Vladimir Riecicky 1, Andreas Spichiger 2
1Corporate Development, K-at-R Management Consulting GmbH, Bern, 3013, Switzerland
2Business School, Bern University of Applied Sciences, Bern, 3014, Switzerland
Correspondence to: Vladimir Riecicky , Corporate Development, K-at-R Management Consulting GmbH, Bern, 3013, Switzerland.
Email: |  |
Copyright © 2012 Scientific & Academic Publishing. All Rights Reserved.
Abstract
Knowledge, once recognized as a dominating driver of competitive advantage, needs major management attention. Given the intangible flavor of the asset, a well-defined management approach is key to sustainable results at low cost. This paper introduces a knowledge asset risk management framework designed to serve this purpose. The framework includes guidance for a streamlined process based on a comprehensive conceptual foundation and special visualization and data validation means. The visualization tools make the data analysis approachable to a broad audience and serve as the common language along the knowledge risk management. The research is based on several implementation cases presented within this paper.
Keywords:
Knowledge Risk Management, Knowledge Measurement, Knowledge Asset, Tacit Knowledge
Cite this paper:
Vladimir Riecicky , Andreas Spichiger , "Knowledge Asset Risk Management Framework", Human Resource Management Research, Vol. 2 No. 4, 2012, pp. 38-45. doi: 10.5923/j.hrmr.20120204.01.
1. Introduction
The supply side of the business today is characterized by a strongly competitive environment. Due to the globalization trends, every player has an access to nearly any production resource. The winners turn the production resources into the smartest products and services. They utilize one special production resource especially well – the knowledge. Knowledge acquisition and retention turns out to be the dominating driver of the competitive advantage today. Consequently, knowledge drain becomes ever more one of the top-risks in the executive agenda.The general objective of this paper is to provide a risk-driven approach to the management of knowledge assets. The specific objectives include the respective conceptual foundation and the related management process. The main questions addressed in this paper are:▪ Which process shall a knowledge management follow?▪ What are the main elements for the measuring knowledge assets?▪ How can bias in the measurement be identified/ maybe eliminated or reduced during the whole process?▪ What are relevant visualizations of the assessment that support the stakeholders in all phases of the process?▪ What are examples of good implementations of the presented framework?This paper builds on the concept introduced in[1]. This case study provides an overview and genesis of the approach. However, it focuses only on the implementation case, rather than providing deeper insights into the framework itself. In the next sections, the framework is explained in detail. First, the process of knowledge risk management is explained, followed by the conceptual foundation of the framework. Finally, several implementation cases are described, followed by future research opportunities.
2. Process
The process presented next is designed to guide a proper use of the framework. It consists of two building blocks: initiation followed by a continuous risk management cycle.
2.1. Initiation
This phase declares the overall goal of the initiative and the respective tailoring of the framework. Different goals for managing the knowledge assets influence the granularity and the relevant knowledge in the scope. A few examples follow.The goal of reducing the headcount of contractors working for the company limits the scope of the analysis to a small number of people. The aim is to assess the knowledge assets that must not be lost due the outplacement of the contractors including the investment required to avoid this knowledge drain.A reorganization of a service support function along ITIL within a company focuses on the special set of knowledge assets required within the new organization. The intended staffing of the service support function will delimit the knowledge of the new organization. Consequently, the aim is to identify the knowledge to be transferred including the expected cost. Based on that, the risk and the cost of the intended change can be considered and appropriate actions taken. The situation is a very different in the case a company wants to expand into a new region. First, the knowledge needed to run the operations in the new region has to be identified. The aim is to estimate the cost of training the work force available in the labor market of the region. The estimates will be used to (re-)assess the decision on expansion in the sense of the cost-benefit analysis.
