Ezeh Ernest1, Okeke Onyeka2, Nwosu David3, Umeh Joel4
1Chemical Engineering Department, Nnamdi Azikiwe University, Awka, Nigeria
2Plastic Production Section, Scientific Equipment Development Institute, Enugu, Nigeria
3General Laboratory, Scientific Equipment Development Institute, Enugu, Nigeria
4Electroplating Section, Scientific equipment Development Institute, Enugu, Nigeria
Correspondence to: Okeke Onyeka, Plastic Production Section, Scientific Equipment Development Institute, Enugu, Nigeria.
Email: |  |
Copyright © 2016 Scientific & Academic Publishing. All Rights Reserved.
This work is licensed under the Creative Commons Attribution International License (CC BY).
http://creativecommons.org/licenses/by/4.0/

Abstract
Research was conducted to determine the level of emission of three greenhouse gases (CO2, H2O and SO2) through vehicular activities within Enugu Metropolis using gaseous pollutant sampler envirotech ARM 433 and fourier transform infra-red spectroscopy. The analysis showed that CO2 emission was above the permissible limits of 350mg/m3 in nine out of the 12 calendar month of the study year, 2015. SO2 and H2O were within their respective emission permissible limits across the length of the year studied. Stochastic model equations showed that relative humidity and traffic density greatly influenced the levels of emission of pollutant/greenhouse gases through vehicular activities in any given environment. Stochastic modeling equation successfully predicted the level of gaseous emissions in 2017 within Enugu metropolis which could be adopted by relevant government agencies towards pollution control measures.
Keywords:
Stochastic modeling, Greenhouse gases, Pollution and meteorological data
Cite this paper: Ezeh Ernest, Okeke Onyeka, Nwosu David, Umeh Joel, Analysis, Evaluation and Stochastic Modeling of Emission Levels of Three Greenhouse Gases (CO2, H2O and SO2) through Vehicular Activities within Enugu Metropolis, World Environment, Vol. 6 No. 3, 2016, pp. 79-83. doi: 10.5923/j.env.20160603.02.
1. Introduction
Clean air is essential for good health of humans, animals and birds [2]. Since the industrial revolution, the quality of the air we breathe has deteriorated considerably mainly as a result of human activities [16]. All the countries in the world are facing a common problem of air pollution. Rising industrial production and dramatic rise in traffic on our roads all contribute to air pollution in our towns and cities. This in turn can lead to serious health problems to living things when the air pollution exceeds permissible limits [17]. Pollution is an undesirable change in the physical, chemical or biological characteristics of air, water and soil that has potential health hazard to any living organism particularly man [4]. Any substance that causes pollution is called a pollutant. A pollutant may include any chemical or agrochemical substance, biotic component or its product or physical factor (heat) that is released by man into the environment that may have adverse harmful or unpleasant effects [4]. Pollutants are residues of the things we make use of and throw away. Air pollution is a deliberate or inadvertent deposition of materials in pure air, which affects the physical and/or chemical properties of the air and subsequently, caused detectable deterioration of air quality [11]. Thus polluted air is one which in addition to its normal constituents contains other substances called pollutants. Air pollution has three components; the emitting source, atmospheric transport and dispersal and the receptor [14]. The degree of air pollution depends on the interaction of these identified components. Air pollution results mainly from gaseous emissions of industry, thermal power stations, automobiles, domestic combustions, smoke from fire, burning coal mines, decaying vegetation, volcanic eruption, sewers and smelting industries [1]. The main air pollutants emitted from these different sources include: carbon components (CO and CO2), Sulphur compounds (SO2 and H2S) nitrogen oxides (NO, NO2 and HNO3) and Ozone e.t.c.According to the World Health Organisation [18], millions of untimely deaths are occurring due to the urban air pollution created from burning of solid fuel. Most of the deaths due to air pollution are from developing nations [13]. Usually, children are the most susceptible to harmful influences due to their tender tissues, higher surface volume ratio and relative inhalation rate for healthier growth and build-up [12]. Other effects air pollutants include, greenhouse effects, global warming, acid-rain, eye-irritation, breathe