpublished data from MOFA, Konongo. Descriptive statistics such as frequency table and percentages was used to analyse the data collected to achieve research questions one, three and four. The Probit model was used to achieve research question two by determining and analysing the factors influencing credit default among the maize farmers in the Asante Akim North District.Because of the dichotomous nature of the dependent variable, normal regression model cannot be used to estimate the unknown parameters of the factors influencing credit default. The Probit model is the most appropriate model used. The general Probit model is expressed as: Yi = C + βiXi+eiIt assumes that βiXi is a normally distributed random variable and the estimated values of the dependent variables are converted into probabilities (for any given value of Xi) with the use of the cumulative normal distribution.Where Yi, is the dichotomous dependent variable which can be explained as;Y = 1, if farmer default. Y = 0, if farmer does not default. C = is the intercept.βi = the regression coefficients that explain the probability of default by maize farmers.Xi = independent variables (i = 1, 2, 3…10). ei = the error term (Such as weather, management, personal health, etc)For this study, the Probit model is specified as followed:Yi = C+ β1STAT + β2AGE + β3OCCUF + β4SEX+ β5MSTAT + β6OUTPUT + β7LANT + β8HHSZ + β9EDUL + β10LAB + eiThe a-prior expectations are:β1 > 0, β2 < 0, β3 < 0, β4 > 0, β5 < 0, β6 < 0, β7 > 0, β8 > 0, β9 < 0, β10 > 0.Definition of the variables used in the study is shown in Table 1.Table 1. Definition of Dummy Variables 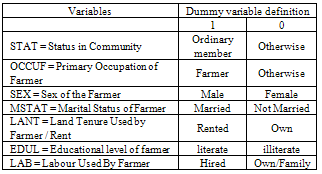 |
| |
|
Definition of Other VariablesAGE = Age of Farmer in yearsOUTPUT = Output - number of bagsHHSZ = Household SizeDefaultDefault is the dependent variable that represents a farmer’s credit repayment status. It is a dichotomous dependent variable which can be explained as 1, if farmer default, and 0, if farmer does not default.Status of FarmerStatus is the status of the farmer in the community. It looks at if the farmer holds any special position in the community. An ordinary farmer is explained as 1 and any farmer with any special position (otherwise) is explained as 0. It has a positive a-priori expectation as default is expected to increase with a farmer being ordinary.Age of FarmerThis is the age of the farmer in years. It is a quantified variable and gives the actual ages of the farmers that made up the sample. It has a negative a-priori expectation as years come with experience in farming and therefore decrease in default.Primary Occupation of FarmerThis is the primary occupation of the farmer. It represents occupation of the farmer with respect to the farmer being a full time farmer. Farmers whose only work is farming are considered here. The a-priori expectation is negative since farmers who live and work as only farmers should be well vest in the farming and therefore be more experienced to be less likely default.Sex of FarmerThis is the sex of the farmers. The dummy variable male is explained as 1being yes and 0 being not a male but female. It has a positive a-priori expectation. Males are more likely to default than females and therefore more males suggest more default rate.Marital Status of Farmer This is the marital status of a particular farmer in the community. It looks at a farmer being married or not. With marital status, 1is explained as yes and 0 as not married. It has a negative a-priori expectation which implies that a married farmer is less likely to default as he or she will have supporting spouse and children to help finance and farm work.Output of Farmers from HarvestThis is the number of bags of maize the farmer got from the harvest from the maize project. It is a quantified variable with one bag representing 100kg of maize. It has a negative a-priori expectation which suggest that a farmer who produces more is less likely not to default. Land Tenure System Used By FarmerThis is whether a farmer rented the land on which he or she farmed on. Some of the farmers farmed on the government reserved land. The dummy was 1 for persons on the reserved land and 0 for otherwise. An a-priori expectation of negative was assigned to it since it did not cost the farmers to farm on it. Extra money which could have been used to rent land was saved and therefore should reduce default. Household Size of FarmerThis is the number of dependant a particular farmer has. It includes the spouse, children and other people who eat from the house. It has been quantified and represents the number of people in a particular household. It has a positive a-priori expectation as more dependents will increase default rate by a particular farmer.Educational level of Farmer This is the number of years the farmer has spent in school. Schooling up to the primary level is considered as illiterate and those from secondary level to the tertiary level are considered as literate. It has been quantified and a negative a-priori expectation is expected as more dependents will increase default rate by a particular farmer. Labour Used by FarmerThis is the labor the farmer used for the production season. Labor is considered whether hired or not. Farmers who do not hired labor used family labor. An a-priori expectation of positive was given since increased use of labor would likely increase cost and therefore increase the likelihood of default.
