Elham I. M. Ibrahim1, Rihab Ali Omer2, Ahmed H. Elsadig3, Mohamed Sir Elkhatim4, Sofia B. Mohamed2
1Haematology Department, Faculty of Medical Laboratory Sciences, National University, Khartoum, Sudan
2National University, National University Research Institute, Khartoum, Sudan
3Faculty of Medicine, University of Khartoum, Khartoum
4National University, Khartoum, Sudan
Correspondence to: Sofia B. Mohamed, National University, National University Research Institute, Khartoum, Sudan.
Email: |  |
Copyright © 2017 Scientific & Academic Publishing. All Rights Reserved.
This work is licensed under the Creative Commons Attribution International License (CC BY).
http://creativecommons.org/licenses/by/4.0/

Abstract
Computational analysis has become an indispensable bioinformatics approaches for the characterization of proteins regarding the physicochemical properties, prediction of signal peptides and 3D structure. Additionally, computational studies of protein–ligand interactions provide a rational basis for the speedy identification of novel leads for drug. To date no any computational analysis evaluating such parameters for GM-CSF-Rα, IL-3-Rα and IL-5-Rα. Hence, the present work aimed at identifying the theoretical basis of the physicochemical, structural and functional proprieties for these proteins using online computational tools.In the present study, different bioinformatics tools were used to characterize the properties and structure of the GM-CSF-Rα, IL3Rα, and IL5Rα proteins. Firstly, the Physico-chemical characterization was computed by ExPasy’s (ProtParam). Then Fingerprinting analysis was done with ScanProsite. Followed by the functional characterization of the transmembrane regions and phosphorylation sites using SOSUI server and NetPhos server respectively. Afterwards, secondary structure prediction and the protein-ligand binding site residues were predicted by PDBSUM, and the detected ligands and their interactions were visualized by LIGPLOT and Protein ligand interaction profiler (PILP) softwares. The residues in GM-CSF-Rα, IL-3Rα and IL-5Rα proteins that may undergo ubiquitination were detected by using the UbPred and BDM-PUB programs, the predicted peptides for sumoylation in GM-CSF-Rα, IL-3Rα and IL-5Rα proteins were detected by GPS-SUMO online service. Finally, the 3D structure of proteins was built by Chimera 1.8 program. In addition, the models were surveyed using ERRAT server; as a confirmation for the quality of the models. Our results revealed that GM-CSF-Rα is stable whereas the IL3Rα and IL5Rα are classified as unstable proteins. All proteins are membrane proteins, acidic and hydrophilic in nature, with serine being the most phosphorylated amino acid. Interestingly, fibronectin type-III (FN3) domain was detected among these proteins. Also, we detected the sequences belonging to the following families: HEMATOPO_REC_S_F2, ASN_GLYCOSYLATION, CK2_PHOSPHO_SITE, PKC_PHOSPHO_SITE, MYRISTYL, CAMP_PHOSPHO_SITE, and TYR_PHOSPHO. Moreover, we detected 9 kinases in GM-CSF-Rα, while 13 kinases in IL-3-Rα and 15 kinases in IL-5-Rα. In GM-CSF-Rα 3 binding sites were detected with two ligands (GOL and NAG), and 5 binding sites in IL-3-Rα and IL-5-Rα with 3ligands (NAG, FUL and BMA) and one ligand (BGC) respectively. Secondary structure prediction showed that Beta sheet dominated all the other conformations. Modeling the 3 D structure of proteins resulted in a quality of less than 90%.computational analysis of GM-CSF-Rα, IL-3-Rα and IL-5-Rα will give a deep insight and provide opportunities for understanding the function of these proteins, and developing novel therapeutics for treating certain leukemia and inflammatory diseases.
Keywords:
In silico analysis, Ligand binds site, Homology modeling and GM-CSF-Rα, IL3Rα, IL5Rα and α-subunit proteins
Cite this paper: Elham I. M. Ibrahim, Rihab Ali Omer, Ahmed H. Elsadig, Mohamed Sir Elkhatim, Sofia B. Mohamed, In Silico Analysis of the Structural and Biochemical Features of the Granulocyte-Macrophage Colony Stimulating Factor (GM-CSF), Interleukin-3 (IL-3) and Interleukin-5 (IL-5) Receptors Subunit α, American Journal of Bioinformatics Research, Vol. 7 No. 1, 2017, pp. 25-47. doi: 10.5923/j.bioinformatics.20170701.03.
1. Introduction
Experimental determination of protein structure and function is becoming increasingly important, as proteins have attracted interest as drug targets, but it is labour intensive time consuming and expensive. Thus, the use of computational tools for appoints structure to a novel protein represents the most effective alternate to experimental methods [1]. In the last years, we have seen the emergence of computational methods that have been developed for predicting the primary, secondary and tertiary structures of proteins, as well as functional analyses, reducing the time needed to conduct experiments and allowing the more rapid acquisition of results. As far as physicochemical and structural characterizations of a protein, there is no doubt that in silico approaches help resolve these problems [2]. The receptors of hematopoietic cytokines: Granulocyte-macrophage colony-stimulating factor (GM-CSF), cytokines interleukin -3 (1L-3), and interleukin -5 (IL-5) are members of a family of proteins referred to as the "cytokine receptor family", which is characterized by the existence of a 200-residue ligand-binding module [3]. These high-affinity receptors consist of multiple subunits: α subunit which is specific for each ligand, and β subunit which is common for the three receptors. [3], [4], [5]. (GM-CSF) and the concerning (IL-3) and (IL-5) cytokines regulate the production and functional activation of hematopoietic cells. (GM-CSF) is a pleiotropic cytokine that monitors the production and function of blood cells, mainly monocyte /macrophages and all granulocytes. It is deregulated in clinical conditions such as rheumatoid arthritis and leukemia, likewise offers therapeutic value for other diseases [6]. GM-CSF also controls dendritic cell and T-cell function, so that linking innate and acquired immunity [7]. Interleukin 3 (IL-3) is a cytokine produced predominantly by antigen-activated T cells that links immunity to the hematopoietic system and plays a considerable role in leukemia as well as various immune pathologies [8]. By actions on several cell types, IL-3 participates to allergic inflammation, autoimmune diseases, and oncogenesis. Importantly, leukemic stem cells from patients with acute myeloid leukemia (AML) and chronic myeloid leukemia (CML) over express the IL-3 receptor α chain (IL3Rα), and this is associated with a poor prognosis in AML [8]. Interleukin 5 (IL-5) is a hematopoietic growth factor, primarily known as a T-cell-derived cytokine, has pleiotropic effects on different target cells, including eosinophils and B cells, and induces cell proliferation, survival and differentiation [9]. The capability of cytokines to impact the course of cell growth and differentiation uniquely rely on their recognition and binding by specific receptors; these cell surface molecules transducer the binding of cytokines into cytoplasmic signals that trigger developmental processes within the cell [10]. The subunits (GM-CSF-Rα, IL-3Rα and IL-5Rα) are cytokine-specific binding proteins, and each α subunit alone binds its specific ligand with low affinity. In contrast, the β subunit does not join any cytokine by itself, but forms high-affinity receptors with α subunits. Human GM-CSF, IL-3 and IL-5 receptors have only one type of β subunit (common β, or βc) which is participated by the three receptors [5]. The thorough structure of GM-CSF-Rα, IL-3Rα and IL-5Rα is identical: they are glycoproteins of - 60-80 kDa having the common motif of the cytokine receptor super family in the extracellular domain and they have a small cytoplasmic domain with a short stretch of an amino acid sequence which is conserved among these α subunits [5]. It has been speculated that the ligand-specific α subunits may have a role in transmitting ligand-specific signals, though the common β subunit plays a major role in signal transduction for proliferation [11]. The GM-CSF, IL-3 and IL-5 receptor α chains form a special subgroup and share features not found in other members of the cytokine receptor family, features which are suggested to be important for their interaction with the common beta chain and for their binding of the structurally-related ligands [5]. So, the main objective of this study is to fulfill a protein analysis of α Subunit of GM-CSF, IL-3, and IL-5 receptors using up-to-date bioinformatics tools, and to highlight the differences and similarities between these proteins. This will further reveal the complex nature of the mechanisms by which these receptors regulate signal transduction of hematopoietic stem cells. In addition, such understanding for these receptors provides opportunities for the development of new therapies to block the action of their cytokines in certain haematological malignancies. Nevertheless, the knowledge of these receptors is placed in context with advances in understanding of the structural biology of other members of the cytokine receptor family.
2. Materials and Methods
2.1. Extraction of Protein Sequences
The protein sequences of hematopoietic cytokines receptors were extracted from UniProt (http://www.uniprot.org/). The UniProt database is a substantial collection of protein sequences and their annotations. It has cross-references to over 150 databases and acts as a central axis to regulate protein information [12]. The protein sequences were retrieved in FASTA format, in order to be analyzed by computational methods.
2.2. Identification of Amino Acid Percentage Composition and Physico-Chemical Properties
The primary structure was predicted using the ProtParam server; it is free online tool (http://web.expasy.org/protparam/) in Expasy. The parameters computed by ProtParam include the molecular weight (M.Wt), isoelectric point (pI), amino acid composition, atomic composition, extinction coefficient (EC), estimated half-life, instability index(II), aliphatic index(AI) and grand average of hydropathicity (GRAVY). The amino acid and atomic compositions are self-explanatory. All the other parameters will be explained below [13]. Isoelectric Point (pI):The calculated isoelectric point (pi) is useful since at this point the solubility is lost and the mobility in an electric field is zero. Isoelectric point is the pH at which the surface of the proteins is covered with the charge but a net charge of the protein is zero.Extinction Coefficients (EC):The extinction coefficient indicates how much light a protein absorbs at a certain wavelength. It is useful to have an estimation of this coefficient for analyzing a protein with a spectrophotometer when purifying it. [14]. It has been shown that it is possible to estimate the molar extinction coefficient of a protein from knowledge of its amino acid composition. For example, the molar extinction coefficient of Tyrosine, Tryptophan and Cystine (Tyrosine does not absorb appreciably at wavelengths >260 nm, while Cystine does) at a given wavelength.Instability Index (II):The instability index provides an estimate of the stability of a protein in a test tube. Statistical analysis of 12 unstable and 32 stable proteins has revealed that there are certain dipeptides, the occurrence of which is significantly different in the unstable proteins compared with those in the stable ones [15]. A protein whose instability index is smaller than 40 is predicted as stable, a value above 40 predicts that the protein may be unstable.Aliphatic Index (AL):The aliphatic index of a protein is defined as the relative volume occupied by aliphatic side chains (Alanine, Valine, Isoleucine, and leucine). It may be regarded as a positive factor for the increase of thermostability of globular [16]. Grand Average of Hydropathy (GRAVY):The GRAVY value for a peptide or protein is calculated as the sum of hydropathy values of all the amino acids, divided by the number of residues in the sequence [17]. Estimated Half-Life:The half-life is a prediction of the time required for half of a protein in a cell to degrade after its synthesis. ProtParam relies on the "N-end rule", which relates the half-life of a protein to the identity of its N-terminal residue; the prediction is given for three model organisms; human, yeast, and E. coli. The identity of the N terminal residue of a protein plays an important role in determining its stability in vivo. Proteins have strikingly different half-lives in vivo, from seconds to hours, depending on the nature of the amino acid at the N terminus and the different models.
2.3. Hydrophobicity Analysis
Percentages of hydrophobic and hydrophilic residues were calculated from the percentage of Amino Acid composition.
2.4. Fingerprinting Analysis
ScanProsite used for fingerprinting analysis, it is free online database and tool (http://prosite.expasy.org/scanprosite/). Also, it has a large collection of biologically meaningful signatures that are described as patterns (regular expressions), used for short motif detection, or generalized profiles (weight matrices) for sensitive detection of larger domains. Each signature is linked to detailed annotation that provides useful biological information on the protein family, domain, or functional sites identified by the signature [18]. PROSITE is copyright. It is produced by the SIB Swiss Institute Bioinformatics. There are no restrictions on its use by non-profit institutions as long as its content is in no way modified. Usage by and for commercial entities requires a license agreement.
2.5. Transmembrane Sequence Analysis
Transmembranase domains were predicted by using SOSUI server, which distinguishes between membrane and soluble proteins from amino acid sequences, and predicts the transmembrane helices for the former [19]. The system SOSUI is available through internet access (http://harrier.nagahama-i-bio.ac.jp/sosui/sosui_submit.html).
