Benjawan Suppasilp1, Chaiyawat Suppasilp2
1School of Economics, Shanghai University, Shanghai, China
2Department of Clinical Epidemiology and Biostatistics, Mahidol University Faculty of Medicine Ramathibodi Hospital, Thailand
Correspondence to: Benjawan Suppasilp, School of Economics, Shanghai University, Shanghai, China.
Email: |  |
Copyright © 2020 The Author(s). Published by Scientific & Academic Publishing.
This work is licensed under the Creative Commons Attribution International License (CC BY).
http://creativecommons.org/licenses/by/4.0/

Abstract
With the development of technology like electronic devices and the internet has invented a new channel of commerce, Electronic commerce (E-commerce). Countless consumers had changed their shopping behavior and consider online shopping channel as the convenience of living. The online shopping channels are new opportunities for investors and retailers to get benefits and advantages of selling goods and services. However, there are still some challenges and obstacles for online retailers to maximize consumer satisfaction and replace the traditional shopping behavior. This research is the study on influential factors affecting online shopping decisions in Bangkok, Thailand, by using Factors, Filtering element and Filtering buying decision theory (FFF model). The surveys were collected in different districts in Bangkok, where is the capital city of Thailand, and the high percentage of mobile penetration and internet users. Also, Thailand’s E-commerce had been growing statistically in the past few years. The study had collected the total number of 419 surveys for analysis. The results found that gender, occupation, address, educational background, income, and privacy are statistically significant factors affecting online shopping decisions in Bangkok. Analyzing by theorized FFF model, the results found that privacy of consumers is the most concerned while security factors and trust and trustworthiness factors were less affected. Therefore, to overcome all challenges and obstacles and maximize online consumer satisfaction, E-marketplace should invent a high security platform and prevent consumers’ personal information and protect consumers’ privacy.
Keywords:
E-commerce, Online shopping, Consumer behaviors, Factors - Filtering elements - Filtering buying behavior model (FFF model)
Cite this paper: Benjawan Suppasilp, Chaiyawat Suppasilp, Influential Factors on Consumers’ Online Purchasing Decision in Bangkok, Thailand, American Journal of Economics, Vol. 10 No. 3, 2020, pp. 163-171. doi: 10.5923/j.economics.20201003.06.
1. Introduction
Electronic commerce (E-commerce) historically meant the process of execution of electronic commercial transactions with technologies such as Electronic Data Interchange (EDI) and Electronic Funds Transfer (EFT). The EDI requires agreement between trading partners in order to govern their electronic trading relationship [1]. It gave a massive opportunity for users to exchange business information, do electronic transactions, and share commercial documentation electronically. In 2000, the definition of E-commerce was changed to the process of purchasing of available goods and services over the internet using secure connections and electronic payment services [2]. Since then, E-commerce has been using as an online channel for business and transformed traditional