4. Findings
Demographic Characteristics of RespondentsThe demographic characteristics of the respondents were examined. It was realised from the study that the mean age of the males was 48.66 years whiles that of the females was 44.64 years. This means that the average age of the sampled males was higher than that of the females. The males also had a larger mean household size than their female counterparts. Both males and females spent the same used the same number of kilometers to their farms and finally the output produced by the males in maize production was greater than the females.Table 2. Demographic characteristics of respondents 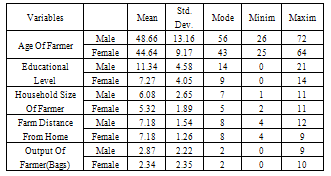 |
| |
|
Analysis of the Factors that Influence Credit DefaultThe Probit model was employed to empirically analyze the cross-section of farmers’ data to determine the factors influencing credit default among the maize farmers in the study area. The Probit model seeks to explain the probability of credit default as a result of any of the ten identified independent variables. The signs of the coefficients of independent variables and their significance were used to determine largely the impact of each variable on probability of credit default among the maize farmers. Of the sample size of 60 farmers, 68.3% were defaulters and 31.7% were non-defaulters. Among the socio-economic factors of the farmers, age of farmer, household size, output, rent system used by farmer and status of farmer were found to significantly influence credit default.The coefficients of age of the maize farmers and output (bags of maize obtained from harvest) are negative (indirect relationship) and significant at 1%. This indicates that there is an indirect relationship between age and output with credit default. An increase in the age (which may relate to experience of farmer), or output will decrease the probability of the farmers defaulting in credit repayment. The implication is that, young farmers are highly probable to default in credit repayment than the aged. Farmers with lower yields or outputs are likely to default in credit repayment than those who obtain higher outputs (measured in yields). This is consistent with Oni et al., (2005), who found age and output (measured in income) to significantly influence default.The coefficient of household size is positive and it is significant at 10%. This indicates that increase in the farmer’s household size will increase the probability of defaulting in credit repayment. It implies that farmers with large household sizes are likely to default in credit repayment than those with smaller household sizes. Oni et al., (2005) however, did not find any relationship between household size and default as it was not significant. The coefficients of land tenure/rent and status of the farmer in the community are positive (direct relationship) and significant at 5%. This indicates that the farmer’s use of free government reserved land or status of the farmer in the community not being ordinary will reduce the probability of defaulting in credit repayment. The implication is that farmers who do not use free lands are likely to default in credit repayment than those who use free lands. Maize farmers with a high status in society are less likely to default in credit repayment than those with ordinary status in society.Table 3. Empirical Estimates of Determinants of Credit Default 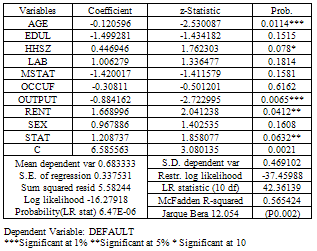 |
| |
|
The McFadden R-squared of 0.57 implies that the explanatory variables account for 56.5% of the variation in credit default. The normality test showed that the Jarque Bera value of 12.054 was significant at 1% we therefore reject the null hypothesis of normally distributed residuals. Rejection of the null hypothesis is due to the fact that the normality test is a non constructive test and that there are outliers in the data.Reasons for Credit DefaultTable 4. Reasons for Credit Defaults 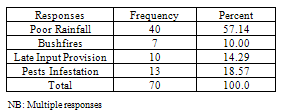 |
| |
|
Apart from the socio-economic factors analyzed to influence credit default, other factors perceived by the farmers as influencing default were also examined. Farmers were asked to list reasons for credit default, and the main reasons provided were: poor rainfall –draught (57%), bushfires (10%), late supply of input (14%) and pests’ infestation (19%). The most prominent reasons for default were poor rainfall and pests’ infestation.Farmers Opinion about SMP and Availability of Other Types of CreditIt can be observed that about 87% of the farmers consider the SMP idea as a good step in the right direction by MOFA. About 7% of the farmers however consider the whole idea as a bad initiative. The maize farmers consider the terms and conditions as problematic. The farmers had hoped for better farming conditions and harvest since they were on the government reserved land, but to their misfortunes, the area proved to be disastrous. There were a lot of fire outbreaks and pests infestations. Secondly, the top up monies given to them was insufficient as labour took a chunk of it. They also complained of the fact that they had to pay for the transportation fee for the input delivery. Also, the farmers complained of very few extension agents. There were only nine (9) extension agents as of 2007 and this even will not be enough to assist the 400 farmers. Finally, the whole system of paying the credit (in cash) at the bank was a very complex procedure for them. Most of the farmers were not highly educated and seeing money and sending it to the bank with all of the formalities was a waste of time and posed difficulties to them.Table 5. Farmers Opinion about SMP and Credit Default 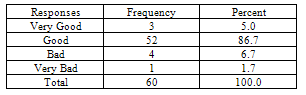 |
