Karobia Pauline N.1, Ngoo Livingstone2, Muriuki James3
1Department of Electrical and Electronics Engineering, Murang’a University of Technology, Murang’a, Kenya
2Department of Electrical and Electronics Engineering, Multimedia University of Kenya, Nairobi, Kenya
3Department of Electrical and Electronics Engineering, Rural Electrification Authority, Nairobi, Kenya
Correspondence to: Karobia Pauline N., Department of Electrical and Electronics Engineering, Murang’a University of Technology, Murang’a, Kenya.
Email: |  |
Copyright © 2021 The Author(s). Published by Scientific & Academic Publishing.
This work is licensed under the Creative Commons Attribution International License (CC BY).
http://creativecommons.org/licenses/by/4.0/

Abstract
In this research, an adaptive method for illegal power connection detection and correction in domestic distribution is presented. The focus is on a domestic supply at the meter box. Illegal power connection has been a major challenge for many years in Kenyan power distribution network. The utility company has estimated to have lost billion dollars yearly due to illegal power connection. The company has been trying to monitor and detect illegal power connection especially on domestic supplies, however the methods used do not satisfactorily identify major cases of these connections. Socio-economic aspects are the major cause that drives the power users to indulge in illegal power usage. Due to this a cost-effective technology need to be developed not limiting to political, economic and engineering aspects to find a lasting solution that involves all power consumers needs and more importantly considering the safety issues of the genuine power users Several types of illegal power usage by consumers are committed but, in this research, illegally connected power users who are aware of their connection status are considered. An adaptive Neural Fuzzy Inference system (ANFIS) is applied to monitor, detect, determine and correct illegal power connection. A model hardware is implemented where several loads are connected to the system to define legal and illegal power connection while also determining the extent to which power is lost. The motivation of this research is to assist the utility company in Kenya to decrease the loss of revenue attributed by non-technical losses which is mainly due to illegal power connection by domestic consumers.
Keywords:
Illegal power connection, Non-Technical Losses, Adaptive system
Cite this paper: Karobia Pauline N., Ngoo Livingstone, Muriuki James, An Adaptive Neuro-Fuzzy Inference System for Detection and Correction of Illegal Power Connection in Kenya, Energy and Power, Vol. 11 No. 2, 2021, pp. 46-53. doi: 10.5923/j.ep.20211102.02.
1. Introduction
Illegal power connection is considered to mean any power usage which is not authorized or not billed by the utility company. These kinds of connections result to non-technical losses in the distribution lines. Due these the utility company is subjected to revenue loss, power usage equipment damage and power supply imbalances. This scenario is a worldwide challenge Kenya being one of the nations affected by it. Due to high rate of population growth, power demand is also increasing on daily basis. The power users intend to indulge in illegal power usage due to high energy prices, poor economic status, lack of jobs, lack of accountability and follow up of power revenue collection procedures and high corruption of utility company staff. In the financial year that ended on June 2020, Kenya power, the major power distributer in Kenya, reported to have suffered power losses worth Ksh 15.9b which translate to (23.5%). This is beyond the allowable (14.9%) (Daily Newspaper). As a result, KPLC plans to reduce the losses to (19.9%) percent before the year 2025. This can be effectively achieved by curbing illegal power connections. Technical losses are allowed natural occurrence in terms of power dissipation losses mainly occurring in power distribution and transmission systems. Non-technical losses on the other side involves illegal connections, meter bypassing, nonbilled power and poor administration. This research assists in finding a more appropriate means of reducing and dealing with the problem currently facing the whole country. Recent papers on -termination of non-technical losses in the distribution lines are reviewed. [1,2]
2. Typical Distribution System at the Consumer Intake Point