2.2. Risk Management Cycle
The next step after the initiation is launching a continuous risk management cycle. This management cycle is designed according to the Deming cycle[2], later referred as the PDCA cycle. The PDCA cycle is entered at the check step.The aim of the Check step is the setup of the knowledge map focused on the goals identified within the initiation. Along with this, the relevant knowledge is scoped and the relevant parties are defined. The Check step is strongly limited to the measurement of the knowledge assets using the knowledge map from the previous step.The Act step is very important for the success of the management action. Stakeholder-oriented visualizations of the knowledge assets give a strong support for the identification and implementation of the concrete measures to deal with the actual knowledge risks. In fact, the framework follows a risk-driven approach to knowledge management ([3] to[12]). The visualization focuses on the relevant aspects, depending on the goals of the initiative.Next, the result is translated into concrete actions that are allocated to the respective resources and deadlines. This is done within the Plan step, ending up with an action plan which will be finally executed as part of the Do step. After the execution is finished, the cycle continues with the Check step, by updating the knowledge map.
3. Conceptual Foundation
This chapter provides a conceptual overview of the framework. The respective model is designed using the Unified Modeling LanguageTM[13]. The abstraction level used discloses only the key framework concepts. Each of the concepts is named, and its semantics briefly described including the relationships to the other concepts. Figure 1 provides an overview.Knowledge Store represents a human resource, who is supposed to possess (store) some fraction of the business relevant knowledge. Each Knowledge Store is assigned a Risk Class. This property reflects the probability of losing the person as an employee, taking into account all the possible drivers, such as the labor market, or simply the age.Knowledge Unit represents a type of knowledge, as opposed to a knowledge asset, represented by a Knowledge Record. Note that knowledge turns into an asset only when recorded within a human brain. Only then it can be translated into tangible actions yielding a business value. Each Knowledge Unit is assigned a Target Profile, which describes a skill set taken into account when estimating the Replacement Effort. A Target Profile can have two alternative meanings. It either reflects a supply-perspective or a demand-perspective. In the supply-perspective a Target Profile specifies the skill set currently available in the labor market. In the demand-perspective the skill set matches the optimal skill set independently from the labor market supply. Note that both of the perspectives follow completely different goals. The quantification under a supply- perspective is the right way to estimate the risk taken by a company due to possible employee turnover. The quantification under a demand-perspective is the right choice in the following business scenario. There is decision to take upon the expansion into a new geographical region. Given the cost of the expansion attributable to knowledge acquisition (equals the knowledge quantification result) is fixed, the labor market must necessarily offer the skill set matching the Target Profile. In case the labor market does not fit the criteria, the expansion needs to be re-assessed.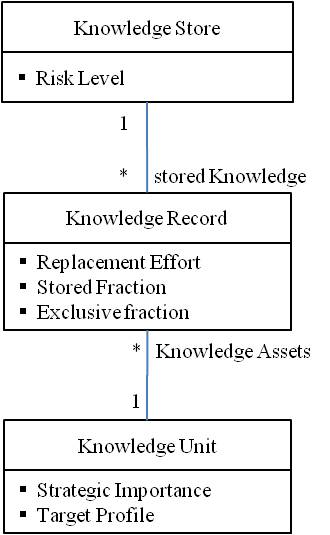 | Figure 1. Conceptual foundation |
The Strategic Importance attribute classifies the knowledge according to its contribution to the company’s competitive advantage.The knowledge possessed by a Knowledge Store is called Knowledge Record. A given Knowledge Store can store many Knowledge Records, whereas a given Knowledge Record is always associated with a single Knowledge Store. The knowledge in scope of a given Knowledge Record is modeled by an association to a Knowledge Unit. The attributes of a Knowledge Record reflect the size and the shape of the respective Knowledge Asset. Thus, Stored Fraction equals the fraction of the Knowledge Unit possessed by the Knowledge Store. Exclusive Fraction equals the fraction of the Knowledge Unit possessed exclusively by the Knowledge Store. Note that Exclusive Fraction is by definition always smaller than Stored Fraction. Replacement Effort equals the total effort needed to pass the Stored Fraction of knowledge to a person of skill set matching the Target Profile of the associated Knowledge Unit. This quantification concept is the core principle of the framework. At the same time, it is the key answer to measurement of tacit knowledge ([14] to[16]), which makes even this kind of knowledge a tangible asset.
