Greg Clare, Nahide Hancer
Department of Design, Housing and Merchandising, Oklahoma State University, USA
Correspondence to: Greg Clare, Department of Design, Housing and Merchandising, Oklahoma State University, USA.
Email: |  |
Copyright © 2016 Scientific & Academic Publishing. All Rights Reserved.
This work is licensed under the Creative Commons Attribution International License (CC BY).
http://creativecommons.org/licenses/by/4.0/

Abstract
A novel approach to indexing eye tracking metrics was used to evaluate selected student apparel designs prior to an annual student fashion show at a large Midwestern university. Participants were asked to focus on areas of six garments that they believed were innovative. Eye tracking data including fixation and gaze metrics were then compared in 60 pairwise combinations and 240 image ranges. Significant differences in pairwise combinations of raw eye tracking data were found and these incidences were then aggregated to create an index of factors of perceived garment innovativeness.
Keywords:
Eye tracking, Consumer behavior, Apparel design
Cite this paper: Greg Clare, Nahide Hancer, Measuring the User Experience of Apparel Design Innovations with Eye Tracking with Multiple Metrics: Lessons from a Student Fashion Show, International Journal of Clothing Science, Vol. 3 No. 1, 2016, pp. 1-10. doi: 10.5923/j.clothing.20160301.01.
1. Introduction
A large Midwestern university conducts an annual fashion show to highlight student fashion design work. This study was intended to help students better understand consumer perceptions of their apparel designs and explored new ways of comparing behavioral patterns of groups of consumers that were assigned visual tasks in computer moderated communications. The study measured the perceived innovativeness of six garments which were presented prior to a runway fashion show. An eye tracking booth was set up in the lobby prior to the fashion show. Participants had not previously seen the garments presented during the eye tracking study. The goal of the study was to compare user experiences of student fashion designs by comparing 2D images in a uniform visual field, similar to browsing fashion images online. Each garment exposure was controlled for scale and placement on the screen. The researchers measured perceived innovation in fashion designs by asking participants to focus on parts of the garment that they perceived were innovative during a slideshow. The research approach could be utilized to help students evaluate their prototypical designs or existing products as part of a course curriculum in apparel design courses. Due to the proliferation of online browsing of fashions by consumers, computer moderated tests of user experience using eye tracking are particularly timely introductions to college classrooms as a means to measure perceived effectiveness of designs by consumers. Evolving technologies such as the Mirametrix S2, Tobii T60 eye tracker and NUIA eyeCharm device highlight the rapid advance in economical eye tracking solutions to gauge user experience in product design.
2. Literature Review
Customer co-creation in designMeasuring the user experience of prototypical apparel designs may benefit marketers by providing the ability to predict future adoption of the product when mass produced. Prior research has measured how consumers look at visual images of products with the goal of understanding consumer behavior (Park, Delong, & Woods, 2012; Rahulan et al., 2012). Most tracking research is qualitative in nature and ways to compare and combine eye tracking metrics within and between subjects and visual stimuli have not been explored extensively in the merchandising literature. The researchers suggest that predictive models be developed that allow students to simultaneously compare statistics from multiple eye tracking data metrics to provide qualitative inferences for further consumer research. Future technologies may be combined with eye tracking tests to explore the combination of haptic research tools like feeling fabric samples, point and click heat maps and eye tracking technology used in combination to test apparel designs both within and across subject groups. The combination of multiple measurement tools in addition to traditional survey data measuring garment preferences will provide multi-dimensional/attribute measurements of clothing designs with the role of reducing production risk. Creating a uniform visual field of fashion images and varying those images within a user eye tracking script which is then compared to related data from different research methods may support the underlying assumptions of data fusion theory (Hall & Llinas, 2001). For this study, the researchers wished to create a baseline comparisons of a single method (i.e. eye tracking) by aggregating multiple eye tracking metrics (saccades and fixations) with statistical comparisons. This approach created and index for garment evaluation. The implication of simultaneous multi-attribute apparel design modeling offers the potential to influence multiple levels of the value chain (Wedel & Pieters, 2008).Eye tracking overviewEye tracking technologies are used to measure movements of the eyes. How consumers look at visual stimuli can be quantified using two methods: saccades and fixations. Saccades demonstrate how the eyes move over stimuli and may be specified to collect a range of metrics for visual image scanning by consumers. Fixation measurement accounts for times when the eye is relatively stable and suggests that the consumer is processing information in the image more thoroughly (Wedel & Pieters, 2008). Computer moderated design analysis with eye tracking has been used for decades to measure user experience but there is a dearth of literature examining consumer experiences with fashion designs from eye tracking data. (Zangemeister et al., 1995; He et al., 2004; Wedel & Pieters, 2008; Holmqvist et al., 2011). Measuring perceived desirability of specific design characteristics may offer the opportunity in the future to modify garment characteristics, gauge interest, and predict a garments success before mass production (Rahulan, 2015). Raw data from eye tracking of apparel design variations may be used through multi-factor eye tracking metrics to create an