As shown in fig.1, This is a correctly connected domestic consumer to a distribution line via a distribution box. The box contain a The utility fuse (cutout), utility energy meter which can either be convectional or digital and a residual circuit breaker. From the RCB, the load is connected from the CCU. Under normal circumstances, digital meters locks if an illegal load is connected at its terminals. However, electricity users have identify other ways of connecting illegal loads directly at the main fuse input or at the output without tampering or switching OFF the energy meter. This means that the meter will still be active despite an illegal load being connected. Another method being used by illegal power users is through connecting a cable at the input or output of the main fuse with the output of the RCB which runs all the way to the CCU. The RCB is switched OFF thus disconnecting the energy meter from the load. These two method are the main ones being used by illegal power users in Kenya. This is due to the fact that, while using digital meters, their is no regular inspections being done by utility field officers unlike in convectional meters where reading of meters is done monthly.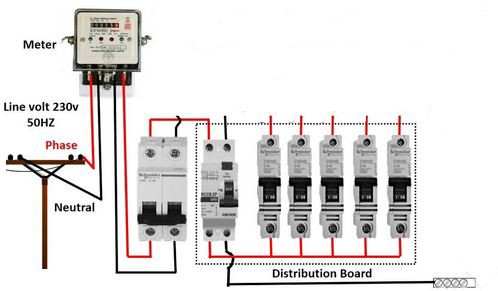 | Figure 1. Typical distribution network |
A. ANALYSIS OF POWER LOSSES IN KENYAN POWER SYSTEMTransmission and distribution of power suffers from two types of Losses which includes:• Technical losses and• Non-technical losses (Commercial)In Kenya, these losses starts all the way from generation, through transmission and distribution up to the consumer intake point. Majority of losses which can be minimized are at the distribution network. Kenya Energy Regulatory Commission has a well defined percentages of losses. Technical and non-technical losses account for (28%) of energy losses in the distribution network according to Kenya Power and Lighting Company (KPLC) data base. The value is more than the allowed percentage set in the energy policy which should be (15%) of losses in the distribution network. The remaining (13%) is absorbed by KPLc into their revenue. These losses are considered as revenue loss where for every one percent (1%) loss is translated to one billion shillings (9.4M USD). This information is From KPLC. The major contributor of Non-technical losses is due to the following factors;• Energy meter tampering which results to the meter read- ing wrong values.• Component failures that periodically increase losses to the network before they are repaired or replaced.• Electricity power cartels who collect money from illegal power users and allow these users to continue utilizing power.• Illegal power connection at the intake point or through Meter bypassing and• Lack of payment of the billed units by the consumer. The main NTLs is bypassing at the cutout and consumers not paying the electricity bills. Although the modern digital meters which are replacing the convectional ones are used in Kenya and are believed to have the ability to solve illegal power usage, the issue of power bypassing still remains as a major challenge thus Non-technical losses becomes very difficult to quantify, detect and eliminate. The issue of illegal power connection has increased drastically due to irregular inspections of the installation by the power utilities, increase of informal settlement in urban areas which result to high demand of power, models of meters whose rate of failure is high thus leading the consumer to use power which is not billed, undetected loads which are connected to the power that the utility company is unaware that they exist and high prices of electricity which drags users to indulge to illegal connection to avoid the high cost. When an illegal load is connected to the system, losses shots drastically increase in the network. The losses are reflected in the utilities’ accounts, and the costs is passed to customers as transmission and distribution charges. Research has shown that, distribution losses in Kenya are calculated as part of the power users’ bills, while in other countries, consumers are charged a flat energy rate. This means that, an rise in non-technical losses in the distribution system would be charged from the legally connected power users. To account for non-technical losses, monitoring, detection and determination of system losses have to be evaluated. The system components and their operations have to be utilized to determine technical losses. Due to the use of less efficient methods of monitoring these losses and knowing where they come from, it is difficult to quantify the total non-technical losses at the domestic intake point thus making the utility company to continue loosing revenue as well as not safeguarding the welfare of the genuinely connected consumers from paying highly costed power.