4. Knowledge Asset Quantification
Recalling[1], the real challenge is getting the replacement effort estimates right. That means estimates without bias caused through gaming (cheating the system) or plain misunderstandings along the estimation process. The other possible source of an estimation bias is a subjective perspective taken: not every employee shares the same level of willingness and/or ability to pass his/her knowledge on somebody else. The method presented next is the way to keep the bias limited at low cost.The method is designed around a voting principle. The knowledge bearers are separately asked two questions concerning the given knowledge unit:▪ How much of the knowledge (expressed as a fraction of the knowledge unit) do you possess?▪ How much effort do you need to pass your knowledge to a person matching the given target profile?Once the data is collected, the voting can be evaluated. A possible example of such a voting follows. Three knowledge stores (Bob, Patty, and Kathie) are interviewed separately concerning the knowledge units “Surfing basics” and “The right surf board”. The answers are given in Table 1.Table 1. Voting on Marginal Effort |
| | | Surfing Basics | The right surf board | Bob | Stored Fraction | 20 | 100 | Replacement Effort | 40 | 150 | Marginal Effort | 2.00 | 1.50 | Patty | Stored Fraction | 80 | 40 | Replacement Effort | 80 | 80 | Marginal Effort | 1.00 | 2.00 | Kathie | Stored Fraction | 50 | 0 | Replacement Effort | 300 | | Marginal Effort | 6.00 | | | | | | Average Marginal Effort | 3.00 | 1.75 | Standard Deviation | 2.65 | 0.35 |
|
|
As can be seen, the data collected was enriched by several calculations (in italics):▪ Marginal Effort equals the effort needed to pass one percent of the given knowledge unit (Replacement Effort divided by Stored Fraction) to a person matching the Target Profile.▪ Average Marginal Effort equals arithmetic average over all calculated Marginal Efforts.▪ Standard Deviation equals standard deviation of the Marginal Effort perceived as a random variable. Now, we are ready to interpret the results. The data gives us two insights. Firstly, as the calculated Average Marginal Effort shows, “Surfing Basics” is nearly twice as big as “The right surf board” in the sense of the replacement effort. Secondly, the quantification of “Surfing Basics” is far less reliable than the one of “The right surf board”, due to the calculated Standard Deviation and its statistical significance. The second insight uncovers an estimation bias that needs to be sorted out prior to further data analysis. This is how the method serves its purpose of bias identification. The real power of the method is the fact that the bias can be identified at very low cost: a single interview with each of the knowledge bearers followed by (fully automated) statistics.Gaming of the system becomes nearly impossible, since the employees are interviewed separately, not knowing each other’s response. Of course, one needs to be aware of the prisoner’s dilemma at this point, which is a natural effect to be expected according the model of economic view of the behavior[17].There is also an additional source of bias that might be disclosed through the voting method – the knowledge unit inventory itself. The knowledge unit inventory might be a source of undesired bias within the knowledge asset quantification process. Indeed, the architecture of the inventory has a huge influence over the quantification. The obvious challenge is scoping the inventory items in a way that serves the analysis the best. A detailed discussion on this topic goes beyond the purpose of this paper, but let us put the essence into simple words: fine grained inventory is the dream of an analyst, but a nightmare of a CFO at the same time. The maintenance of a fine grained inventory will require too much effort compared to the benefits. On the other hand a coarse grained inventory makes it really hard to analyze the resulting knowledge map. You end up with knowledge units:▪ so big, that literally everybody possesses some fraction of each of them, which makes it an impossible task to uncover knowledge gaps. ▪ that are difficult to classify. All of them are in some way of important, but it depends a lot on the person asked. ▪ that overlap too much, which makes your quantification useless (the algebra of the framework does not hold under such circumstances).Let’s revisit classification of the knowledge units within the inventory. The main question is: How to avoid a bias coming from subjective opinions of the executives? Or, in other words: How to link the classification to the company’s strategy in a transparent and consistent way?The answer provided at this point comes out of a practical implementation case from a software development department. The department provides IT services well defined in form of service level agreements. The key insight was the relation between the classification of the IT services according to the service level agreements and the classification of the knowledge units: the knowledge is a core asset needed to fulfill the service level