Aronu C. O.1, Ogbogbo G. O.2, Bilesanmi A. O.1
1Department of statistics Nnamdi Azikiwe University, Awka, Nigeria
2Department of statistics Delta State Polytechnic, Oghara, Nigeria
Correspondence to: Aronu C. O., Department of statistics Nnamdi Azikiwe University, Awka, Nigeria.
Email: |  |
Copyright © 2012 Scientific & Academic Publishing. All Rights Reserved.
Abstract
This study is on determining the risk of survival of commercial banks in Nigeria using Survival Analysis. Survival analysis involves the modelling of time to event data; where death or failure is considered an event in the survival analysis literature. The survival analysis can be used for socio-economic research to investigate complex phenomena such as unemployment, employment, inflation, supply and demand for bank loans, life expectancy of the products, the producer and consumer to mention a few. The source of data used in this study is secondary data obtained from financial reports, NDIC (Nigeria Deposit Insurance Corporation) and CBN (Central Bank of Nigeria) publications. From the findings it was observed that about 67% of the twenty five commercial banks that met the 25 billion Naira capitalization in year 2005 survived to year 2011. Also, it was observed that the explanatroy variables Merger history, Age of operation in Nigeria, Revenue and Asset has no significant contribution to the survival of the banks.
Keywords:
Capitalization, Merger, Age, Asset, Revenue, Inflation, Liquidation
Cite this paper: Aronu C. O., Ogbogbo G. O., Bilesanmi A. O., Determining the Survivorship of Commercial Banks in Nigeria, American Journal of Economics, Vol. 3 No. 4, 2013, pp. 185-190. doi: 10.5923/j.economics.20130304.01.
1. Introduction
The financial health of the banking industry in any nation is seen as an important prerequisite for economic stability, development and growth. As a consequence, the assessment of the financial condition of banks in any nation should be seen as a fundamental goal for regulators of the financial institution in that nation which Nigeria is not an exception[1]. Nigerian economy has so far been faced with national and global economic challenges and as such, the financial institutions, especially the banking sector has an option of sanitizing and restructuring its operational processes in order to survive the depressed economy, as well as embarking on a consolidation exercise which would have some wider structural effects on the industry and on the economy as a whole. Basically, banking is a service industry operated by human beings for the benefit of the general public while making returns to the shareholders;[2]. As such, it is natural that the services provided thereof by the industry cannot be 100% efficient; thus, there is always room for improvement. The banking sector in the third world economies has been grossly under managed when compared with their counterparts in the developed countries of the world. This has made it imperative for Nigerian banks to sanitize and restructure their operational processes so as to be in line with the global trends, and to survive the depressed economy.After the reform of Nigeria banking sector in 2004, which made way for changes in service delivery, merger and acquisition amongst banks in Nigeria. Mergers are commonplace in the banking sector of most developing countries of the world but just becoming prominent in Nigeria.[3], defined merger and acquisition as another way of saying survival of the fittest that is to say a bigger, more efficient, better-capitalized, more skilled industry and is primarily driven by Business motives or market forces and regulatory interventions.[4], queried if merger of banks so far in Nigeria has really enhanced growth and survival of the Banking sector in Nigeria. The issues therefore, which this study intend to address are whether merger has a significant contribution to survival of banks in the Nigeria commercial banking sector and the identification of some explanatory variables which may contribute significantly to the survival of the Nigerian Banks so far.
2. Notations and Methodology
The population of commercial deposit banks in Nigeria as at year 2004 are the 25 commercial deposit money banks that survived the N25 billion recapitalization exercises imposed by CBN (Central Bank of Nigeria) in year 2004. This population of commercial deposit banks that met the recapitalization imposed by the CBN was used in this study.The type of data used in this study is secondary data from financial reports, NDIC (Nigeria Deposit Insurance Corporation) and CBN (Central Bank of Nigeria) publications.