indexing algorithm of eye tracking metric statistical comparisons. The use of eye tracking to measure prospective users experience of garment images might be useful for evaluating apparel oriented E-commerce websites, or even entire apparel lines through combination of passive (i.e. screen mounted) and active (i.e. eyeglass) eye tracking technologies. Consumer panels assembled by retailers could routinely evaluate apparel product lines (e.g. private label and national brands) before finalizing production and store orders. For this pilot study, the researchers controlled evaluation of the garment images by specifying that participants looked at portions of the garment (top versus bottom) that they believed were innovative using a passive eye tracking approach. The researchers limited the ranges per garment to front and back, top and bottom to reduce the statistical complexity of the analysis and provide baseline data for future comparisons of the approach. By directing consumer focus on and evaluation of two regions of the garment to assess design innovativeness supports current neuroscience research suggesting that consumers follow a hierarchy of evaluation from lower to higher levels of conscious perception of visual stimuli that requires cognitive comparisons. (Vogt et al., 2010; Tusche et al., 2010; Pieters & Wedel, 2012) The researchers propose that development of meta cognitive algorithmic repeated measure design indices from analysis of combinations of eye tracking metrics may offer the potential to better understand consumer thought processes when assigned a product evaluation and comparison task. Product evaluations offer additional benefits at the garment prototype stage such as student fashion designs. By engaging multiple consumers in evaluation of apparel design visual ranges in images highlighting targeted design elements (e.g. fabric, shape, texture), firms could create databases which assist in modeling consumer design perceptions over time by designer. Metacognitive design data might be used to influence designers based on consumer weighting of prior design evaluations before developing a new apparel line to increase probable success of mass market goods. Tools such as metacognitive apparel design databases may help mitigate risks in mass market production of fashion designs by introducing consumer co-creation and modification as part of the design process. Low cost eye tracking technologies and increased delivery channels for consumer eye tracking afforded by the “Internet of Things” will support the development of material goods as byproducts of multi-user computer network interactions and real-time feedback (Gubbi et al., 2013). Industry pressures to proactively meet the needs of changing consumer tastes, maximize profits, and real time predictive analytics will require new technological innovations that help mitigate risk before a garment is mass produced. Researcher development of new computer moderated metacognitive design analytics tools support the need for additional research into developing low cost scalable consumer research systems. Training future generations of students to better understand and become engaged in addressing the challenges of designing for the end consumer through real-time pre-production design communications must be a top priority in fashion design schools and industry globally.
3. Methods
Six images of student fashion designs were used for the study based on an invitation to apparel design students to participate in the study. Four student’s designs were chosen for the study (garment 1 and 2, designer A; garment 3, designer B; garment 4 and 5, designer C and garment 6, designer D. Garment number four was selected as a control garment for innovativeness in the experimental group according to a faculty panel. The fashion designs were photographed on models with a black background; gray boxes were placed over the heads of the models in Adobe Photoshop and the models did not wear footwear to minimize distractions from the garments being evaluated. Both the front and back of the garments were photographed and then composited a single image of the garment including both the front and back for the eye tracking script (Figure 1).A convenience sample of 21 participants was recruited in the lobby outside the fashion show event who had purchased tickets to attend the annual fashion show. The characteristics of the sample are presented in Table 1. The participants completed informed consent documents followed by a short paper survey and were escorted to an eye tracking booth set up in the lobby area. No financial incentives were provided to participants of the study. The six resulting images were transferred to Mirametrix EyeMetrix software and an eye tracking script was created. The script duration for each of the six eye tracking images was 20 seconds during which participants evaluated the top and bottom of the garment for innovativeness. Each subject sat in front of a calibrated computer monitor with a MiraMetrix S2 passive eye tracking device positioned below the monitor. The subjects’ pupillary movements were then calibrated for eye tracking using MiraMetrix Record software. The researchers monitored participants eye movements during eye tracking on an adjacent monitor concealed from participants. The booth surrounding the monitor and eye tracking device were partially enclosed to help reduce participant distractions during data collection in the busy foyer area outside the fashion show.Table 1. Characteristics of the Sample 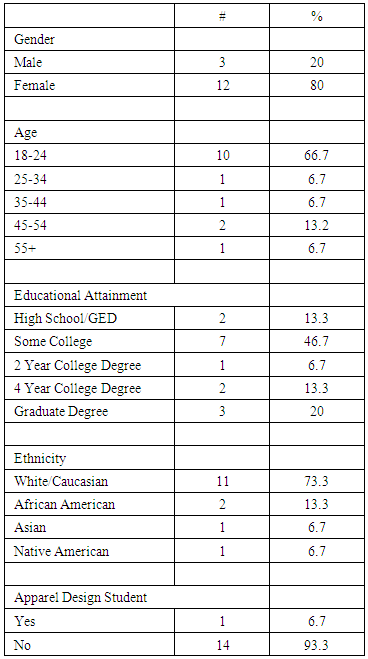 |
| |
|
Prior to commencing eye tracking procedures, participants read an onscreen instruction slide with instructions to focus on parts (front and back and top and bottom) of the following six garment images that they felt were innovative. Participants were also instructed to look at the regions surrounding the images (white space) if they felt there was nothing innovative about the garment being examined. A final instruction asked participants to verbally confirm their understanding of the instructions and say ‘begin’ when they were ready to start viewing the six garment images. After the six images were reviewed, participants were thanked for participating in the study and were seated in the fashion show auditorium.