3. Related Studies
Recent research papers that are addressing illegal power connection monitoring and detection systems have been considered in this paper. In [1] a device for determining illegal power consumption via wireless detection method has been proposed. The device detect illegally connected power consumption using concurrent data before using previously analyzed collected parameters. The device can secure the electricity consumers by minimizing high-resolution data of the customers to a manageable degree. This assist in destroying the high-quality data prompt electricity consumption detected by the consumer energy meter. Internet communication is used to detect the consumer(s) activities at the power intake point. The information pertaining the illegal power consumption is sent to the energy company with details of the suspected energy meter and the amount of un-billed load. Application of expert systems in the determination of high occurrence of NTLs in the distribution systems is addressed in [2]. The application of artificial intelligence in reducing electricity tapping and illegal connection is suggested using ANN. The approach assist in analyzing the consumer’s electricity usage behaviour through a set of inspection parameters. It involves random sampling of power users who represent other power users connected in that specific radius supplied by the same distribution transformer. This sampling method applied may be biased. This may result in the intended inspection not representing all the energy users connected. The bias is gotten from inspection data which makes the predetermined predictions patterns. The data which is captured includes type of energy meter and connection type for a group of consumers. For M customers 0; 1..........; M − 1 for the past N months, 0; 1.........; N − 1 feature of matrix F is computed, where element
is considered to be the daily average kWh consumption feature during the month. Therefore: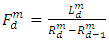 | (1) |
Where;
is the Kilo watt hour consumption rise on the energy meter to the specified date
while the latter date
being the number of days of energy meter reading for customer M. Analysis of periodic data using Artificial Neural Network for electricity consumption is a major problem reason being:1) Parameters collected for electricity usage may have errors resulting to noise.2) Analyzed data is big, thus it makes it difficult to conclusively give the periodicity of the used electricity especially in 1-D time series.3) Electricity consumed parameters are complex, therefore generalization capability is limited.The research highlighted the use of wide component learning which frequently utilizes the 1-D time series, every layer calculates electricity consumption using the following equation; | (2) |
Where;
is taken as the output of the fully connected layer of
neuron, n being the length of 1-D data input (x) and
is the weight of the neuron having a bias b. The calculated value is then sent to the next layer through activation function for determining the extent of effect of the next predicted consumer. The activation function is given by; | (3) |
Where;
is the output after the determined activation. But the technical issue with this approach is that it only considers the positive values which make it not sufficient for the identification of power tapping [3]. The paper addresses electricity fraud by the use of universal anomaly detection. The obtained real-time identification is in a smart network. Several number of consumers connected to one transformer are monitored to determine an unusual consumer behaviour based on electricity consumed values, rate change of electricity usage, date and time of consumed units are recorded. In [4], consumption of energy of the past few years are analyzed to find the cause of increased losses in the distribution lines. Fourteen years are subdivided into five successive clusters and the starting is taken for one year. Fuzzy logic is used as a mean for identifying the number of suspected illegally connected energy users in the first phase. The phase consider every energy user who is connected in the area with high increase in technical losses for analysis. For each customer a time series of billed units are considered for a set-up. Chosen time series data and their relationship is used to put down the fuzzy sets of malicious electricity users. Then, total guessed value of every customer is set by fuzzy logic. All users, whose percentage guessed value is more than the allowable value, are stated as illegal electricity users. [5], identifies the extent of electricity illegally consumed by way of identifying the area where power tapping has occurred. This is considered to manage the illegal consumption in the identified area using neural-fuzzy logic. Advanced Metering Infrastructure is being used with relative entropy to calculate the difference between probability distribution gotten from power usage disparity [6], [7]. The other technology applied is where a GSM based technology is used for determination of illegal power connection [8], [9]. ANFIS [10] is considered in modeling, controlling, and parameter collection in a complex system. The Combination of ANN and fuzzy-set theory provides advantages that assist to overcome the disadvantages in both techniques. ANFIS model is trained without relying on Mathematical model. Expert knowledge is solely employed on to operate the system. The ANFIS model has the advantage of having both numerical and linguistic knowledge which apply the ANN’s ability in classifying data and generating the necessary patterns. Compared to the ANN, the ANFIS model is transparent to the user and leads to less memorization errors. Again, several advantages includes the adaptation aspect, ability to handle nonlinear parameters and the capacity to any continually learn any time newly detected values [11]. The approach is a complete rule-based fuzzy logic model where the rules are developed during the training process [12].