2.1. Methodology
The first use of survival analysis and duration models comes from medical research. Survival analysis involves the modelling of time to event data; where death or failure is considered an "event" in the survival analysis literature. “Although at the beginning the survival analysis was used to study death as an event specific to medical studies and demographical studies”;[5], explained that as from the 1970s statistical techniques such as survival analysis have been increasingly used in economics and social sciences. Besides the fact that survival data are not normally distributed, they often contain incomplete information, censored subjects. Censoring and abnormality distribution of survival data raises specific methodological and statistical techniques required for an adequate analysis. Censoring of subjects may be on the right or left. It is vital to include censored subjects in the statistical analysis. The survival analysis can be used for socio-economic research to investigate complex phenomena such as unemployment, employment, inflation, supply and demand for bank loans, life expectancy of the products, the producer and consumer to mention a few. Survival analysis, adapted in conventional econometric modelling data, received the title of duration models in studies from[6];[7];[9];[10];[11];[12]. Using survivalanalysis involves the simultaneous observance of the following conditions: the researcher must analyze empirical data for all subjects in the study. Most commonly examination entails the specification of a linear-like model for the log hazard. In terms of the hazard function the model specifies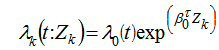 | (1) |
For given covariates Z, which could be treatment, age, sex, income, education, History of merger, revenue, Total Assets; where
an arbitrary unknown baseline hazard function and
denotes a p – dimensional vector of regression parameters. See Appendix for the conditional density equation and the survival function of T given Z mathematical forms.
2.1.1. Kaplan – Meier Method
The Kaplan-Meier method is a nonparametric technique for estimating time related events (the survivorship function). Ordinarily it is used to analyze death as an outcome, in biostatistics or medical statistics, but in recent years these techniques have also gained popularity in the social sciences or industrial statistics. A plot of the Kaplan-Meier estimate of the survival function is a series of horizontal steps of declining magnitude which, when a large enough sample is taken, approaches the true survival function for that population. The value of the survival function between successive distinct sampled observations is assumed to be constant. The survival function
is the cumulative survival probability that a bank will merge or be liquidated. Here
to denote the survival function of T, then  | (8) |
where T denotes survival time – duration of operating in the banking industry and F(t ) is distribution function of T ; F(t) measures the probability time of survival of the banks up to time t.
2.1.2. Cox Regression
Cox regression can be used to determine whether a characteristic of subjects affecting the survival and if so, how much and in what direction (to increase or decrease). Survival prediction can be difficult if not taking into account all factors that influence it. It is therefore necessary to identify those variables that affect the survival and that can be used in the calculation of a predictive indicator of survival. A method to determine such an indicator, and associated survival curve, is called Cox proportional-hazard regression. Cox proportional- hazard model is a semi-parametric method that enables one to determine the effect of different variables on the hazard. Assuming that we have "n" units’ individual under observation, then the model will have the form: | (9) |