4. Results
The characteristics of the sample are presented in Table 1. Six files were omitted from the analysis due to high eye tracking error rates which may have been compounded by distractions inherent in collecting eye tracking data in field settings which, resulted in a sample size of n=15 subjects with a mean error count M=62.24 and SD=87.96. Raw data from the eye tracking device was compiled in EyeMetrix Analyze software and then transferred to IBM SPSS version 21 software for analysis. Four visual ranges on each garment were specified in the EyeMetrix Analyze software consisting of the top/bottom= 2 and front/back=2 for each of the six garments. A pairwise comparison was completed in a 4X6 repeated exposure matrix comparing the region observations of each garment (e.g Garment 1 Top Front Side to Garments 2, 3, 4, 5, 6 Top Front Side et cetera). This method resulted in 60 pairwise comparisons per method tested: gaze observations, time to first gaze hit in each region, fixation in each region, and region fixation duration resulting in 240 range comparisons of perceived garment innovation measured. Within subjects t-test were used to compare the repeated measures of subject attention to component regions of the fashion design (top/bottom and front/back). This approach to analyzing eye tracking data may allow researchers to compare the magnitude of eye tracking signals for whole image review tasks and determine differences among the garment regions. Paired sample t-tests are useful when the data scores from eye tracking data are widely scattered based on diverse subject observations and durations. The accumulated percentages of gaze observations across all garments are presented in Figure 1. 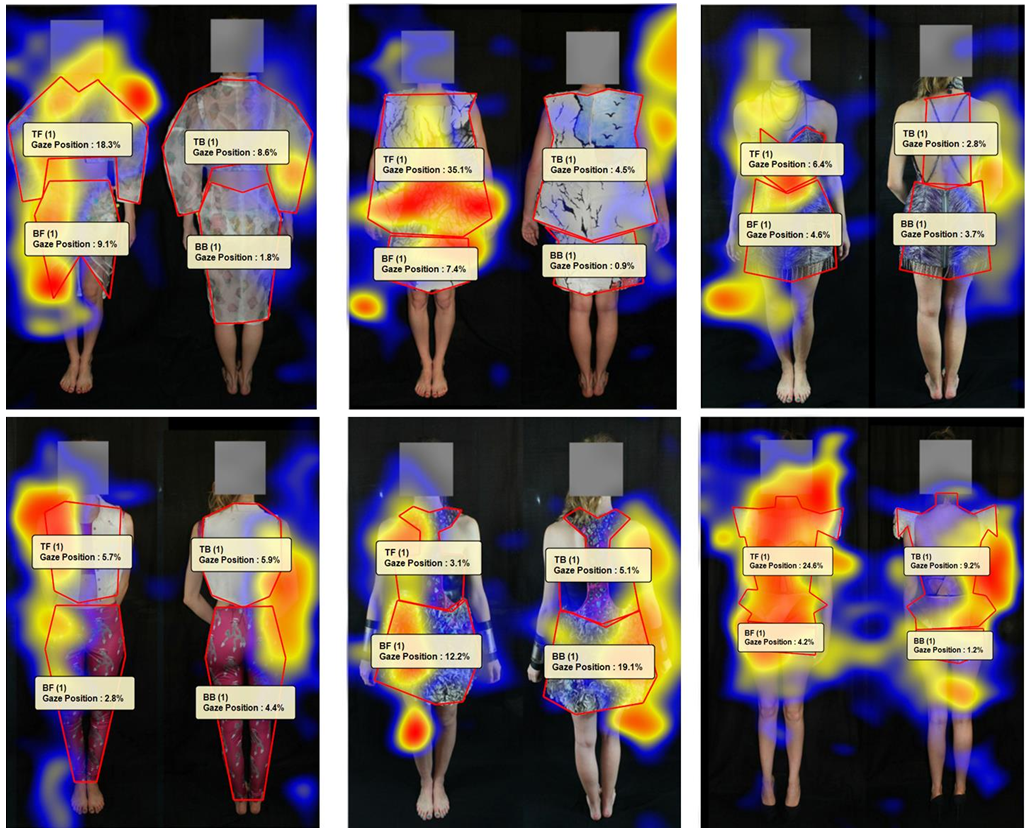 | Figure 1. Participant percentage of gaze observations per garment region, top/bottom and front and back of perceived garment innovativeness |
