Nahla E. Abdelraheem1, Ghada M. El-Tayeb2, Lamia O. Osman3, Samar A. Abedlrhman3, Aisha S. Ali4, Ahmed H. Elsadig5, Sofia B. Mohamed6
1Department of Histopathology, National University, Khartoum, Sudan
2Department of Pharmaceutical Chemistry, National University, Khartoum, Sudan
3Department of Clinical Chemistry, National University, Khartoum, Sudan
4Department of Microbiology, National University, Khartoum, Sudan
5Faculty of Medicine, University of Khartoum, Khartoum, Sudan
6Department of Vector and biomedical studies, Tropical Medicine Research Institute, Khartoum, Sudan
Correspondence to: Sofia B. Mohamed, Department of Vector and biomedical studies, Tropical Medicine Research Institute, Khartoum, Sudan.
Email: |  |
Copyright © 2016 Scientific & Academic Publishing. All Rights Reserved.
This work is licensed under the Creative Commons Attribution International License (CC BY).
http://creativecommons.org/licenses/by/4.0/

Abstract
The protein product of the normal KRAS gene performs an essential function in normal tissue signaling, and the mutation of a KRAS gene is an essential step in the development of many cancers. Despite the reported association of KRAS gene mutations with human disease susceptibility, the comprehensive computational analysis of coding, noncoding and regulatory SNPs, and their functional impacts on protein level, still remains unknown. In this study, we performed a comprehensive analysis of the functional and structural impact of non-synonymous (nsSNP) that are deleterious to KRAS structure and function. A sequence homology-based approach was adopted for screening nsSNPs in KRAS, including nine different in silico prediction algorithms; SIFT, PROVEAN, MutPred, SNP&GO, PhD-SNP, PANTHER, I-Mutant 2.0, MUpo and DUET. Moreover, to understand how mutations can affect the strength of the interactions that bind proteins together we submitted data to ELASPIC servers and evolutionary conservation data was obtained from the ConSurf web server. The PDB structure of KRAS protein was obtained from RCSB Protein Data Bank (PDB ID: 4QL3) and Structural theoretical models of KRAS were created using mutation3D and chimera servers. Our results demonstrate that 14 nsSNPs in the antiviral KRAS gene may be deleterious to KRAS structure and function.14 deleterious and high-risk nsSNPs were identified in KRAS gene. 11 of these are located at highly conserved amino acid sites in the protein domain (RAS). Additionally, we detected 4 nsSNPs in the core residues of the protein and 10 SNPS at interface. Finally, we classified six SNPs as top high-risk (Q61E, A146T, N116S, K147E, G60R and A59V) which may alter the putative structure of KRAS’s domain, particularly in G1, G2, G3, G4 and G5 motif regions. These same regions are critical to the protein's structure and function. This study adopted an extensive in silico analysis of the highly polymorphic KRAS gene and will be a valuable resource for future targeted mechanistic and population-based studies.
Keywords:
KRAS gene, Insilico analysis, MutPred, PhD-SNP, DETU, ELASPIC, Mutation3D and UCSF chimera program
Cite this paper: Nahla E. Abdelraheem, Ghada M. El-Tayeb, Lamia O. Osman, Samar A. Abedlrhman, Aisha S. Ali, Ahmed H. Elsadig, Sofia B. Mohamed, A Comprehensive in Silico Analysis of the Functional and Structural Impact of Non-Synonymous Single Nucleotide Polymorphisms in the Human KRAS Gene, American Journal of Bioinformatics Research, Vol. 6 No. 2, 2016, pp. 32-55. doi: 10.5923/j.bioinformatics.20160602.02.
1. Introduction
Single Nucleotide Polymorphism, also known as SNP, accounts for the most common form of genetic mutation in human. It has been reported that ∼93% of all human genes represent at least one SNP [1]. Therefore, they are liable for generating the majority of biological variations among individuals. An understanding of the relationship between these genetic variations and their phenotypic effects could therefore be a step toward exploring the causes of various disorders or diseases. SNPs can fall within the coding regions (coding SNPs) or noncoding regions of genes (noncoding SNPs), or in the intergenic region between two genes [2, 3]. While the two others are quite natural in the human genome and phenotypically neutral [4], nonsynonymous coding SNPs (nsSNPs) are thought to have the principal impact on phenotype by changing the protein sequence. As they cause amino acid alteration in the corresponding protein product, it may exert deleterious effects on the structure, function, solubility, or stability of proteins [5]. Beside these the nsSNPs perturb gene regulation by modifying DNA and transcriptional binding factors [6] and the maintenance of the formational integrity of cells and tissues [7]. Thus, it is likely that nsSNPs play a major role in the functional diversity coded proteins in human populations and often associated with human diseases. Indeed, earlier studies have revealed that more than 50% of the mutations associated with inherited genetic disorders are resulted by nsSNPs [8]. Disease-causing mutations are frequently observed at either core or interface residues mediating protein interactions. Mutations at core residues frequently destabilize protein structure while mutations at interface residues can specifically affect the binding energies of protein-protein interactions. Missense mutations at protein–protein interaction sites, called interfaces, are important contributors to human disease. Interfaces are non-uniform surface areas characterized by two main regions, “core” and “rim”, which differ in terms of evolutionary conservation and physicochemical properties. Moreover, within interfaces, only a small subset of residues (“hot spots”) is crucial for the binding free energy of the protein–protein complex [9]. Protein-protein interactions are critical for nearly every process in the cell and deleterious mutations hindering these interactions can have severe consequences for the associated cellular function. A variety of efforts from personalized medicine to understand viral evolution require knowing how specific mutations effect the protein-protein interactions. Conversely, designing proteins with improved binding or altered specificity requires that the impact of mutations on the native interface be understood. Currently this information is not available experimentally on the proteome-wide scale necessary for these tasks. Towards this end, considerable effort has been devoted towards developing methods to predict the impact of mutations on binding affinity. Most of these approaches rely on physics based methods that attempt to faithfully model on the atomic level the interactions determining protein-protein binding affinity. However, a major obstacle of such approaches is the need for the reconstruction of the full-atomic model for every mutant complex, which limits the accuracy of the approach (since the position of the side-chains is difficult to model) and reduces the computational speed and the range of applications(since rebuilding the full-atomic model is generally them most time-consuming step) [10]. The KRAS (Kirsten rat sarcoma viral oncogene homolog) (ID: 3845) gene belongs to a family of oncogenes called the Ras family, located on the short (p) arm of chromosome 12 at position 12.1 and consists of six exons. It provides instructions for making a protein called K-Ras protein (~21 kDa) which is involved primarily in regulating cell division, differentiation, and apoptosis. As a part of a signaling pathway known as the RAS/MAPK pathway, the protein relays signals from outside the cell to the cell’s nucleus, these signals instruct the cell to grow and divide or to mature and take on specialized functions (differentiate). K-Ras protein is a GTPase; acting like a switch to transmit signals, it must be turned on by attaching to a molecule of GTP, and turned off (inactivated) when it converts the GTP to GDP [11]. A Point mutation in KRAS codons 12 and 13 are predictive of poor prognosis of lung cancer while mutation at codons 61 and 146 were associated with shorter progression-free survival in colorectal cancer patients receiving anti-epidermal growth factor receptor treatment [12]. Bioinformatics has become a very important part of different areas of biology. Bioinformatics tools and techniques to aid in the processing and extraction of useful results from large amounts of raw data; textual mining of biological literature, analysis of gene and protein expression, simulation and modeling of DNA, RNA, and protein structures, comparison of genetic and genomic data and helps analyze and catalogue the biological pathways and networks that are an important part of systems biology [13]. Although there are presently several published articles describing the association of SNPs in the KRAS gene with different types of diseases, computational analysis has not yet been undertaken on the functional and structural consequences of nsSNPs in this gene. In the current study, we employed different publicly available bioinformatics tools and databases for a comprehensive analysis of nsSNPs in KRAS gene. As the majority of disease mutations affect protein stability, we also proposed modeled protein structures of the mutant proteins and compared them with the native protein in order to evaluate stability changes.
2. Material and Methods
2.1. Dataset Collection
The amino acid sequence [Uniprot: P01116] of KRAS protein was obtained from UniProt Protein Database (http://www.uniprot.org). The information about SNPs of KRAS gene of Homo sapiens was obtained from the db-SNP (http://www.ncbi.nlm.nih.gov/SNP/). Structure of KRAS protein [PDB IDs: 4QL3] was retrieved from RCSB Protein Data Bank.
2.2. Non-Synonymous SNPs Analysis
Functional effects of nsSNPs were predicted using the following in silico algorithms: SIFT (http://sift.jcvi.org/), PROVEAN (http://provean.jcvi.org), PhD-SNP (http://snps.biofold.org/phdsnp/phd-snp.html), SNPs&GO (http://snps-and-go.biocomp. unibo.it/snps-and-go/), and MutPred (http://mutpred.mutdb.org/) [14]. nsSNPs predicted to be deleterious by at least four in silico algorithms were categorized as high-risk nsSNPs and were selected for further analysis.
2.2.1. Assessment of the Functional Impacts of Deleterious nsSNPs by SIFT and PROVEAN
SIFT (Sorting intolerant from tolerant)Predicts whether an amino acid substitution affects protein function based on the degree of conservation of amino acid residues in sequence alignments derived from closely related sequences [15]. The SIFT scores range from 0 to 1, and scores ≤0.05 are predicted by the algorithm to be damaging amino acid substitutions, whereas scores >0.05 are considered to be tolerated. PROVEAN (Protein Variation Effect Analyzer):Is a software tool that predicts whether an amino acid substitution has an impact on the biological function of a protein grounded on the alignment-based score [16]. The score measures the change in sequence similarity of a query sequence to a protein sequence homolog between without and with an amino acid variation of the query sequence. If the PROVEAN score ≤-2.5, the protein variant is predicted to have a “deleterious” effect, while if the PROVEAN score is >-2.5, the variant is predicted to have a “neutral” effect.
2.2.2. Prediction of Disease Related Mutations SNPs by SNPs&GO
SNPs&GO [17] is also a support vector machine (SVM) based on the method to accurately predict the mutation related to disease from protein sequence. The input is the FASTA sequence of the whole protein, the output is based on the difference among the neutral and disease related variations of the protein sequence. The RI (reliability index) with value of greater than 5 depicts the disease related effect caused by mutation on the function of parent protein. The PHD SNP and PANTHER, algorithms were also used in the display of output.
2.2.3. Prediction of Harmful Mutations by MutPred
The MutPred server (http://mutpred.mutdb.org/) [18] was employed to classify an amino acid substitution (AAS) as disease-associated or neutral. In addition, it predicts molecular cause of disease/deleterious AAS. MutPred is based upon SIFT and a gain/loss of 14 different structural and functional properties. The output of MutPred contains a general score (g), i.e., the probability that the amino acid substitution is deleterious/disease-associated, and top 5 property scores (p), where p is the P-value that certain structural and functional properties are impacted.