2.6. Prediction of Hydrophobic Residues
The hydrophobic residues were predicted by using pepwheel, it is available at (http://emboss.open-bio.org/wiki/Appdocs) on the EMBOSS Wiki. pepwheel program draws a helical wheel diagram for a protein sequence. This displays the sequence in a helical representation as if looking down the axis of the helix. It is useful for highlighting amphipathicity and other properties of residues around a helix. By default, aliphatic residues are marked with squares; hydrophilic residues are marked with diamonds, and positively charged residues with octagons, although this can be changed [20].
2.7. Prediction of Phosphorylation Sites
Phosphorylatiuonsites were predicted by using NetPhos 3.1 server, it is publicly available at the (http://www.cbs.dtu.dk/services/NetPhos/). The NetPhos 3.1 server produces neural network predictions for serine, threonine and tyrosine phosphorylation sites in eukaryotic proteins in eukaryotic proteins using ensembles of neural networks. Both generic and kinase specific predictions are performed. The kinase specific predictions are identical to the predictions by NetPhosK 1.0. Predictions are made for the following 17 kinases: ATM, CKI, CKII, CaM-II, DNAPK, EGFR, GSK3, INSR, PKA, PKB, PKC, PKG, RSK, SRC, cdc2, cdk5 and p38MAPK. [21].
2.8. Protein Ubiquitination Sites Prediction
UbPred and BDM-PUB programs were used to predict ubiquitylation sites [22]. In UbPred, lysine residues with a score of 0.62 were considered ubiquitylated. For BDM-PUB, the balanced cut-off option was selected. UbPred was developed by Predrag Radivojac (Indiana University, School of Informatics), Vladimir Vacic (Columbia University) and Lilia Iakoucheva (University of California, San Diego). It is publicly available at the (http://www.ubpred.org/). BDM-PUB it is publicly available at the (http://bdmpub.biocuckoo.org/). Copyright © 2006-2009. The CUCKOO Workgroup, USTC.
2.9. Protein Sumoylation Sites Detection
The identification of small ubiquitin-like modifiers (SUMOs) sites was carried out with the help of GPS-SUMO web server (http://sumosp.biocuckoo.org/. It is a novel web server developed for the prediction of both sumoylation sites and SUMO-interaction motifs (SIMs) in proteins. Copyright © 2006-2014. The CUCKOO Workgroup. [23]. In addition, the primary structure of these peptides was drawn using pepdraw, a tool to draw peptide primary structure and calculate theoretical properties.
2.10. Signal Peptide Prediction (Predisi)
Prediction of signal peptides was performed using PrediSi (PREDIction of SIgnal peptides). It is new software for predicting signal peptide sequences and their cleavage positions in bacterial and eukaryotic proteins. Available at (http://www.predisi.de/) [24]. Coordinated by Karsten Hiller Institute for Microbiology, Technical University of Braunschweig.
2.11. Protein – Ligand Binding Sites Detection
The identification of specific ligand-binding site on the three proteins was performed by PDBsum, LIGPLOT and PLIP. PDBsum (http://www.ebi.ac.uk/pdbsum) is a pictorial database that provides an at-a-glance overview of the contents of each 3D structure deposited in the Protein Data Bank (PDB). It shows the molecule(s) that make up the structure (ie protein chains, DNA, ligands and metal ions) and schematic diagrams of the interactions between them [25]. Schematic 2-D representations of protein-ligand complexes from standard Protein Data Bank file input were automatically generated by the LIGPLOT (http://www.ebi.ac.uk/thornton-srv/software/LIGPLOT/program). The results of interactions shown are those mediated by hydrogen bonds and by hydrophobic contacts. Hydrogen bonds are indicated by dashed lines between the atoms involved, while hydrophobic contacts are represented by an arc with spokes radiating towards the ligand atoms they contact. The contacted atoms are shown with spokes radiating back [26]. Additionally, 3D structures of these protein –ligands complexes were presented by the protein–ligand interaction profiler (PLIP), a novel web service for fully automated detection and visualization of relevant non-covalent protein–ligand contacts in 3D structures, freely available at (http://plip.biotec.tu-dresden.de/plip-web/plip/index) [27].
2.12. 3D Structure of the Proteins
The 3-dimensional structure anticipation we applied CPH models 3.2 servers (http://www.cbs.dtu.dk/) to predict the PDB of proteins. It is a protein homology modeling server, where the template realization is based on profile-to profile arrangement, guided by secondary structure and presentation prognosis [28]. Visualization and characterization of the protein model were done by Chimera (version 1.8) Chimera (http://www.rbvi.ucsf.edu/chimera) is developed by the Resource for biocomputing, Visualization, and Informatics at the University of California, San Francisco (supported by NIGMS P41-GM103311) software [29].
