Sankul Seth
AVP, Data, Analytics & Science, Pensacola, Florida, United States
Correspondence to: Sankul Seth, AVP, Data, Analytics & Science, Pensacola, Florida, United States.
Email: |  |
Copyright © 2023 The Author(s). Published by Scientific & Academic Publishing.
This work is licensed under the Creative Commons Attribution International License (CC BY).
http://creativecommons.org/licenses/by/4.0/

Abstract
In today’s data-driven world, organizations and consumers have high expectations for what data can accomplish for business growth, product offerings, and personalized customer experiences. This expectation stems from the vast amount of data consumers agree to share with the organizations they interact with and purchase from. Data can serve as a powerful tool when collected, organized, analyzed, and visualized with predictive modeling. As a business grows, so does the number of data sources it has in its ecosystem. Organizations can be held back by siloed data storage and technologies that limit their view of their company’s big picture. The solution is an integrated data platform that provides a one-stop, holistic view of an organization’s data.
Keywords:
Analytics, Big Data, Data Centers, Data Management, Data Science, Data Storage, Data Processing, Data Technology, Data Visualization, Enterprise Data, Integrated Data Platform, Machine Learning
Cite this paper: Sankul Seth, What Does the Next Generation of an Integrated Data Platform Look Like?, American Journal of Database Theory and Application, Vol. 7 No. 1, 2023, pp. 1-6. doi: 10.5923/j.database.20230701.01.
1. Introduction
It is hard to escape the longstanding statement that data is the new oil. A phrase first credited to mathematician Clive Humby, marketing leader Michael Palmer expanded on this assertion, stating, “Data is just like crude. It’s valuable, but if unrefined it cannot really be used. It has to be changed into gas, plastic, chemicals, etc. to create a valuable entity that drives profitable activity; so must data be broken down, analyzed for it to have value” [1]. These comparisons began in the days of early online data collection and only continue to grow as data becomes a primary focus for organizations looking to tap into new insights, improve their business activities, and maximize customer relationships. Because of this, there is a heightened need for data management architectures that can offer big-picture insights that turn data into valuable assets while emphasizing the importance of data protection and security in light of regulatory requirements. The question is: how does an organization tap into the massive value of data when there is more of it than ever? The answer: an integrated data platform. To tackle this need, it is essential for organizations to avoid the common pitfalls in designing and implementing integrated data platforms, such as a lack of clear goals, inexperienced leadership, inadequate talent, and failure to focus on scalability. After addressing these shortcomings, it is possible to create a targeted strategy to identify and achieve the key components of a successfully integrated data platform. These components include data science, storage, processing, visualization, data centers, big data, analytics, and machine learning. Each component is crucial in enabling organizations to extract value from their data, make informed decisions, and drive business growth. The rapid growth of artificial intelligence and related technologies will further enhance data’s accuracy and potential impact as an actionable resource [2]. Organizations that invest in next-generation technologies, such as cloud computing and open-source software, can gain a competitive edge by leveraging integrated data platforms.
2. The Current State of Data Management and What It Means for the Future
Effective data management is essential for organizations to unlock the full potential of their raw data and derive actionable insights. Managing data encompasses various aspects, including data collection, storage, organization, analysis, and governance. Organizations that manage their data effectively gain a competitive advantage by harnessing its value to understand customer behavior, optimize operations, and deliver personalized experiences. This ability to gather insights from data requires comprehensive technologies and skillsets that can organize, review, and derive actionable insights from past behaviors and activities. These insights are crucial in developing effective customer relationships and enhancing product experiences.There is a vital need for robust data infrastructure, reliable data governance frameworks, and advanced analytics capabilities to extract value from data. Additionally, organizations on the cutting-edge of methodologies and techniques for data evaluation, including data mining, statistical analysis, and predictive modeling, can uncover meaningful patterns and trends to set themselves apart from their competition. Innovative technologies, such as big data analytics, artificial intelligence (AI), and machine learning (ML), positively impact leading data management practices. These technologies provide organizations with powerful tools to process and analyze vast amounts of data, enabling them to make data-driven decisions and deliver personalized customer experiences at scale [3]. That said, when an organization’s data is spread across different teams, projects, and departments, data storage and analysis can become siloed, resulting in data insights that cannot incorporate the big picture. A more effective solution is an integrated data platform that can centralize the data spread across all departments, stakeholders, technologies, and channels to visualize past, present, and future outcomes founded on the most vital information from each source.