2.3. Protein Stability Analysis
The stability of KRAS protein was predicted using the following in silico algorithms: I-mutant 2.0 (http://folding.biofold.org/i-mutant/i-mutant2.0.html), Upro (http://www.ics.uci.edu/~baldig/mutation. html) and DUET server http://structure.bioc.cam.ac.uk/duet.
2.3.1. Analysis of the Effects of nsSNPs on the Protein Stability by I-Mutant 2.0 and MUpro
I-Mutant 2.0 is a SVM based tools i.e., support vector machine based tool that leads to automatic protein stability change prediction which is caused by single point mutation [19]. The initiations were done either by using protein structure or more precisely from the protein sequence. The output is a free energy change value (ΔΔG). Positive ΔΔG value infers that the protein being mutated is of higher stability and vice versa is also true. MUpro: is also a support vector machine-based tool for the prediction of protein stability changes upon nonsynonymous SNPs. The value of the energy change is predicted, and a confidence score between -1 and 1 for measuring the confidence of the prediction is calculated. A score <0 means the variant decreases the protein stability; conversely, a score >0 means the variant increases the protein stability.
2.3.2. Predicted Change in Folding and Unfolding Free Energy up on Mutation Using DUET Server
DUET: A web server for an integrated computational approach to study missense mutations in proteins. DUET consolidates two complementary approaches (mCSM and SDM) in a consensus prediction; obtained by combining the results of these parate methods in an optimized predictor using Support Vector Machines (SVM).SDM: The method SDM, introduced in relies on amino acid propensities derived from environment-specific substitution tables for homologous protein families that feed a statistical potential energy function and encompass an evolutionary view of the constraints from the immediate residue environment. The approach compares amino acid propensities for the wild-type and mutant proteins in the folded and unfolded states in order to estimate the free energy differences between wild type and mutant. The website is at: http://www-cryst.bioc.cam.ac.uk/ sdm/sdm.php.mCSM: mCSM is a machine learning method to predict the effects of missense mutations based on structural signatures .The mCSM signatures were derived from the graph-based concept of Cutoff Scanning Matrix (CSM), originally proposed to represent network topology by distance patterns in the study of biological systems. mCSM uses a graph representation of the wild-type residue environment to extract geometric and physicochemical patterns (the last represent edinterms of pharmacophores) that are then used to represent the 3D chemical environment during supervised learning. These signatures have been successfully applied in a range of tasks including protein structural classification and function prediction, as well as large-scale receptor based protein ligand prediction [20]. The mCSM website is available at:http://structure.bioc.cam.ac.uk/mcsm.
2.4. Prediction of the Stability Effects upon Mutation in Both, Domain Cores and Domain-Domain Interfaces
ELASPIC is a novel ensemble machine learning approach that predicts the effects of mutations on protein folding and protein-protein interactions. Here we present the ELASPIC web server, which makes the ELASPIC pipeline available through a fast and intuitive interface. The web server can be used to evaluate the effect of mutations on any protein in the Uniprot database, and allows all predicted results, including modeled wild-type and mutated structures, to be managed and viewed online and downloaded if needed. It is backed by a database which contains improved structural domain definitions, and a list of curated domain-domain interactions for all known proteins, as well as homology models of domains and domain-domain interactions for the human proteome. Homology models for proteins of other organisms are calculated on the fly, and mutations are evaluated within minutes once the homology model is available [10].
2.5. Identification of Functional SNPs in Conserved Regions by Using ConSurf Web Server
Evolutionary conservation of amino acid residues in KRAS was determined using the ConSurf web server (consurf.tau.ac.il/). ConSurf-DB provides evolutionary conservation profiles for proteins of known structure in the PDB. Amino acid sequences similar to each sequence in the PDB were collected and multiply aligned using CSI-BLAST and MAFFT, respectively. The evolutionary conservation of each amino acid position in the alignment was calculated using the Rate 4Site algorithm, implemented in the ConSurf web-server. The algorithm takes explicitly into account the phylogenetic relations between the aligned proteins and the stochastic nature of the evolutionary process. Rate 4 Site assigns a conservation level for each residue using an empirical Bayesian inference. Visual inspection of the conservation patterns on the 3-dimensional structure often enables the identification of key residues that comprise the functionally-important regions of the protein [21].
2.6. Structural Analysis