2.13. Validation of 3D Models
Structural validation of proteins models was done by ERRAT; a program for verifying protein structures determined by crystallography. Error values are plotted as a function of the position of a sliding 9-residue window. The error function is based on the statistics of non-bonded atom-atom interactions in the reporting structure (compared to a database of reliable high-resolution structures) (http://services.mbi.ucla.edu/ERRAT/) [30].
3. Results
3.1. The Sequences Retrieve
The sequences for receptors of three hematopoietic cytokines: GM-CSF-Rα, IL3Rα, and IL5Rα proteins were retrieved from Uniprot database (www.uniprot.org), Homo sapiens database. The UniProt database is a substantial collection of protein sequences and their annotations. It has cross-references to over 150 databases and acts as a central axis to regulate protein information [12], using these sequences in FASTA format for further analysis. The description of proteins were analyzed in this study were shown in (Table 1). Table 1. Proteins examined in this study 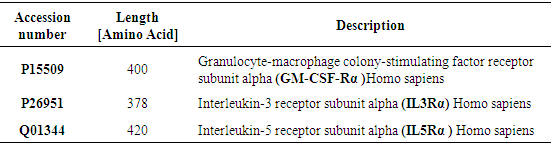 |
| |
|
3.2. Primary Structure Prediction
The parameters were computed by ProtParam. The Atom composition of proteins, the percentage of their hydrophobic and hydrophilic residue content, and their amino acid composition are shown in (Table 2), (Table 3) and (Table 4) respectively. Table 2. Atoms composition and formulas for GM-CSF-Rα, IL-3Rα, and IL-5Rα proteins 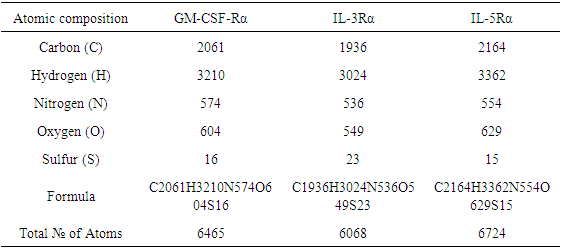 |
| |
|
Table 3. Hydrophilic and hydrophobic residue content 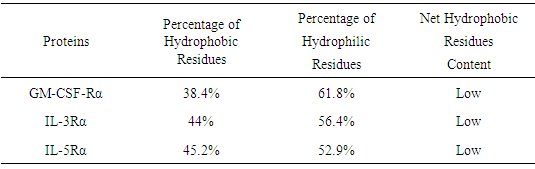 |
| |
|
Table 4. Amino acid composition (in %) of GM-CSF-Rα, IL-3Rα, and IL-5Rα proteins using ProtParam tool 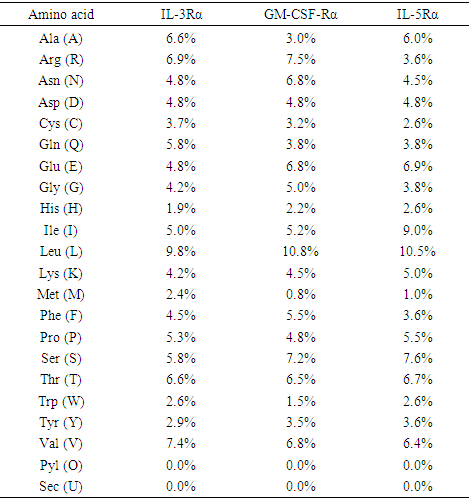 |
| |
|
3.3. Physicochemical Analysis
The Physico-chemical characteristics involve the molecular weight, isoelectric point, total number of positive and negative residues, extinction coefficient, and grand average of hydropathicity are depicted in (Table 5).Table 5. Physical and Chemical Characters of the Primary Structures of Predicted Proteins in Theory 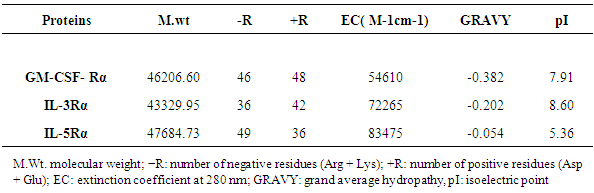 |
| |
|
3.4. Half Lifetime, Stability and Solubility
The estimated half-life, instability index (II) and aliphatic index(AI) of proteins are shown in (Table 6).Table 6. Estimated half-life of GM-CSF-Rα, IL-3Rα, and IL-5Rα proteins using ProtParam tool 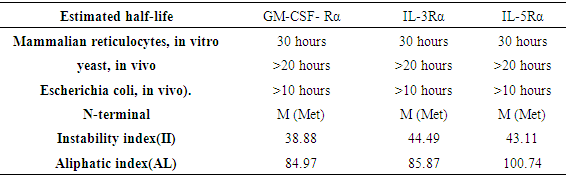 |
| |
|
3.5. Functional Site Predication
The potential domains as well as characteristic motifs and patterns contained in GM-CSF-Rα, IL-3Rα, and IL-5Rα proteins were investigated by ScanProsite. The results are shown in (Tables 7, 8, 9 and 10).Table 7. Domains detected in GM-CSF-Rα, and IL5Rα proteins using ScanProsit 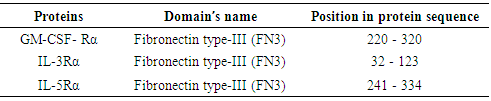 |
| |
|
Table 8. GM-CSF- Rα protein expression profiles using ScanProsit 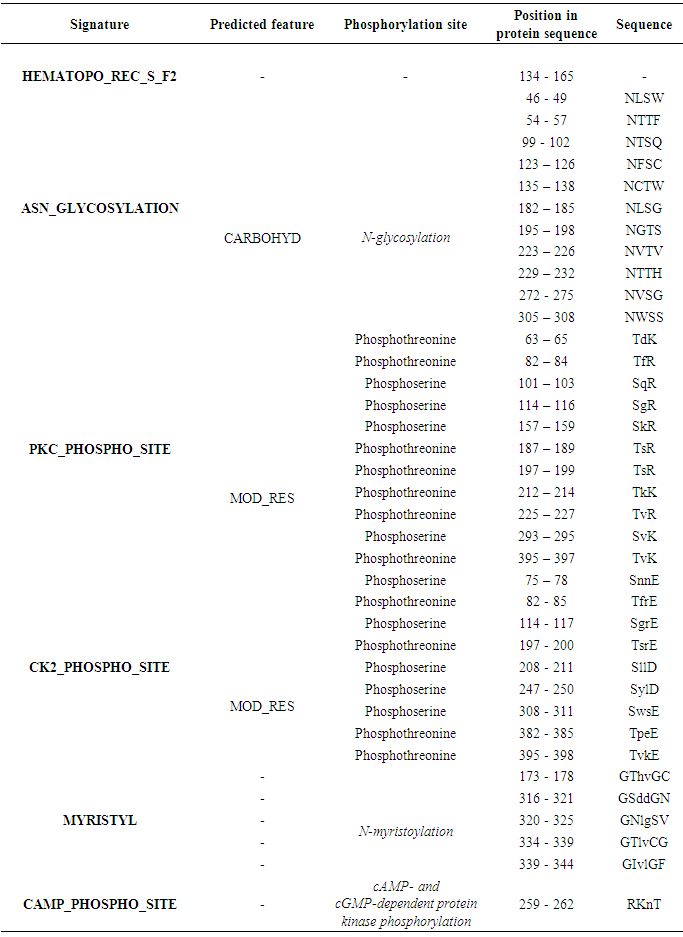 |
| |
|
Table 9. IL-3Rα protein expression profiles using Scanprosit 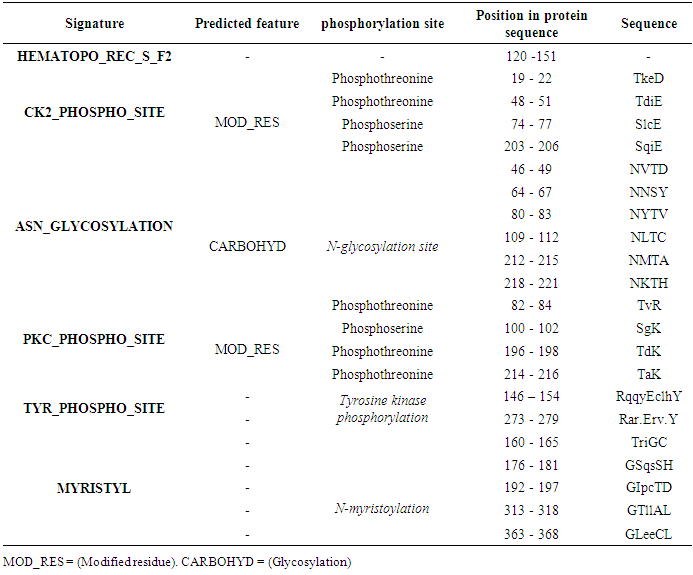 |
| |
|
Table 10. IL-5Rα protein expression profiles using Scan Prosit 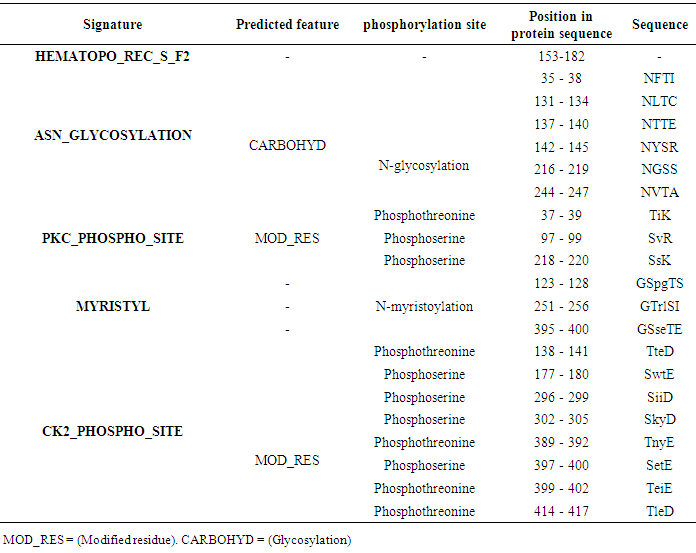 |
| |
|
3.6. Prediction of the Transmembrane Site
The SOSUI server performed the identification of transmembrane region. The transmembrane regions and their length were classified in (Table 11).Table 11. Transmembrane sequence analysis of SOSUI server 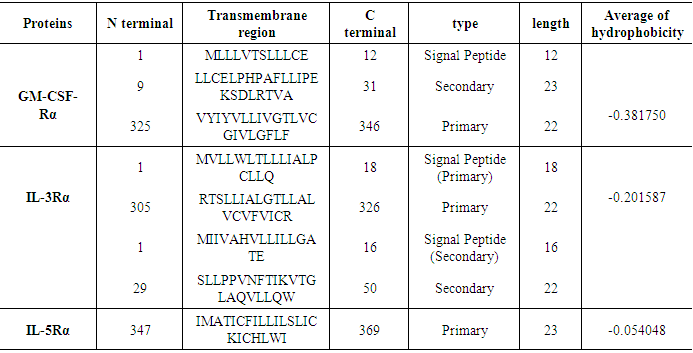 |
| |
|
3.7. Helical Wheel Predicted by Pepwheel Program
The Hydrophilic residues for protein sequences were predicted by utilizing pepwheel program. By default, aliphatic residues are marked with squares; hydrophilic residues are marked with diamonds, and positively charged residues with octagons. The result summarizes in fig 1, 2 and 3.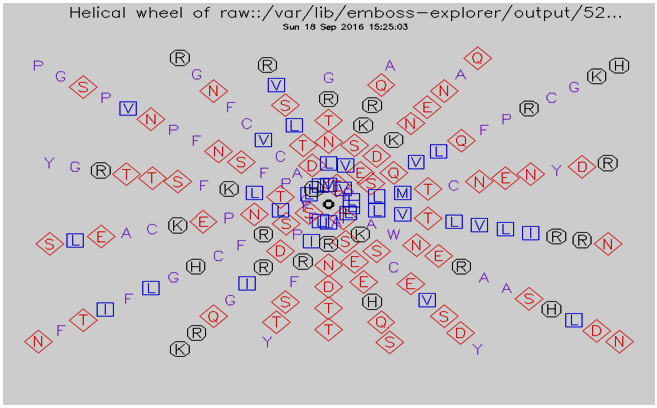 | Figure 1. Helical wheel predicted by pepwheel for GM-CSF-Rα Protein |
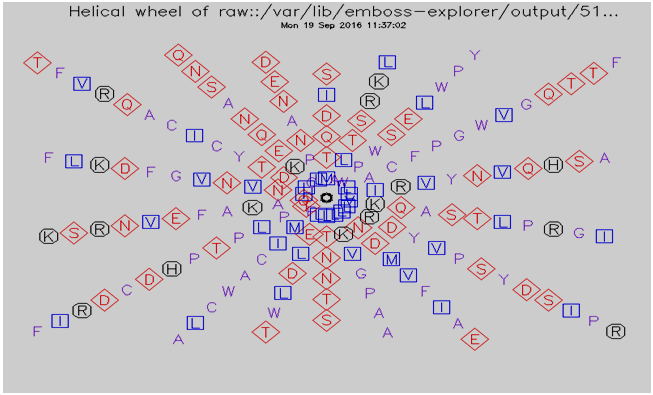 | Figure 2. Helical wheel predicted by pepwheel for IL-3Rα Protein |
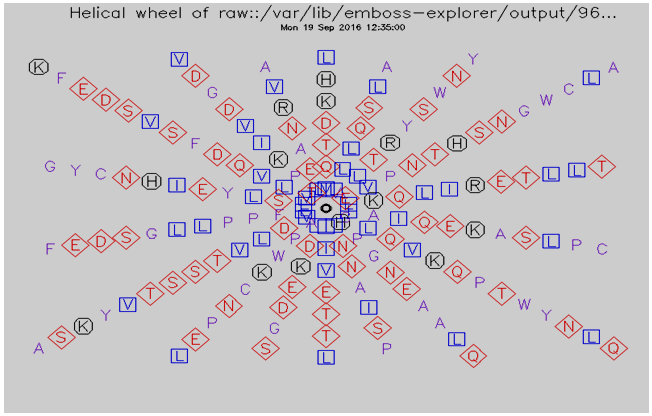 | Figure 3. Helical wheel predicted by pepwheel for IL-5Rα Protein |
3.8. Prediction of Phosphorylation Sites