Significance differences were found in fourteen pairwise combinations of gaze observations among the garments (Figure 2/Table 2). Two significant differences were found in combinations of time to 1st gaze among the garments (Figure 3/Table 3). Eight significant differences were found in combinations of region fixation duration among the garments (Figure 4/Table 4), and eighteen significant differences in fixation in each region (Figure 5/Table 5). For purposes of this pilot study, the image exposures were not randomized and the order of garment viewing was consistent for each participant. Future studies will compare combinations from randomized and non-randomized scripts with a larger between groups sample. The results provide some evidence that subjects use a probabilistic mental model of assigning relevance to different regions of garments observed. Probabilistic mental models are measures of confidence in completing a general task such as evaluating garment innovativeness with confidence increasing through repetition of the task (Gigerenzer et al., 1991). Researchers aggregated the significant differences in observations from one garment compared to another and counting those incidences across all exposures. The resulting count data of significantly different regions between and within participants may be useful for comparing apparel designs, but further study is needed. The total count of significant differences between garment areas between and within subjects may offer an unweighted index of perceived garment innovativeness. The total from each method of eye tracking data may then be weighted by varying assigned tasks and allow creation of a composite score for garment design specifications such as innovativeness, design preferences, purchase intentions or other variable. However, the means to measure multiple garment evaluation factors of the same garments among a population may offer help designs evaluate and make