2.6.1. Detection of nsSNPs Location in Protein Structure Using Mutation3D
Mutation3D (http://mutation3d.org) is a functional prediction and visualization tool for studying the spatial arrangement of amino acid substitutions on protein models and structures. It is intended to be used to identify clusters of amino acid substitutions arising from somatic cancer mutations across many patients in order to identify functional hotspots and fuel downstream hypotheses. It is also useful for clustering other kinds of mutational data, or simply as a tool to quickly assess relative locations of amino acids in proteins.
2.6.2. 3D Modeling
RMSD Protein Data Base was used to generate 3D structural models for wild type KRAS (UniProtKB 4QL3) and each of the 13 high-risk nsSNPs in KRAS’s RAS domain.
2.6.3. Modeling Amino Acid Substitution; H-Bonding and Clash
UCSF Chimera is a highly extensible program for interactive visualization and analysis of molecular structures and related data. Chimera (version 1.8) software was used to scan the 3D (three-dimensional) structure of specific protein, and hence modifies the original amino acid with the mutated one to see the impact that can be produced. The outcome is then a graphic model depicting the mutation [22]. Chimera (version 1.8) currently available within the Chimera package and available from the Chimera web site http://www.cgl.ucsf.edu/chimera/.
3. Results
3.1. Retrieval of SNPs
The dbSNP was utilized for retrieving the SNPs in the human KRAS gene. A total of 137 SNPs were founded in the coding region, among them 45 were synonymous, 83 non-synonymous and missense, 6 non-synonymous and nonsense, and 3 frame-shift mutations (Fig. 1). Only missense non-synonymous coding SNPs were chosen for further analysis. 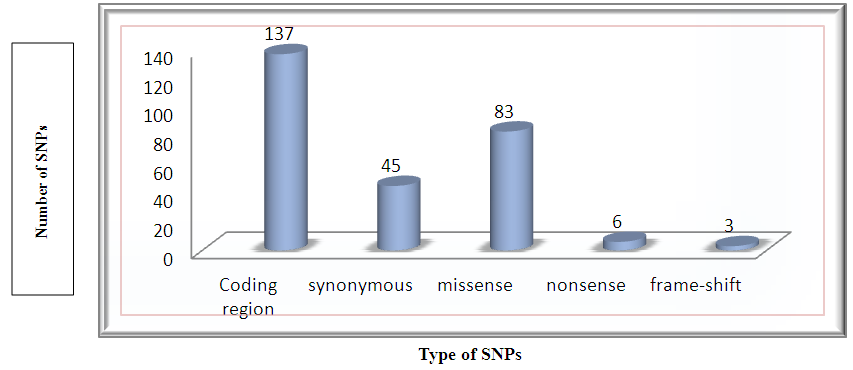 | Figure 1. A graphical representation of the distribution of missense, synonymous, nonsense and frame shift SNPs for KRAS gene (based on the dbSNP database) |
3.2. Prediction of Tolerated and Deleterious nsSNPs
nsSNPs were submitted to the SIFT program to predict their effect on protein function, out of the 83 SNPs screened, 19 variants were found to be deleterious and 8 tolerated. Among the SNPs analyzed, SIFT couldn’t find 57 SNPs.
3.3. Deleterious nsSNPs by PROVEAN Server
Missense nsSNPs were also submitted to the PROVEAN server. 17 SNPs were considered to be Deleterious and Six of them were neutra. A significant correlation between the results obtained from the evolutionary-based approach SIFTS and the structural based approach PROVEAN was detected. Out of the total 15SNPs were predicted as Deleterious by PROVEAN and SIFT suggesting that these nsSNPs may disrupt both the protein function and structure. The detailed result has been depicted in Table 1.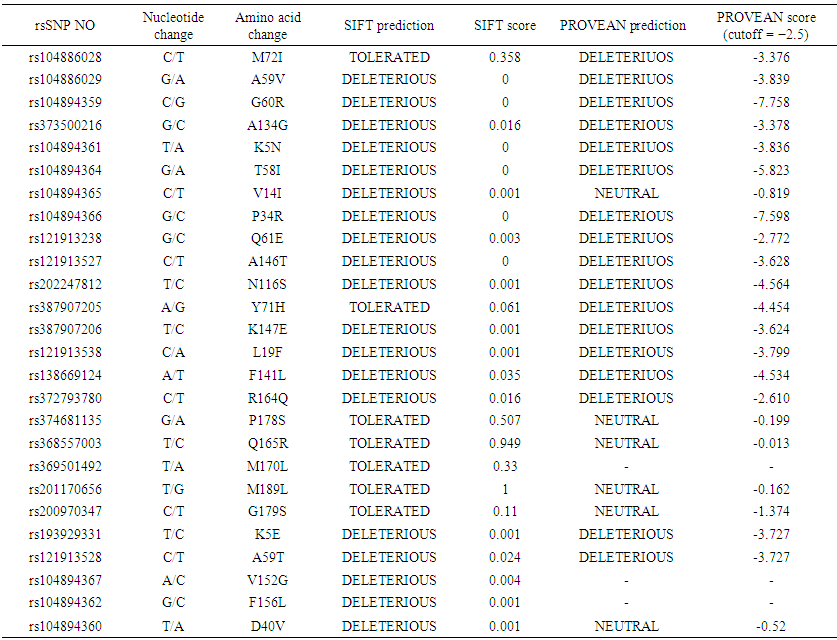 | Table 1. List of non-synonymous SNPs of the human KRAS gene analyzed by SIFT and PROVEAN |
3.4. Damaging nsSNPs Found by SNPs&GO, PHD-SNP and PANTHER
SNPs&GO is a server for the prediction of single point protein mutations likely to be involved in the insurgence of diseases in humans. The results of SNPs & GO for the inputs of all 26 nsSNPs are given in Table 1. The results are displayed in terms of neutral or disease causing mutation. 18out of 26 mutations were predicted to have disease causing abilities while the rest were neutral by PHD-SNP. Also, 12 nsSNPs were disease while the rests were neutral by SNP& GO. PANTHER server was also used. Out of 26 SNPs, the server could not predict the disease causing ability of 11 SNPs. Among the 15 SNPs predicted, 9 were found to be disease causing and the rest were neutral (Table 2).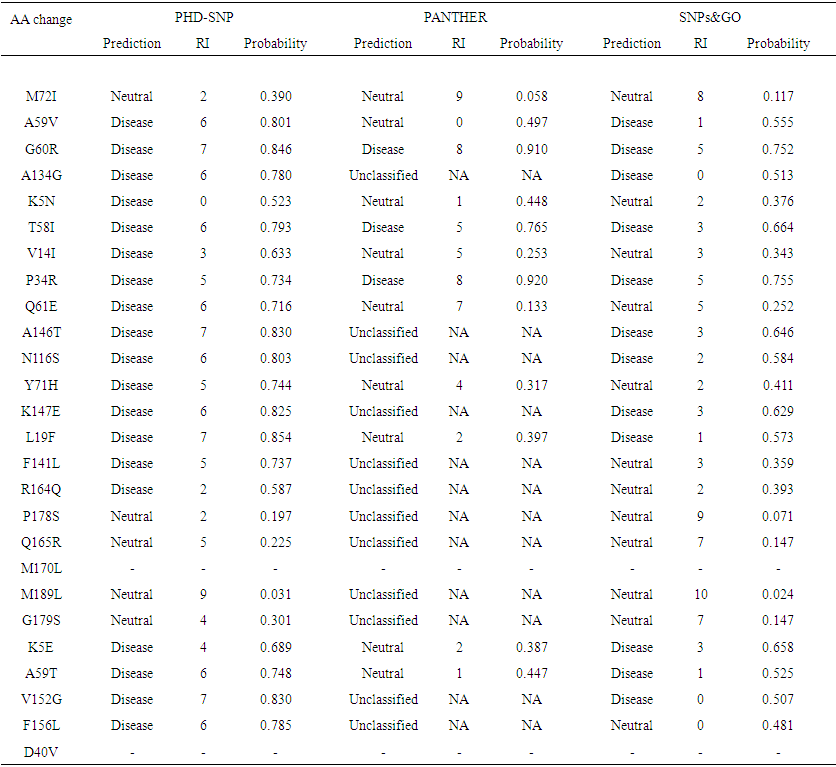 | Table 2. List of non-synonymous SNPs analyzed for disease association by SNP&GO, PHD-SNP and PANTHER |
3.5. Disease Related Amino Acid Substitution Prediction by MutPred
MutPred analysis was done to determine the degree of tolerance for each amino acid substitution on the basis of physio-chemical properties. Table 3 summarizes the result obtained from MutPred server. This implied that some nsSNPs may account for potential structural and functional alterations of KRAS.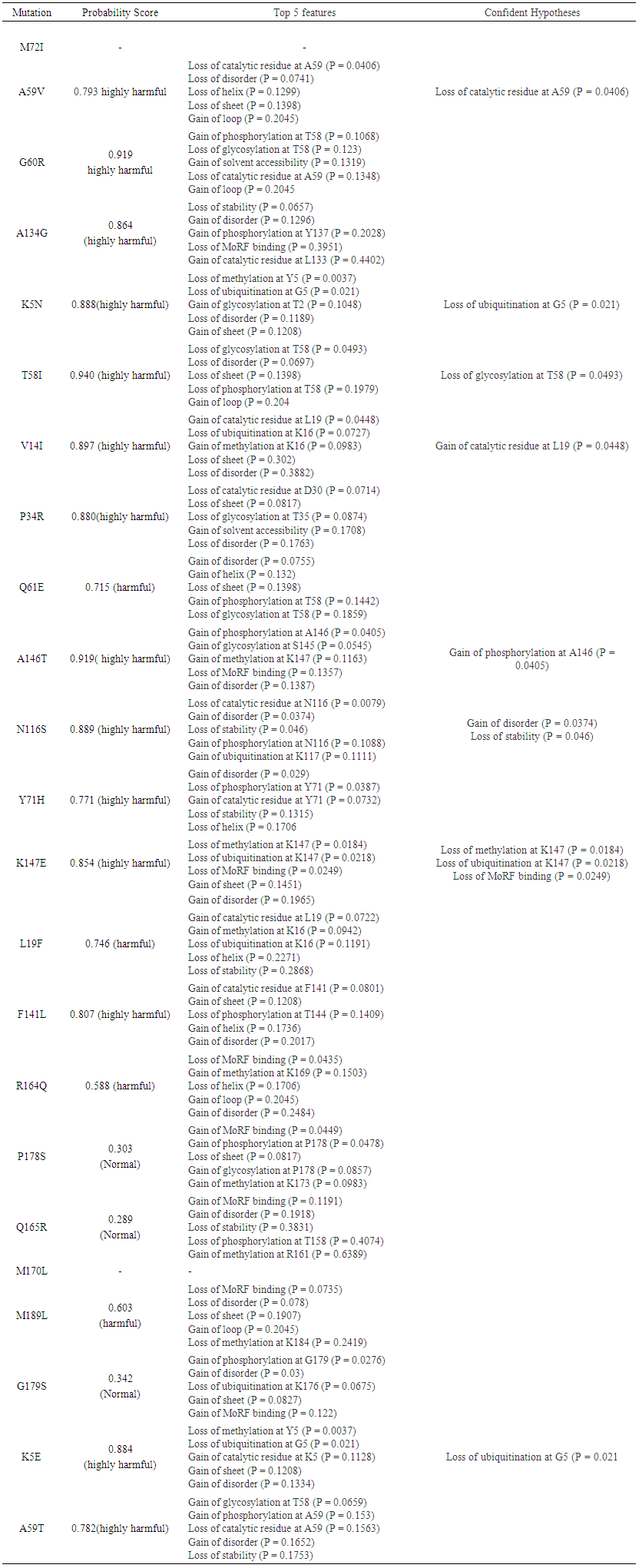 | Table 3. Prediction of functional effects of nsSNPs using MutPred |
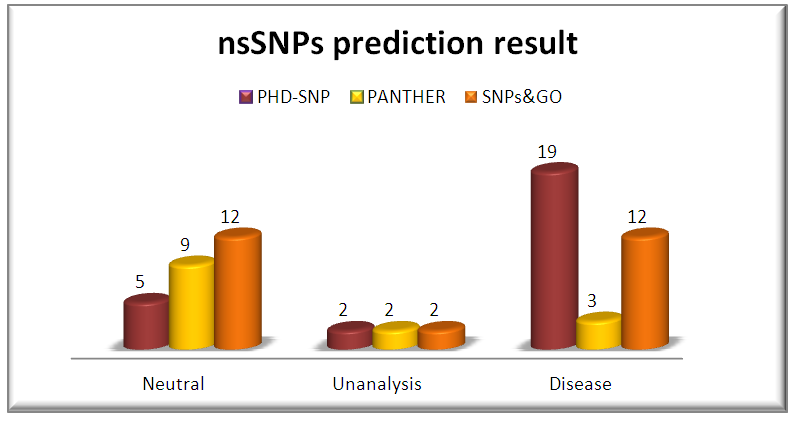 | Figure 2. Graphical representation of Disease variations by PHD-SNP, PANTHER and SNP&GO |
3.6. Identification of Functional nsSNP
Changes in the protein stability of missense variants were examined using I-Mutant 2.0 and MUpro software. The results for the outputs of Deleterious missense SNPs by SIFT and PROVEN analysis (G60R, A134G, K5N, T58I, P34R, Q61E, A146T, N116S, K147E, L19F, F141, R164Q, K5E and A59V) and one SNP was found deleterious by PROVEN and disease by PHD-SNP (Y71H). The predicted results were either increase or decrease of the free energy change upon mutation. 13 out of 14 SNPs were found decreasing for the free energy by I-Mutant and 10 SNPs by Mupro. MUpro couldn't calculate 2 nsSNPs. And Y71H SNP was found decreasing by both softwares. The results are shown in (Table 4).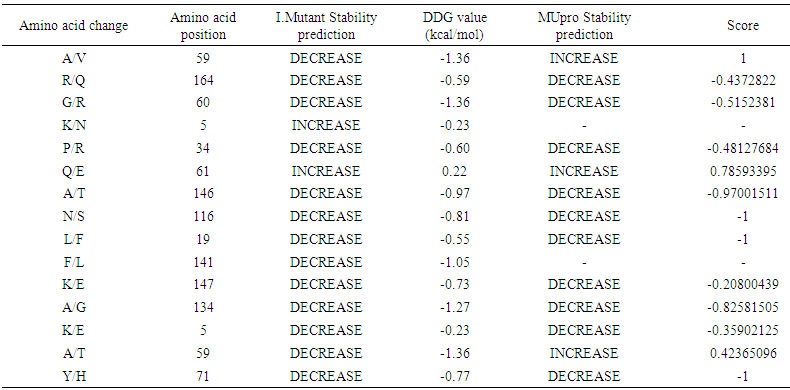 | Table 4. Prediction of mutant protein stability by I-Mutant 2.0 and MUpro |