shopping beyond expectations. E-commerce today gained a high level of popularity since its convenience and reliability. People highly accept in online shopping, and it becomes the shopping nature of consumers. In the foreseeable future, E-commerce will be the ultimate tool for commerce with sales boom around the world. E-commerce business types can be classified into four basic types of commerce, which are B2B, B2C, C2C, B2G. Business-to-Business (B2B): the online transactions of goods or services through online channels between company to company. It does not engage in sales to the consumer public. Business-to-Consumer (B2C): companies sell their goods or services through online channels to consumers who are the end-users. Consumers buy products or services for self uses. The shop websites open for any users or visitors. Consumer-to-Consumer (C2C): this type of E-commerce encompasses all electronic transactions of goods or services between individuals through the third-party platform. Business-to-Government (B2G): the government offers a project and put out tenders1 which companies bid for. Then the government reviews and selects the company that meets their price expectation.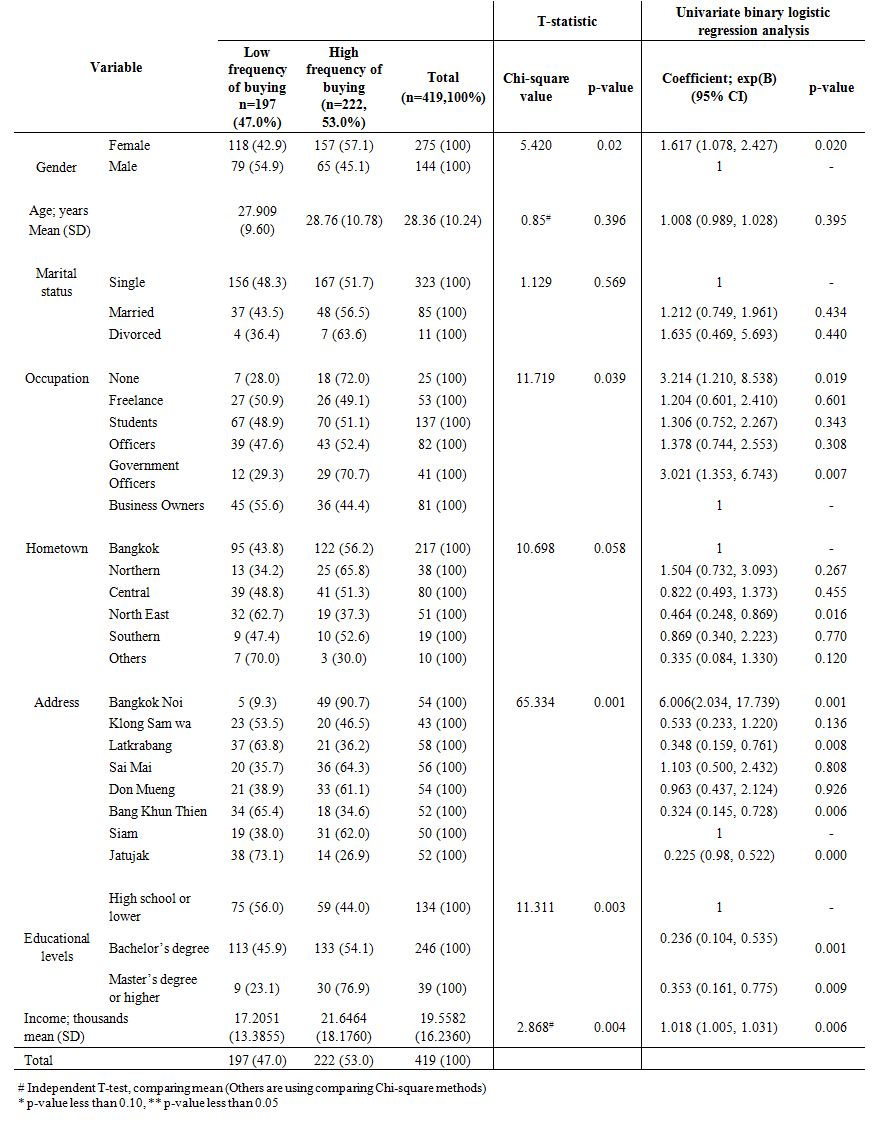 | Table 1. Demographic data and Univariate binary logistic regression analysis |
E-commerce in ThailandThailand is a member of the ASEAN community in South East Asia with the 4.1 percent of GDP growth in 2018 by the Office of the National Economic and Social Development Council of Thailand [3]. The E-commerce growth of Thailand ranks first place from total countries in ASEAN with a value of 24.70 billion US dollars or 3.2 trillion baht in 2018 [4]. According to Electronic Transaction Development Agency, ETDA [5], Other countries with a high value of E-commerce transactions are Burma, Vietnam, Singapore, and the Philippines, with the amount of 19.41, 8.21, 4.61 and 1.84 billion US dollar respectively. The growth of E-commerce in Thailand has been increasing continuously about 8 to 10 percent each year since 2014, which can be assumed that the E-commerce market in Thailand had expanded rapidly [5]. Comparing between the growth of E-commerce in 2017 and 2018, the value of E-commerce in 2017 is 2, 762,503 million baht, and the value of 2018 is 3, 150, 232 million baht.Besides, entering international E-marketplace, Thai companies still need to improve the application system and increase the domestic market share. In Thailand, countless applications are offering online channels for both producers and consumers. However, to increase the number of online transactions, a company must know what the consumers’ requirements and concerns about their safety of online purchasing are and what are the influential factors for consumers to purchase through online channels.Motivation of studyIn this paper, the main theory for this study is Factors, Filtering elements, Filtering buying decision (FFF model). Adapting the FFF theories to indicate independent variables which possibly affect consumer’s online purchasing decision in Bangkok, Thailand. The study is the first study in Thailand that use FFF theory as commercial analysis. The purpose of this study is finding the most influential factors on online shopping decision, and which factors encourage consumers to shop online, which factors consumers concern the most and the least in the FFF theory.