modifications to new designs more quickly. A paired samples t-test was conducted to compare: gaze observations in each design region first gaze in each region, region fixation duration, and fixations in each region between the six garment images. A summary of significant differences between garments for each testing protocol may found in tables (2-5). Directionally significant mean differences between eye tracking tests may be found in Figure 6 which were summed to provide the novel garment design index summarized in figure 7. Anecdotally, the futuristic design may seem innovative to the casual observer, however additional research is needed to test the approach combined with other measures of consumer preferences such as click maps or survey measures. 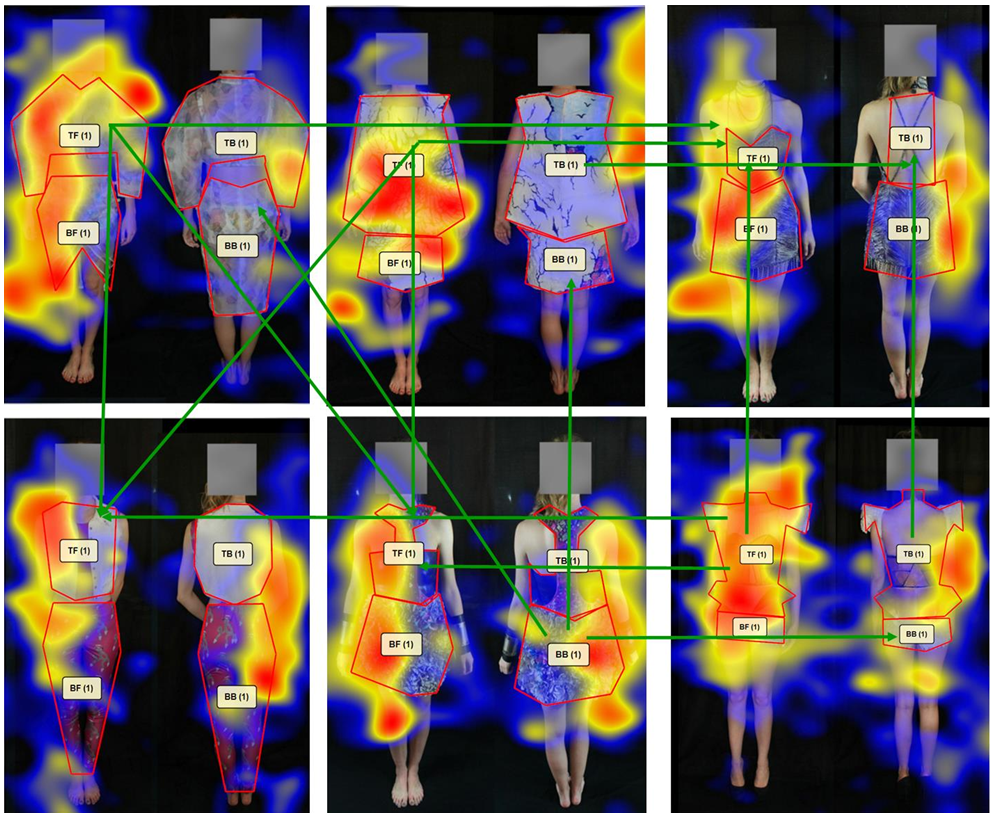 | Figure 2. Paired samples t-Tests showing directionally significant gaze observations of perceive garment innovativeness |
Table 2. Significant pairwise comparisons of gaze observations in each region 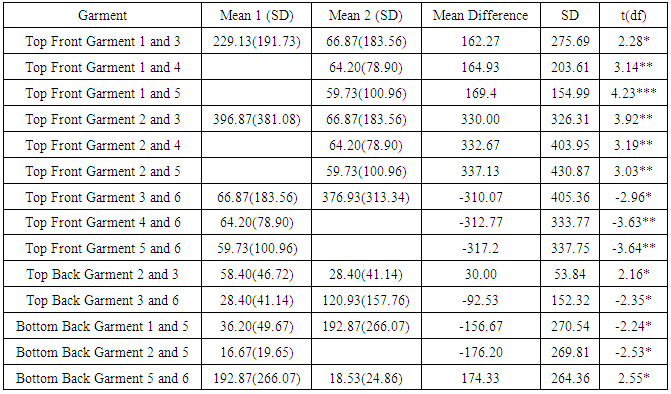 |
| |
|
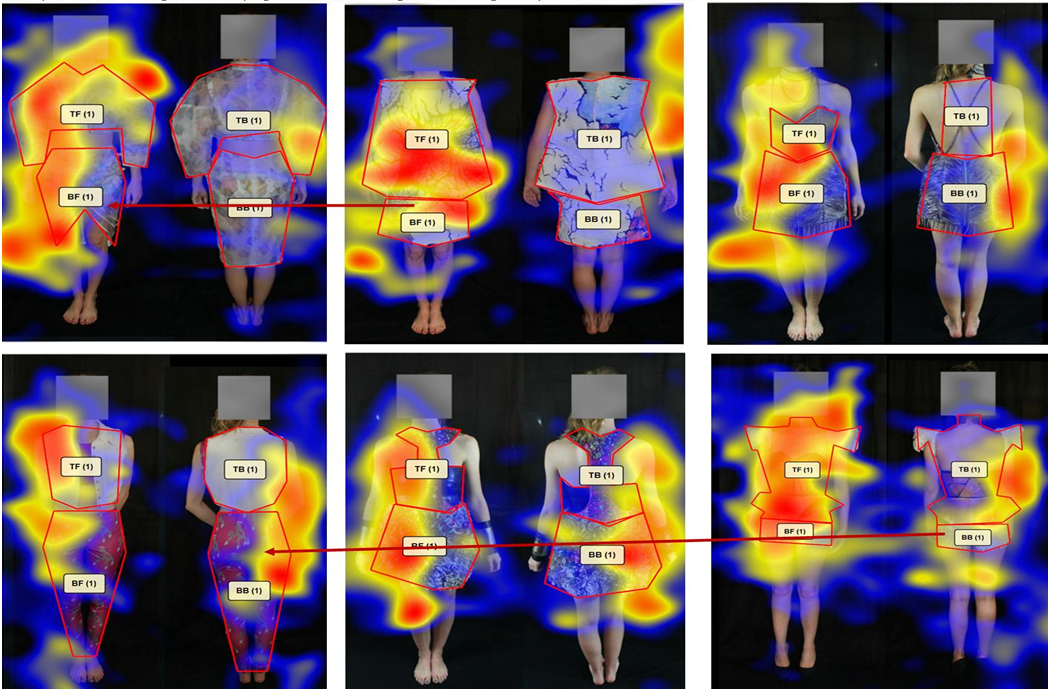 | Figure 3. Paired sample t-tests showing directionally significant time to first gaze for perceived garment innovativeness |
Table 3. Significant pairwise comparisons of time to first gaze in each region  |
| |
|
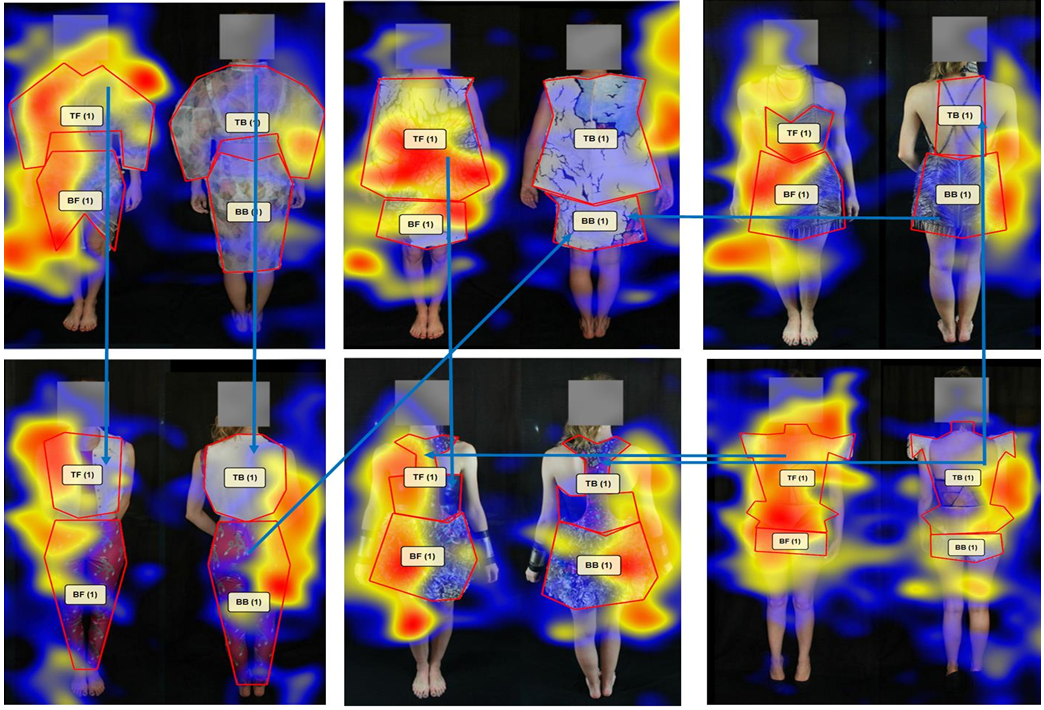 | Figure 4. Paired samples t-tests showing directionally significant region fixation duration of perceived garment innovativeness |
Table 4. Significant pairwise comparisons of garment region fixation duration 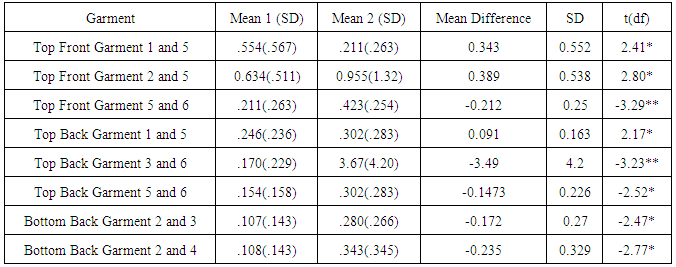 |
| |
|
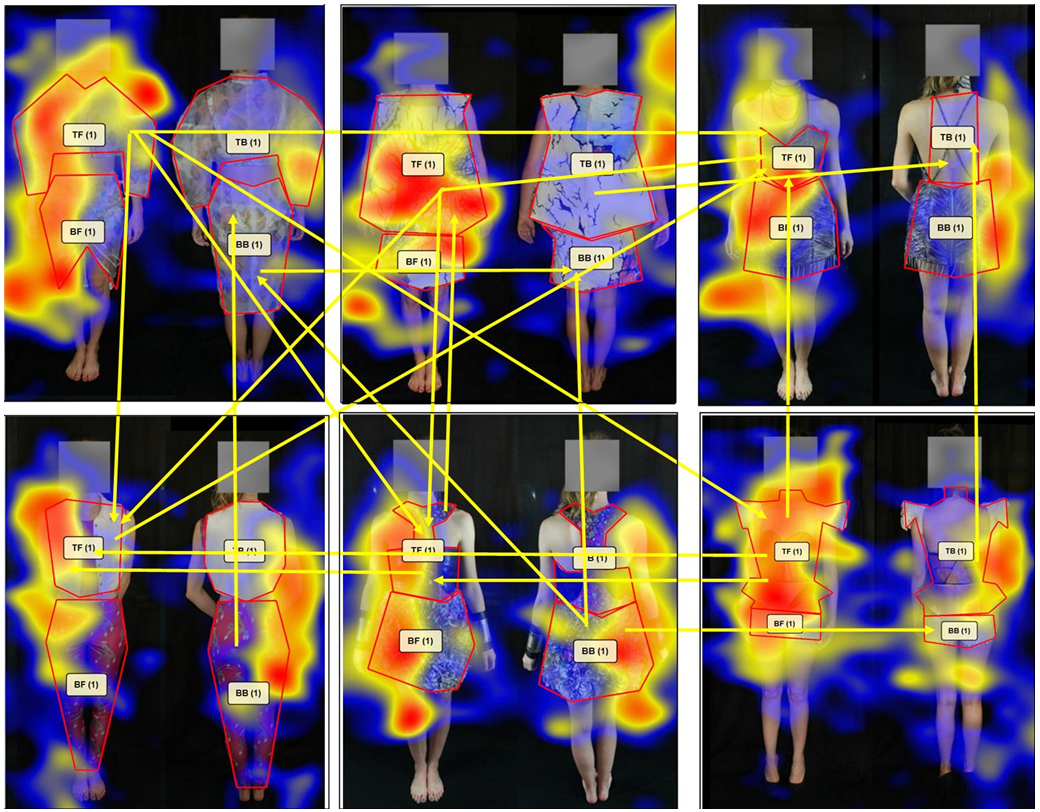 | Figure 5. Paired sample t-test showing directionally significant fixations in each region for perceived garment innovativeness |
Table 5. Significant pairwise comparisons of fixations in each region 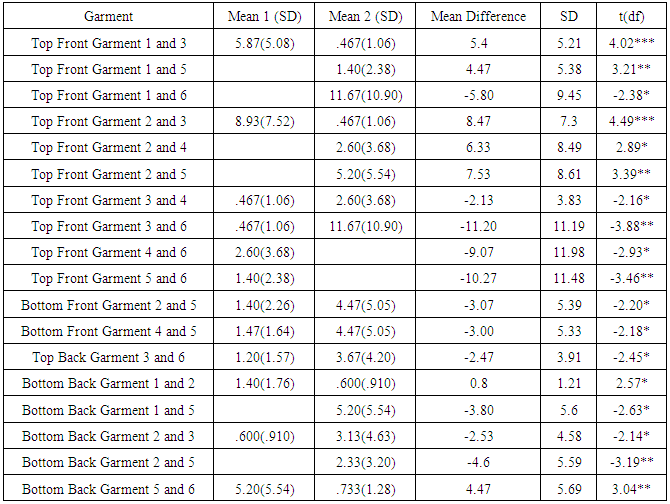 |
| |
|