4. System Design and Modeling
The consumer power intake system, which is shown in figure 2 is used for the analysis of various illegal connected power consumption loads at the meter box. The system is developed in Proteus software environment. It consists of: A microcontroller (ATMEGA2656), An LCD display, Two current sensors (ACS758), A relay (5v 1 channel) and AC loads.A. Detection methodologyIn this research, monitoring and detection of illegal power connection at the consumer intake point as well is considered.First, single phase current signal obtained using Proteus simulation of a distribution point is analyzed using a MATLAB generated code to generate data for training. Secondly classification is done where the ANFIS is trained to classify the status of the monitored intake point. Figure 3 represent sample simulation model describing the stages of the system.1) Pre-processing stage: Current drawn by illegally connected loads are obtained through simulation with different rated loads.2) Processing stage: Original illegal drawn current signal is processed by the microproccessor at various rated loads.3) Training: The processed parameters for various considered loads are used for training the ANFIS system.4) Classification stage: Trained ANFIS based classifier detects the type of connection that occurs in the system.B. Adaptive intelligent classifierAn intelligent method for classifying the nature of connection at an individual intake point is presented. The intelligent techniques applied in this research includes Fuzzy logic and ANFIS methods to monitor and detect illegal power usage.1) Fuzzy Logic System (FLS): This is a system utilizes the method of reasoning that relates to human reasoning. The method is flexible, tolerant to indefinite data and utilizes natural language. Hence, this system will classify the type of power connection that occurs in the meter box. 6 steps are followed for training the system.• Step 1: Problem statement and parameter classification (sensor values)• Step 2: Defining the input and output fuzzy sets with variable names• Step 3: Defining member function for the variable• Step 4: Generating the rules• Step 5: Building and testing the system• Step 6: Validating the system2) Adaptive Neuro Fuzzy Inference System (ANFIS): Adaptive Neuro Fuzzy Inference System is used to allow the model to be less dependent trained language and more structured in its operation. The system has two inputs Current 1(S1) and Current 2(S2) and one output which is the difference between the two currents (S1-S2) which were independently determined. MATLAB R2015b software is used to run the ANFIs model in this research. The experimented data is are as in which serves as training database for the ANFIS model. Substractive clustering is applied where data groups are represented in clusters to generate the required fuzzy inference system. Gaussian MFs are selected because of its high accuracy prediction. Hybrid learning method is employed to the system training due to its high efficiency, capability of handling complex and nonlinear problems even in situation where target parameters are not given, minimizes the complexity of the problem especially when dealing which huge amount of data and ANFIS trained data gives results with minimal errors.
5. Results and Discussion
A. Proteus simulation results at different connected loadThe system to monitor the activities at the energy meter box on power usage at the consumer intake point is as shown in figure 2 MATLAB SIMULINK environment is used to generate the code to run the system. Proteus software is considered in the design because it has features that allow modeling, simulating and analyzing the system. Proteus software also contain libraries for interaction with a micro-controller and any analogue or digital system connected to it. The system randomly select electric power load connected at the meter box.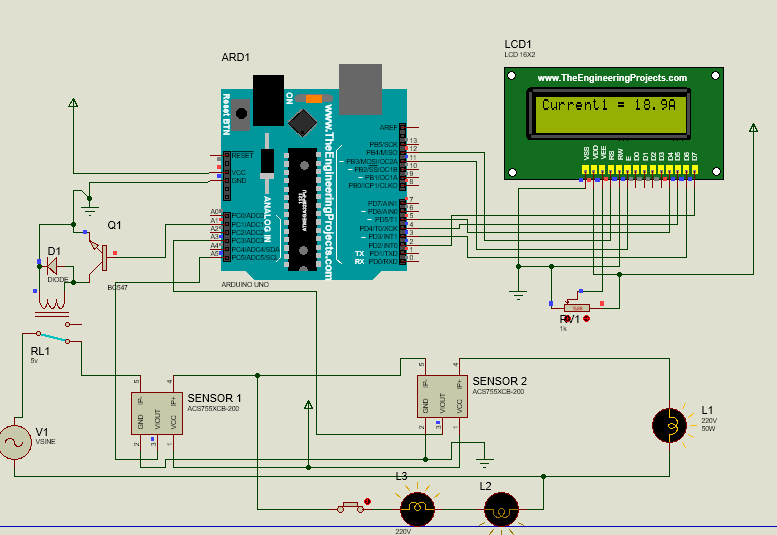 | Figure 2. Simulated model |