3.7. Impact of nsSNPS on KRAS Protein Stability
DUET is the effects of nsSNPs on protein stability. The results display the predicted change in folding free energy upon mutation (Ginkcal/mol). A positive value (and red writing) corresponds to a mutation predicted as destabilizing; while a negative sign (and blue writing) corresponds to a mutation predicted as stabilizing. The information displayed includes them CSM(i) and SDM(ii) individually predicted protein stability changes, the combined DUET prediction (iii), a structural summary of the mutation highlighting the wild-type residue and position number, the mutation and its 3D environment (iv). The protein and mutation can also be visualized (v). The results are shown in figures (3-17), each figure shows regression analysis for the stability predictions generated by DUET in comparison with the experimentally measured variation in stability for the considered datasets.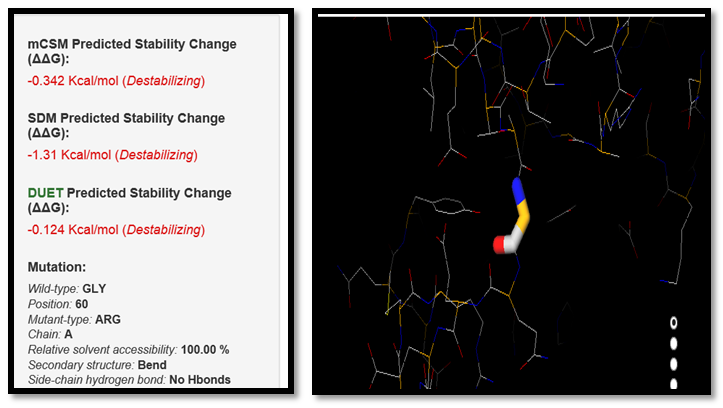 | Figure 3. GLY60ARG SNP analysis of DUET server |
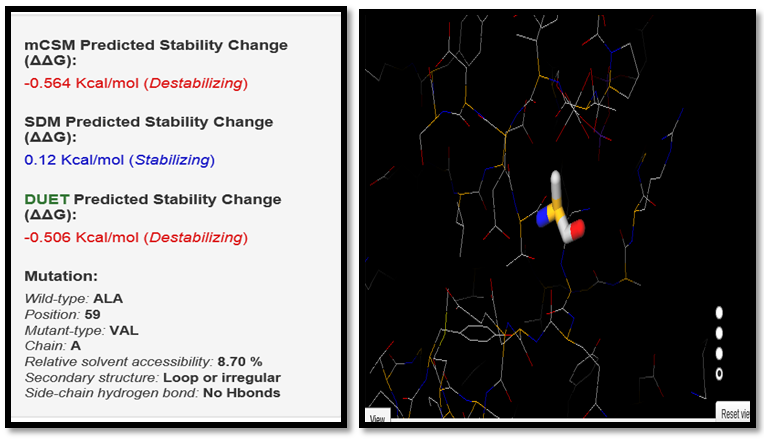 | Figure 4. ALA59VAL SNP analysis of DUET server |
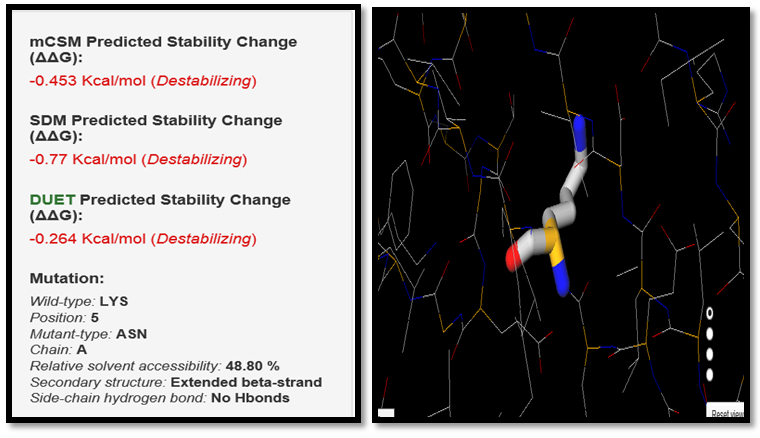 | Figure 5. LYS5ASN SNP analysis of DUET server |
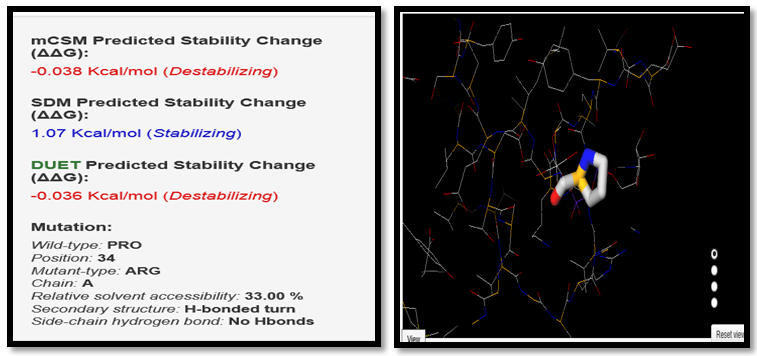 | Figure 6. PRO34ARG SNP analysis of DUET server |
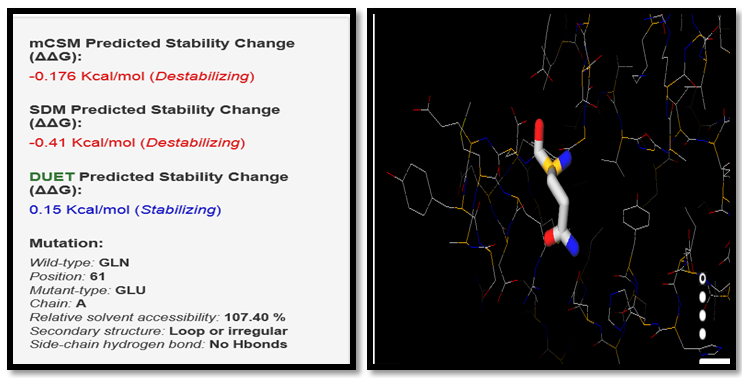 | Figure 7. GLN61GLU SNP analysis of DUET server |
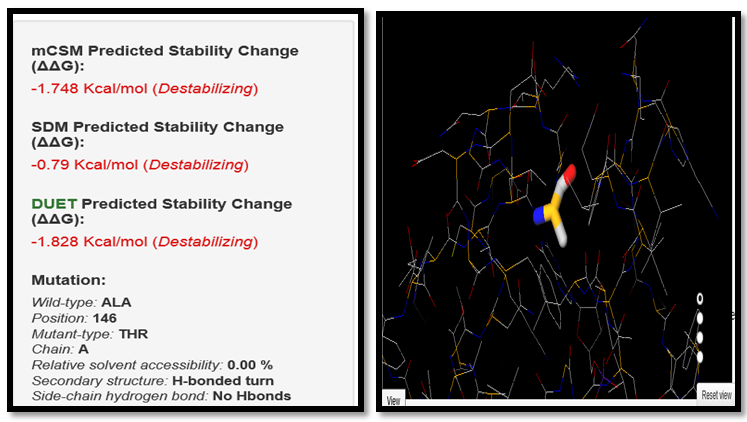 | Figure 8. ALA146THR SNP analysis of DUET server |
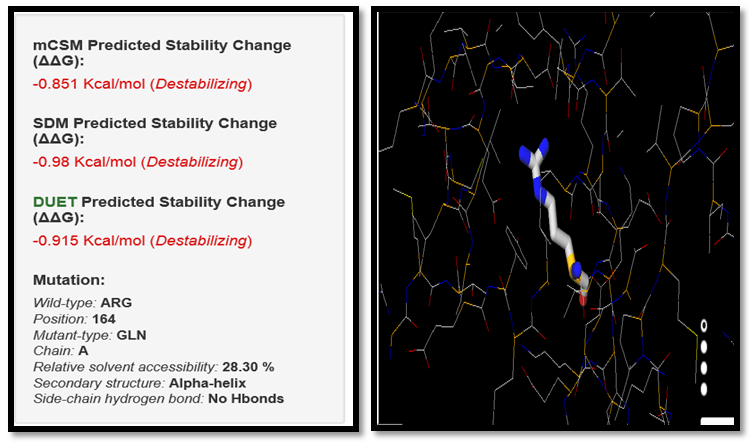 | Figure 9. ARG164GLN SNP analysis of DUET server |
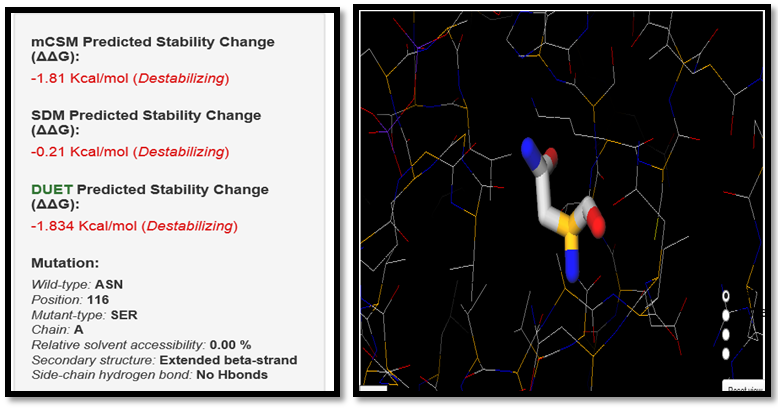 | Figure 10. ASN116SER SNP analysis of DUET server |
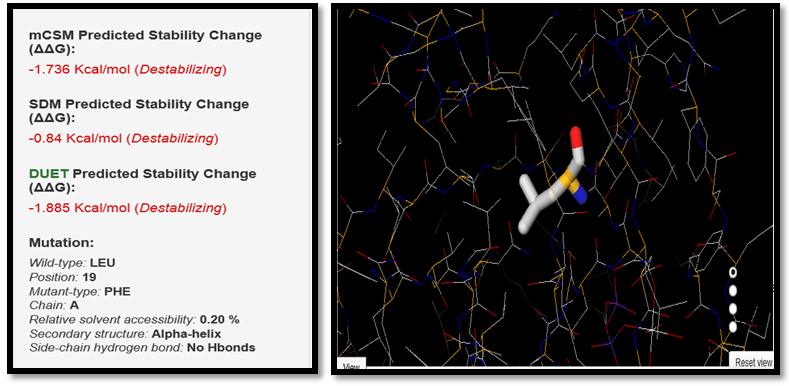 | Figure 11. LEU19PHE SNP analysis of DUET server |
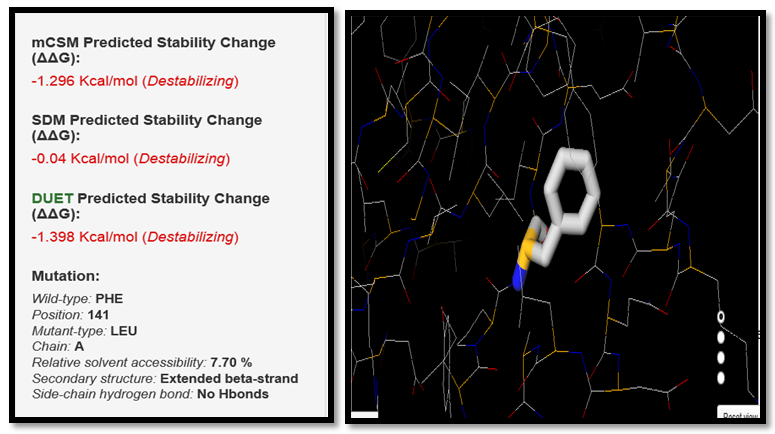 | Figure 12. PHE141LEU SNP analysis of DUET server |
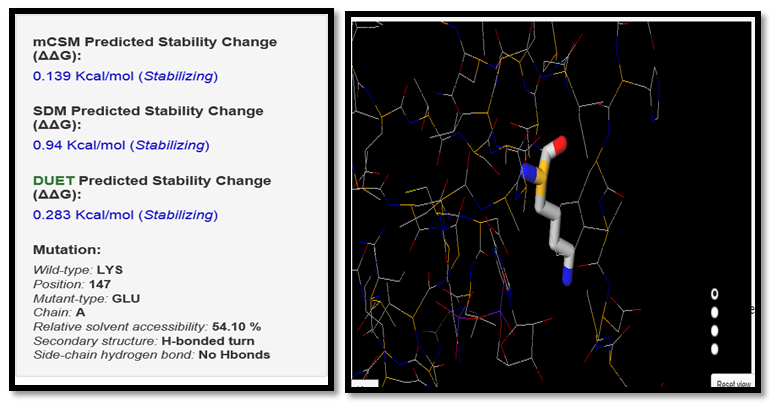 | Figure 13. LYS147GLU SNP analysis of DUET server |
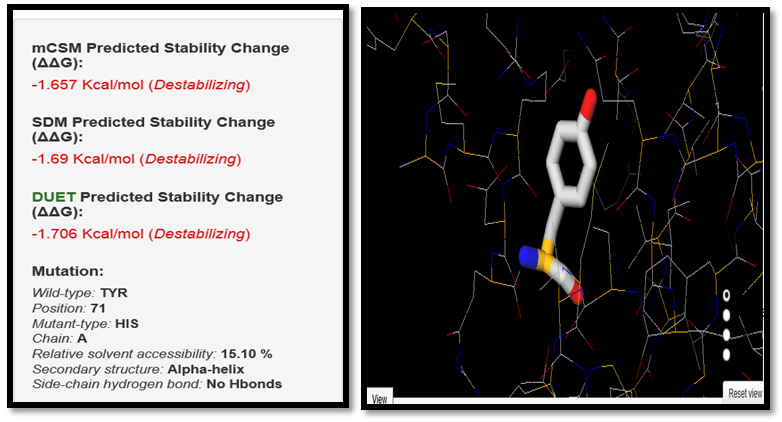 | Figure 14. TYR71HIS SNP analysis by DUET server |
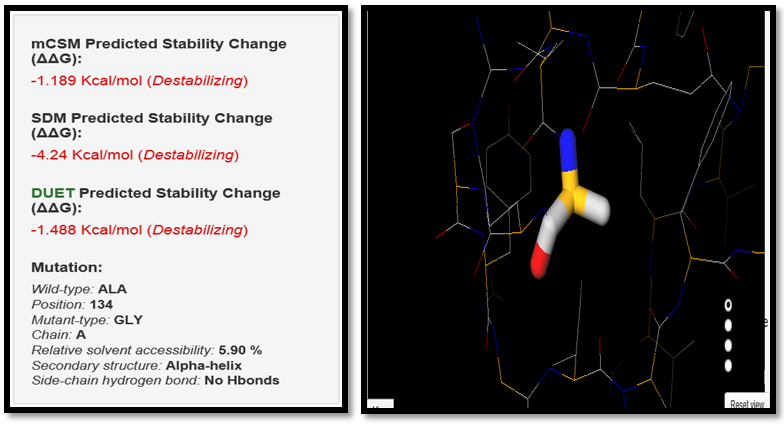 | Figure 15. ALA134GLY SNP analysis of DUET server |
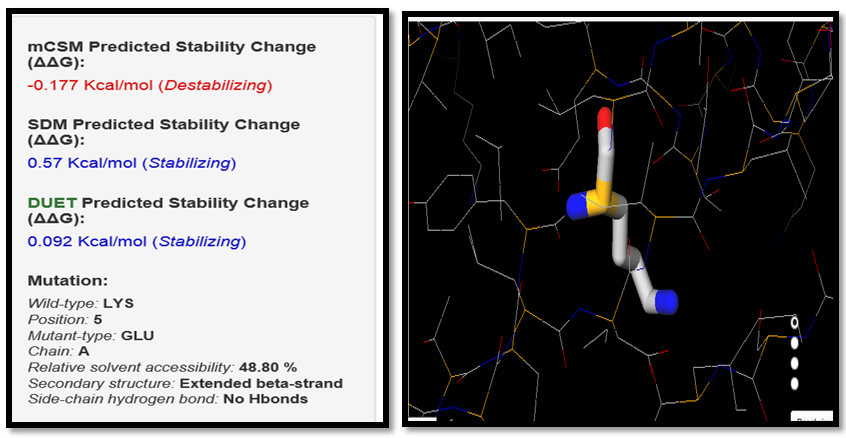 | Figure 16. LYS5GLU SNP analysis of DUET server |
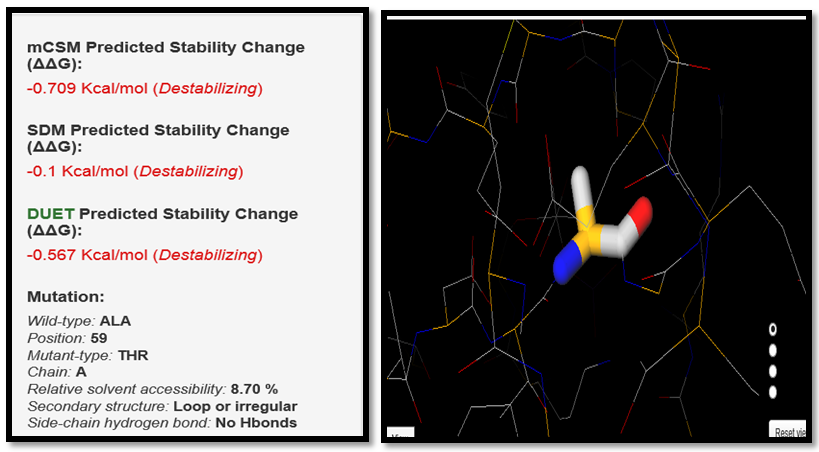 | Figure 17. ALA59THR SNP analysis of DUET server |
3.8. Prediction of Stability Effects upon Mutation in Both, Domain Cores and Domain-Domain Interfaces by ELASPIC
We classified nsSNPs into two groups depending on their structural location: core or interface. To designate a residue as participating in a protein interaction interface, we used ELASPIC server. We obtained core residue positions for 4 snSNPs and interfaces for 9 snSNPs. The result is explained in (Table 5).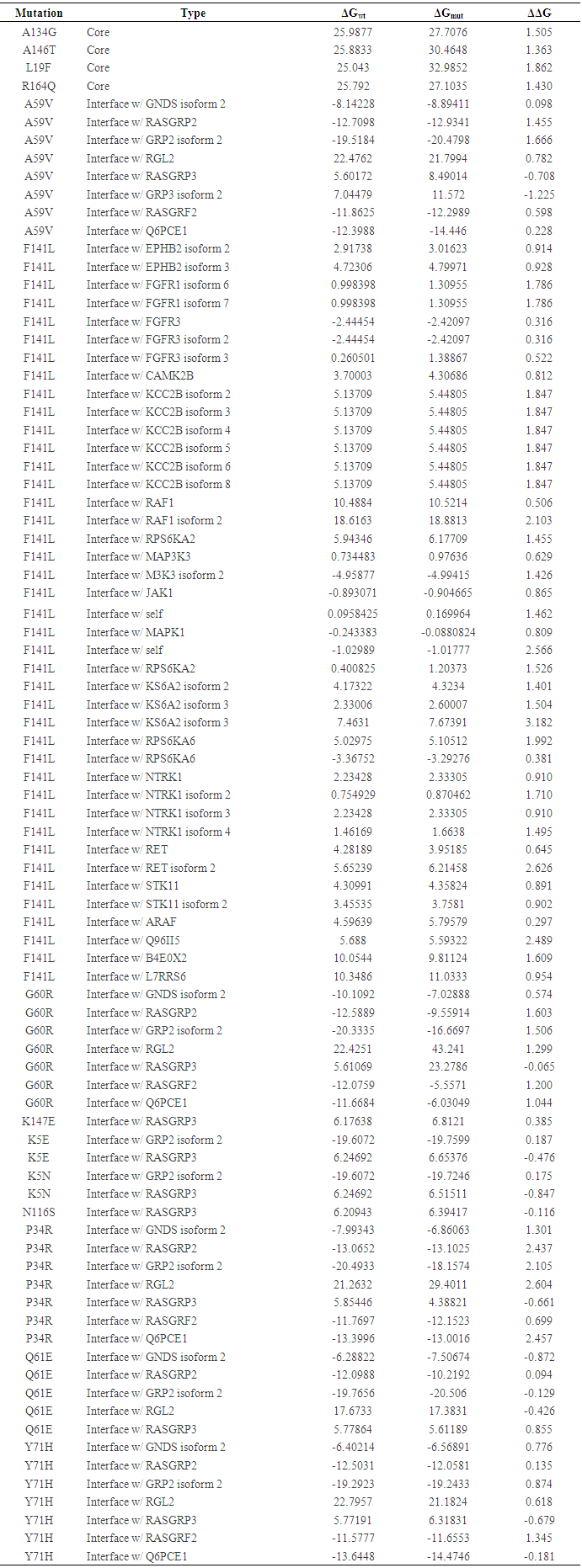 | Table 5. Stability effects nsSNPs in domain cores and domain-domain interfaces by ELASPIC |
3.9. Distributions of nsSNPs in RAS Domain by Mutation3D Server
We Detected 11nsSNPs located in a protein domain structure (G60R, A134G, P34R, Q61E, A146T, N116S, K147E, L19F, F141, Y71H and A59V), 2 nsSNPs were uncovering K5E and Q164R and one SNP hasn’t founded T58I. This 11snSNP were considered higher risk for KRAS protein. The result is shown in (fig 18).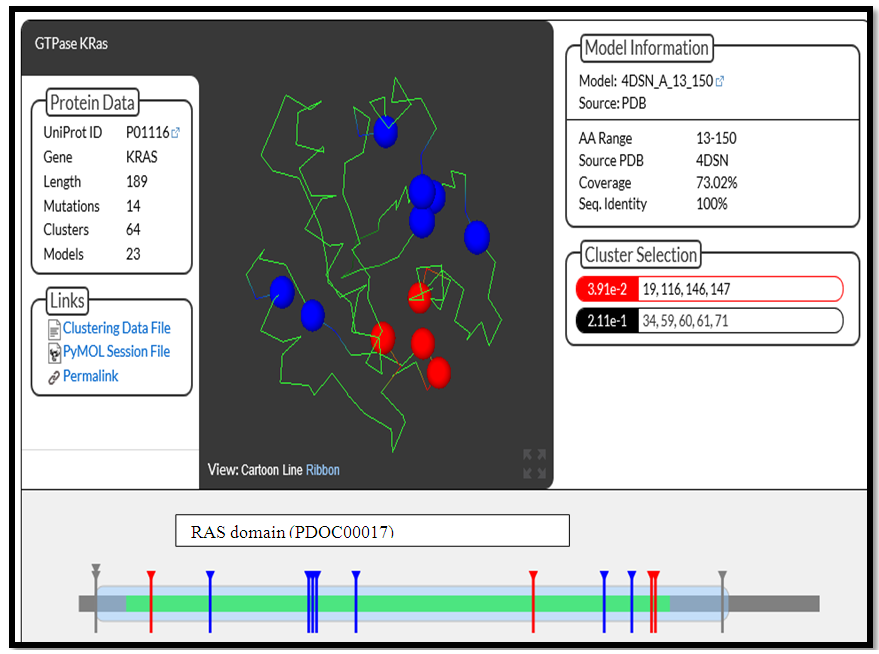 | Figure 18. Structural models for wild type KRAS and 11 high-risk nsSNPs in the RAS domain |
3.10. Conservation Profile of High-Risk Non-Synonymous SNPs
The nsSNPs that are located at highly conserved amino acid positions tend to be more deleterious than nsSNPs that are located at non-conserved sites. To further investigate the potential effects of the 11 high-risk nsSNPs plus 5K and Q164 SNPs, we calculated the degree of evolutionary conservation of all amino acid sites in the KRAS protein using the ConSurf web server. ConSurf analysis revealed that all residues are highly conserved (Conservation Score of 7–9) except Q164 SNP is conserved (Conservation Score of 6) (Table 6).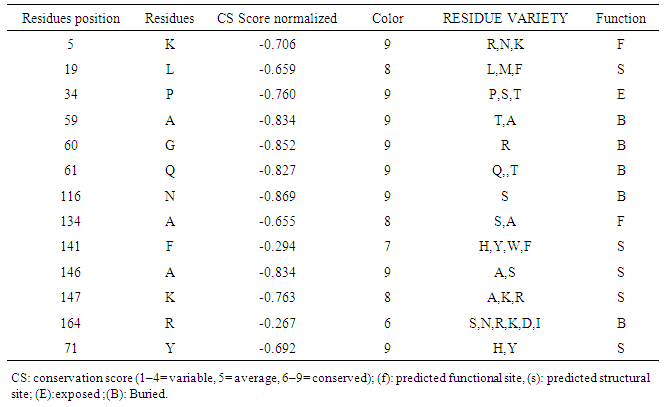 | Table 6. Conservation profile of amino acids in KRAS that coincide in location with high-risk nsSNPs |
3.11. Homology Modeling of New and Wild Amino Acids of Deleterious nsSNPs
Protein 3D structural information is an important feature for predicting the effects of deleterious nsSNPs. Analysis of the protein structure provides information about the environment of the mutation.The H-bonding interactions and clashes of wild type and mutant of 14 nsSNPS were calculated by Chimer program. The native and mutant protein structure for the 14 nsSNPs of human KRAS (PDB ID: 4QL3) are shown in Figures (19-32).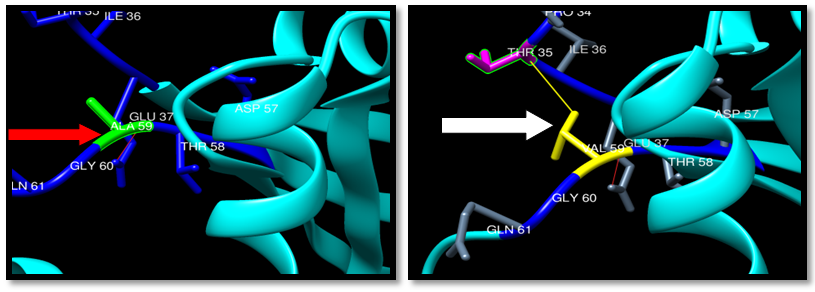 | Figure 19. Rs121913527 (A59V) SNP structure: Single H-bonding interaction in wild type and mutant, one clash between mutant and THR35. Wide type green color, mutant Yellow color, H-bonding (Red line) and Clash (Yellow line) |
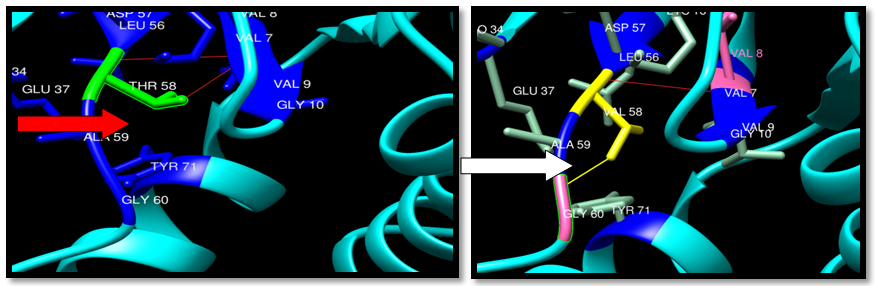 | Figure 20. Rs121913527 (T58V) SNP structure: 2 H-bonding interaction in wild type and one in mutant, one clash between mutant and GLY60. Wide type green color, mutant Yellow color, H-bonding (Red line) and Clash (Yellow line) |