3. What Is an Integrated Data Platform?
The concept of an integrated data platform starts with the fundamental role of data integration. Data integration is the process of consolidating diverse forms of data from multiple sources into a single, centralized destination. This destination could be a database, data warehouse, or any desired location. Data integration enables firms to collect, review, and refine raw data from various sources in or beyond an organization to provide a 360-degree comprehensive and holistic view of the organizational data. With this widely informed point of view, organizations can leverage past, present, or future insights drawn from data to support and improve business value by offering a critical understanding of both operational and consumer needs. An integrated data platform takes integration a step further. It consolidates data from diverse sources into a centralized destination, often demanding translation and reorganization from different interfaces. The integrated data platform does not simply store the information but transforms, re-organizes, analyzes, and interprets that data in its entirety, enabling organizations to gain a complete view of their data in one place. It facilitates a broad view of data management, evaluation, and analysis, providing critical insights for improved business operations and personalized customer relationships. This type of platform may also be referred to as an “omnichannel” platform, as it takes all the channels of data moving in and out of an organization and puts it in one place. It can also overlap with an “optichannel” platform, which aims to optimize end-user functions through recommended actions based on their activity or persona [4].
3.1. Drivers for Organizations to Implement an Integrated Data Platform
While the concept of an integrated data platform is not entirely new, recent advancements in data intelligence and AI have brought it to the forefront of organizational growth. The drivers for implementation do not stop at rapidly evolving technologies. The need for data protection and security has become increasingly important, enforced by regulations such as General Data Protection Regulation (GDPR), California Consumer Privacy Act (CCPA), and other data compliance laws [5]. This has sparked discussions and debates around data privacy and the responsible use of personal data. Further, a centralized data platform results in better identity governance and breach protection. In fact, Gartner predicts that 30 percent of critical infrastructure organizations will experience a security breach by 2025, which doesn’t include additional risks for enterprises and small businesses. To mitigate these risks, experts such as Ruggero Contu, research director at Gartner, are reiterating the importance of a holistic approach to security so that information technology (IT,) operational technology (OT), and Internet of Things (IoT) security can be managed in a coordinated effort. “SRM leaders should accelerate efforts to discover, map and assess the security posture of all cyber-physical systems in their environment,” says Contu. “Invest in threat intelligence and join industry groups to stay apprised of security best practices, upcoming mandates and requests for inputs from government entities” [6].
4. In Action: Integrated Data Platforms Use Cases
Data helps create a clear picture of an organization’s operations, interactions, productivity, and efficiency, which means the benefits of an integrated data platform span every industry. From healthcare to finance to retail to manufacturing, the advantages of an integrated data platform are pervasive, making it an essential tool for organizations across diverse sectors. Relevant use cases can help illustrate how an integrated data platform can be leveraged to improve business growth and efficiency.
4.1. Use Case: Marketing Personalization
An omnichannel data platform can greatly enhance marketing personalization efforts for companies. By integrating data from various channels, such as website interactions, social media engagements, email communications, and purchase history, companies can gain a comprehensive view of each customer’s preferences, behaviors, and needs. This unified customer profile enables companies to deliver highly targeted and personalized marketing campaigns.For example, a retail company can use an omnichannel data platform to analyze a customer’s browsing history, previous purchases, and social media interactions. This information allows the company to create personalized recommendations and targeted promotions that align with the customer’s interests and preferences. This level of personalization enhances the customer experience, increases engagement, and improves the chances of conversion and repeat purchases [7].