The NetPhos 3.1 server predicted Phosphorylation site (Serine, Threonine and Tyrosine) and Kinase site for GM-CSF-Rα, IL-3Rα proteins and IL-5Rα. The results showed that cdc2, unsp, PKA, PKC, CKII, CKI, ATM, INSR and DNAPK were common in all proteins. Whereas RSK, p38MAPK, cdk5 and GSK3 were placed in IL-3Rα and IL-5Rα, EGFR and SRC only found in IL-5Rα. The results shown in Table 12 and figure 4, 5 and 6.Table 12. Phosphorylation and Kinase sites predicted in GM-CSF-Rα protein by using NetPhos3.1 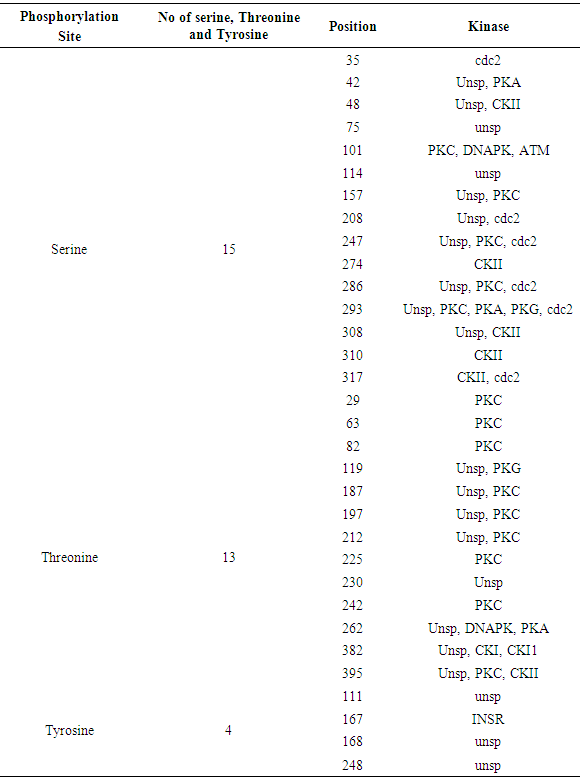 |
| |
|
Table 13. Phosphorylation and Kinase sites predicted in IL-3Rα protein by using NetPhos3.1 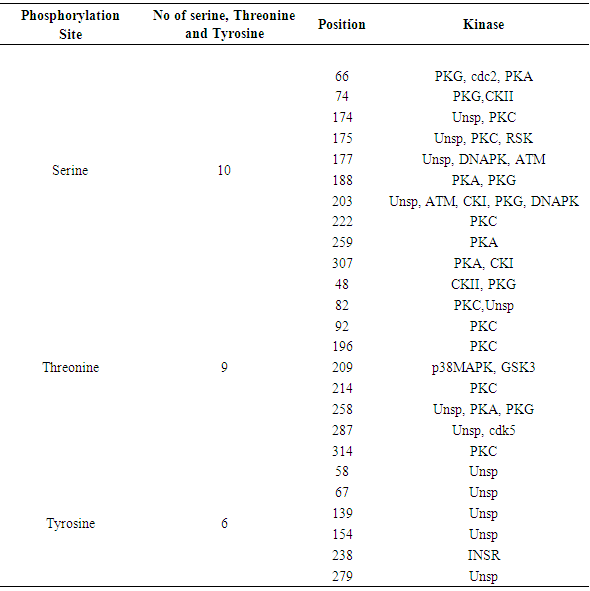 |
| |
|
Table 14. Phosphorylation and Kinase site predicted in IL-5Rα protein by using NetPhos 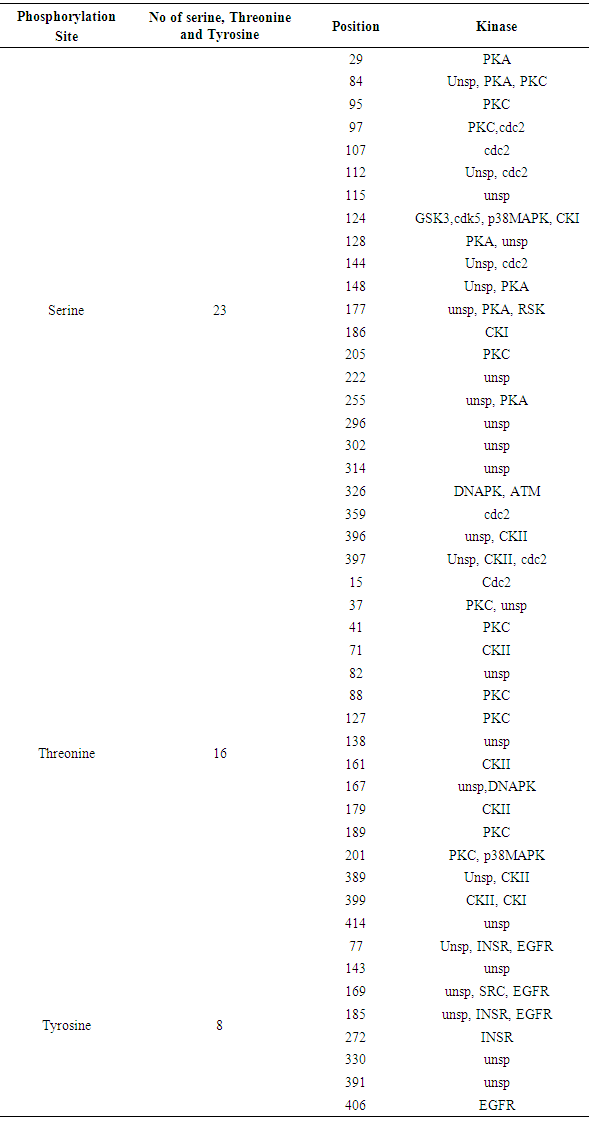 |
| |
|
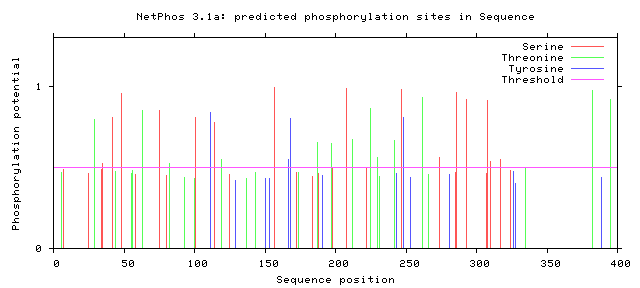 | Figure 4. Predicted Phosphorylation sites in GM-CSF-Rα protein |
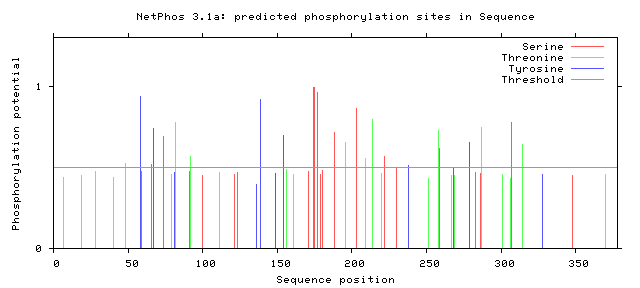 | Figure 5. Predicted Phosphorylation sites in IL-3Rα protein |
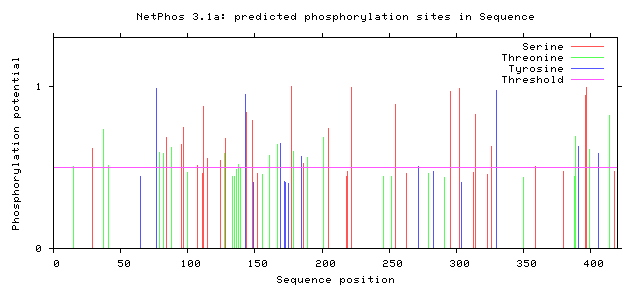 | Figure 6. Predicted Phosphorylation sites in IL-5Rα protein |
3.9. Prediction of Protein Ubiquitination Sites
The residues in GM-CSF-Rα, IL-3Rα and IL-5Rα proteins that may undergo ubiquitylation were analyzed by using the UbPred and BDM-PUB programs. In the GM-CSF-Rα, 13 and 2 Ubiquitination sites were predicted by UbPred and BDM-PUB respectively. Only one site (K 387) was conformation by both tools. In IL-3Rα 6 and 3 Ubiquitination sites were predicted by UbPred and BDM-PUB respectively. Also, only one site (K 361) was conformation by both tools. In IL-5Rα 9 and 4 Ubiquitination sites were predicted by UbPred and BDM-PUB respectively. Only one site (K 393) was conformation by both tools. The result shown in tables (16), (17), (18), (19), (20), and (21). Table 16. Predicted Ubiquitination sites in GM-CSF-Rα protein detected by Bayesian Discriminate Method (BDM) 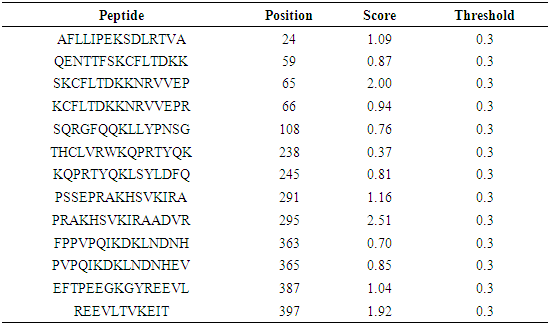 |
| |
|
Table 17. Predicted Ubiquitination sites in GM-CSF-Rα protein detected by UbPred server  |
| |
|
Table 18. Predicted Ubiquitination sites in IL-3Rα protein detected by Bayesian Discriminate Method (BDM) 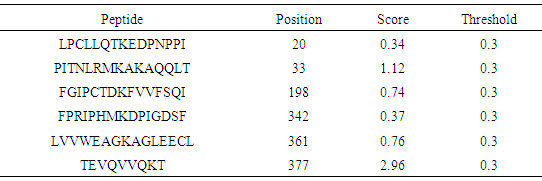 |
| |
|
Table 19. Predicted Ubiquitination sites in IL-3Rα protein detected by UbPred server 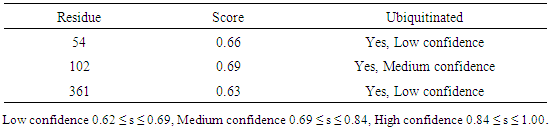 |
| |
|
Table 20. Predicted Ubiquitination sites in IL-5Rα protein detected by Bayesian Discriminate Method (BDM) 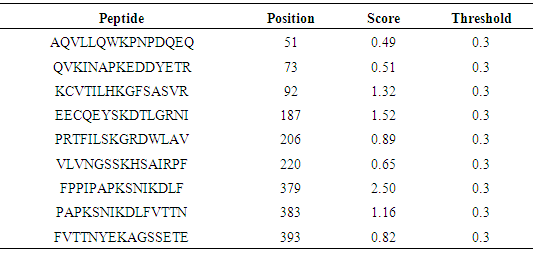 |
| |
|
Table 21. Predicted Ubiquitination sites in IL-5Rα protein detected by UbPred server 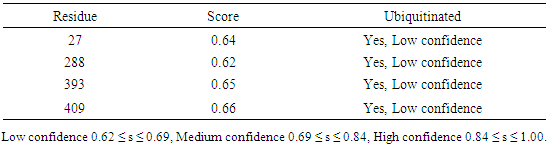 |
| |
|
3.10. Protein Sumoylation Sites Detection
The predicted peptides for sumoylation in GM-CSF-Rα, IL-3Rα and IL- 5Rα proteins by using GPS-SUMO online service are displayed in (Table 22).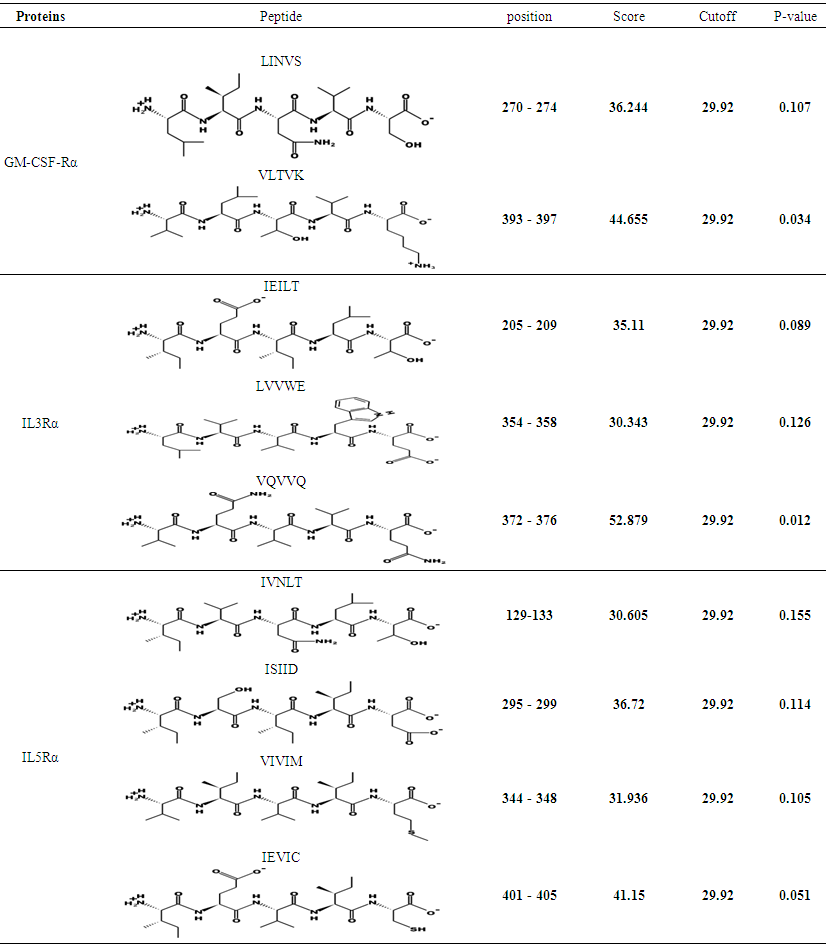 | Table 22. Sumoylation sites in GM-CSF-Rα, IL-3Rα and IL-5Rα proteins detected by GPS-SUMO |
3.11. Ingle Peptide Prediction (Predisi)
Prediction of signal peptides was performed using PrediSi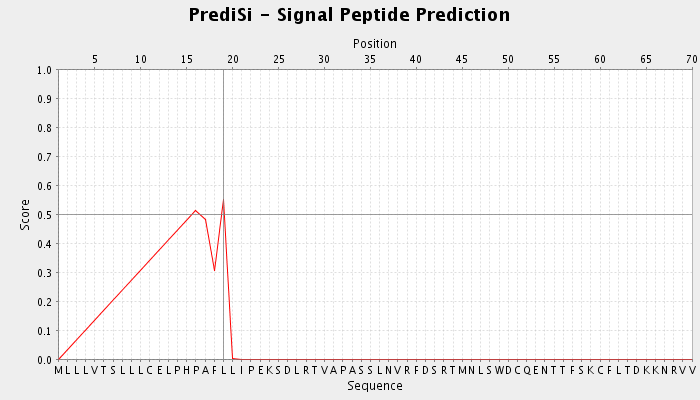 | Figure 7. Signal peptide prediction for GM-CSF-Rα protein detected by Predisi tool |
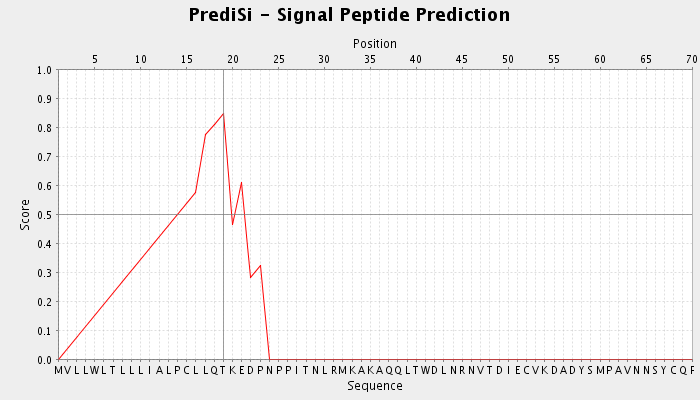 | Figure 8. Signal peptide prediction for IL3Rα protein detected by Predisi tool |
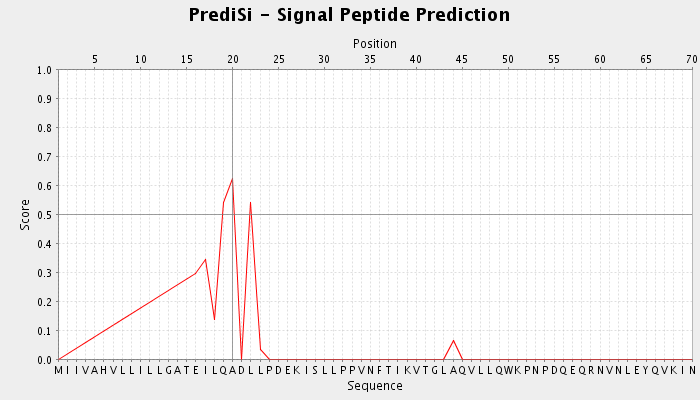 | Figure 9. Signal peptide prediction for IL5Rα protein detected by Predisi tool |
3.12. Protein–Ligand Binding Site Recognition
The predicted protein-ligand binding site residues by using PDB sum, and visualized by both LIGPLOT and PLIP softwares on the three proteins are presented in figures (10)-(24). An interaction diagram with interaction data is provided for each binding site.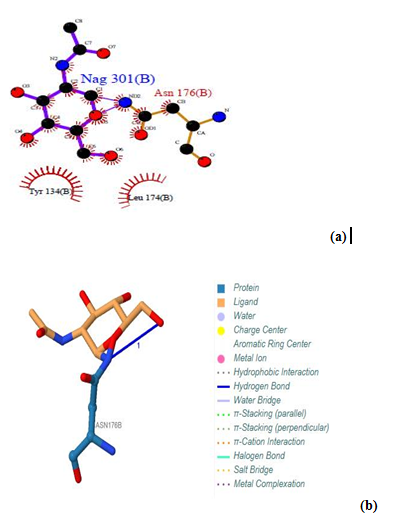 | Figure 10. Prediction of binding site of GM- CSF-Rα proteins. (a) LIGPLOT diagram of Nag (B) 301 binding site, showing the interactions of the residue Asn 176(B) with the surrounding protein residues. (b) Nag (B) 301 binding site using protein-ligand interaction profiler |
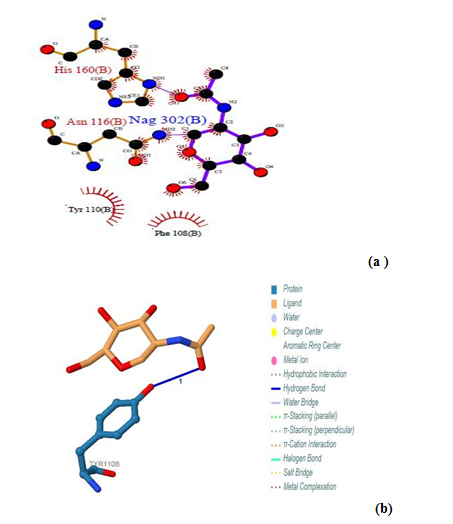 | Figure 11. Prediction of binding site of GM- CSF-Rα proteins. (a) LIGPLOT diagram of Nag 302 (b) binding site, showing the interactions of the residues Asn 116(B) and His 160 (B) with the surrounding protein residues (B) Nag 302 (B) binding site using PILP |
3.12.1. GM- CSF-Rα Protein
Three binding sites have been detected in position 301, 302 and 303. Tow ligands were found; GOL (glycerol) [Glycerin; propane-1, 2, 3-Triol]and NAG (Acetyl glucosamine) (N-Acetyl-D-Glucosamine), by the LIGPLOT NAG has interaction with Asn 176, and interaction with Asn 116 and His 160 residues. Also Gol has interaction with Ser 255. No ligands interactions found by PILP. 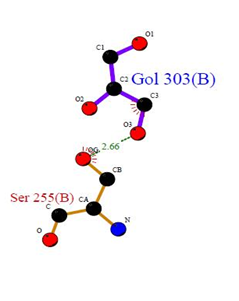 | Figure 12. Prediction of binding site of GM- CSF-Rα proteins. (a) LIGPLOT diagram of Gol 303 (B) binding site, involving the interactions of the residue Ser 255 (B) with the surrounding protein residues |