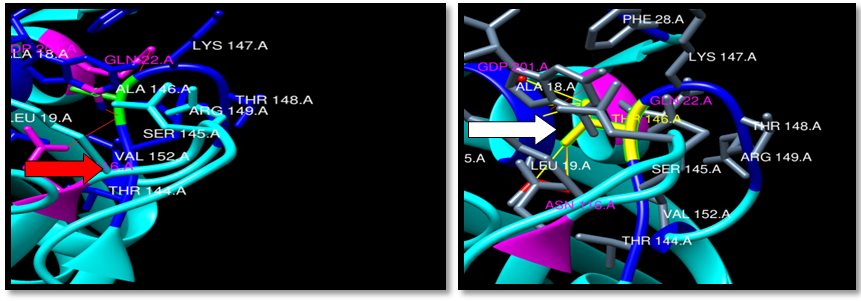 | Figure 21. Rs121913527 (A146T) SNP structure: 3 H-bonding interaction in wild type and 2 in mutant, 5 clash between mutant and CDP 201 Logan, LEU19 and ALA18. wild type green color, mutant Yellow color, H-bonding (Red line) and Clash (Yellow line) |
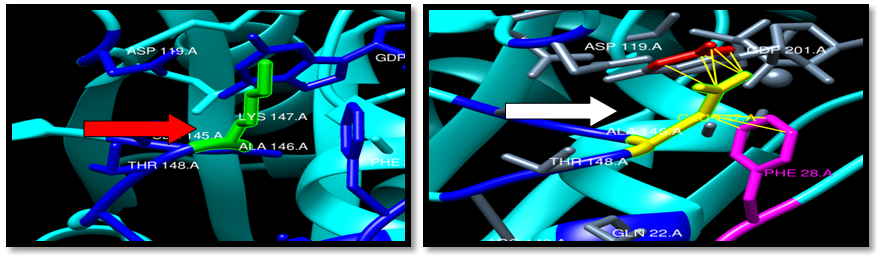 | Figure 22. Rs387907206 (K147E) SNP structure:9 clash between mutant and CDP 201 Logan and PHE28 . wild type green color, mutant Yellow color and Clash (Yellow line) |
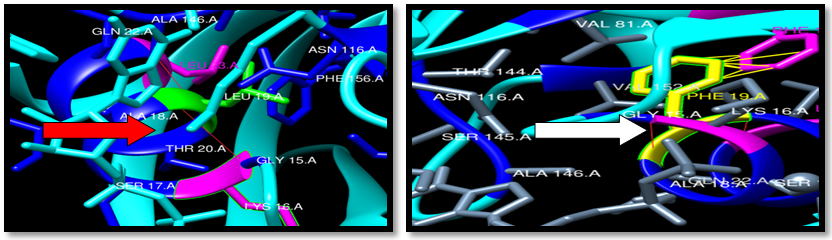 | Figure 23. Rs121913538 (L19F) SNP structure: 2 H-bonding interaction in wild type and mutant. 8 clash between mutant and PHE 56. wild type green color, mutant Yellow color, H-bonding (red line) and Clash (Yellow line) |
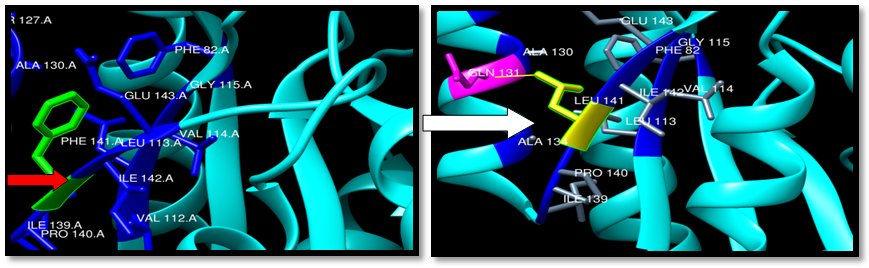 | Figure 24. Rs138669124 (F141L) SNP structure: wild type green color, mutant Yellow color and single clash between mutant and GLN 131 (Yellow line) |
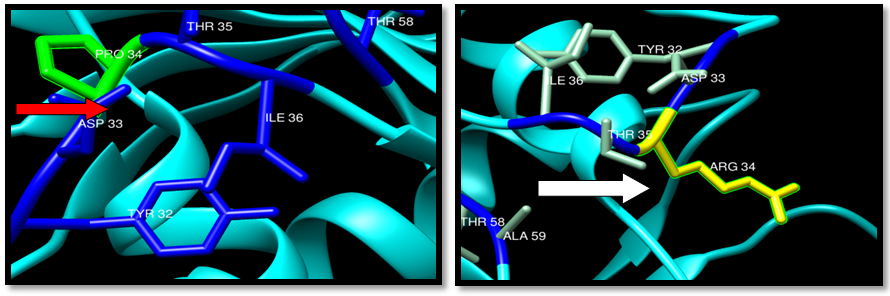 | Figure 25. Rs104894366 (P34R) SNP structure: wild type green color and mutant Yellow color |
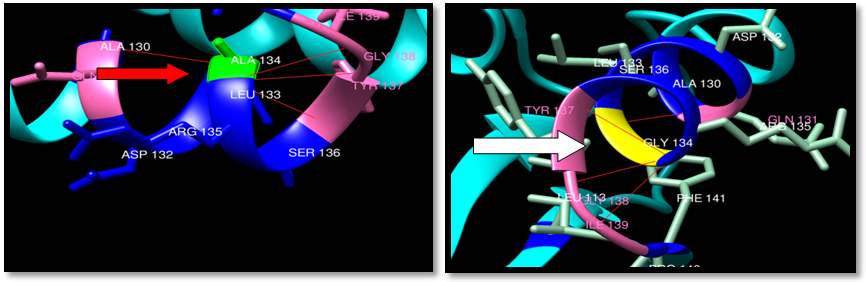 | Figure 26. Rs373500216 (A134G) SNP structure: 4H-bonding interaction in wild type and 4 -bonding interaction in mutant. wild type green color, mutant Yellow color, H-bonding (red line) |
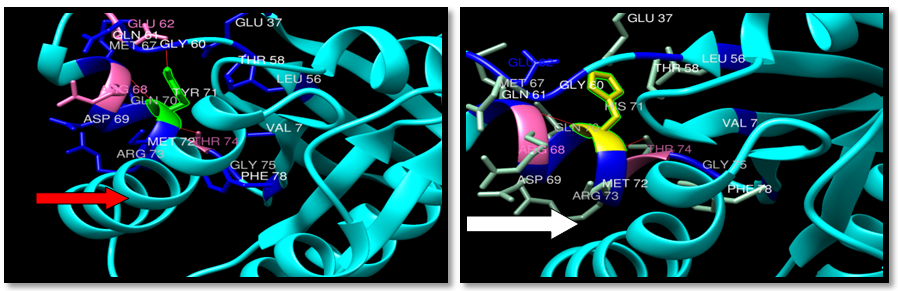 | Figure 27. Rs387907205 (Y71H) SNP structure: 3 H-bonding interaction in wild type and 2 -bonding interaction in mutant. wild type green color, mutant Yellow color, H-bonding (red line) |
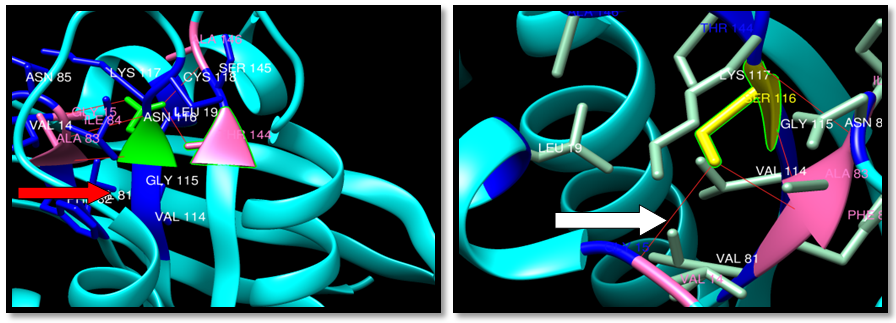 | Figure 28. Rs202247812 (N116S) SNP structure: 5 H-bonding interaction in wild type and 3 -bonding interaction in mutant , wild type green color, mutant Yellow color, H-bonding (red line) |
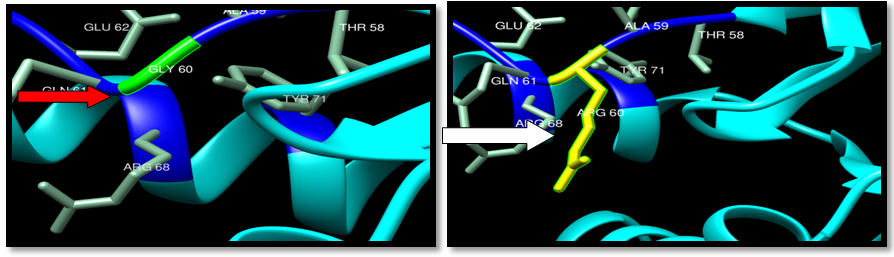 | Figure 29. Rs104894359 (G60R) SNP structure: wild type green color and mutant Yellow color |
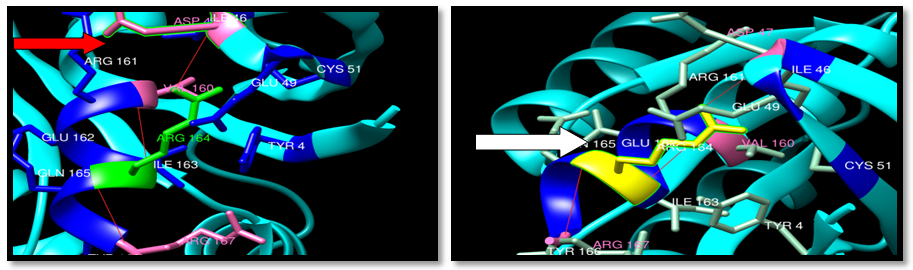 | Figure 30. Rs372793780 (R164Q) SNP structure: 3 H-bonding interaction in wild type and mutant. wild type green color, mutant Yellow color, H-bonding (red line) |
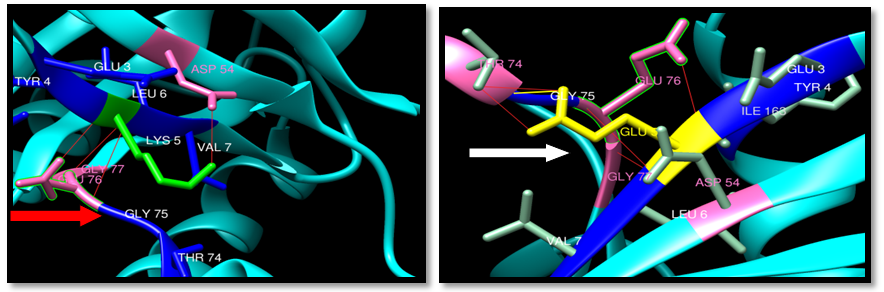 | Figure 31. Rs193929331 (K5E) SNP structure: 4 H-bonding interaction in wild type and 5 H-bonding interaction in mutant and one clash THE74. wild type green color, mutant Yellow color, H-bonding (red line) and clash by yellow color |
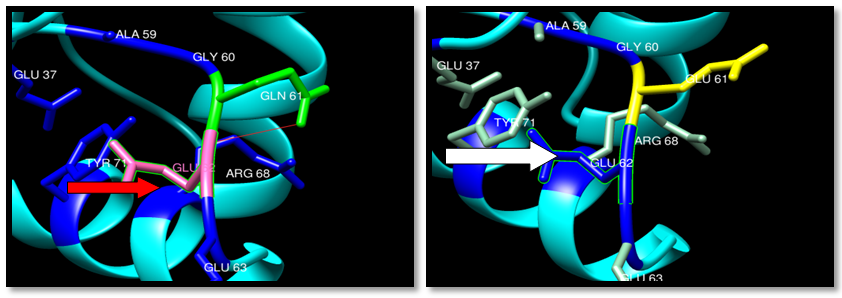 | Figure 32. Rs121913238 (Q61E) SNP structure: Single H-bonding interaction in wild type. wild type green color, mutant Yellow color and H-bonding (red line) |
4. Discussion
Most of the nsSNPs of KRAS are still uncharacterized in terms of their disease causing potential. So, this study has been conducted to explore and extend the knowledge related to the effect of nsSNPs on the stability and function of the KRAS gene using bioinformatics approach. The methods used are based on different aspects and parameters describing the pathogenicity and provide clues on the molecular level about the effect of mutations. It is not easy or even possible to predict the pathogenic effect of SNPs using single method. Therefore, we used multiple methods to compare and rely on the results predicted. In the present study we used nine different in silico prediction algorithms; SIFT, PROVEAN, MutPred, SNP&GO, PhD-SNP, PANTHER, I-Mutant 2.0, MUpo and DUET, To sort tolerant and intolerant SNPs, our result showed 14 out of 26 SNPs were deleterious by SIFT and PROVEN (G60R, A134G, K5N, T58I, P34R, Q61E, A146T, N116S, K147E, L19F, F141, R164Q, K5E and A59V). While 3 nsSNPs were found disease causing by SNP&GO, PhD-SNP and PANTHER (G60R, T58I and P34R) and 13 nsSNPs were found disease causing by SNP&GO, PhD-SNP (G60R, A134G, T58I, P34R, V152G, A146T, N116S, K147E, L19F, F141, R164Q , K5E and A59V). By comparing the output of the 5different insilico tools SIFT, PROVEAN, SNP&GO, PhD-SNP and PANTHER (G60R, A134G, T58I, P34R, A146T, N116S, K147E, L19F, F141, R164Q, K5E and A59V) mutations were found to be functionally significant. The differences in prediction capabilities can be attributed to the fact that every method uses different sets of sequences and alignments. For further analysis we used MutPred tool to determine the degree of tolerance for each amino acid substitution on the basis of physio-chemical properties, the result showed (G60R, A134G, K5N, T58I, P34R, Q61E, A146T, N116S, K147E, L19F, F141, R164Q , K5E and A59V) were harmful and highly harmful. Prediction of Loss of ubiquitination at; G5(P = 0.021 for K5E, Loss of catalytic residue at A59 (P = 0.0406) for A59V, Loss of ubiquitination at G5(P = 0.021) for K5N, Loss of glycosylation at T58 (P = 0.0493) for T58I, Gain of phosphorylation at A146 (P = 0.0405) for A146T, Gain of disorder (P = 0.0374), Loss of stability (P = 0.046) for N116S, while loss of methylation at K147 (P = 0.0184), ubiquitination at K147 (P = 0.0218) and MoRF binding (P = 0.0249) were predicted for K147E and Loss of ubiquitination at G5 (P = 0.021 was predicted at K5E.This implied that some nsSNPs may account for potential structural and functional alterations of KRAS. For