4.2. Use Case: Improved AI Applications
The integration of AI tools within an omnichannel data platform provides numerous advantages. For one, it enables businesses to gain valuable insights and make real-time data-driven decisions. These AI tools can efficiently process and analyze the diverse datasets generated by different channels, extracting patterns, trends, and correlations that might not be easily identifiable through traditional methods. By leveraging AI, companies can derive meaningful and actionable insights from their data faster, leading to improved decision-making processes.The benefits of an omnichannel data platform extend beyond just analyzing data. The platform also plays a crucial role in training and refining AI models. AI algorithms rely on vast amounts of high-quality data to learn and make accurate predictions or recommendations. By integrating data from multiple channels and touchpoints, the platform provides a rich and comprehensive dataset that can be used to train AI models. This diverse data set enhances the accuracy and effectiveness of AI-driven applications, as it captures a more encompassing view of customer behavior and preferences.There is a symbiotic relationship between an omnichannel data platform and AI tools. AI tools enhance the platform’s capabilities by rapidly analyzing data and extracting valuable insights while the platform contributes to the growth and improvement of AI models by providing the necessary data for training and refinement. This feedback loop allows businesses to optimize their AI models continuously, ensuring they remain effective and aligned with evolving customer needs and behaviors [8].
5. Design and Implementation of Integrated Data Platforms Mistakes
There are significant business intelligence (BI) challenges facing organizations today, and they include combining information from many sources, poor data quality, lack of data talent, and poor presentation of data [9]. Developing and implementing a successful BI strategy in tandem with an integrated data platform result in a successful set of methodologies, processes, architectures, and technologies that transform raw data into meaningful and useful information for business purposes. To do so, it is essential for organizations to avoid these four mistakes.
5.1. Mistake One: Not Identifying and Documenting Goals
The first mistake is investing in technologies before clearly identifying and documenting goals in tandem with the overall business model. When organizations are concerned about keeping up with the competition, it is easy to think that adopting the newest technologies is one way to stay ahead. This simply is not true. Mindlessly adopting technologies without a strategy or the talent to back them up leads to more problems than solutions. It is more important to strategically align technologies with the overall business model and customer-centric goals. This is accomplished with a clear understanding of the organization's objectives, challenges, and opportunities informed by all levels of that organization, including leadership, operations, and IT departments, before selecting and implementing specific technologies. By defining goals upfront, organizations and their IT teams can better identify their integrated data platform’s necessary functionalities and features. This approach ensures that the chosen technologies align with their strategic objectives and enables them to derive maximum value from their data assets.
5.2. Mistake Two: Lack of Leadership Experience and Visibility
The second mistake is lacking a leader with the right experience and exposure to champion data integration with a centralized platform. Successful implementation of an integrated data platform requires strong leadership backed by the skillsets and know-how to create a viable strategy, foster alignment across the organization, and tackle roadblocks with a committed vision when they arise. A leader who understands the complexities and challenges associated with data integration can guide the organization in making informed decisions and driving the implementation process effectively. This leader should deeply understand data management principles, emerging technologies, and industry best practices. With their leaders’ guidance, organizations can implement technology solutions that meet market standards and align with their goals [10].
5.3. Mistake Three: Lack of Talent
Another mistake organizations make is that they do not have the right talent who knows how to design and implement the technology to the market standard and in the context of the organization’s goals. A leader is a vital cog in the machine, but they’re still only one crux in a much larger ecosystem of an organization’s data. They require a team of knowledgeable, adaptable, and dedicated individuals who can make the leader’s vision a reality. That said, it is a common mistake for organizations to underinvest in skilled talent with the necessary expertise in data integration, data management, and related technologies. Organizations need individuals who understand the complexities of integrating diverse data sources, can design efficient data pipelines, and know current data quality and governance standards. By acquiring or developing the right talent, organizations can ensure that the integrated data platform is implemented effectively and optimized for their specific needs.