2. Factors, Filtering Elements, Filtering Buying Decision (FFF Model)
The consumers' behavior model is a base theory for consumers who would like to purchase a product or services. This theory is used and applied to most of consumer behavior theory, including Factors, Filtering elements, Filtering buying behavior model (FFF model). The FFF model is developed by Dr. Ujwala Dange and Professor Vinay Kumar [6]. The FFF model is developed based on the consumers' behavior model, but the developers had noticed the differences in buying behavior between the physical store and online store. Therefore, the developers decided to include concerned elements and buying behavior of consumers when they would like to purchase online, which are filtering elements and filtering buying behavior. 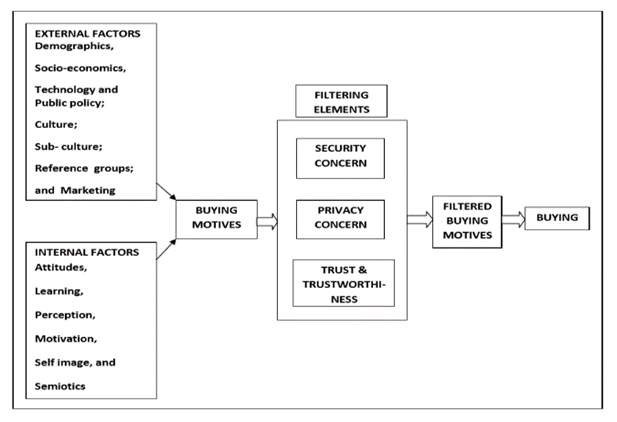 | Figure 1. Online consumer buying behavior motive model, Factors (F), Filtering elements (F) and Filtered buying motive (F); (FFF Model) |
Factors - The FFF model clarifies that the external factors are demographics, socioeconomics, technology and public policy, culture, sub-culture, references group, and marketing campaigns or promotion. For the internal factors, the inner motive from an individual’s perspective toward online shopping which are attitudes, learning, perception, motivation, self-image, and semiotics. In addition, it also states that there are two types of motives while shopping, which are functional and non-functional motives.Filtering elements – There are three risks that consumers could face when they purchase goods and services through online channels, which are a security risk, privacy risk, and trust and trustworthiness risk of the application. As online shopping, sellers and consumers can only interact on the internet by messaging or emailing to each other, and the consumers cannot see actual products but photos. In addition, consumers have to pay money in advance before the products are delivered.Filtering buying motive - After a consumer has been through the influential factors, both external and internal and filtering elements, security, privacy, trust and trustworthiness, the consumer might rethink those processes again and consider buying it or not.Relevant Studies Jutarat Kaidrasamee [7] studies about factors affecting the decision making on purchasing products from the online application in Bangkok metropolitan region. She had studied several factors and subfactors effect on decision making, including gender, age, education background, monthly income, package, price, delivery channel, marketing campaign, attitudes and acceptance of technology, security, and trustworthiness and services. According to the results, the main group of online shopping consumers are female (64.4%) and male (35.6%). Consumers in the age between 21 to 25 are the majority group of online consumers with the percentage of 40.74. In contrast, consumers in age 26-30 and 31-35 years old are another big group of online shopping, 35.56% and 12.59%, respectively. On the other hand, consumers in age 36-40, 15-20, 41-45 and 46 above, 6.17%, 3.70%. 0.99% and 0.25, respectively, they could prefer to shop through the physical stores than the online channel. The highest educations for online shopping consumers are Bachelor’s degree (76.05%), a Master’s degree (16.05%), and below Bachelor’s degree (7.9%). The occupations prefer to shop online is private company employees (63.46%), public company employees (16.54%), business owners (11.36%), students (7.41%), housewife (0.74%) and freelance (0.49%) respectively. The monthly income of online consumers will be ranked as below 15,000 baht (9.38%), 15,000-25,000 baht (49.88%), 25,001-35,000 baht (24.94%), 35,001-45,000 (7.41%), 45,001-50,000 baht (3.46%) and above 50,000 baht (4.94%). The researcher had hypothesized and analyzed the influential factors affecting online shopping decisions. From the analysis of the study, the results had proved that the different ages, gender, educational background, and monthly income had no effects on making a decision on buying online. This is because everyone can access to the internet and smartphone in Thailand, and people can access to the information online equally. However, the different