The multi-input system is an effective hybrid monitoring and detecting system that identify both external and internal illegal connection. The system mainly deals with current values. It comprises of a service line delivering a voltage of 240 volts at 60Hz, two sensing modules, one before and the other one after the cutout. A serial monitor will be connected to generate the information to be sent to the utility company via a GSM.Identification of illegal connected loads is based on the following assumptions:• That Current sensor one is supposed to detect the incoming current from the service line,• Current sensor two is connected at the output of the cutout and expected to detect the same value as current sensor one if only no load is connected illegally,• In the event of an illegal load, Current sensor two is expected to sense a different current when connected at the input or output terminals of the cutout. This value will not be the same as that sensed by current sensor one,• The microcontroller computes the two current values coming from the sensors in the event of legal and illegal connection. If there is a predetermined difference the interrupting module is triggered switching OFF the entire load from the main supply,• Immediately when the power flow is interrupted the microcontroller process another signal containing the status of the said energy meter in form of an short message that is sent to the utility receiving device via a GSM. The device will periodically record the same status of the connection until the device is reset by the utility company staff.The device is designed such that its supposed to adapt to any changes of current. This is crucial to ensure no consumer is mistakenly detected as illegally connected. The device continually maintain the current status until the issue is resolved. This process is secure and saves the utility company from loss of revenue, power interruptions which is due to unaccounted demand while protecting genuine consumers from inflated bills.1) Normal condition: The two currents detected under normal condition is as shown in figures 3 and 4. In an ideal case, the microcontroller identifies the two values of the current sensor. If the two values are the same, in this case (Current 1= Current 2= 16.2A) as shown in figures 3 and 4. This indicates that there is no illegal connected load that is detected. Therefore, the current from the supply equals the current drawn by the load after the cutout. It shows that the power consumed by the consumer is correct and will therefore correspond with the value recorded by the energy meter for billing thus the connection is termed as a health connection.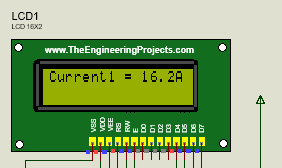 | Figure 3. Sensor 1 (Normal operation) |
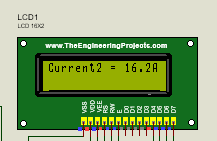 | Figure 4. Sensor 2 (Normal operation) |
2) Abnormal condition: As indicated in figure 5 and 6, when a load of 50 watts, 220v halogen electric bulb is connected normally, a one 100 watts, 220 volts and 50 watts, 220v halogen electric bulb are externally connected, current sensor 1 indicates a current of 30.2A while current sensor 2 detects 27.0A. Since the values are different and the value is greater than 1.8A, thus an illegal load exist. The microprocessor compares the two value as shown in figure 7 and process a signal shown in figure 8 that triggers the relay which switches OFF the legal and external load (illegal) connected loads. Several load are connected to the system and data corrected is shown in table 1. The results are obtained where S1 and S2 are current sensed parameters and dif(S1-S2) is the difference between the two sensed current of each case represented. It is evident that different externally connected loads will inject a predetermined NTLs in the distribution line.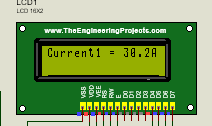 | Figure 5. Sensor 1 (Abnormal operation) |