agreements. Consequently, exactly the same classification scheme as the one for IT services was used and so the problem reduced to linking knowledge units to the respective IT services.The former paper[1] introduced the notion of knowledge redundancy level, however it failed to provide comprehensive guidance on how to derive this variable. The challenge is again gaining reliable (unbiased) data at low cost in a simple and achievable way. Well, the answer is surprisingly easy, once the question is translated to mathematical terms. Let’s derive the answer in several steps. Let’s also assume there is no bias due to the knowledge unit inventory itself.Recalling the definition of knowledge redundancy level[1], it equals the fraction of the given knowledge unit that is being possessed by at least two employees. Now, how to utilize voting in order to estimate the desired fraction? Should each of the employees make a bet on the fraction taking the average of the bets at end as a result? No, we can do much better.The answer is based on the basics of the set theory. Given three knowledge bearers for a particular knowledge unit, the knowledge possessed by the respective knowledge bearer can be expressed as a set. That means there are three sets that eventually overlap in some way. This representation is a correct algebraic way of representing the subject (the proof is beyond the scope of this paper), which makes it easy to explain the method of calculating the knowledge redundancy level.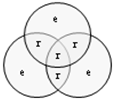 | Figure 2. Knowledge redundancy level |
Looking at the sets (Figure 2), it becomes clear that the areas tagged by “r” represent the redundant knowledge according to the definition. Consequently, the areas tagged by “e” represent the complementing fraction of the knowledge – knowledge that is being exclusively possessed by exactly one knowledge bearer. Thus, since we translated the problem into set theory, we are allowed to calculate the redundant fraction of the knowledge applying set algebra. Clearly, the knowledge redundancy level equals the difference between 100% and the sum of areas tagged by “e”!How does that translate back to the voting method? Instead of asking the employees which portion of knowledge - they think - is possessed by at least two employees, we ask a much simpler question: What is the fraction of the knowledge unit, nobody else but you possesses? In fact, as the experience shows, this question is far easier to answer.There is a straightforward way to identify bias, also coming along with set theory. The sum of exclusive fractions must not be bigger than 100%. Given it is bigger than 100%, further justification needs to be done prior to analysis. The example below demonstrates the situation pointing to a possible bias concerning the knowledge unit “The right surf board”. Table 2. Voting on Redundancy Level |
| | | Surfing Basics | The right surf board | Bob | Exclusive Fraction | 10 | 80 | Patty | Exclusive Fraction | 30 | 30 | Kathie | Exclusive Fraction | 20 | | | | | | Total Exclusive Fraction | 50 | 110 |
|
|
According to Table 2 Bob and Patty probably overestimate their fraction of exclusively possessed knowledge, since the sum of the Exclusive Fractions is bigger than 100%, which is clearly not possible.
5. Why Visualizations?
For the sake of relevant changes of the initiative, it is worthwhile to identify good visualizations of the risks concerning the knowledge assets. The visualizations support the whole PDCA cycle, improving the level of common understanding among the stakeholders involved. The stakeholders can make direct links to their own contexts, and the incidences of the past are easily to identify in the visualizations. The visualization for a certain stakeholder considers his/her authority and competences for implementing further measures.For the persons concerned, the presentation has to be authentic, representing the knowledge of those persons concerned in an adequate way. The visualization of the past is comparable with their perception of the changes in reality. The visualization of the changes must also be appropriate compared to the visualization of the current state.For the principal and the agent, a good representation of the current and the future situation must be found.The manager of a knowledge management project is mostly interested in the visualization of the measures implemented and their impacts on the current situation. For future measures, the project manager’s interest is in visualizing the risks addressed by the measures and what their impacts will be in terms of the future shape of the knowledge assets.Good visualizations support the change process. A certain presentation can be suitable for a specific step during the change process, but not for the other steps. All stakeholders should be open to identify adequate visualizations required during the change.As the framework is supposed to be implemented as an ongoing process, one single visualization common to all stakeholders is a must, in order to implement the change successfully. This common visualization is introduced next.