further confirmation, we analyzed the effect of these nsSNPs using I-Mutant 2.0 and MUpo for 14 nsSNPs which were detected deleterious by SIFT and PROVEN to determinate the stability of protein upon mutation. Above results demonstrated that protein stability was changed due to SNPs alteration. Additionally, we performed DUET analysis to determinate the effects of nsSNPs on protein stability, and the data that expressed as the change in Gibbs Free Energy (DDG) showed that 11 out of 14 nsSNPS affected the protein stability. The combination of the prediction tools results in 14nsSNPs (G60R, A134G, K5N, T58I, P34R, Q61E, A146T, N116S, K147E, L19F, F141, R164Q, K5E and A59V) indicated as the most damaging mutations in KRAS gene. Furthermore, This 14 SNPs were analyzed, structurally and functionally by 5bioinformatic tools; ELASPIC servers, ConSurf web server, mutation3D, PDB and Chimera. Interface residues play different roles in protein-protein interactions and display both different conservation patterns and different types of interactions depending on their relative position within the interface [23]. The “core” residues are defined as residues which are exposed in the monomeric protein but buried in the protein complex. Core residues are typically hydrophobic with a composition strongly divergent from the composition of the remainder of the protein surface [24]. Core residues supply the bulk of the energy driving association by hydrophobic interactions [25]. The hydrophobic interactions within the complex cause the core region to become tightly packed upon complex association with little room for conformational variability. For these reasons, the core residues are strongly conserved during evolution [26] and mutations in this region are usually more strongly unfavorable when compared to mutations at the periphery of the interface. In cancer, 3D location of mutations at an interface has served as evidence that protein interactions may be important for metastasis site determination. So ELASPIC server applied to classify the nsSNPs according to interface and core residues in KRAS protein, the result showed 4 SNPs were in core (A134G, A146T, L19F and Q164R) and the rest SNPs were in interface. The SNP that occurs in the protein core affects all the protein interactions, and the SNP occurs at an interface only affect the interactions mediated by the interface. Our findings suggest that perturbation to cancer pathways may in fact be mutation-specific and point to the need for analysis methods for tumor-specific network topologies. Mutation3D server was used for nsSNPs location in RAS domain, the result showed 11 SNPs out of 14 located in RAS (G60R, A134G, Y71H, P34R, Q61E, A146T, N116S, K147E, L19F, F141 and A59V). Our ConSurf and Clustal Omega results indicated that 11 nsSNPs were found in the highly conserved region and predicted to have a potential impact on KRAS protein also it predicted that 116N, G60, 59A and 61Q were important structural residue (highly conserved and buried) and that 34P was an important functional residue (highly conserved and exposed). For the 14SNPs 3D structure of protein, H bond and clash was shown between wild type and mutant using the visualized chimera program. 12 nsSNPs out of 14 (Y71H, T58I, P34R, Q61E, A146T, N116S, K147E, L19F, F141, R164Q, K5E and A59V) have differing number of H-bonding between wild and mutant residues indicating that mutation will affect the protein stability, also clash was detected in mutant structure and that indicates the change in the environment of the protein which may affect structure and function. KRAS is G protein and have the Ras domain, it contains a six-stranded beta sheet, 5 alpha helices: and G domain (166 amino acids) which binds guanosine nucleotides, about 20kDa. The G domain contains five G motifs that bind GDP/GTP directly, G1- P-loop binds the beta phosphate of GDP and GTP, G2 - Also called Switch I, consists of Threonine-35 that binds the terminal phosphate (γ-phosphate) of GTP and the divalent Magnesium ion present in the active site, but makes no contacts with GDP, G3- Called the Switch II, has the DXXGQ motif, where the Aspartate-57 is specific for guanine binding rather than adenine and Glutamine-61 is the crucial residue that activates a catalytic water molecule for hydrolysis of GTP to GDP,G4 - LVGNKxDL motif, provides the specific interaction to guanine and G5 - SAK consensus sequence, the alanine-146 is specific for guanine rather than adenine. The two switch motifs, G2 and G3 are the main parts of the protein that move upon activation by GTP. This change in the conformation of the two switch motifs is what mediates the basic functionality as a molecular switch protein. This GTP bound state of Ras is On-state and GDP bound state is the Off-state. Ras also binds a magnesium ion which helps to coordinate nucleotide binding [27]. Out of 14 deleterious SNPs we found 6 nsSNPs are located in these motifs and it has big roles in altering protein functions.Rs104886029 A59V: Mutant residue is smaller than the wild-type residue. This will cause a possible loss of external interactions. In the 3D-structure the mutation