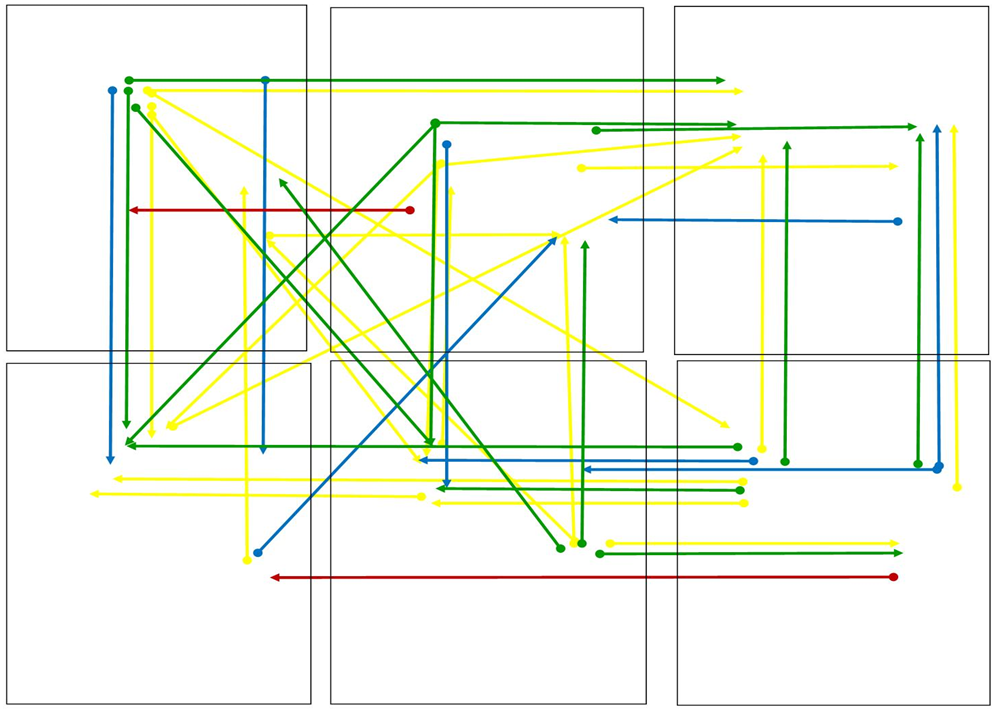 | Figure 6. Color-coded diagram showing directionally significant pairwise combinations of perceived garment innovativeness |
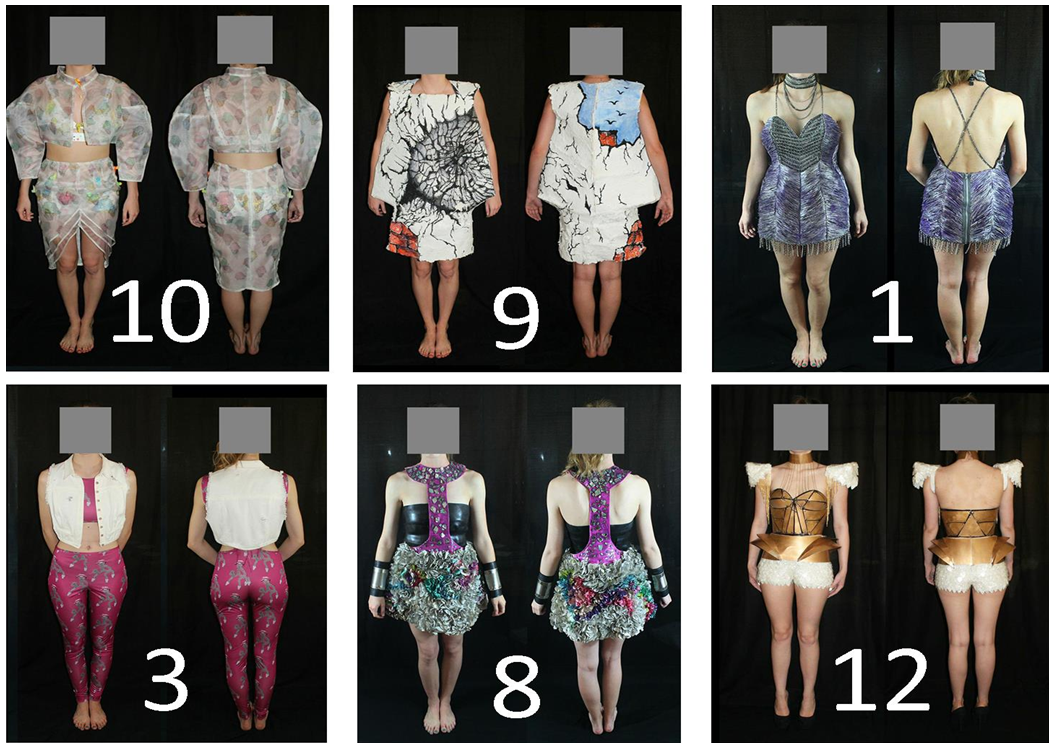 | Figure 7. Count of significant differences in pairwise combinations of repeated measures t-tests of fixation and gaze tests of perceived garment innovativeness |
5. Analysis and Conclusions
Eye tracking offers exciting opportunities to engage design students with the ultimate customers that may purchase their clothing designs. By learning how consumers view a design, early career refinements to design methods may be possible through the use of eye tracking laboratories at apparel production and design schools globally. The benefits to industry for pre and post-cognitive design processes offer the benefit of reducing risk in mass production and better prediction of consumer demand.While the findings of Figure 7 should be interpreted with caution for the assumption that garment six is the most innovative, despite face validity from the perspective of the researchers, the method offers promise for combining eye tracking metrics into composite scores to quantify the user’s experience in a prescribed set of research goals. However, equal caution should be exercised in selecting garment two as the most innovative design based solely on the gaze tracking statistics generated by EyeMetrix Analyze (Figures 1-2) from the aggregated subject heat map interpreted independently since each metric accounts for different observation criteria. More study is needed to assess the validity of compositing multiple eye tracking metrics across image comparisons among groups of consumers.The researchers believe that drawing conclusions from a single eye tracking metric among dozens available in eye tracking software suites may not provide the most holistic view of consumer opinion. While the findings remain qualitative in nature, they offer promising