6. Coral Reef Visualization
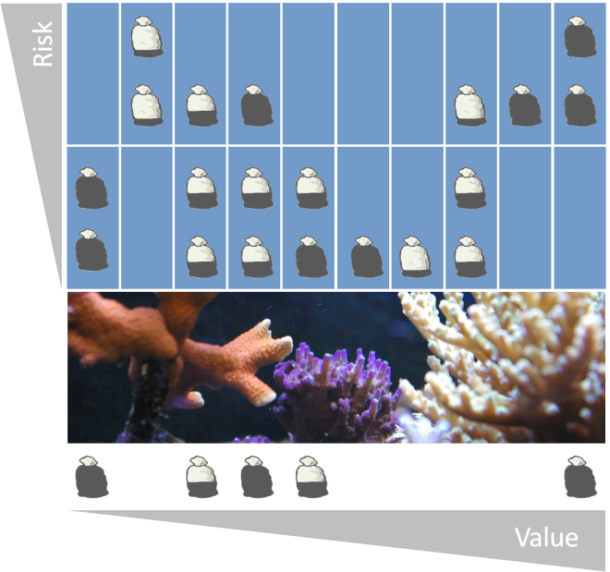 | Figure 3. Knowledge map example |
One of the main goals of any analysis framework is to provide the best possible way of data presentation, in order to achieve the purpose of the analysis. The current version of the framework comes with a considerable enhancement. The new graphical presentation has been designed in a way that allows communication of the knowledge map through different levels and skill sets within the organization without losing the common understanding of the message.The visualization relies on an analogy of a commonly known phenomenon in nature: the coral reef. It is surprisingly well suited to represent knowledge asset related risk issues. Let’s have a look at how this works.In the horizontal dimension there is a coral reef representing the knowledge assets. Firm specific knowledge is in fact similar to corals: ▪ It is pressured: firm-specific knowledge is one of the major assets of the company ([18] to[20]).▪ It lives only within its biotope: firm specific knowledge cannot be acquired (bought) from outside the company.▪ It grows very slowly: firm-specific knowledge can be acquired only on the job.▪ Once a piece of coral breaks off, it dies immediately: firm-specific knowledge is not applicable outside the company. The exception to this is with a direct competitor. However, it is extremely difficult to re-use the acquired knowledge at the competitor site, because it is just a fraction of the whole system.The coral pieces are sorted according to their strategic importance to the company. The most precious corals (Knowledge Units) are at the right hand side.In the vertical dimension, there are Knowledge Stores. Each row represents a single Knowledge Store. Again Knowledge Stores are sorted. In this case, the sorting order has a slightly different meaning. The ones at the bottom are those Knowledge Stores who are the most resistant to employee turnover. On the other hand, the Knowledge Stores at the top are at high risk due to employee turnover. The ordering depends on many different characteristics, such as the value of the given Knowledge Store in the labor market, the age, or the particular tenure.The last part of the picture is sand bags distributed over the bay. Sand bags represent a means of securing the coral reef from the waves of employee turnover. In conceptual terms, they represent Knowledge Assets. The analogy works as follows:▪ Sand bags make the wave break before it reaches the particular coral piece and causes damage to it: The point of knowledge risk management is the optimal protection of the coral.▪ Full (heavy) sand bags protect better than empty ones: the more knowledge is possessed by different Knowledge Stores of a given Knowledge Unit, the less the employee turnover induces any risks – if one leaves, the others are still there.▪ The sand bags far from the coast are swept away by waves more easily than those near to the shore: Unstable Knowledge Stores are more likely to leave the company. There is one more special row of sand bags lying out of the water on the solid ground. These sand bags represent the knowledge redundancy level, in the sense of back up sand (the more sand in the bag, the higher the knowledge redundancy level of the particular Knowledge Unit).Now, when we have inspected all the components of the knowledge map and explained their semantics, we are ready to make some observations based on the example knowledge map presented in Figure 3.Let’s start at the left hand side with the first Knowledge Unit. This is the least important unit, but still enjoying the best possible protection – two full sand