Where,
is the vector of variables factor,
is the vector of regression coefficients,
is the hazard calculated for each individual i and
is the baseline hazard function which is the probability of liquidated banks when all the explanatory variables are zero.
2.2. Data Presentation
Table 1. Data on the 25 deposit money banks in Nigeria in year 2005 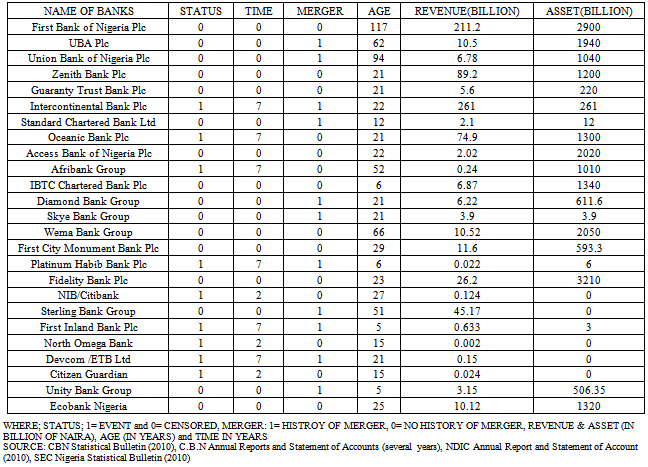 |
| |
|
3. Data Analysis
Using the data in Table 1 to run the Kaplan-Meier analysis and the Cox regression analysis respectively, the following output was obtained:
3.1. Kaplan-Meier
Table 2. Case Processing Summary 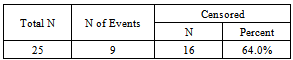 |
| |
|
Table 3. Survival Table 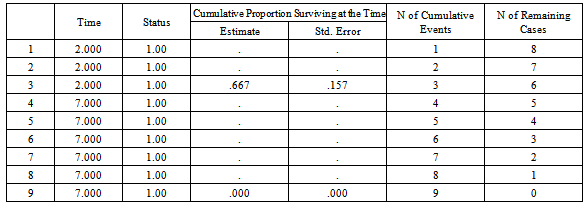 |
| |
|
Interpretation 1:From the survival function graph displayed above (Figure1), we can observe that about 80% of the banks survived at the 2.2 years of observation that is 2007 and some months while 67% of the banks survived to 2011 (i.e. 7 years of observation from 2005).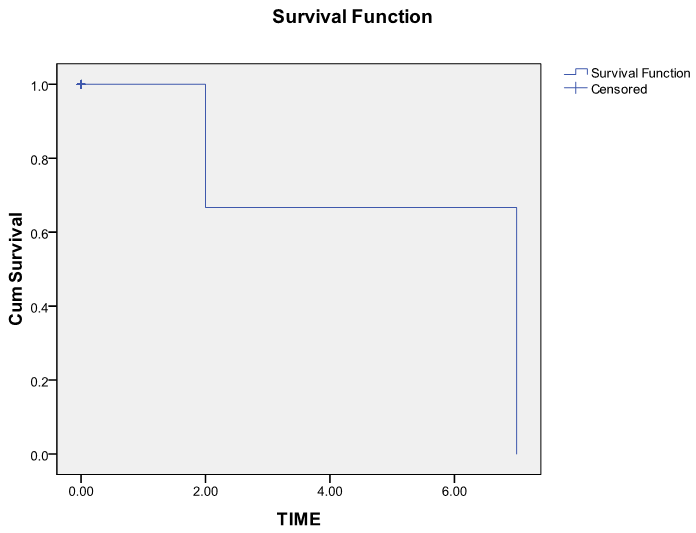 | Figure 1. Graphical expression of the Survival function |
3.2. Cox Regression
Table 4. Case Processing Summary 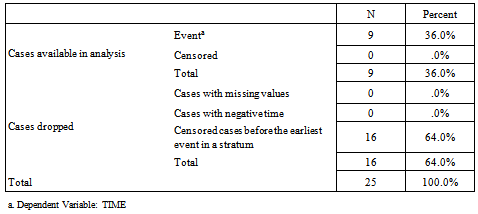 |
| |
|
Block 0: Beginning BlockTable 5. Omnibus Tests of Model Coefficients 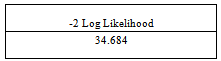 |
| |
|
Block 1: Method = EnterTable 6. Iteration Historyb 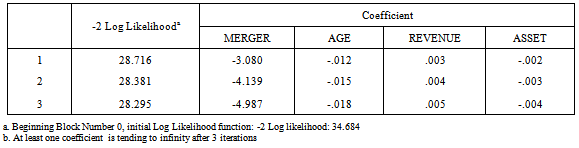 |
| |
|
Table 7. Omnibus Tests of Model Coefficientsa  |
| |
|
Table 8. Variables in the Equation  |
| |
|
| | Mean | MERGER | .444 | AGE | 20.444 | REVENUE | 37.455 | ASSET | 286.667 |
|
|
Interpretation 2:From the result of the Cox regression analysis presented above we can observe from “Block 0” (Table 5) that the value of -2log likelihood for the null model was obtained as 34.684. It was observed from Table 7 in Block 1, that there is no significant difference in assessing simultaneously the contribution of the explanatory variable in the model since the overall Score test value was given as
; it was equally observed from the omnibus test of model coefficient result that the addition of the explanatory variables improved the model in “Block 1” since there is a reduction in the value of -2 log likelihood = 28.295 relative to the -2 log likelihood = 34.684 in the null model of “Block 0”; from the result displayed in the Variables in Equation Table 8, it was observed that none of the explanatory variables contributed significantly to the risk of survival of the banks since there Wald test measure was obtained as 1.171, 0.247, 0.607 and 1.140 respectively and a corresponding p-value of 0.279, 0.619, 0.436 and 0.286 which falls on the acceptance region of the null hypothesis using 95% confidence interval; it was equally observed from the variables in the Equation result that if the variable Age is increased by a year the risk of liquidation of the banks is decreased by 1.8%, since Exp(B)= 0.982, in addition, the hazard risk of a bank liquidating is reduced by 99.3%, since Exp(B)= 0.007, if a bank move from not having history of Merger to having history of Merger. Also, it was observed that when Asset is increased by one unit the risk of hazard of survival of the banks is reduced by 0.4%, since Exp (B) = 0.996.