directions for weighting consumer responses through multiple comparisons of image viewing behaviors. Developing new algorithms which combine multiple eye tracking metrics offers the potential of strengthening apparel prototyping in the future. Advances in computer processing speed and storage capacity underlie the potential to simultaneously interpret eye tracking metrics such as gaze observations, time to first gaze, fixations in each region and region fixation duration among others. Combining eye tracking metrics may provide apparel designers with a deeper understanding of consumer preferences with logical extension to design variants and improvements. In this study, given the task of focusing on parts of the garment that participants felt were the most innovative suggested a corollary between the gaze observations in each region and fixations in each region as the best predictors of garment variability between subjects. The researchers suggest that eye tracking metrics involving repeated exposures should combine diverse raw data measurements to compare subject responses and would like to further test the value of creating eye tracking data composite scores to evaluate user experience data in further studies. In addition, future studies should explore the benefits of employing multiple subsystems simultaneously in consumer experience research. For example, collecting gestural data from a clickable heat map based survey on a tablet PC and eye tracking data simultaneously. Comparisons within and between datasets for both methods could be explored to increase the predictive value of eye tracking metrics and weighting systems for data mining.
References
[1] | Gigerenzer, G., Hoffrage, U., & Kleinbölting, H. (1991). Probabilistic mental models: a Brunswikian theory of confidence. Psychological review, 98(4), 506. |