5.4. Mistake Four: Inability to Scale
The last mistake is the inability to manage growing data as the business and channels grow. Data seldom decreases. As businesses and their channels grow, data volume, variety, and velocity also increase. Even the most effective integrated data platform can suffer when organizations do not address the ongoing need for internal review and improvement when a business is expanding, pivoting, or adapting to the market. It is vital for organizations to have scalable infrastructure and data management practices that can handle the growing data volumes. This includes implementing robust data storage solutions, optimizing data processing capabilities, and ensuring data governance and compliance. By proactively managing growing data, organizations can maintain the performance and efficiency of their integrated data platforms as their business and channels continue to expand.
6. Segments of a Successful Integrated Data Platform
In today’s data-driven business landscape, enterprise organizations face the challenge of managing and extracting value from vast amounts of data. To address this challenge, they rely on various components and technologies that form the foundation of an integrated data platform. These components include data science, data storage, data processing, data visualization, data centers, big data, analytics, and ML (Figure 1).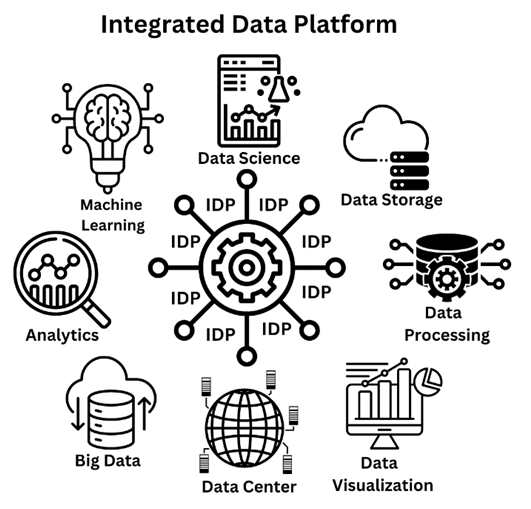 | Figure 1. Integrated Data Platform Components. Image courtesy of author |
6.1. Data Science
Data science is an interdisciplinary field that combines statistics, mathematics, computer science, and domain expertise to extract insights and knowledge from data. It involves techniques such as data mining, data analysis, and predictive modeling [11]. Data science is essential for enterprise businesses as it enables them to uncover patterns, trends, and correlations within their internal and customer data, leading to data-driven decision-making, improved operational efficiency, enhanced business strategies, and better customer relationships.
6.2. Data Storage
Data storage refers to the process of capturing, organizing, and persistently storing data in a structured manner to handle vast amounts of data from various sources. Efficient data storage solutions, such as databases and data warehouses, are crucial for enterprises to store their data securely and reliably. Proper data storage ensures data accessibility, integrity, and availability, facilitating timely and accurate data analysis, decision-making, and compliance with data regulations.
6.3. Data Processing
Data processing involves transforming raw data into a more meaningful and usable format. It encompasses activities such as data cleaning, integration, aggregation, and data transformation. This is where that “crude oil” becomes “refined,” according to Palmer’s analogy [1]. Enterprise businesses rely on data processing to derive valuable insights from their data and prepare it for analysis. Effective data processing improves data quality, reduces redundancy, translates terms from one department to the next, and enables seamless integration of disparate data sources, facilitating comprehensive analysis and informed decision-making.
6.4. Data Visualization
Data visualization refers to the graphical representation of data to communicate complex information in a visual and intuitive manner. It involves creating charts, graphs, dashboards, and other visual elements that enable users to interpret and understand data easily. Data visualization plays a crucial role in any organization as it allows stakeholders to gain insights from data quickly, identify patterns and trends, and communicate findings effectively. By presenting data visually, organizations can facilitate data-driven decision-making, improve data comprehension, and enhance communication across teams [12].
6.5. Data Center
A data center is a physical facility that houses computer systems, servers, networking equipment, and storage systems for managing and storing enterprise data. Data centers provide a secure and controlled environment to host critical IT infrastructure and ensure uninterrupted operation. They are vital to enterprise businesses as they offer scalable and reliable infrastructure to support data storage, processing, and management. Data centers also provide measures for data backup, disaster recovery, and data security, safeguarding the organization's valuable information assets.