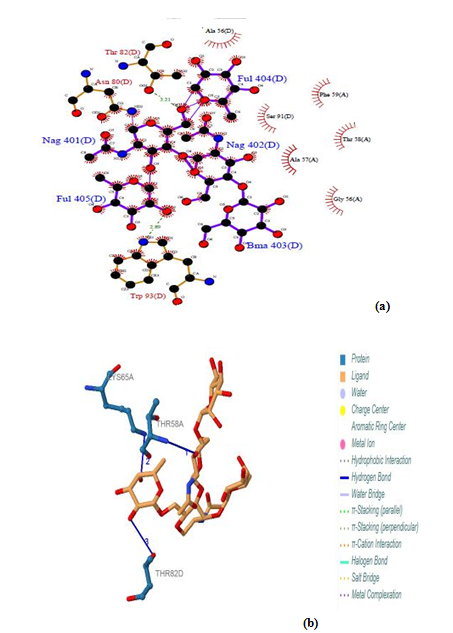 | Figure 13. Prediction of binding site of IL-3Rα protein (a) LIGPLOT diagram of NAG 401(D) to FUL 405(D) binding sites (b) Composite ligand consists of NAG:D:401, NAG:D:402, BMA:D:403, FUL:D:404, FUL:D:405 visualized by PILP |
3.12.2. IL-3Rα Protein
Five binding sites have been detected in position 401, 402, 403, 404 and 405 and visualized by PLIP & LIGPLOT tools. Three ligands were found; NAG (N-Acetyl-D-Glucosamine), FUL (Beta-L-Fucose) [6-Deoxy-Beta-L-Galactose] and BMA (Beta-D-Mannose, Alpha-D-Mannose). No interactions found by LIGPLOT and PILP.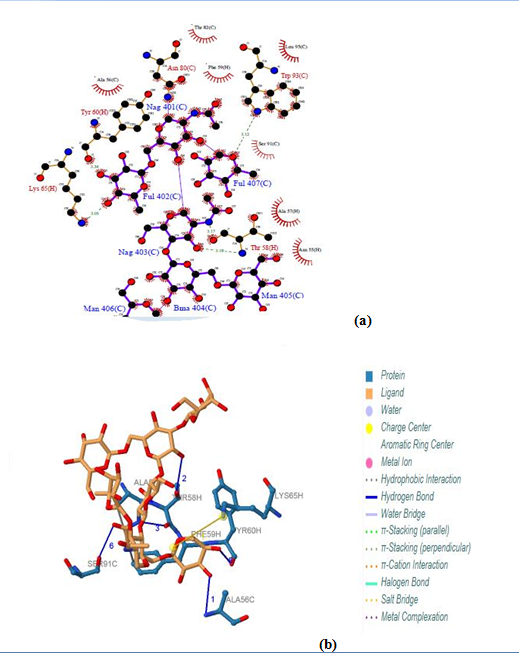 | Figure 14. Prediction of binding site of IL-3Rα protein. (a) LIGPLOT diagram of NAG 401(C) to FUL 407(C) binding sites (b) Composite ligand consists of NAG:C:401, FUL:C:402, NAG:C:403, BMA:C:404, MAN:C:405, MAN:C:406, FUL:C:407 by PILP |
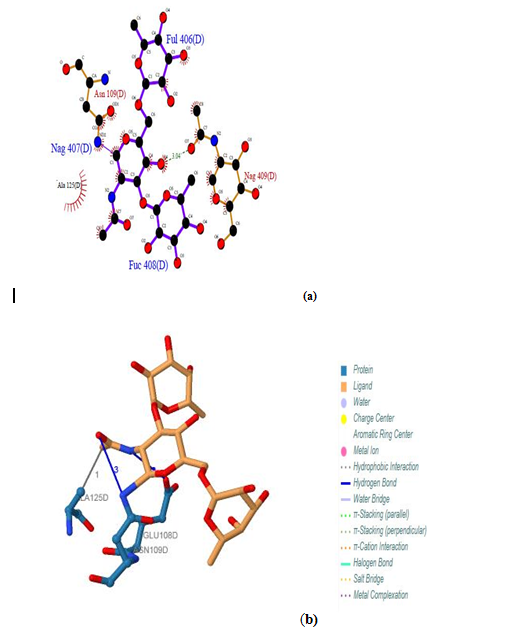 | Figure 15. Prediction of binding site of IL-3Rα protein. (a) LIGPLOT diagram of FUL: D:406, NAG: D:407, FUC: D:408 binding sites (b) Composite ligand consists of FUL: D:406, NAG: D:407, FUC: D:408 by PILP |
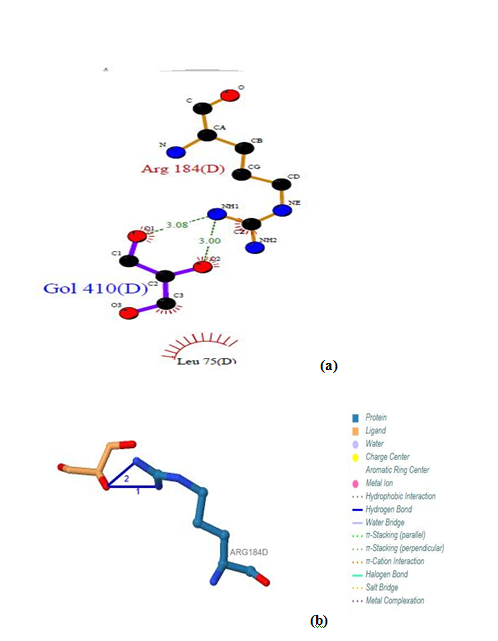 | Figure 16. Prediction of binding site of IL-3Rα protein. (a) LIGPLOT diagram of GOL 410 (D) binding site (b) GOL 410 (D) binding site by PILP |
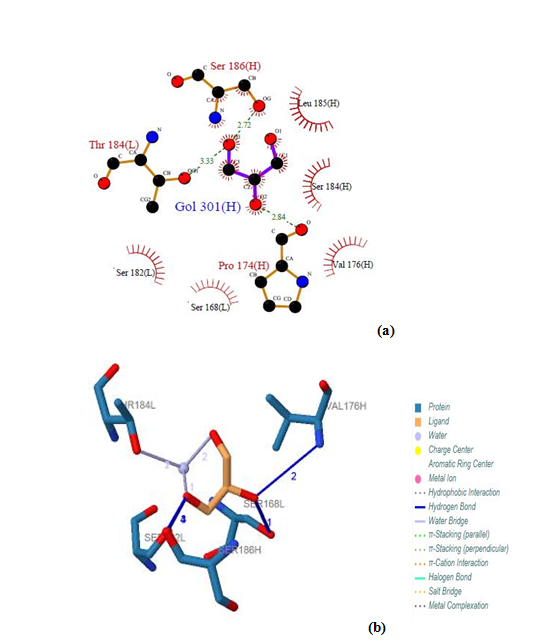 | Figure 17. Prediction of binding site of IL-3Rα protein. (a) LIGPLOT diagram of GOL 301 (H) binding site (b) GOL 301 (H) by PILP |
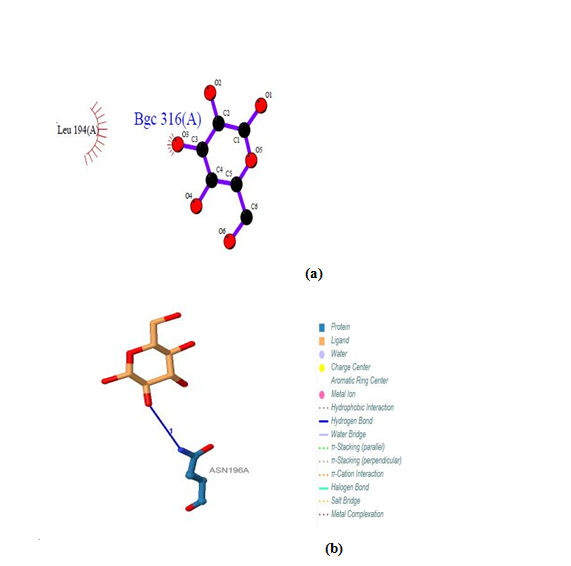 | Figure 18. Prediction of binding site of IL-5 Rα protein. (a) LIGPLOT diagram of 316 (A) binding site (b) 316 (A) binding site by PILP |
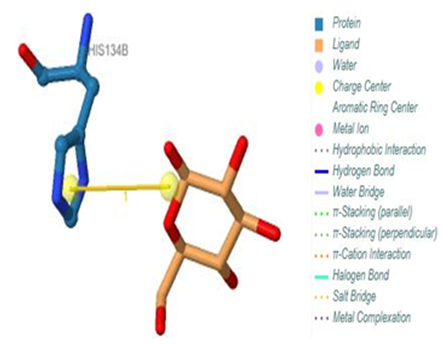 | Figure 19. Prediction of binding site of IL-5 Rα protein. 316 (B) binding site by PILP. NO result found by using LIGPLOT server for this site |
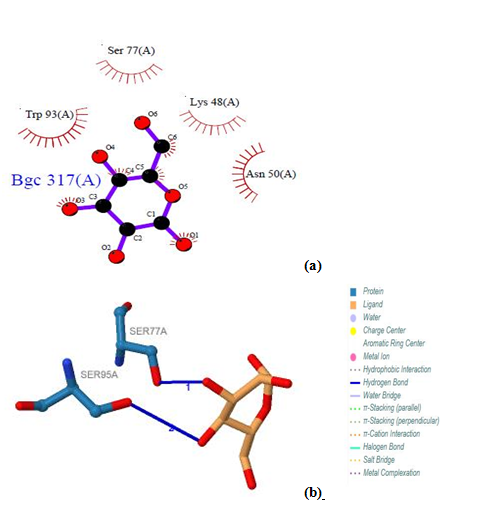 | Figure 20. Prediction of binding site of IL-5 Rα protein. (a) LIGPLOT diagram of 317 (A) binding site (b) 317 (A) binding site by PILP |
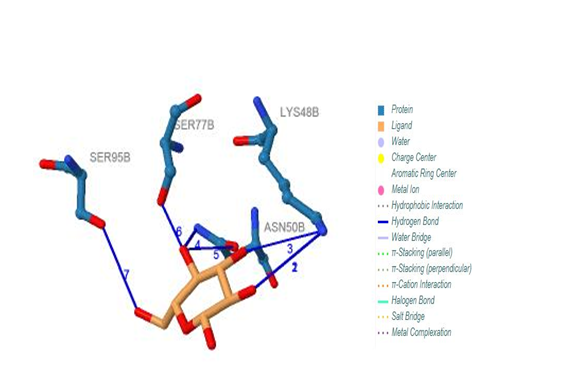 | Figure 21. Prediction of binding site of IL-5 Rα protein. 317 (B) binding site by PILP. NO result found by using LIGPLOT server for this site |
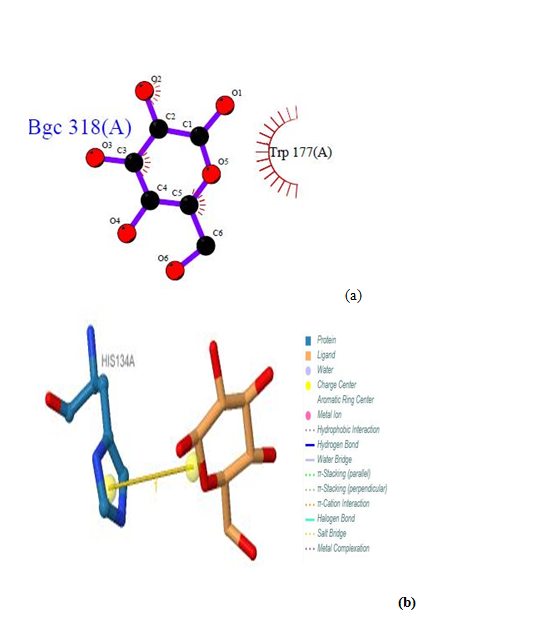 | Figure 22. Prediction of binding site of IL-5 Rα protein. (a) LIGPLOT diagram of 318 (A) binding site (b) 318 (A) binding site by PILP |
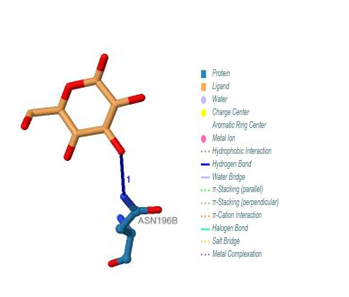 | Figure 23. Prediction of binding site of IL-5 Rα protein. 318 (B) binding site by PILP. No result found by using LIGPLOT server for this ligand |
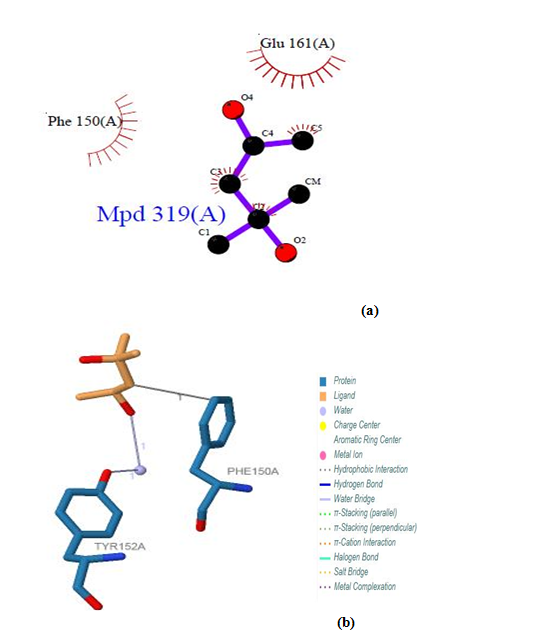 | Figure 24. Prediction of binding site of IL-5 Rα protein. (a) LIGPLOT diagram of 319(A) binding site (b) 319 (A) binding site by PILP |
3.12.3. IL-5 Rα Protein
Five binding sites have been detected in position 316, 317, 318 and 319, and visualized by PLIP & LIGPLOT tools. One ligand was detected; BGC - Beta- D- Glucose. No interactions found by LIGPLOT and PILP.
3.13. Protein Secondary Structure Prediction
The secondary structure prediction was carried out with the help of PDBsum software. The results are shown in figure 25, 26 and 27.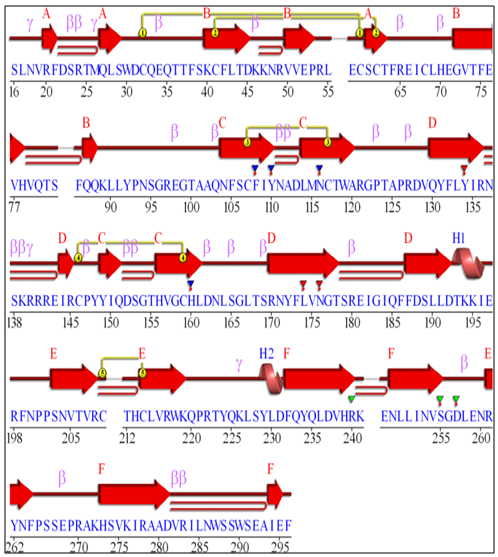 | Figure 25. GM-CSF-Rα secondary protein structure |
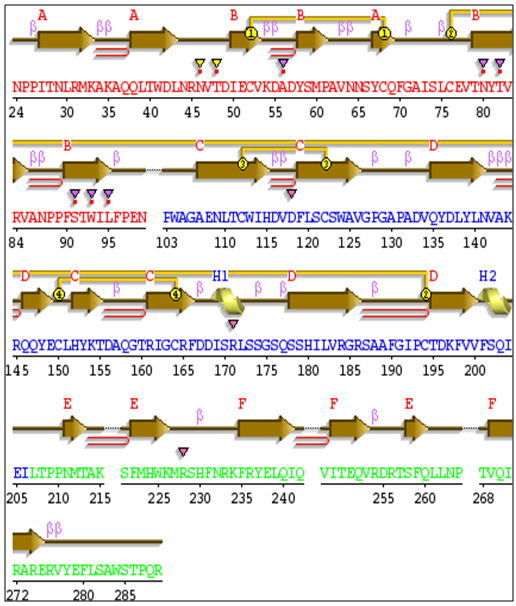 | Figure 26. IL-3Rα secondary protein structure |
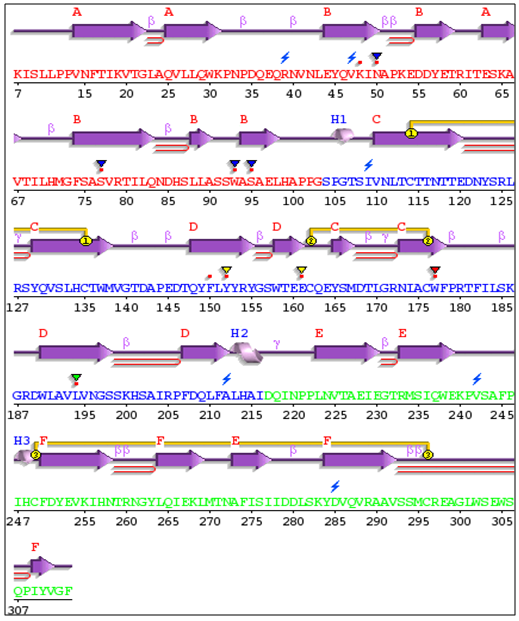 | Figure 27. IL-5Rα secondary protein structure |
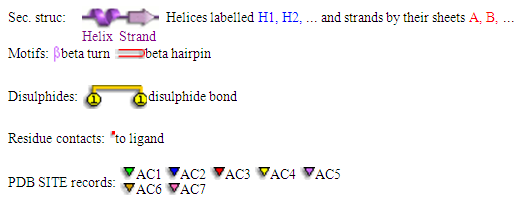
3.14. 3D Structure of the Proteins
3D structure of proteins was determined by homology modeling, using CPH models 3.2 server. Visualization of the proteins model was done by Chimera (version 1.8) program. The results are shown in figures 28, 29 and 30.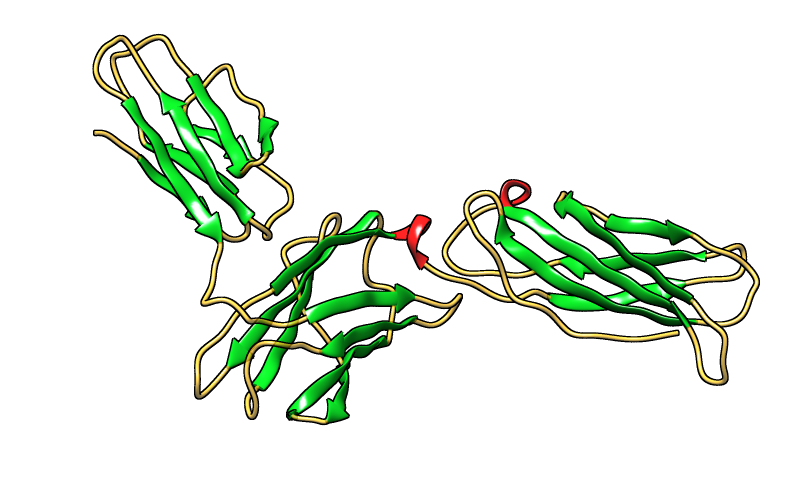 | Figure 28. Three dimensional structure of GM-CSF-Rα protein [PDB 4RS1] |
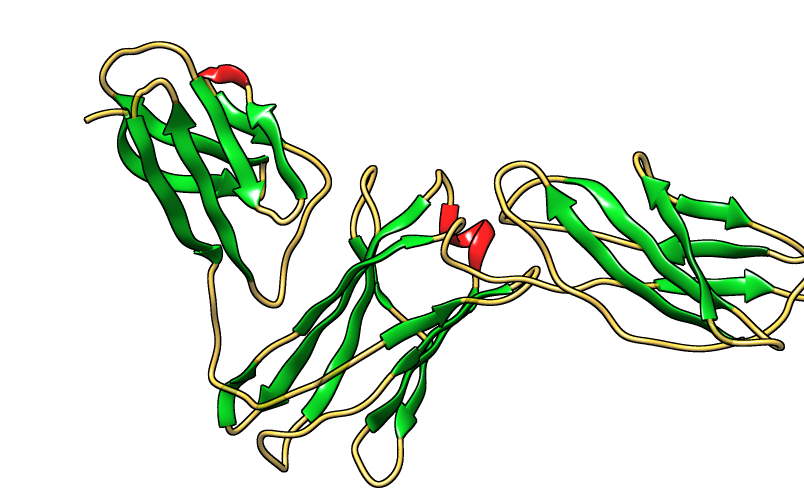 | Figure 29. Three dimensional structure of IL-3Rα protein [PDB 4jzj] |
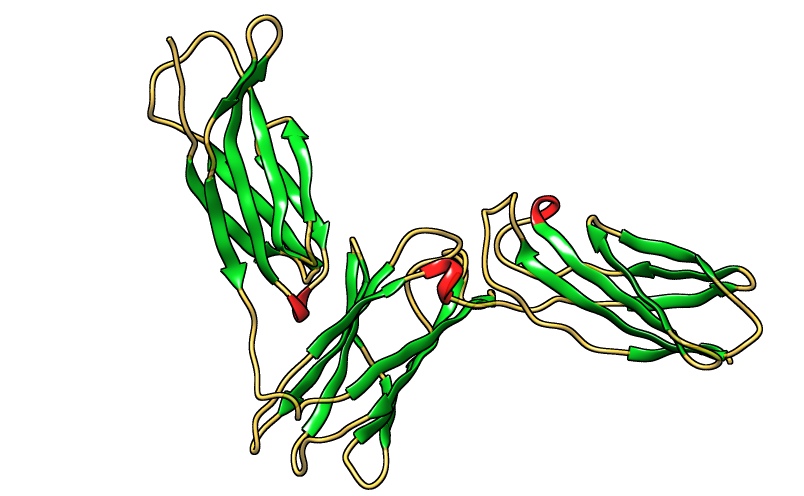 | Figure 30. Three dimensional structure of IL-5Rα protein [PDB 3va2] |
3.15. Validation of Proteins
The validation of the modeled structure was carried out using ERRAT. The results are shown in figure 31, 32 and 33.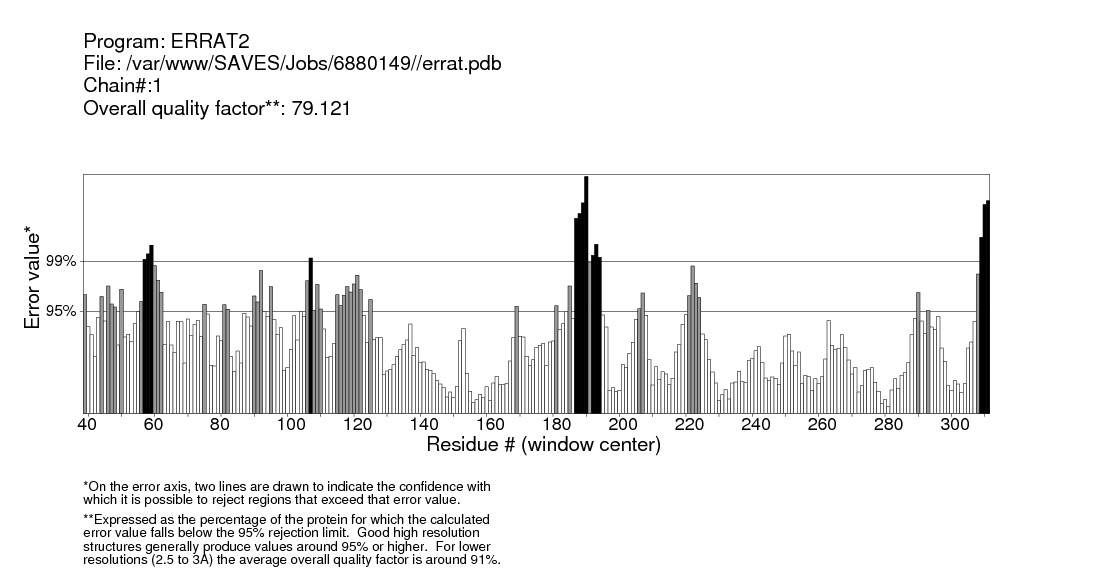 | Figure 31. Validation ofGM-CSF-Rα protein by ERRAT server |
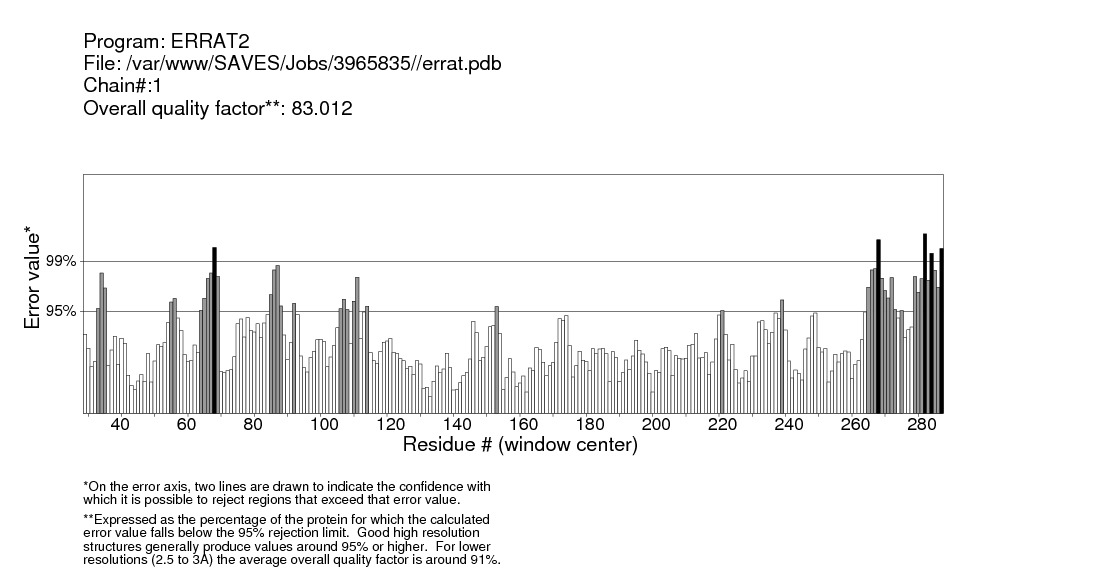 | Figure 32. Validation of IL-3Rα protein by ERRAT server |