occupation still has effects on online purchase because the consumers’ personal interests are different, and their responsibility toward their occupation might be an effect on online purchasing. For other hypotheses, the results showed that the package of products, price, delivery channel, marketing campaign, attitudes, and acceptance of technology, security, and trustworthiness and services have positive effects on buying-decisions.Swati Sachdeva Khosla [8] studies about the impact of psychological risk factors on the buying decisions of the consumers by using factors, filtering elements, and filtering behavior model as a theoretical framework. He focusses on the filtering elements part; security, privacy and trust, and trustworthiness, as the most possible concern for customers who shop online. He had conduct questionnaire surveys consisted of Likert’s five-point scale for measuring attitudes and behavior of 500 participants. The result of his study shows that the first factor that consumers most concerned about is privacy while buying online. These items include no possibility to touch the products, their credit card details, and their fear of losing social contact. The second factor is security, which is the fear of not getting the delivery on time after the payment. The last factor that they concern is trustworthiness, which is the quality of the product, the anxiety of false information online, fear of unmatched of actual products, and the shown-on website and anxiety of choosing the wrong products. In conclusion, various external and internal factors affect consumer behavior, but in the case of online shopping, the filtering elements filter the buying motives of consumers.According to Sunisa Throngjit [9], the researcher of factors affecting the decision on online purchasing through E-marketplace, several factors could be able to influence the buying decision of consumers. The researcher had used the five theories, demographic theory, consumer behavior theory, 6Ps marketing theory, quality of web service theory, and brand loyalty and brand awareness theory to analyze affecting factors on online purchasing decisions. The number of collected surveys is 417 surveys of participants who had ever experienced online purchasing through E-marketplace. Regarding the result of demographic theory, the differences in gender, age, marital status, occupation, and monthly income had not affected to an online purchasing decision. However, the difference in education affects online purchasing decision because the higher education consumers have, the easier to access online platform for online shopping. For the other factors such as web efficiency, privacy, brand royalty, marketing promotion, product, brand awareness, price, and product variety, Throngjit concluded that these factors had statistically significant at p-value equals to 0.000. These results implied that each independent variable has a direct relation with the online purchasing decision. However, the only variable that does not statistically affect online purchasing decision is the ability to find a new website replacement, because the statistically significant equals to .651, which is higher than 0.05. In conclusion, Miss Throngjit would suggest all companies related to the E-marketplace platform to concern all these affecting variables since it could directly affect consumers’ experiences and expectations of online shopping.
3. Research Methodology
The methodology for this paper is qualitative and quantitative analysis, using survey questionnaires as tools for collecting data. Survey questions will be about general information of participants (demographic information and socio-economic characteristic) and opinions towards online shopping (influential factors and others) to identify the relationship to an online purchasing decision.ParticipantsTargeted participants will be Thai people who live in Bangkok and have online shopping experiences by E-marketplace. The reason for choosing Bangkok as the target city because Bangkok is the capital of Thailand, and people in Bangkok adapt and use the internet and technology in their daily living. The number of participants is calculated from Cochran’s sample size formula [10].