difficulties, genetic abnormalities, blood poisoning, heart diseases, damage to fruits and vegetables and blackening and corrosion of building materials [9].Air pollution control involves a number of measures which include, treatment of industrial wastes before disposal by industries, enlightenment of citizens through different media on the dangers of indiscriminate disposal of waste materials, regular maintenance of vehicles to ensure complete combustion of petrol and construction of motor parks in every nook and cranny of the society to reduce traffic jams which could lead to increased pollution from exhaust fumes of vehicles [7]. On the other hand, air pollution control involves an inter play of issues and interests that are dealt with on daily basis. Many issues involved in air pollution control are mobile sources, cost benefit-ratios in control enterprise and the complimentary efforts of regulatory agencies.Control techniques for limiting pollutant formation and emission include system enclosure, emission capture, products reformations and feed stock modifications e.t.c. [15]. In principle the emission level air quality problem could be resolved with the use of a reliable, fully validated mathematical model based on fundamental description of atmospheric transport and chemical process [10]. The model involves emission patterns, metrology, chemical transformations and removal processes. The efficient management of air resource means the ability to translate a specified time and location pattern of discharges of gaseous residuals into the resulting time and space pattern of ambient concentrations, including consideration of synergistic effects and chemical and physical reactions in the atmosphere after discharge [3]. This translation reflects mostly the impact of various meteorological processes on the transportation and dilution of pollutants in the atmosphere. A host of mathematical and decision support techniques have been developed and employed to aid in forming air-quality planning optimization models. The first category examines deterministic models in which parameters are assured to be known with certainty in advance. In the late seventies and eighties, researchers began to recognize short comings in deterministic models. This led to stochastic approaches that mainly deal with great variability in meteorological conditions [8]. Hence the stochastic approach which accounts for the uncertainties in the parameters. Stochastic models are based on the analysis of past and present data and consist of the observed relationship between variables describing ambient air quality and variable describing the pollution source.This research aims to assess the pollution level of the greenhouse gases (CO2, SO2 and H2O) through vehicular emission in Enugu Metropolis. The objectives are to employ stochastic model in relation to understanding variables that precipitates persistent presence of greenhouse gases in our environment and predict the future emission levels with a view to formulating air pollution control policy.
2. Materials and Methods
The meteorological data (traffic density, wind speed, dry-bulb temperature and relative humidity were obtained from Enugu State University of Science and Technology, meteorological observation. The quantity of gasoline (litres) consumed per hour was obtained through questionnaire analysis.The quantity of CO2, H2O and SO2 emitted through vehicular emission was obtained and analysed using gaseous pollutant sampler environtech ARM 433 and Fourier – transform infra-red spectroscopy [13]. This is an independent instrument used for monitoring and quantifying gaseous pollutants like SO2, COx, H2S etc, in ambient condition using chemical methods. Sampled is bubbled through reagents that absorb specific gaseous pollutants and absorbing media analyzed. Analysis: The data obtained were subjected to analysis of variance and regression at 99% confidence level.The model: Level emission  | (1) |
and the transform is as follows; | (2) |
WhereX1 = volume of trafficX2 = Dry bulb temperature (oC)X3 = Wind speed (km/hr)X4 = Relative humidity (%)The models seeks to evaluate and verify the dependence of At(γ) on meteorological conditions (X1, X2, X3 and X4) at 99% confidence level.The second model predicts the level of gaseous pollution of the environment at any given time thus: | (3) |
The prediction performance of the model is based on the availability of parameters such as the meteorological data that influence the level of emission of gases in a given environment. The stochastic model was calculated using a speed optimized version, SMSIM version 2.0 [10].