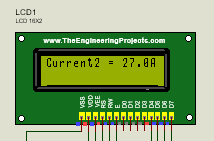 | Figure 6. Sensor 2 (Abnormal operation) |
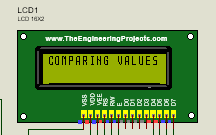 | Figure 7. Comparing (S1 and S2) |
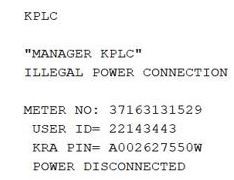 | Figure 8. GSM signal |
3) System Training: The training and testing parameters of the ANFIS are as shown in table 1 are used in MATLAB SIMLINK environment. Four Gaussian membership function are applied in the analysis of the model. Hybrid method is used for optimization for training data. 200 epochs are used in this study. Development of the ANFIS system model, analysis of the monitored information and detected data are implemented as follows. Confusion matrices is applied to validate each case created to evaluate illegal connected load performance using ANFIS algorithm. The matrix indicates four results data:1) VL (Very Low)-Normal operation(Difference =0A)2) L (Low) -Normal operation (Difference <1.8A)3) H (High) - Abnormal operation (Difference >1.8A)4) VH (very High)-Abnormal operation (Difference >> 1.8) Table 1. Simulation Sensor Data at Different Rated Loads 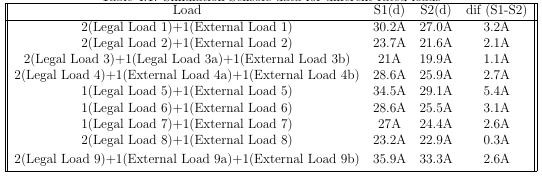 |
| |
|
The datasets for power connection scenario is divided randomly in 20 rules (figure 9) based on the four scenarios. Then they are inserted into ANFIS model for training and analysis for the purpose of classifying power connection as legal or illegal, respectively. The ANFIS structure is as shown figure 10. ANFIS for the above-stated purpose comprises of five layers, two inputs namely sensor 1 and sensor 2, one output that classifies the connection status into one of the four connection categories namely very low (VL), low (L), high (H) and very high (VH). Initially crisp sets of input variables are represented in the form of fuzzy sets as depicted in table 2. There is one output variable that determines the connection category of value that ranges from 0 to 1. ANFIS is used to refine the 20 rules. Membership functions are hypothesized and tuned to assign membership values between 0 and 1 to assign the degree of truth to each linguistic label (very low, low, high and very high) based on value of the input variable (S1 and S2). The output variable of each rule are also updated based on the actual sensor data. ANFIS-based illegal power connection analysis results are shown in table 2. The ANFIS based illegal power detection and collection Susceptibility Analysis Component is used to assess the consumer activities at the meter box in Kenya distribution system. It is observed (figure 11) that highest current sensor difference averaging to 0.965 respectively.Table 2. Output Classification 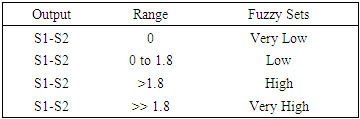 |
| |
|
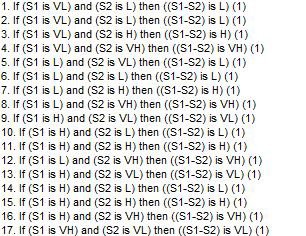 | Figure 9. Fuzzy Rules |
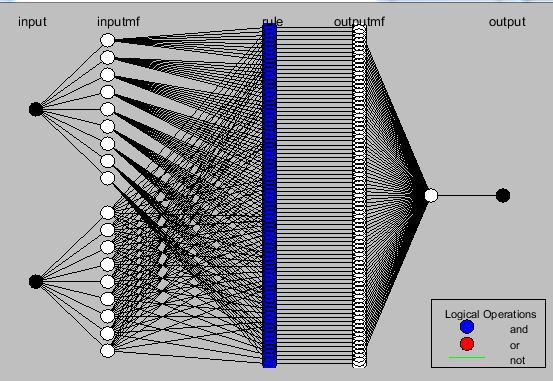 | Figure 10. ANFIS Structure |
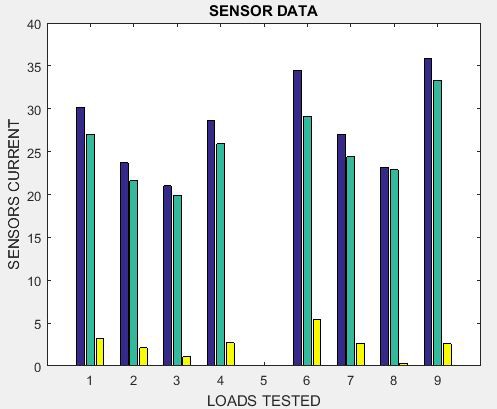 | Figure 11. Comparative analysis of various Loads considered illegal |
It is evident (figure 11 and 12) that a highly rated load (e.g Load 6) drew very high current from the line therefore the difference between the two sensor is also high. The higher the difference the higher the loss introduced to the line. The projections from the ANFIS unit is for a given set of input and outputs values are accorded by the use of rule viewer where any change in input parameters correspond to change in the expected output, as shown in figure 12. For example when S1=30.2A and S2=27 the output (S1-S2)=2.02A. These results is a clear indication that the ANFIS model has the a high ability to project power in monitoring, detecting and determining illegal loads profile connected at the consumer intake point for any set inputs. In case of data volume, the ANFIS simulation time is much better.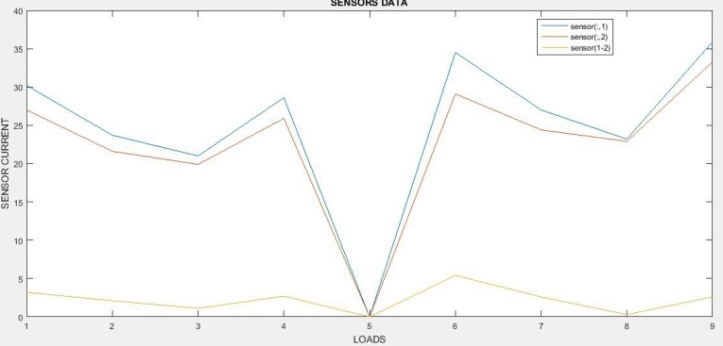 | Figure 12. Observed Sensor Values |