is located in a loop in G1 motif. Single H-bonding in wild type and mutant. One clash with THE in 35 in G2 motif, so this SNP can affect the protein functions. The SNP is located in highly conserved residue which made it highly damaging for the protein. The mutant-type residue was buried in the interface with GNDS isoform 2, RASGRP2, GRP2 isoform 2, RGL2, RASGRP3, GRP3 isoform 2, RASGRF2 and Q6PCE1 proteins indicating that the mutation will cause loss of catalytic residues P= 0.0406. Rs121913238 Q61E: the mutant residue is smaller than the wild-type, in addition to that the wild type residue is neutral, and in comparison with the negatively charged mutantant residue this can cause repulsion between the mutated residue and neighboring residues. In the 3D-structure the SNP is located in a loop in G3 motif. Single H-bond in wild type and no H-bonding in mutant that may affect the stability of the protein. The SNP is highly conserved and harmful. This indicates that the mutation is damaging to the protein. Also, the variant is annotated with disease, damaging and increase the stability of protein. The mutant-type residue was buried in the interface with GNDS isoform 2, RASGRP2, GRP2 isoform 2, RGL2 and RASGRP3 proteins. The mutation will cause Gain to disorder (P = 0.0755), Gain of helix (P = 0.132), Loss of sheet (P = 0.1398) Gain of phosphorylation at T58 (P = 0.1442). Loss of glycosylation at T58 (P = 0.1859).Rs104894359 G60R: the mutated residue is smaller than the wild-type, in addition, the wild type residue was neutral, in comparison with the positively charged of mutant this can cause repulsion between the mutated residue and neighboring residues. In the 3D structure the SNP is located in a loop in G3 motif. The wild-type residue is a glycine, the most flexible of all residues. This flexibility might be necessary for the protein's function. Mutation of this glycine can abolish this function. No H-bonding and clash between the wide and mutant residues. The SNP is highly conserved and high harmful indicating that the mutation is damaging to the protein. The variant is annotated with disease, damaging and decrease the stability of protein. The mutant-type residue was buried in the interface with GNDS isoform2,RASGRP2, GRP2 isoform2, RGL2, RASGRP3, RASGRF2 and Q6PCE1. Also mutation will cause Gain of phosphorylation at T58 (P = 0.1068), Loss of glycosylation at T58 (P = 0.123), Gain of solvent accessibility (P = 0.1319), Loss of catalytic residue at A59 (P = 0.1348) and Gain of loop (P = 0.2045).Rs202247812 N116S: The mutant residue is smaller than the wild-type residue. In the 3D-structure the SNP is located in extended beta-strand in G4 motif. 5 H-bonding interaction in wild type and 3 -bonding interaction in mutant. The SNP is highly conserved and high harmful. This indicates that the mutation is damaging to the protein. The variant is annotated with disease, damaging and decrease the stability of protein. The mutant-type residue was a structural site in the interface with RASGRP3 proteins. The mutation will cause Gain of disorder (P = 0.0374), Loss of stability (P = 0.046).Rs121913527 A146T: The mutant residue is bigger than the wild-type residue. The wild-type residue was more hydrophobic than the mutated residue. The hydrophobicity and size difference between wild-type and mutant residue can disrupt the H-bonding interactions with the neighboring residues, and hence the protein framework. In the 3D-structure the SNP is located in a loop in G5 motif.3 H-bonding interaction in wild type and 2 in mutant, 5 clashes between mutant and CDP 201logan, LEU19 and ALA18 The SNP is highly conserved and high harmful. This indicates that the mutation is damaging to the protein. The variant is annotated with disease, damaging and decrease the stability of protein. The mutant-type residue was structural in the core. The mutation will cause Gain of phosphorylation at A146 (P = 0.0405).Rs387907206 K147E: The mutant residue is positively charged and wild-type is negative. In the 3D-structure the SNP is located in a loop in G5 motif. 9 clash between mutant and CDP 201 Logan and PHE28. The SNP is highly conserved and high harmful, which indicates that the mutation is damaging to the protein. The variant is annotated with disease, damaging and decrease the stability of protein. The mutant-type residue was structured in the interface with RASGRP3 protein. The mutation will cause Loss of methylation at K147 (P = 0.0184), Loss of ubiquitination at K147 (P = 0.0218) and Loss of MoRF binding (P = 0.0249).
5. Conclusions
The available data from the NCBI dbSNP database for KRAS gene has been analyzed through several SNP analyzing tools and the predicted deleterious SNPs were evaluated for their deleterious effect on the protein function and stability. 14 SNPs were predicted deleterious; those are (G60R, A134G, K5N, T58I, P34R, Q61E, A146T, N116S, K147E, L19F, F141, R164Q, K5E and A59V). Also, we found these mutations cause a change in protein affinity that can result in malfunction of the protein interaction network. Among them, 6 SNPs were predicted to be located in the motif regions G1, G2, G3, G4 and G5 (G60R, Q61E, A146T, N116S, K147E and A59V) and have the most probability to increase cancer susceptibility.