bags, both situated at the bottom, and thus pretty much risk free.On the other hand, the Knowledge Unit on the extreme right is the most important one, however it is purely protected. It is true that there are also two full sand bags protecting the reef, but both of them are risky bags (in the upper part of the map). Due to this, the respective Knowledge Unit requires management attention and knowledge management measures to be taken. There is also another Knowledge Unit that needs to be taken care of. It is the fourth unit from the right. Clearly this is still a fairly important Knowledge Unit to the company. However there is only a small fraction of the knowledge left within the company. That means the knowledge is already partially lost and the company is being exposed to a serious business risk. This case requires immediate management action. We could take one Knowledge Unit after the other and perform a similar analysis. As a result, we would end up with a list of measures to take in order to mitigate the risk. The measures translate to filling up the right sand bags with the right amount of sand.Taking measures costs time and money. This is where a cost/benefit analysis comes in. The benefits are clearly visible from the future shape of the knowledge map, but what about the cost? Fortunately, the knowledge quantification approach answers the question right away. As a result of the voting algorithm described earlier we get the knowledge transfer effort for each knowledge unit. Recall that the voting yields a reliable estimate of the price (knowledge transfer effort) of the sand (cf. Table 1).There is a nice saying that translates from German as follows: If you do not decide, life is going to decide for you! Well, this is very much the case in knowledge risk management. Indeed, if you decide against taking measures, the shape of the knowledge map is going to change anyway – probably in a way you will not like.So far, it looks like sufficient investment in the right measures will keep you out of trouble. But it will not. In order to explain the phenomenon, let’s make use of the coral reef analogy again. The ocean is not just waves, but also underwater currents. Such currents are not visible above the water surface, but are still much more powerful than waves. They move the sand bags from one place to the other – whether you like it or not.Let’s describe one such current. Imagine, Kate is an employee who is a respected professional within the company possessing considerable knowledge, however concerning rather unimportant Knowledge Units (the left bottom corner of the map). Kate considers herself to be a part of the company and does not think of leaving it at all. The company recognizes Kate’s talents and promotes her into a core business. Kate acquires a big portion of very important knowledge as a consequence. Now, the Kate’s value in the labor market has risen dramatically. The competitors are willing to engage Kate even at premium remuneration. Kate becomes a high risk employee in terms of employee retention.What happened in terms of currents? The “current” took the sand bags of Kate and moved them to the right (promotion) and then upwards (higher labor marker attention) (Figure 4).Now it should be clear that it is probably not a good idea either to ignore the currents or to deny their existence. The key is recognition of the currents and accounting for them within the decision making process. 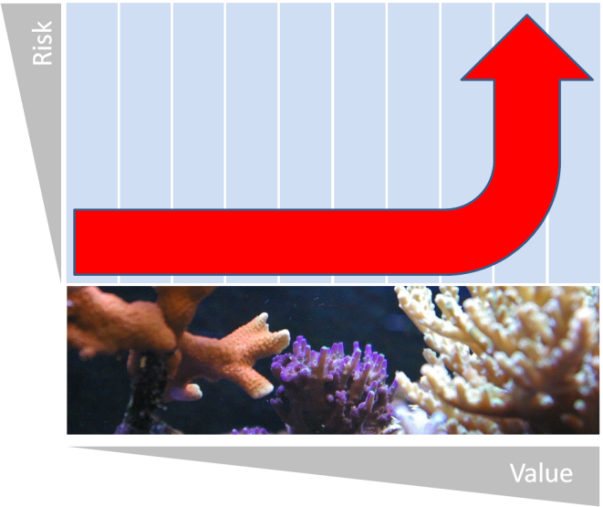 | Figure 4. Current |
7. Implementation Cases
The purpose of this section is to describe the known framework implementation cases pointing to the key insights gained by practitioners.