| |
|
Other Types of Credit Available to the Maize FarmersSome of the farmers who benefited from the 2005 SMP had other sources of credit. About 93.3% had no other source of credit apart from the credit from the SMP whiles about 7% sourced credit from other sources like relatives, friends, friends, money lenders and banks. All the farmers indicated that, the credit was not enough for the farming activities.Table 6. Other Sources of Credit 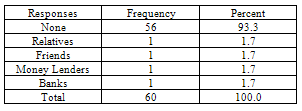 |
| |
|
5. Conclusions and Recommendations
Results of the Probit model revealed that five explanatory variables were significant while the other five variables were insignificant. The significant variables include age, household size, rent, status of farmer and output. The insignificant variables were educational level, labour used, sex, primary occupation of farmer and marital status. The autonomous level of loan default is 6.585563. All of the farmers complained of late input provision which extended the farming activities into the late season. Poor rainfall, pests’ infestation and bushfires were therefore a resulting effect of the late arrival of the credit package.The terms and conditions of MOFA offered to the farmer should be reviewed and made much more flexible to suit the farmers.The age of the farmer, household size and rental arrangement should be considered when providing farmers with credit facilities. Priority should be given to older farmers with small household size. Farmers who also own their land or have free access to land should also be given consideration. Farmers who also have prominent status in the community should also be considered as they will have access to basic resources like land, credit, and labour to help improve credit repayment. MOFA and other stakeholders in Agriculture should also ensure that the farmers have access to the inputs as early as possible since findings from the study has shown that the late arrival of inputs pushed the farming activities into the late season which caused the additional reasons which the farmers give as reasons for default in the credit repayment. Since the main aim of the project was to solve the maize shortages problem in the country due to draught and high demand, farmers from different areas should also be included to the project to ensure that a single misfortune will not affect all of them. For this, it has been corrected by MOFA and new areas are now part of the ongoing project but a more still wider coverage will be necessary.
References
[1] | Codjoe, N. A. S. (2007). Supply and utilization of food crops in Ghana, 1960-2000 |
[2] | Oni, O. A., Oladele, O. I., and Oyewole, I. K. (2005). Analysis of Factors Influencing Loan Default among Poultry Farmers in Ogun State, Nigeria, Department of Agricultural Economics, University of Ibadan, Nigeria |
[3] | Alamgir, M. and Arora, P. (1991). Providing Food Security For All. (IFAD Studies in Rural Poverty No. 1) Intermediate Technology Publishers. Pp. 73-74 |
[4] | Bliss, C. I. (1934a).The Method of Probits. Science 79, 38-39 |
[5] | Bliss, C. I. (1934b). The Method of Probits. Science 79, 409-410 |
[6] | Cuony, H. N. (2007). Determinants of Credit Participation and Its Impact on Household Consumption: Evidence from Rural Vietnam |
[7] | FAO. (1994). "Rural Poverty in the 1990s," in Rural Development Special No. 15. Ghana Universities Press, Accra, Ghana |
[8] | Ghate, P. B. (1992). “Interaction Between the Formal and Informal Financial Sectors: The Asian Experience”, World Development, No. 1., XIV |
[9] | ISSER. (2006). Review of Performance of Ghanaian Economy in First Half of 2006, Institute of Statistical, Social and Economic Research, University of Ghana |
[10] | Khalid, M. (2003). Access to Formal and Quasi-Formal Credit by Smallholder Farmers and Artisanal Fishermen: A Case of Zanzibar. Research Report No. 03.6 |
[11] | Kudiabor, C. D. K. (1974). "Policy Objectives and Strategies for Integrated Rural Development in Ghana," in Rural Development in Ghana. Ghana Universities Press, Accra, Ghana, Pp.26-32 |
[12] | Kwarteng, J. A and Towler, M. J. (1994). Agricultural Credit – West African Agriculture. Macmillan Publishers |
[13] | MOFA. (2001). Agriculture in Ghana. Facts and Figures. Statistics, Research and Information Directorate (SRID), Ministry of Food and Agriculture, Ghana |
[14] | Ogunfiditimi, T. O. (1981). Adoption of improved farm practices. A choice under uncertainty. Indian Journal of Extension Education Vol. XVIII, Nos.1 & 2 |
[15] | Zeller, M. (1994). “Determinants of Credit Rationing: A Study of Informal Lenders and Formal Credit Groups in Madagascar”, World Development, Vol. 22 No. 12, pp. 1895 – 1907 |