n0 = the sample sizeZ2 = the abscissa of the normal curve that cuts off an area α at the tails (1 - α equals the desired confidence level, e.g., 95%, Z value will equal to 1.96)e = the desired level of precision,p = the estimated proportion of an attribute that is present in the population, q =1-p. As mentioned, we want to calculate a sample size of a large population by assuming the maximum variability, which is equal to 50% (p =0.5) and taking 95% confidence level with ±5% precision the calculation for required sample size will be
From the calculation, the total number of participants should be 384 participants for survey questions. Nevertheless, to prevent the information lost and incomplete information, I would like to collect 460 surveys for this research, which is approximately 20 percent of the calculated participants number. Participants selection methods will be random sampling selection by using districts in Bangkok as an edge of these surveys. There are 51 districts in Bangkok; however, the researcher would like to select eight districts for selecting survey information since these eight districts are the highest population density area in Bangkok. 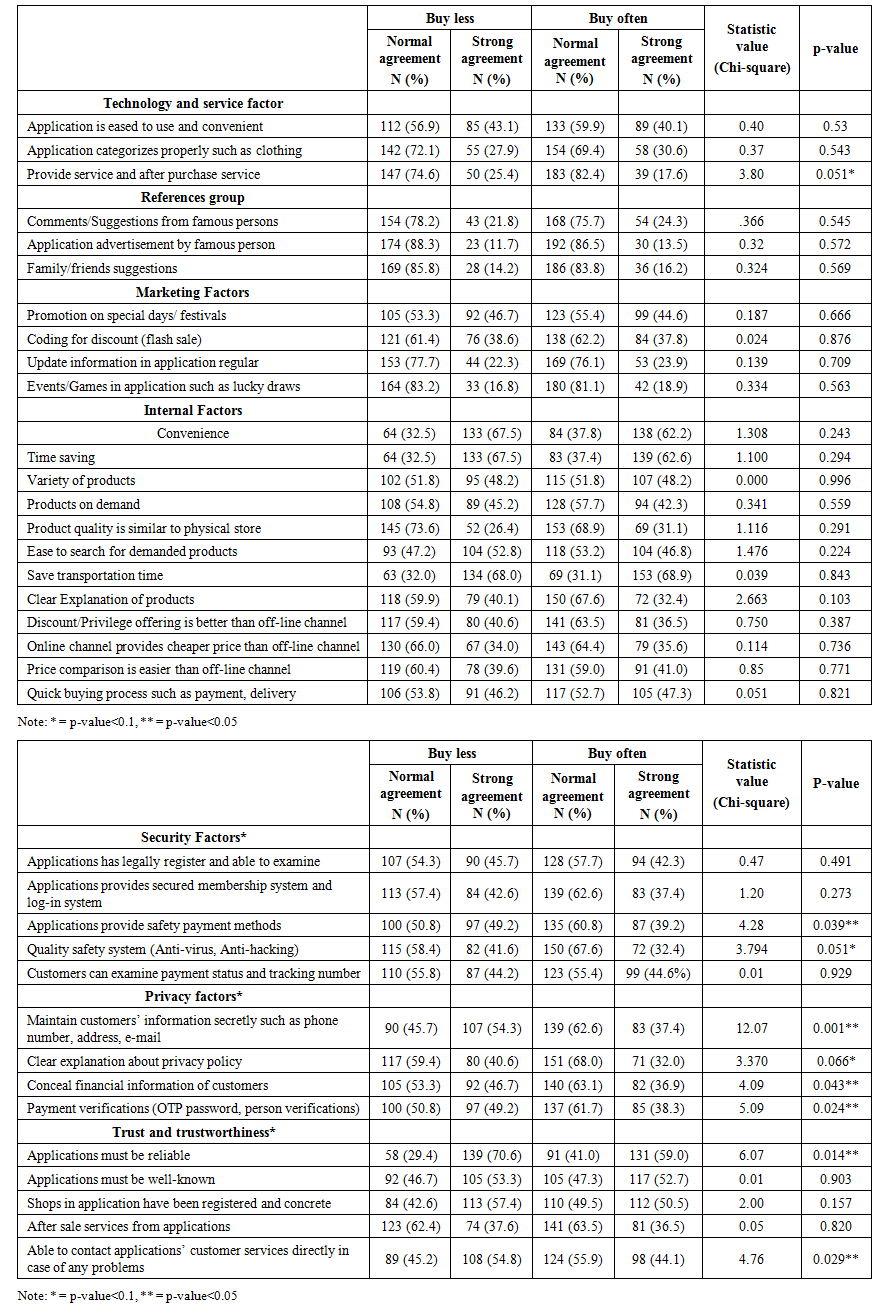 | Table 2. External factors, Internal factors and filtering elements’ s statistic value |
Data analysisThere are 419 from 480 or about 87.29% that have complete and enough data to be analyzed, which all data will be described with descriptive statistics. As everyone from the surveys has ever done shopping online before, two major groups are divided among them; regular customers who buy over one time per month, and the regular customers who buy less than one time per month. Then, Chi’s square and Fisher’s exact test is applied to find an association between Demographic Data and the habits of regular customers. Next, regarding surveys about internal and external factors, the point is ranked from 1 to 5 when 5 is the highest score. Chi’ square and Fisher’s exact test are used here to study for the group who do a lot of online shopping and the group who do less online shopping whether they share the same opinions about the factors or not. Lastly, all demographic data and factors will be analyzed by Univariate Binary Logistic regression, in order to study how each factor or the level of association can affect to the group who buy a lot and the group who buy less. All in all, every factor is statistically and theoretically significant that they will be brought to the analytics in the Multivariate Binary Logistic regression model, as shown below.
4. Result of Study
The total of the sample population is 275 females, 65.65%, and 144 males 34.36%. The average age of total sample participants is 28.36 years old, with a standard deviation of 10.24. The marital status of total participants is single (77.08%), married (20.28%), and divorced (2.62%). The occupations of total participants are none (5.96%), freelance (12.64%), students (32.69%), officers (19.57%), government officers (9.78%), and business owners (19.33%). The hometowns of total participants are Bangkok (51.78%), Northern (9.06%), Central (19.09%), North East (12.17%), Southern (4.53%), and others (2.38%). The current address of total participants is at Bangkok Noi (12.88%), Klong Sam Wa (10.26%), Lat Kra Bang (13.84%), Sai Mai (13.36%), Don Muang (12.88%), Bang Khun Thien (12.41%), Siam (11.93%) and Jatujak (12.41%). The educational backgrounds of total participants are primary (3.34%), high school (28.63%), Bachelor’s (58.71%), Master’s (7.15%) and Ph.D. (1.67%). The average income of total participants is 19,558.2 baht, with the standard deviation of 16.236.Regarding to Chi-square and Univariate regression analysis, the results shows that gender has statistically significant affected online shopping decision, which means female has higher opportunity to be in high frequency of buying group than male by 1.617 times. The marital