6.6. Big Data
Big data refers to large and complex data sets that exceed the processing capabilities of traditional data management tools. It is characterized by the three V’s: volume (massive amounts of data), velocity (high speed at which data is generated), and variety (data from diverse sources and formats) [13]. Enterprise businesses encounter significant data challenges due to the exponential growth of data from internal and external sources. Big data technologies empower organizations to derive valuable insights and make informed decisions from vast and complex datasets by enabling distributed processing, scalable storage, flexible data integration, powerful analytics, and visualization capabilities.
6.7. Analytics
Analytics involves the exploration and examination of data to uncover meaningful patterns, correlations, and insights. It encompasses various techniques, such as statistical analysis, data mining, predictive modeling, and business intelligence. Analytics is crucial for enterprise businesses as it enables them to derive actionable insights from their data, make informed decisions, identify trends, optimize processes, and drive business growth. By leveraging analytics, organizations can uncover opportunities, mitigate risks, and gain a competitive edge in the marketplace [9].
6.8. Machine Learning
Machine learning (ML) is a subset of AI that focuses on developing algorithms and models that enable computers to learn from data and make predictions or take actions without explicit programming. It involves training models on historical data to identify patterns and make accurate predictions or decisions on new or unseen data. ML is important for enterprise businesses as it enables automation, enhances data analysis capabilities, enables personalized customer experiences, and improves operational efficiency. By leveraging ML within data needs, organizations can automate processes and low-added value tasks, optimize resource allocation and improve efficiencies, all while opening up time and energy for internal talent to focus on more demanding tasks such as problem-solving and innovation [8].
7. The Future of Integrated Data Platforms
The future of data management holds immense potential when founded on a core, integrated data platform. As organizations continue to collect and generate massive volumes of data, it is vital for these entities to emphasize the need for scalable and efficient data management systems. There is growing pressure from individuals, governments, and businesses alike to prioritize data privacy and security to address concerns around the responsible use of personal data, especially with the growing prevalence of natural language processing (NLP) [14]. As rapid advancements prevail, such as the integration of real-time data processing and the adoption of cloud-based data platforms, organizations will continue to leverage rising technologies to facilitate agile and scalable data management. Businesses that invest in integrated data platforms and next-generation tech, such as the cloud and open-source software, can veer away from legacy technology frameworks and gain a competitive edge. The rapid growth of AI and related technologies will accelerate data’s accuracy and potential impacts as an actionable resource. The overall impact of data insights trickles down from organizations to the individuals who operate within or utilize the services of that organization. As organizational uses of data increase, so do individual use cases. The repercussions of leveraged data in daily life will rapidly surge across many personal facets, such as finance, transportation, entertainment, household utilities, and personal devices [3].
8. Conclusions
For organizations to succeed in today’s data-driven marketplace, it is vital that they commit to data as the frontline for understanding their customers and operations. The next generation of an integrated data platform is crucial for organizations to strengthen that frontline and garner massive value from their data in today's business landscape. With the ever-increasing volume of data and the emphasis on data protection and security, an integrated data platform provides a centralized solution for data management, evaluation, and analysis. From there, organizations can extract value from their data, make informed decisions, and drive business growth. As organizations continue to invest in next-generation technologies, the accuracy and impact of data as a valuable resource will significantly improve. Advanced analytics, artificial intelligence, and cloud capabilities integrated into these platforms will revolutionize data interpretation and provide increasingly accurate predictive insights for strategic decision-making. Additionally, investing in advancements in data integration, data quality, and interoperability will further enhance the value derived from an integrated data platform. To remain competitive in the market, technology is only one part of the picture. Organizations across various industries must commit to a documented data strategy that combines the objectives of leadership and their expert data team. Without dedicated talent and leadership, organizations can struggle to achieve all key components of a robust data platform, including data science, data storage, data processing, data visualization, data centers, big data, analytics, and ML. From there, it’s possible to unlock the full potential of ceaseless data in tandem with overall business goals, enabling organizations to gain a competitive edge. By staying at the forefront of technological advancements, committing to best practices, and continuously evolving their data strategies, organizations can position themselves for success in the dynamic data-driven landscape of the future, where individuals expect personalized, seamless experiences. A robust integrated data platform acts as the foundation for any organization in any industry to commit to data-driven decisions, ongoing technological advancement, and sustained business growth.