4. Conclusions
From the findings of the study as interpreted in section 3.1 and 3.2 respectively, it was observed that about 67% of the twenty five commercial banks that met the 25 billion Naira capitalization in year 2005 survived to year 2011. Also, it was observed that the explanotroy variables Merger history, Age of operation in Nigeria, Revenue and Asset has no significant contribution to the survival of the banks which gave answers to the question poised by[3] “does merger boost the survival and capital base of banks?” and[4] who queried if merger of banks so far in Nigeria has really enhanced growth and survival of the Banking sector in Nigeria. It was equally observed from the findings that if the Age is increased by a year the risk of liquidation of the banks is decreased by 1.8%; the hazard risk of a bank liquidating is reduced by 99.3%, if banks move from not having history of Merger to having history of Merger. Also, it was observed that when Asset is increased by one unit the risk of hazard of survival of the banks is reduced by 0.4%.
Appendix
Under the model (1), the conditional density and the survival function of T given Z have the forms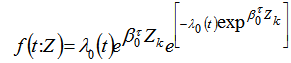 | (2) |
where,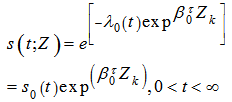 | (3) |
While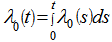 | (4) |
the baseline cumulative hazards function and 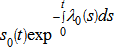 | (5) |
the baseline of the survival function. The conditional cumulative hazard function of T given Z has the form 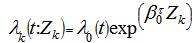 | (6) |
In the case of Z= 0 or 1, the ratio of the hazard functions under (1) has the form  | (7) |
This ratio represents that the covariates has multiplicative effects on the hazard function. The proportional hazards model has been commonly used in survival analysis. The main reason is availability of the partial likelihood approach proposed by[12]. The approach is efficient since the estimator of β is asymptotically equivalent to the estimator of β given by the full likelihood function.
References
[1] | Madubueze, J. A. Mega-Banking in Nigeria’s Business Environment: Emerging Issues. The Nigerian Banker – Journal of Chartered Institute of Bankers of Nigeria, January – March, 2007, Pp 46 – 54. |
[2] | Adegbaju, A. A. and Olokojo, F. O. Recapitalization, Mergers and Acquisition’ as Alternative Growth Strategy to Nigerian Firms under SAP Nigeria Institute of Management Seminar, Lagos, 2007. |
[3] | Umoren , A. O., Oyerinde, D. T. and Olokoyo, O. F. (2007). Merger And Acqiusition In Nigeria: Analysis Of Performance Pre-And Post Consolidation. Journal of Banking and Finance & Economic; Chumek Ventures, Lagos. 2007, Pp 151-157. |
[4] | Uffot, E. and Onwuamaeze, D. “A Matter of Survival” http://www.newswatchngr.com; 2010, Jan. O5 |
[5] | Danacica, D. and Babucea, A. Using Survival Analysis in Economics. Scientific Annals of the Alexandru Loan, Cuza University of Labsi: Economic Sciences Series, No. 1, 2010. |
[6] | Kiefer, N.M. Economic Duration Data and Hazard Functions. Journal of Economic Literature, 1988, 26: 646 - 679. |
[7] | Moffitt, R. A. New Developments in Econometric Methods for Labor Market Analysis. In: O. Ashenfelter, and D. Card (eds). Handbook of Labor Economics. Chapter 24: 1367-1397. 1999. |
[8] | Klein, J. P., and Moeschberger, M. L. Survival Analysis: Techniques for Censored and Truncated Data. Springer Verlag: New York, 2005. |
[9] | Santarelli, E. The Duration of New Firms in Banking: An Application of Cox Regression Analysis. Empirical Economics, 2000, 25: 315 – 325. |
[10] | Halling, M. and Hayden, E. Bank Failure Prediction: a Two – Step Survival Time Approach. IFC Bulletin; 2008, No. 28, Pp. 48 – 73. |
[11] | Andersen, P. k., Klein, J. P., Knudesen, K. M., and Tabaneray, P. Estimation of Variance in Cox’s Regression Model with Shared Gama Frailties. Biometrics, 1997, 53, 1475-1484. |
[12] | Cox, D. R. Regression Models and Life Tables (with discussion); Journal of the Royal Statistical Society Series B, 1972, 34, 187–220. |