7.1. Software Engineering Department
The case within this chapter is a follow-up to the one described in[1]. In the meantime, knowledge risk management has become an important part of daily management practice of the department. The following plot shows, how the knowledge assets have evolved in shape.As can be seen in Figure 5, the Internal Redundancy Level improved, even though not rapidly. This can be explained by the fact that the improvements cannot be made at the same pace when the knowledge assets approach the optimal shape.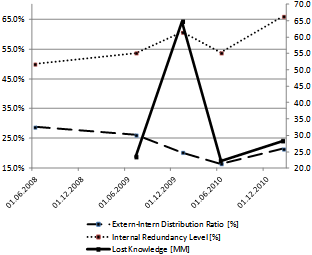 | Figure 5. Comparative view of knowledge map |
Recalling[1], knowledge transfer did not prove to be the most efficient means for closing the knowledge gaps within the department. Consistent with this insight, no more investments have been directed to knowledge transfer. The primary measure to reduce knowledge risk was task assignment within the operations. The results prove that the department found a sustainable way for knowledge asset risk management.Let’s examine the other two key performance indicators. Extern-Intern Distribution Ratio as well as the Lost Knowledge show worse performance than before. This fact can be easily explained by taking a look at the respective knowledge flow log. Both indicators are a consequence of sourcing management decisions. The tenure of one of the external contractors has been terminated, whereas within a different context, a higher level of outsourcing has been reached, due to the internal capacity bottleneck.Generalizing the above statements, the organization is in terms of knowledge risk management at a maturity level, where not only the risks are known and as such manageable, but also their origin in the sense of causality at the root of them is well understood.The department is organized in several teams. One of those teams conducted the update of the knowledge map following the enhanced version of the framework. The team used dedicated software to run the data collection (voting) and generating coral reef visualization as described in this paper. Note that the knowledge data collected in the past has not been considered along the update in order to avoid any additional influences to the voting. The insights gained can be summarized as follows:▪ The duration of data collection decreased rapidly (10% of the former duration), due to parallel computer supported process.▪ Knowledge bearers felt more involved within and more committed to the process, since their own opinion was required instead of the former method of collective data elicitation.▪ The data collected deviated strongly from the former data (46% less in total amount of the knowledge assets). However, the new data was assessed by the line management as matching better the current shape of the knowledge assets in place. The source of deviation can be clearly attributed to the voting mechanism that disclosed a bias in the former estimates. As a major insight, a very special behavioral pattern was disclosed: knowledge bearers were too pessimistic in their estimates when voting collectively, as opposed to the individual voting.Clearly, the methodical enhancements together with the computer aided data collection contributed not only to streamlining the process, but also yielded more reliable results than before.
7.2. Reorganization
The context of this implementation case is a reorganization of the whole IT department of PostFinance. The purpose of the reorganization was to separate the functions of software development from software maintenance. The newly created software maintenance team employs around 40 professionals and is supposed to completely cover the entire software maintenance for all the applications developed by the IT department. The number of applications totals 120, and the yearly maintenance budget is around CHF 120m. The challenging part of the reorganization is the rapid acquisition of the required knowledge by the software maintenance team from the colleagues from within the development departments (who used to maintain the applications in the past).As part of the initiation phase the goal of the framework utilization was an identification of knowledge gaps within the target software maintenance function and setup of the action plan to tackle the respective risk.In response to the risk identified, the reorganization was postponed. The coral reef visualization played a major role in articulating the risk. Decision makers from line management favored the visualization, due to its expressive power and rapidly adopted the “language of corals”. The data collection was done in two steps. The first step aimed at Stored Fraction. After the first step was finished, further data collection (Replacement Effort and Exclusive Fraction) was done, but only for a limited number of Knowledge Units. The Knowledge Units in scope were only the ones at risk, due to the Stored Fraction. This is the way the project decided to go in order to gain more efficiency processing high data volumes (500 Knowledge Units) in a very limited time frame (2 weeks).The main obstacles were experienced during the search for the right architecture of the knowledge unit inventory. It took several iterations to get it right. The key was understanding the essence of the knowledge subject first, then the partitioning of the respective knowledge assets into the Knowledge Units succeeded.
8. Conclusions and Future Research
The research supported by the empirical experience underlines the importance of the initiation phase as a major success driver of any risk management initiative based on the framework.Similarly, finding the right visualizations appears to be a major issue, when it comes to stakeholder management. The coral analogy serves exactly this purpose, and it serves it very well.The introduced bias identification means clearly improve the data reliability making the bias visible at literally no additional cost.Since the framework is relatively new compared to other similar approaches, there are many research opportunities there. This chapter points to several of those in the form of research questions:▪ What is the optimal knowledge redundancy level within an organization in terms of cost/benefit?▪ Which of the business performance indicators (market share, net income, share price, etc) correlates the best with the knowledge risk position of a company?▪ What are the typical currents within an organization of a given corporate culture, and how can they be identified and managed? ▪ What are the maturity levels of Knowledge Risk Management and how do they relate to the known models, such as KMMM®?