status does not statistically affect online shopping decision. By using univariate regression analysis, comparing to divorced group as a dummy variable, the single and married group have higher opportunity to be in high frequency of buying group by 1.212 and 1.635 times; however, it is not statistically significant at 0.005. The different in occupation does affect online shopping decision statistically. Comparing to business owner’s occupation, unemployed, government officers have high opportunity to be in high frequency of buying group by 3.214 and 3.021 times. Other occupations also have higher opportunity to be in high frequency of buying group at 1.204, 1.306 and 1.378 times, comparing to business owners’ group. The hometown slightly does not affect online purchasing decision. Comparing with Bangkok as a dummy variable, Northern has higher opportunity to be in high frequency of buying group by 1.504 times, while other hometowns have high opportunity to being low frequency of buying group. The current addresses have statistically and significantly affected consumer’s online shopping decision. Comparing with the Siam district, Bangkok Noi and Sai Mai districts have higher opportunity to be in high frequency of buying group. While other districts have higher opportunity to be in low frequency of buying group. For the educational background, it does affect online shopping decision. Comparing to high school or lower degree, Bachelor;s degree and Master’s degree or higher have high opportunity to be in low frequency of buying group with statistical significance at 0.005. The different age does not affect online purchasing decisions statistically; however, the study found that the increase in age for one year, the opportunity of online shopping increase by 1.008. The monthly income also affects online shopping decisions statistically, which means the growth of monthly income in every thousand baht, the higher the opportunity to increase the online shopping decision by 1.018. To analyze individual variables from external factors, internal factors, and filtered elements. The statistical study found that consumers in Bangkok concern about technology and services factors, particularly in services and after-sale service, with a statistical value of 3.80 and asymptotic significance (P-value) less than 0.100. In addition, the safety system, such as anti-virus and virus protection, is another consumers’ concern, at statistic value at 3.794 and P-value less than 0.100. The privacy policy also has a statistical significance value at 3.370 and a P-value less than 0.100, which consumers slightly concern about the privacy policy of application. Other factors that consumers concern, and they are statistically significant toward online shopping decisions at P-value less than 0.050. There are safety payment methods, concealing consumers’ financial information, payment verification, application’s reliability, concealing consumers’ personal information, and application’s customers' services. Analyzing all independent variables simultaneously by using Multivariate binary logistic regression analysis. To see which variables are the most concern for customers in the high frequency of buying group when analyzing all variables at the same time. The results showed that gender still is a crucial variable for consumers, and females have higher opportunities to be in high frequency of buying group than males. The monthly income is still the significant variable that affects online shopping decisions, but the exponential Beta had changed from 1.018 to 1.033. This means every increase in a monthly income of thousand baht, the increase in online shopping will be 1.033. The last factor that statistically affects online shopping decision is internal factors, which are the perception and attitudes of consumers toward online shopping decision. This implies an increase of one score in internal factors will