3. Results and Discussion
X1 = Traffic density; X2 = Wind speed; X3 = Dry-bulb temperature; X4 = Relative humidity. Table 1 shows that the months of September to December, 2015 witnessed the highest traffic density in the metropolis which could be due to approach and celebration of yuletide season, while the lowest traffic density was recorded in the months of February, March and May.Table 1. Average traffic density, wind speed, dry-bulb-temperature and relative humidity across the seasons of the year (Jan-Dec, 2015) 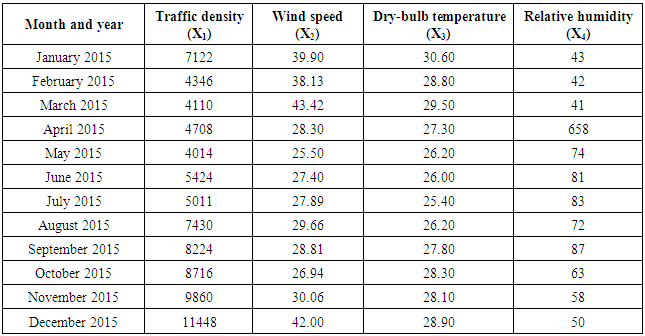 |
| |
|
The wind speed was highest in the months between December to March, which could be due to the wind movement from the Sahara region, a sign of peak of dry-season. The dry bulb temperature has averagely similarly values across the period under review. The percentage relative humidity was highest in the months of June, July and September which were peak period of rainy season while the lowest percentage of relative humidity was between the months of January –March which represents peak periods of dry-season.Table 2 shows that average quantity of three greenhouse gases (CO2, SO2 and H2O) emitted as a result of gasoline consumption through vehicular activities was highest in the months of June, July and September while the least emission was in the months of January to April. This observation could be because the months of June to September were peak periods of rainy season, in which the humidity is usually very high and temperature low thus necessitating the increased pressure in the engine to heat-up and circulation fuel-flow [6]. However, the months with comparable low greenhouse gas emission (January to April) were periods of dry season in which the humidity is usually low and temperature of the surrounding usually high.Table 2. Average rate of emission of greenhouse/pollutant gases from automobile exhaust per hour in 2015, within Enugu Metropolis 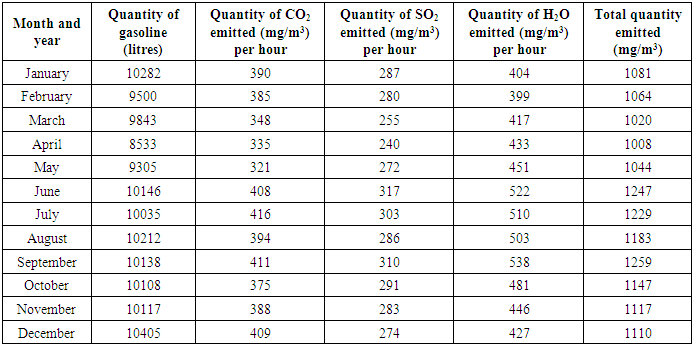 |
| |
|
The result shows that the quantity of SO2 and H2O emitted per hour of the period of the study covering Enugu Metropolis were within the permissible emission range of 350 and 4000mg/m3 respectively [5]. The high level of vehicular activities within the metropolis resulted to the emission of CO2 per hour above the permissible level of 350mg/m3 in the months of January, February, June, July, August, September, October, November and December. The emissions at this level could increase the average global temperature which is capable of triggering severe weather events with wild extremes in temperature and precipitation [6]. In other to verify the dependence of level of greenhouses emission on X1, X2, X3 and X4, the results of Table 1 and 2 were evaluated by subjecting it to stochastic and regression analysis at 99% confidence level.Since the critical value of t was 2.95, for X1, t – calculated was 70.25, which suggests that volume of traffic can influence the level of greenhouse gas emission at any given time. The t – calculated for X2 was 0.337 and lower than the critical t (2.95). This shows that dry bulb temperature may not influence the level of greenhouse gas emission at any time. For X3, the t – calculated (0.869) was lower that the critical t (2.95), with suggests that wind speed may not influence the level of emission of greenhouse gases at any particular period (especially through vehicular activities).The calculated value of t for X4 was 292.06 and this represents a significant higher value compared to the critical t (2.95). The consequence of this is that X4 (relative humidity) has a major influence on the level of emission of greenhouse gases though vehicular activities in an environment at any given time.The stochastic model equation 2 was reduced to Log At(γ) = bo + B1logX1 + B4logX4, which represents the meteorological conditions that influence the level of emission of gases in a given environment and thus futuristic emission of gases from vehicular activities could be predicted. The 2017 emission levels within Enugu metropolis was successfully predicted using the stochastic model equation as presented in Table 3.Table 3. Result of observed and predicted levels of greenhouse gas emission 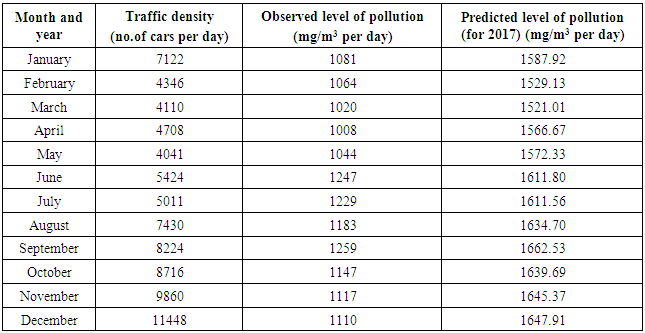 |
| |
|
The result of Table 3 shows that in 2017, the level of emission of pollutant/greenhouse gases through the exhaust of vehicles will increase by over 60% which could be due to the increase in number of vehicles on the road, the increase in the number of poorly maintained vehicles on the road (due to economic stagnations) and vagaries of the weather conditions.