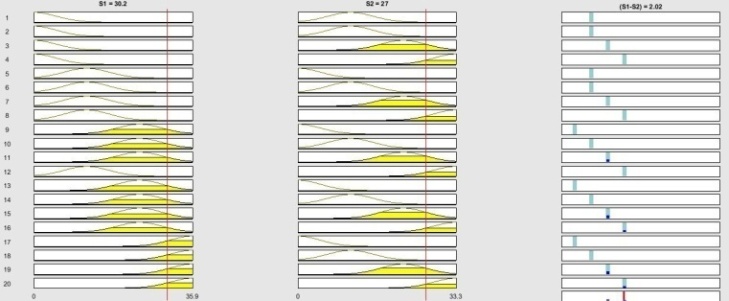 | Figure 13. Surface view of Loads considered illegal |
6. Conclusions
In this research, design and simulation of an experimental ANFIS based power system to monitor and detect illegal power connection in Kenya has been achieved. It has considered illegal power tapping at the surface head which leads to NTLs leading to a reduction of power revenue to the utility companies and power instability in the distribution lines which is due to uncontrolled loads. Genuine connected power users are as well safeguarded from inflated electricity bills brought by NTLs. The system is automated and with remote monitoring of illegal connection and sending SMS whenever there are abnormal readings at the customer intake point, the developed system is able to help utility company to reduce the incidences of household electricity theft. An automatic interrupting switch is integrated into the unit so as to remotely cut OFF the power supply to any consumer who tries to indulge in illegal connection. This design, therefore, removes the manual inspection with its attached consequences of time-consuming. ANFIS was employed since it is an important applications in real-world data mining projects, due to its capability to discrete decisions enhanced by continuous analysis.
References
[1] | Bin-Halabi, Ahmed and Nouh, Adnan and Abouelela, Moham- mad, Remote detection and identification of illegal consumers in power grids, vol 7, pp 71529–71540, 2019. |
[2] | Glauner, Patrick and Meira, Jorge Augusto and Valtchev, Petko and State, Radu and Bettinger, Franck, The challenge of non-technical loss detection using artificial intelligence: A survey, 3rd ed., 2016. |
[3] | Razavi, Rouzbeh and Gharipour, Amin and Fleury, Martin and Akpan, Ikpe Justice” A practical feature-engineering framework for electricity theft detection in smart grids, vol.238, pp.481-494, 2019. |
[4] | Spirić, Josif V and Stanković, Slobodan S and Dočić, Miroslav B., Identification of suspicious electricity customers, vol. 95, pp.635-643, 2018. |
[5] | Palaniyappan, S, Fuzzy Logic Based Electricity Theft Identification in Distribution Systems, vol.4, No.4, pp.13-18, 2019. |
[6] | Singh, Sandeep Kumar and Bose, Ranjan and Joshi, Anupam” IET Cyber-Physical Systems: Theory & Applications, vol. 3, No.2, pp. 99- 105, 2018. |
[7] | Nabil, Mahmoud and Ismail, Muhammad and Mahmoud, Mohamed and Shahin, Mostafa and Qaraqe, Khalid and Serpedin, Erchin, Deep Learning-Based Detection of Electricity Theft Cyber-Attacks in Smart Grid AMI Networks, pp 73–102, 2019. |
[8] | Mucheli, Nitin K and Nanda, Umakanta and Nayak, D and Rout, PK and Swain, SK and Das, SK and Biswal, SM, Smart Power Theft Detection System, pp.302–305, 2019. |
[9] | Saad, Muhammad and Tariq, Muhammad Faraz and Nawaz, Amna and Jamal, Muhammad Yasir, Theft detection based GSM prepaid electricity system, 2017 3rd IEEE International Conference on Control Science and Systems Engineering (ICCSSE), pp.435–438, 2017. |
[10] | Prasad, K.; Gorai, A.K.; Goyal, P. Development of ANFIS models for air quality forecasting and input optimization for reducing the computational cost and time. Atmos. Environ. 2016, 128, 246–262. |
[11] | Srisaeng, P.; Baxter, G.S.; Wild, G. An adaptive neuro-fuzzy inference system for forecasting Australia’s domestic low cost carrier passenger demand. Aviation 2015, 19, 150–163. |
[12] | Ghiasi, M.M.; Arabloo, M.; mohammadi, A.M. Application of ANFIS soft computing technique in modeling the CO2 capture with MEA, DEA, and TEA aqueous solutions. Int. J. Greenh. Gas Control 2016, 49, 47–54. |