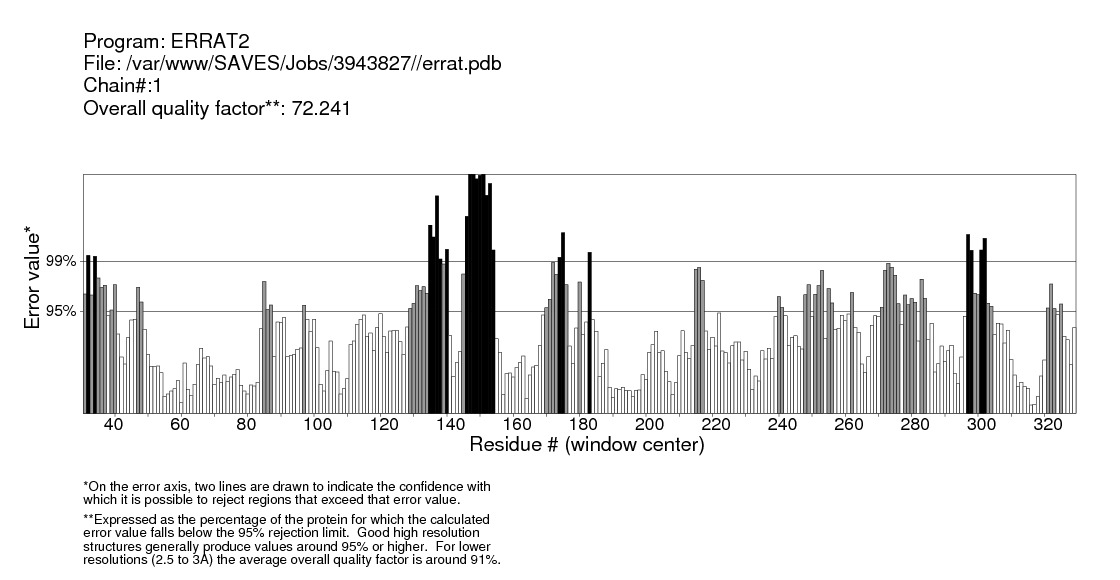 | Figure 33. Validation of IL-5Rα protein by ERRAT server |
4. Discussion
Computational analysis of protein sequences has become a highly rich scope of renewed science and a highly interdisciplinary area, where statistical and algorithmic procedures have a significant role. The present study was to perform sequence and structure analysis of three proteins, GM-CSF-Rα, IL3Rα, and IL5Rα. ProtParam software was used to find out the physiochemical properties for the proteins from their sequences, which are essential for understanding proteins function. Leucine (Leu) amino acid was found in rich amounts in these proteins, while Pyrrolysine (Pyl) and Selenocysteine (Sec) were absent. This may explain the high aliphatic index (AL) of these proteins, indicating that they are stable for a wide range of temperature. An isoelectric point above 7 (7.91 for GM-CSF-Rα and 8.60 for IL-3Rα) as well as a higher number of positive residues (+R) indicates that these proteins has a positive charge, whereas IL5Rα protein which is below 7 (5.36) and a higher negative residues (-R) has a negative charge. This value, computed isoelectric point, has an advantage in developing buffer system for purification by isoelectric focusing method [31]. The Instability index (II) less than 40 in GM-CSF-Rα indicates that it may be stable for a wide range of temperatures whereas the IL3Rα, and IL5Rα classified as an unstable proteins. The lower value of (GRAVY) in all proteins may be a signal for the possibility of better interaction with water like a protein of hydrophilic nature. The N-terminal of these proteins sequences considered is M (Met). Therefore estimated half-life is 30 hours (mammalian reticulocytes, in vitro), >20 hours (yeast, in vivo) and >10 hours min (Escherichia coli, in vivo) [31]. Another parameter, extinction coefficient (EC) at 280 nm. EC, which is important in the quantitative study of protein–protein and protein–ligand interactions in solution, is calculated from amino acids composition and found to be higher among these proteins. Fingerprinting analysis was performed by ScanProsite detecting Fibronectin type III (FN3) domain in GM-CSF- Rα and IL-5Rα proteins. These domains are found in many different proteins including cell surface receptors and cell adhesion molecules [32]. Koide A. and coworkers revealed that it is a small independent folding unit which occurs in many animal proteins involving in ligand binding. The beta-sandwich structure of FN3 exceedingly look alike that of immunoglobulin domains [33].Protein signatures are dynamic mining tool eligible to identify protein sequences having the same functional residues, belonging to the same class of proteins from the numerous sequences in the non-redundant databases [14]. Among these three proteins, sequences belonging to the following families were detected: HEMATOPO_REC_S_F2, ASN_GLYCOSYLATION, CK2_PHOSPHO_SITE, PKC_PHOSPHO_SITE, MYRISTYL, CAMP_PHOSPHO_SITE, and TYR_PHOSPHO_ .SOSUI server classified all proteins as membrane proteins, primary and secondary in nature. And the transmembrane region of all proteins is rich in hydrophobic amino acids. The helix of proteins is visualized using PepWheel. Another substantial aspect of the protein analysis concerns post-translational modifications (PTMs). They are known to be essential mechanisms in the eukaryotic cells associated with protein functions and signaling networks. A growing body of evidences suggested that the complex signaling networks involved in the regulation of cellular pluripotency are strictly controlled by multiple mechanisms, including post-translational modifications (PTMs) [34]. Therefore, it is important to use bioinformatics tools to predict the sites for post translational modifications in proteins analysis. Protein phosphorylation is one of the most abundant post-translational modifications. It is implicated in the regulation of many cellular processes and states. Many signaling pathways involved in the embryonic development and the modulation of gene expression for cellular pluripotency and differentiation are starting from the activation of growth factor receptors that are recognized receptor tyrosine kinases (RTKs; e.g., FGFR and IGF1R) or receptor serine/threonine kinases (e.g., TGFβR and BMPR1/2). [34]. Phosphorylation is the most common and important mechanism of acute and reversible regulation of protein function. Protein phosphorylation has a significant role in essentially all aspects of cell biology. Most polypeptide growth factors and