increase the online shopping decision by 1.061. After using multivariate binary regression analysis, there are some changes in the significance of variables such as occupation, hometown, current address, education, and filter elements such as security factors, privacy factors, and trust and trustworthiness of application. The results from the analysis are consistent with the study of Baubonienė and Gulevičiūtė [11], researchers from Lithuania, their study is about E-commerce factors influencing consumers’ online shopping decision. The reasons consumers would like to shop online are internal factors such as convenience and simplicity as the first reason. For second reason is the lower price of products through online channels. Also, gender plays another significant variable affect online shopping decisions. However, the study is not consistent with the study of Miss Jutarat Kaidrasamee [5]. The study shows that the only variable affects online shopping decision is occupation, and there are no statistical association with age, gender, monthly income, and educational background.
5. Discussion
The increase of uses of smartphones and the internet impacts an individual’s daily life, also the shopping behavior. Many consumers start using online shopping channels for convenience and time-saving. Since anyone can access to the internet and use the online shopping application, there are some influential factors causing consumers whether to buy or not. From the study, the online consumers mostly are freelance, students, officers, government officers, and business owners with an average age at 20s years old and in 8 different districts in Bangkok. The researcher found that three main issues should be focused.Table 3. Influential factors on high frequency of online shopping decision by using Multivariate binary logistic regression analysis 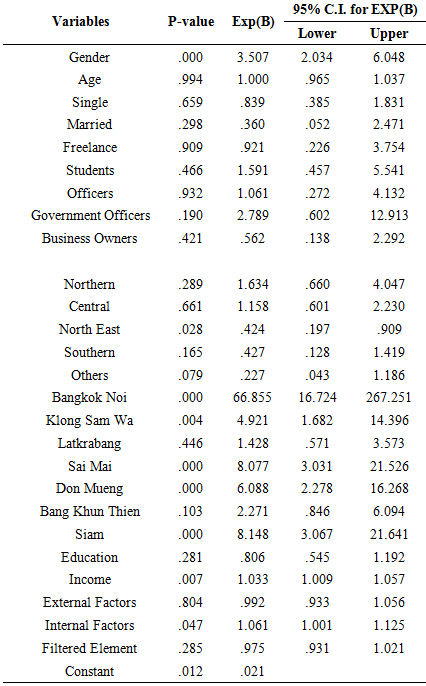 |
| |
|
Factors in demographic data that statistically affect online shopping decisions are gender, occupation, hometown, current address, educational background and income. To increase sale volume or introducing new products through online channels, online sellers and companies can use segmentation marketing, focusing on female consumers with the average age of 20s – 40s years old, who are living in Bangkok Noi district and Sai Mai district. Also, the study found that government officers have a high opportunity to purchase through online channels. The internal factors also statistically and significantly affect online shopping decisions. The perception and attitudes toward online shopping are significant factors to drive consumers’ motivation to shop online. The most concern variables from internal factors are convenience, time saving and save transportation cost. Companies and online sellers should provide convenient application like easy to use and easy to make a payment. Obviously, online shopping will save time and transportation cost for consumers; however, the companies and sellers should ensure that the delivery system and transportation are qualified and systemized. Thus, consumers can spend less time on checking and tracking delivered goods and products. This is because the perception and attitudes toward online shopping are really significant for high frequency of buying group; so, companies and sellers should increase or maintain positive feelings toward online shopping.The monthly income of consumers is another significant factor affecting online shopping decisions. Since the monthly income is a limited source in each month, consumers must allocate their resource efficiency. The price of the product will be the main motivation for online consumers to buy online because consumers would compare prices in both online channels and offline stores to obtain maximum profits they can get. Therefore, companies and online sellers should set reasonable prices to befit with the quality and quantity of products.