[2] | Gubbi, J., Buyya, R., Marusic, S., & Palaniswami, M. (2013). Internet of Things (IoT): A vision, architectural elements, and future directions. Future Generation Computer Systems, 29(7), 1645-1660. |
[3] | He, X., Zemel, R. S., & Carreira-Perpiñán, M. Á. (2004, June). Multiscale conditional random fields for image labeling. In Computer vision and pattern recognition, 2004. CVPR 2004. Proceedings of the 2004 IEEE computer society conference on (Vol. 2, pp. II-695). IEEE. |
[4] | Holmqvist, K., Nyström, M., Andersson, R., Dewhurst, R., Jarodzka, H., & Van de Weijer, J. (2011). Eye tracking: A comprehensive guide to methods and measures. Oxford University Press, pp. 405-6. |
[5] | Hall, D., & Llinas, J. (Eds.). (2001). Multisensor data fusion. CRC press. |
[6] | Park, J., DeLong, M., & Woods, E. (2012). Exploring product communication between the designer and the user through eye-tracking technology. International Journal of Fashion Design, Technology and Education, 5(1), 67-78. |
[7] | Pieters, R., & Wedel, M. (2012). Ad gist: ad communication in a single eye fixation. Marketing Science, 31(1), 59-73. |
[8] | Rahulan, M., Troynikov, O., Watson, C., Janta, M., & Senner, V. (2015). Consumer behavior of generational cohorts for compression sportswear. Journal of Fashion Marketing and Management, 19(1), 87-104. |
[9] | Tusche, A., Bode, S., & Haynes, J. D. (2010). Neural responses to unattended products predict later consumer choices. The Journal of Neuroscience, 30(23), 8024-8031. |
[10] | Vogt, J., De Houwer, J., Moors, A., Van Damme, S., & Crombez, G. (2010). The automatic orienting of attention to goal-relevant stimuli. Acta psychologica, 134(1), 61-69. |
[11] | Wedel, M., & Pieters, R. (2008). A review of eye-tracking research in marketing. Review of marketing research, 4(2008), 123-147. |
[12] | Zangemeister, W. H., Sherman, K., & Stark, L. (1995). Evidence for a global scanpath strategy in viewing abstract compared with realistic images. Neuropsychologia, 33(8), 1009-1025. |