cytokines stimulate phosphorylation upon binding to their receptors [35].The phosphorylation site prediction showed that serine is the most phosphorylated amino acid among these proteins, with different kinases for each protein. cdc2, Unsp, PKA, PKC, DNAPK, ATM, CKII and PKG kinases acting on GM-CSF-Rα protein, while PKG, cdc2, PKA, CKII, Unsp, PKC, PKC, RSK, DNAPK, ATM, CKI and PKA, Unsp, PKC, cdc2, GSK3, cdk5, p38MAPK, CKI, RSK, DNAPK, ATM, CKII acting on IL-3Rα and IL-5Rα proteins respectively. Ubiquitination is an important and popular protein posttranslational modification than earlier expected. Regulation of transcription factor activity, budding of retroviral virions, receptor endocytosis and lysosomal trafficking, control of insulin6 and TGF-β signaling pathways are examples of just a few processes that depend on ubiquitination. [22]. UbPred predicted that 2 lysine residues in GM-CSF-Rα undergo ubiquitination. In contrast, BDM-PUB predicted that 13 lysine residues undergo ubiquitination. Both UbPred and BDM-PUB predicted that residue (K 387) undergo ubiquitination. Similarly, UbPred predicted that 3 lysine residues in IL3Rα undergo ubiquitination, BDM-PUB predicted 6, and both predicted (K361). For IL5Rα, UbPred predicted 4, BDM-PUB predicted 9, and (K393) predicted by both of them. Small ubiquitin-like modifiers (SUMOs) play an essential role in the regulation of a variety of biological processes such as cellular signaling by modifying specific lysine residues in protein substrates. There are numerous clues that the aberrance of SUMO regulation is extremely associated with various diseases, such as cancers. Thus, the identification of SUMO modification sites in proteins is essential for understanding the biological functions and regulatory mechanisms of SUMOs, and provides possible targets for further diagnostic and therapeutic considerations. The process by which proteins being covalently modified by SUMOs is named sumoylation, which is one of the most significant and ubiquitous post-translational modifications (PTMs) of proteins [23]. In the present study; 2 residues in GM-CSF-Rα, 3 in IL-3Rα and 4 in IL-5Rα proteins are possible sumoylation sites detected by GPS-SUMO online service. The prediction of signal peptides has become a substantial application of genomics and proteomics studies. After translocation of the protein across the cell membrane, the N-terminal signal peptide is usually cleaved off by an extracellular signal peptidase. The cleavage site for the signal peptidase is located in the c-region. However, the degree of signal sequence conservation and length, as well as the cleavage site position, differs significantly between different proteins. Furthermore, main variations were observed between eukaryotic and bacterial signal sequences. So, for different objectives, it is advisable to recognize signal peptides and their corresponding cleavage positions [24]. According to Predisi software, the three proteins examined here were predicted for secretion, with different scores and cleavage sites. Detection of protein–ligand binding sites is important to protein function annotation and drug designing. Xing Du et al defined “ligand” as any molecule capable of binding to a protein with a high specificity and affinity [36]. It is important to understand thoroughly the protein-ligand interactions in order to give a deep insight into the protein function. In addition, such understanding can facilitate the discovery, design, and development of drugs [36]. For GM- CSF-Rα; 3 binding sites have been detected and visualized by PLIP & LIGPLOT. Likewise, 5 binding sites have been detected for IL-3- Rα and IL-5- Rα. Protein secondary structures are steady local conformations of a polypeptide chain. They are significant in preserving a protein three-dimensional structure. Secondary structure prediction for these proteins showed that Beta sheet dominated all the other conformations. Protein 3D structure is very important in understanding the protein interactions, functions and their localization. Homology modeling approach is the most common structure prediction method. CPH server for Homology modeling and Chimera for visualization of these models were utilized in this study. Reliability of these models were further checked by ERRAT, where a model having more than 90% residues in suitable region is considered as good quality model. Results from ERRAT showed low quality of these sequences (less than 90%). It remains to be seen whether the lessons learned from this study can be applied to other members of this cytokine receptor super family.