6. Conclusions
Online shopping channels have become a new alternative for people to purchase goods and services. Comparing offline shopping behavior, online shopping channels had changed consumers’ behavior in terms of variety of products alternative, price comparison, and expected quality of products. Consumers might have positive or negative experiences from online shopping. To maximize consumers’ satisfaction, studying of influential factors affecting online shopping decision is significant for companies and online sellers. The FFF model is designed for consumers’ online shopping behavior due to the filtered elements, security, privacy and trust and trustworthiness of application or online channels that consumers concern. To summarize all influential factors affecting online shopping decision, external factors (demographic information, socioeconomic, technology and services), internal factors (convenience, time-saving and transportation cost), security factors ( anti-virus system and payment methods), privacy factors (concealing personal information, financial information, payment verification and privacy policy), and trust and trustworthiness factors (reliability of application and application’s services) are the most concerned factors for consumers in Bangkok, Thailand.All in all, any factors could not be significant if consumers have negative attitudes and perception toward online shopping. Companies and online sellers should provide quality products and services to impress consumers and create positive experiences and perception toward online shopping. Thus, consumers will have trust in online shopping channels.
Note
1. To put out a tender means to ask companies to say formally at what price they would like to charge for a project.
References
[1] | Nava-Macali J. (2016). Knowledge on the use and benefits of E-commerce. Global Advanced Research Journal of Management and Business Studies. Vol.5 No.5 pp.124-128. |
[2] | Commerceland, n.d. History of E-commerce. Retrieved 2019, August from https://www.ecommerce-land.com/index.html. |
[3] | Office of the National Economic and Social Development council of Thailand (2019). Gross Domestic Product: Q1/2019. National Account Division, Thailand. |
[4] | Languepin Olivier, 2019 February. Thai e-Commerce shoots up to 3.2 trillion baht in 2018. Retrieved 2019, August from https://soso.bz/SqnZwQt. |
[5] | Electronic Transaction Development Agency, ETDA (2019). E-commerce value in Thailand. Electronic Transaction Development Agency, Thailand. <www.etda.or.th>. |
[6] | Dr. Ujwala Dange and Prof. Vinay Kumar, 2017. A study of factors affecting online buying behavior: A conceptual model. Retrieved from http://ssrn.com/abstract=2285350. |
[7] | Jutarat Kaidrasamee, 2015. Factor affecting the decision making on purchasing products from the online application in Bangkok metropolitan region. An independent study. Faculty of Commerce and Accountancy, Thammasat University, Thailand. |
[8] | Swati Sachdeva Khosla (2018). Impact of Psychological Risk Factors on the Buying Decisions of the Consumers. International Journal of Management Studies. Vol.5, Issue 3. pp. 54-59. |
[9] | Sunisa Throngjit (2016). Factors affecting decision on the online purchasing through E-marketplace. Thailand, An independent study. Faculty of Commerce and Accountancy, Thammasat University. |
[10] | Glenn D. Israel (2003). Determining Sample Size. University of Florida, United State and. |
[11] | Živilė Baubonienė, Gintarė Gulevičiūtė (2015). E-commerce factors influencing consumers online shopping decision. Social Technology. Vol.5, pp.74-81. |