References
[1] | V.Riecicky (2011): “Case Study: Replacement Cost Based Approach to Risk Management of Knowledge Assets” |
[2] | Deming, W. Edwards (1986). Out of the Crisis. MIT Center for Advanced Engineering Study. ISBN 0-911379-01-0. |
[3] | Peter Massingham, (2010) "Knowledge risk management: a framework", Journal of Knowledge Management, Vol. 14 Iss: 3, pp.464 – 485 |
[4] | Aljafari, R., Sarnikar, S. (2010): "A Risk Assessment Framework for Inter-Organizational Knowledge Sharing" Sprouts: Working Papers on Information Systems, 10 (29). |
[5] | James Arrow (2008): “Knowledge-Based Proactive Project Risk Management”, AACE INTERNATIONAL TRANSACTIONS |
[6] | Mostafa Jafari, Jalal Rezaeenour, Mohammad Mahdavi Mazdeh, Atefe Hooshmandi, (2011): "Development and evaluation of a knowledge risk management model for project-based organizations: A multi-stage study", Management Decision, Vol. 49 Iss: 3, pp.309 - 329 |
[7] | Samer Alhawari, Louay Karadsheh, Amine Nehari Talet, Ebrahim Mansour (2012) " Knowledge-Based Risk Management framework for Information Technology project", International Journal of Information Management, Volume 32, Issue 1, February 2012, Pages 50-65 |
[8] | Eduardo Rodriguez, Ph.D. John S. Edwards (2008): “Before and After Modeling: Risk Knowledge Management is Required”, ERM Symposium 2008 Casualty Actuarial Society – PRMIA. Schaumberg, IL: Society of Actuaries |
[9] | G Haltiwanger, R E Landaeta, C A Pinto, A Tolk (2010): “Understanding the relationship between Risk Management and Knowledge Management: A literature review and extension”, International Journal of Knowledge Management Studies |
[10] | Edwards, J. S. (2009): “Business processes and knowledge management.”, M. Khosrow-Pour (Ed.), Encyclopedia of Information Science and Technology (Second ed., Vol. I, pp. 471-476). Hershey, PA: IGI Global. |
[11] | Mäenpää, Irinja; Voutilainen, Raimo (2012): “Insurances for human capital risk management in SMEs”, VINE, Volume 42, Number 1, 2012 , pp. 52-66(15) Emerald Group Publishing Limited |
[12] | Eduardo Rodriguez PhD Candidate Aston Business School, Export Development Canada EDC Ottawa Canada (2009): “Knowledge Management and Enterprise Risk Management Implementation in Financial Services”, |
[13] | Object Management Group: “Unified Modeling LanguageTM “ |
[14] | Xu Ye, Zhang Hui (2010): “Literature Review on Enterprise Tacit Knowledge Management”, International Conference on EBusiness and EGovernment |
[15] | Gopesh Anand, Peter T. Ward, Mohan V. Tatikonda (2010): “Role of explicit and tacitknowledge in Six Sigma projects: An empirical examination of differential project success”, Journal of Operations Management, Volume 28, Issue 4, July 2010, Pages 303–315 |
[16] | J. Scott Holste, Dail Fields (2010) "Trust and tacit knowledge sharing and use", Journal of Knowledge Management, Vol. 14 Iss: 1, pp.128 – 140 |
[17] | Brickley, J. / Smith, C. / Zimmerman, J. (William E. Simon Graduate School of Business Administration, University of Rochester 2009): “Managerial Economics and Organizational Architecture” |
[18] | Carayannis, Elias G. (2008): “Knowledge-Driven Creative Destruction, or Leveraging Knowledge for Competitive Advantage: Strategic Knowledge Arbitrage and Serendipity as Real Options Drivers Triggered by Co-Opetition, Co-Evolution and Co-Specialization”, IP Publishing Ltd., Industry and Higher Education, v22 n6 p343-353 |
[19] | Rafael Andreu, Joan Baiget, Agustı´ Canals (2008): “Firm-Specific Knowledge and Competitiv Advantage: Evidence and KM Practices”, John Wiley & Sons, Ltd. |
[20] | Heli C. Wang, Jinyu He, Joseph T. Mahoney (2009): “Firm-specific knowledge resources and competitive advantage: The roles of economic- and relationship-based employee governance mechanisms”, Strat. Mgmt. J., 30: 1265–1285 |