4. Conclusions
The study showed that factors like traffic density and relative humidity can greatly influence the level of emission of pollutant/greenhouse gases especially through vehicular activities at any time in any environment which were validated using stochastic model equation. Stochastic model equation was used to predict the level of emission of greenhouse/pollutant gases for 2017 and the prediction revealed increase in level of emission by over 60%. The consequence of this development to man and his ecosystem should be unpleasant. Policy makers across every government level could adopt this model approach in coming up with strategies that will control and regulate the level of emission of pollutant gases to the environment.
References
[1] | Breton C.V. and Marutani N. (2004). Air Pollution and Epigenetics: Recent finding. Curr Environ Health Persp, 1:35-45. |
[2] | Chaunhan A., Pawar, M. and Kumar, R. (2010). A case study of Haridwar and Dehradin using Air quality index, Journal of American Science, 6(9): 55-62. |
[3] | Cheng Y.L., Bai Y.H., Li, J.I. and Liu, Z.R. (2007). Modeling of air quality with a modified two dimensional models: A case study in the Pearl River Delta region of China. Journal of Environmental Sciences 19: 572-577. |
[4] | Colls, J. (1997). Air pollution: An Introduction, E & FN Spon, London. pp: 440-447. |
[5] | EAQS (2013). European Air Quality Standards. Gazette of European Commission. pp 2-9. |
[6] | Fahimeh, A. and Azadeh, I. (2012). Influence of meteorological parameters on air pollution in Isfahan, International Conference on Biology, Environment and Chemistry, 46(4): 929-939. |
[7] | Frey, H.C., Zhai H. and Rouphail, N. M. (2009). Regional on road vehicle running emissions modeling and evaluation for conventional and alternative vehicle technologies. Environ Sci. and Technology, 43: 8445-8449. |
[8] | Fronza, G. and Melli. P. (1984). Assessment of emission abatement levels by stochastic programming. Atmospheric Environment, 18(3): 531-535. |
[9] | Gaffrey, J.S. and Marley, N.A. (2009). The impacts of combustion emissions on air quality and climate from coal to biofuels and beyond. Atmospheric Environment, 43: 23-36. |
[10] | Kohn, R.E. and Burlingame, D.E. (2000). Air-quality control model combining data on morbidity and pollution abatement, Decision Science, 2:300-310. |
[11] | Kooter, I.M., Van Vugt A., Jedynska, P., Tromp, M., Houtzager, M. and Ver beck, R. (2011). Toxicological characterization of diesel engine emissions using bio diesel and a closed soot filter. Atmospheric Environment, 45: 1574-1580. |
[12] | Kumar, N., Chu, A. and Foster A. (2008). Remote sensing of ambient particles in Delhi and its environs: estimation and validation, international Journal of Remote Sensing. 29: 3383-3405. |
[13] | Madhukar, R. and Srikantaswamy, S. (2013). Assessment of air quality in Bidadi Industrial Area, Ramana gram District Karnataka, India. Journal of Environmental Science, Computer Science and Engineering and Technology, 2(4): 1135-1140. |
[14] | McDonald J.D., Eide, I., Seagrove J., Zielinska, B. Whitney, K. and Lavson, D.R. (2004). Relationship between composition and toxicity ofmotor vehicle emission samples. Environ Health prospect, 112: 1527-1538. |
[15] | Najjar Y.S.H. (2008). Modern and appropriate technologies for the reduction of gaseous pollutants and their effects on the environment Clean Techn Environ policy, 10:269-278. |
[16] | Najjar, Y.S. (2014). Gaseous pollutants formation and their harmful effects on health and environment. Innovative energy policies vol. 1: 1-8. |
[17] | Pope, C.A., Burnett, R.T., Thun M.J., Calle, E.E., Krewski D. and Ito, K. (2002). Lung cancer, cardio pulmonary mortality and long term exposure to fine particulate air pollution, Atmospheric Environment, 45:1042-1049. |
[18] | WHO (2010). Guidelines for Air quality, World Health Organization, Geneva. pp121-123. |