5. Conclusions
Computer-assisted description of the features of various proteins is an important mission in the search for proteomes knowledge. The structural and functional analysis of the α subunits of these receptors provides an insight into their mechanism of activation and for the development of therapeutics. Further work is now needed to extend these observations in order to support advances in therapeutic options. Also, comparison In silico analysis between α and β subunits is required to help understanding the role of α subunits in the overall function of these receptors.
ACKNOWLEDGEMENTS
This work was carried out with the support of National University Research Institute.
References
[1] | Athanasia Pavlopoulou and Ioannis Michalopoulos: State-of-the-art bioinformatics protein structure prediction tools. International journal of molecular medicine 28: 295-310. DOI: 10.3892/ijmm.2011.705. (2011). |
[2] | Edwards YJ and Cottage A. Bioinformatics methods to predict protein structure and function. A practical approach. Mol Biotechnol. 23(2): 136-66.2003. DOI:10.1385/MB:23:2:139. |
[3] | Goodall GJ, Bagley CJ, Vadas MA, Lopez AF. A model for the interaction of the GM-CSF, IL-3 and IL-5 receptors with their ligands. Growth Factor. 8:87.1993. PMID: 8466757. |
[4] | Toshio Kitamura, Atsushi Miyajima. (1992) Functional Reconstitution of the Human Interleukin-3 Receptor. Blood. 80: 84-90. |
[5] | Sakamaki K, Miyajima I, Kitamura T, Miyajima A. Critical cytoplasmic domains of the common beta subunit of the human GM-CSF, IL-3 and IL-5 receptors for growth signal transduction and tyrosine phosphorylation. EMBO J. Oct; 11(10):3541-9. 1992. PMCID: PMC55681. |
[6] | Hansen G et al. The structure of the GM-CSF receptor complex reveals a distinct mode of cytokine receptor activation. Cell, 8; 134(3):496-507. 2008. 10.1016/j.cell.2008.05.053. |
[7] | Hercus. et al. The granulocyte-macrophage colony-stimulating factor receptor: linking its structure to cell signaling and its role in disease. Blood. 114:1289-1298.2009. DOI 10.1182/blood-2008-12-164004. |
[8] | Sophie E. Broughton . et al . Dual Mechanism of Interleukin-3 Receptor Blockade by an Anti-Cancer Antibody. Cell Reports. 8, 410–419. 2014. http://dx.doi.org/10.1016/j.celrep.2014.06.038. |
[9] | Taku Kouro, Kiyoshi Takatsu. IL-5- and eosinophil-mediated inflammation: from discovery to therapy. International Immunology, Vol. 21, No. 12, pp. 1303–1309. 2009. doi:10.1093/intimm/dxp102. |
[10] | J. FernandoBazan. Structural design and molecular evolution of a cytokine receptor superfamily. Proc. Natl. Acad. Sci. USAVol. 87, pp. 6934-6938.1990. |
[11] | Toshio Kitamura, Atsushi Miyajima. Functional Reconstitution of the Human Interleukin-3 Receptor. Blood. 80: 84-90. 1992. www.bloodjournal.org. |
[12] | The UniProt Consortium. UniProt: a hub for protein information. Nucleic Acids Research, 43: 204-212. Database issue.2015. doi:10.1093/nar/gku989. |
[13] | Sheoran. S, Pandey. B, Sharma. P, et al.In silico comparative analysis and expression profile of antioxidant proteins in plants. Genet. Mol. Res. 12 (1): 537-551.2013. http://dx.doi.org/10.4238/2013.February.27.3. |
[14] | Rost.B, Eyrich. V. Large-scale analysis of secondary structure prediction. Proteins. Suppl. 2001. PMID:11835497 5:192-9. |
[15] | Prajapati. C, Bhagat. C.In-Silico Analysis and Homology Modeling of Targets Proteins for Clostridium botulinum. International Journal Pharmaceutical sciences and research. 3: 2050-2056. 2012. DOI: http://dx.doi.org/10.13040/IJPSR.0975-8232.3(7).2050-56. |
[16] | Mundaganore S., Mundagnore D, Ashokan V. In Silico Validation OF Middle East Respiratory Syndrome (MERS) Virus Protins for Better Drug Development. International Journal of Applied Sciences and Biotechnology. 1: 272-278. 2013.DOI: 10.3126/ijasbt.v1i4.9184. |
[17] | K. Guruprasad, B. Reddy, M. Pandit. Correlation between stability of a protein and its dipeptide composition: a novel approach for predicting in vivo stability of a protein from its primary sequence. Protein Engineering. 4: 155-161.1990. PMID:2075190. |
[18] | Ikai A. Thermostability and aliphatic index of globular proteins. Journal of Biochemistry. 88: 1895-1898.1980. DOI: https://doi.org/10.1093/oxfordjournals.jbchem.a133168. |
[19] | Kyte J and Doolittle R. A simple method for displaying the hydropathic character of a protein. Journal of Molecular Biology. 1982. 157: 105-132. |
[20] | Castro E. de, Sigrist. C.J., Gattiker. A, et al. ScanProsite: detection of PROSITE signature matches and ProRule-associated functional and structural residues in proteins. Nucleic Acids Research.34: 362-365.2006. DOI:10.1093/nar/gkl124. |
[21] | Blom. N. Gammeltoft. S, Brunak. S. Sequence- and structure-based prediction of eukaryotic protein phosphorylation sites. Journal of Molecular Biology. 294:1351-1362. 1999. DOI:10.1006/jmbi.1999.3310. |
[22] | Radivojac .P, Vacic, V, Haynes C, Cocklin, R.R, Mohan, A, et al. Identification, analysis, and prediction of protein ubiquitination sites. Proteins. 78: 365–380.2010. doi: 10.1002/prot.22555. |
[23] | Qi Zhao, Yubin Xie, Yueyuan Zheng. GPS-SUMO: a tool for the prediction of sumoylation sites and SUMO-interaction motifs. Nucleic Acids Research, 42.325-330.2014. doi: 10.1093/nar/gku383. |
[24] | Karsten Hiller, Andreas Grote, Maurice Scheer, Richard Mu¨nch and Dieter Jahn. PrediSi: prediction of signal peptides and their cleavage positions. Nucleic Acids Research, Vol. 32, Web Server issue W375–W379.2004. DOI: 10.1093/nar/gkh378. |
[25] | Tjaart A. P. de Beer, etal. PDBsum additions. Nucleic Acids Research,42:292–296. 2014. doi:10.1093/nar/gkt940. |
[26] | Wallace AC1, Laskowski RA, Thornton JM. LIGPLOT: a program to generate schematic diagrams of protein-ligand interactions. Protein Eng. 8(2):127-34. 1995.PMID:7630882 |
[27] | Sebastian Salentin, Sven Schreiber, V. Joachim Haupt, Melissa F. Adasme, and Michael Schroeder. PLIP: fully automated protein–ligand interaction Profiler. Nucleic Acids Research, 43, Web Server issue W443–W447.2015. doi: 10.1093/nar/gkv315. |
[28] | Nielsen M., Lundegaard C., Lund O., Petersen TN. CPHmodels-3.0 - Remote homology modeling using structure guided sequence profiles. Nucleic Acids Research. 38, 2010. doi:10.1093/nar/gkq535. |
[29] | Pettersen EF, Goddard TD, Huang CC, Couch GS, Greenblatt DM, Meng EC, Ferrin TE. 2004 UCSF Chimera--a visualization system for exploratory research and analysis. J Comput Chem. 25(13):1605-12.2004. DOI 10.1002/jcc.20084. |
[30] | Ujala Sehar, Muhammad Aamer Mehmood, Salman Nawaz, Shahid Nadeem, Khadim Hussain, Iqra Sohail, Muhammad Rizwan Tabassum, Saba Shahid Gill, and Anam Saqib. Three dimensional (3D) structure prediction and substrate-protein interaction study of the chitin binding protein CBP24 from B. thuringiensis. Bioinformation. 2013. PMCID: PMC3746096. |
[31] | Sofia B. Mohamed and Mohamed M. Hassan. Insilico Validation of Babesia Bovis Merozoite Surface Antigen-1, Merozoite Surface Antigen-2b and Merozoite Surface Antigen-2c Proteins for Vaccine and Drug Development. International Journal of Bioinformatics and Biomedical Engineering. 2(1): pp. 30-39. 2016. ISSN: 2381-7402. |
[32] | Leahy DJ, Hendrickson WA, Aukhil I, Erickson HP. (1992) Structure of a fibronectin type III domain from tenascin phased by MAD analysis of the selenomethionyl protein. Science. 6;258 (5084):987-91.1992. PMID:1279805. |
[33] | Koide A1, Bailey CW, Huang X, Koide S. The fibronectin type III domain as a scaffold for novel binding proteins. J Mol Biol. 284(4):1141-51.1998. DOI:10.1006/jmbi.1998.2238. |
[34] | Sefton BM. Overview of protein phosphorylation. Curr Protoc Cell Biol. Chapter 14: Unit 14.1.2001. doi: 10.1002/0471143030.cb1401s00. |
[35] | Wang, Y.-C., Peterson, S. E., & Loring, J. F. Protein post-translational modifications and regulation of pluripotency in human stem cells. Cell Research, 24(2), 143–160. 2014. http://doi.org/10.1038/cr.2013.151. |
[36] | Xing Du etal. Insights into Protein–Ligand Interactions: Mechanisms, Models, and Methods. Int. J. Mol. Sci.17: 144. 2016. doi:10.3390/ijms17020144. |