References
[1] | Palmer, M. (2006, November). Data is the New Oil. ANA Marketing Maestros. https://ana.blogs.com/maestros/2006/11/data_is_the_new.html. |
[2] | Kumar, S. (2021) Data Intelligence and Planning using AI and Machine Learning with SAP Analytics Cloud - SAC. International Journal of Computer Trends and Technology, 69(2), pp 1-4. |
[3] | Lacroix, S., Ostermeyer, E., Le Duigou, J., Bornard, F., Rival, S., Mary, M.-F., & Eynard, B. (2023) Lessons learnt in industrial data platform integration. Procedia Computer Science, 217, pp 1660-1669. https://doi.org/10.1016/j.procs.2022.12.366. |
[4] | Kurris, M. (2021, July 2) Omnichannel Vs. Optichannel: Cutting the Price, Not the Precision. NG Data. https://ngdata.com/omnichannel-vs-optichannel/. |
[5] | Murray, C. (2023, April 23) U.S. Data Privacy Protection Laws: A Comprehensive Guide. Forbes. https://www.forbes.com/sites/conormurray/2023/04/21/us-data-privacy-protection-laws-a-comprehensive -guide/?sh=4b3ffea5f925. |
[6] | Moore, S. (2021, December 2) Gartner Predicts 30% of Critical Infrastructure Organizations Will Experience a Security Breach by 2025. Gartner. https://www.gartner.com/en/newsroom/press-releases/2021-12-2-gartner-predicts-30--of- critical-infrastructure-organi. |
[7] | Kim, T., Jo, H., Yhee, Y., et al. (2022) Robots, artificial intelligence, and service automation (RAISA) in hospitality: sentiment analysis of YouTube streaming data. Electronic Markets, 32, 259-275. https://doi.org/10.1007/s12525-021-00514-y. |
[8] | Agrawal, P., Arya, R., Bindal, A., Bhatia, S., Gagneja, A., Godlewski, J., Low, Y., Muss, T., Paliwal, M. M., Raman, S., Shah, V., Shen, B., Sugden, L., Zhao, K., & Wu, M.-C. (2019) Data Platform for Machine Learning. In Proceedings of the 2019 International Conference on Management of Data (SIGMOD '19) (pp. 1803-1816). Association for Computing Machinery. https://doi.org/10.1145/3299869.3314050. |
[9] | Castellanos, M., Dayal, U., et al. (2018) Enterprise Application Integration: Challenges, Opportunities and Roadmap for Future Research. Information Systems Frontiers, 20(4), 731-751. |
[10] | Carillo, K. D. A., et al. (2019) How to Turn Managers into Data-driven Decision Makers: Measuring Attitudes towards Business Analytics. Business Process Management Journal, 25(3), 553-578. |
[11] | Vasant, D. (2013) Data science and prediction. Communications of the ACM, 56(12), pp 64–73. https://doi.org/10.1145/2500499. |
[12] | Dhar, V. (2013) Data science and prediction. Communications of the ACM, 56(12), 64-73. https://doi.org/10.1145/2500499. |
[13] | Laborde, R. (2020) The Three V's of Big Data: Volume, Velocity, and Variety. Oracle Life Sciences. https://blogs.oracle.com/life-sciences/post/the-three-vx27s-of-big-data-volume-velocity-and-variety. |
[14] | Baldwin, T., Leins, K., & Rogers, A. (2021) 'Just What do You Think You're Doing, Dave?' A Checklist for Responsible Data Use in NLP. ArXiv. https://arxiv.org/abs/2109.06598. |