References
[1] | A. Chakravarti, “To a future of genetic medicine,” Nature, vol. 409, pp.822–823, 2001. |
[2] | Carninci. P, Kasukawa. T, Katayama. S et al., 2005. “The transcriptionall and scape of the mammalian genome,” Science. 309:1559–1563. |
[3] | Liu. J, Gough. J, and Rost. B. 2006. “Distinguishing protein-coding from non-coding RNAs through support vector machines,” PLoS Genetics.2:4-29. |
[4] | Ng .P. C. and Henikoff. S. 2006. “Predicting the effects of amino acid substitutions on protein function,” Annual Review of Genomics and Human Genetics. 7:61–80. |
[5] | Smith .E.P, Boyd. J, Frank. G.R et al., 1994. “Estrogen resistance caused by a mutation in the estrogen-receptor gene in a man,” The New England Journal of medicine. 331. pp. 1056– 1061. |
[6] | Lander E. S. 1996. “The new genomics: global views of biology,” Science.274: 536–539. |
[7] | Thomas. R, R. McConnell, J. Whittacker, P. Kirkpatrick, Bradley. J, and. Sand ford. R. 1999. “Identification of mutations in the repeated part of the autosomal dominant polycystic kidney disease type 1 gene, PKD1, by long-range PCR,” The American Journal of Human Genetics 65:39–49. |
[8] | Doniger S.W, Kim H. S., Swaine D. etal. 2008. “Acatalog of neutral and deleterious polymorphism in yeast. PLoS Genetics.vol. 4, no.8, ArticleIDe1000183. |
[9] | BILLUR H. E, HOFREE. M, CARTER.H .2015. Identifying Mutation Specific Cancer Pathways Using A Structurally Resolved Protein Interaction Network. Pacific Symposium on Biocomputing. 20: 84-95 |
[10] | Jeffrey R. B and Yang Z. 2015. Predicting the Effect of Mutations on Protein Protein Binding Interactions through Structure-Based Interface Profiles. PLOS Computational Biology. DOI:10.1371. 1-25. |
[11] | https://ghr.nlm.nih.gov/gene/KRAS.accssed in february2016. |
[12] | Gregory J. Riely, Mark G. Kris, Daniel Rosenbaum, Jenifer Marks, Allan Li, Dhananjay A. Chitale, Khedoudja Nafa, Elyn R. Riedel, Meier Hsu, William Pao, Vincent A. Miller, and Marc Ladanyi. 2008. Frequency and Distinctive Spectrum of KRAS Mutations in Never Smokers with Lung Adenocarcinoma. Clin Cancer Res.14: 5731–5734. |
[13] | http://www.biotecharticles.com/Bioinformatics-Article/Importance-and-Applications-of-Bioinformatics-in-Molecular-Medicine-214.html. |
[14] | Adzhubei. IA, Schmidt .S, Peshkin. L, Ramensky. VE, Gerasimova. A, et al. 2010. A method and server for predicting damaging missense mutations. Nat Methods 7: 248–249. |
[15] | Ng P. C., Henikoff. S. 2006. Predicting the Effects of Amino Acid Substitutions on Protein Function. Annu Rev Genomics Hum Genet. 7: 61-80. |
[16] | Narayana S. A, Harika. V and Sreenivasulu. K. 2015. In silico Evaluation of Nonsynonymous Single Nucleotide Polymorphisms in the ADIPOQ Gene Associated with Diabetes, Obesity, and Inflammation. Avicenna J Med Biotech 7: 121-127. |
[17] | Calabrese R., Capriotti. E, Fariselli. P, Martelli. P. L, Casadio. R. 2009. Functional Annotations Improve the Predictive Score of Human Disease-related Mutations in Proteins. Human Mutation. 30: 1237-1244. |
[18] | Li B, Krishnan VG, Mort ME, Xin F, Kamati KK, Cooper DN, Mooney SD, Radivojac P. 2009. Automated inference of molecular mechanisms of disease from amino acid substitutions. Bioinformatics. 25: 2744–2750. |
[19] | Capriotti E., P. Fariselli, R. Casadio. 2005. I-Mutant2.0: Predicting Stability Changes upon Mutation from the Protein Sequence or Structure. Nucleic Acids Res.33: 306-310. |
[20] | Douglas E.V. Pires, David B. Ascher and Tom L. Blundell. 2014. DUET: a server for predicting effects of mutations on protein stability using an integrated computational approach. Nucleic Acids Research. 42: 314–319. |
[21] | Ashkenazy H, Erez E, Martz E, Pupko T, Ben-Tal N. 2010. ConSurf 2010: calculating evolutionary conservation in sequence and structure of proteins and nucleic acids. Nucleic Acids Res. 38: 529–33. |
[22] | Sofia B. M, Mohamed M. H. 2016. Insilico Validation of Babesia Bovis Merozoite Surface Antigen-1, Merozoite Surface Antigen-2b and Merozoite Surface Antigen-2c Proteins for Vaccine and Drug Development. International Journal of Bioinformatics and Biomedical Engineering. 2: 30-39. |
[23] | Levy ED. 2010. Asimple definition of structural regions in proteins and it s use in analyzing interface evolution. Journal of molecular biology. 403: 660–70.doi:10.1016/j.jmb.09.028PMID:20868694. |
[24] | Talavera D, Robertson DL, Lovell SC. 2011. Characterization of protein-protein interaction interfaces from a single species. PloSone. 6: e21053. doi:10.1371 /journal.pone 0021053 PMID:21738603. |
[25] | Andreani J, Faure G, Guerois R. Versatility and in variance in the evolution of homologous heteromeric interfaces.2012. PLoS computational biology. 8: e1002677. doi:10.1371/journal.pcbi.1002677 PMID: 22952442. |
[26] | Guharoy. M, Chakrabarti. P. 2005. Conservation and relative importance of residues a cros protein-protein interfaces. Proceedings of the National Academy of Sciences of the United States of America. 102: 15447–52.PMID:16221766. |
[27] | Omim- Neuroblastoma Ras Viral Oncogene Homolog; Nras. |