Yasin Bakış1, Hasan H. Otu2, 3, O. Uğur Sezerman4
1Department of Biology, Abant İzzet Baysal University, Bolu, 14280, Turkey
2Department of Bioengineering, Istanbul Bilgi University, 34060, Eyup, Istanbul, Turkey
3BIDMC Genoics Center, Harward Medical School, Boston, 02215, USA
4Biological Sciences and Bioengineering, Sabancı University, İstanbul, 34956, Turkey
Correspondence to: Yasin Bakış, Department of Biology, Abant İzzet Baysal University, Bolu, 14280, Turkey.
Email: |  |
Copyright © 2012 Scientific & Academic Publishing. All Rights Reserved.
Abstract
Phylogenetic analysis relies on alignment of related sequences from different species to obtain the distances between these species. The quality of the alignment and the distance measure would depend on the alignment parameters that are used. In this work, we propose to use Relative Complexity Measure (RCM) method to find the distances between the sequences which is a parameter independent measure. We used DNA sequences from Candida species and phylogenetic trees were obtained using un-weighted pair-group with arithmetic mean method. We used three reduced alphabets for the DNA sequences which were clustered by taking into account different physico-chemical properties of DNA. RCM gives as good results as the distance determination method and among the physico-chemical properties, Keto/Amino grouping is found to give the most accurate tree which is topologically closest to the desired phylogeny.
Keywords:
Phylogenetics, DNA, Reduced Alphabets, Relative Complexity Measure, Candida
Cite this paper: Yasin Bakış, Hasan H. Otu, O. Uğur Sezerman, Inferring Phylogenies from Physico-Chemical Properties of DNA, American Journal of Bioinformatics Research, Vol. 2 No. 1, 2012, pp. 1-6. doi: 10.5923/j.bioinformatics.20120201.01.
1. Introduction
DNA sequencing methodology has become one of the most widely used techniques in molecular biology and DNA sequences submitted to databases has increased exponentially each year, resulting in an enormous increase in the size and amount of data generated[1]. Phylogenetic analysis of molecular sequences is indispensible in the areas of Systematic and Evolutionary Biology and DNA sequences are important resources for phylogenetic analysis. It is becoming a very common procedure to analyze relationships within a taxonomic group by isolating sequenced DNA and constructing the phylogeny. Methods for constructing phylogenies have been developed by the discipline of Phylogenetics or Cladistics[2].Most of the methods are based on multiple alignment of DNA sequences and calculation of distances (proportional to insertions, deletions and mutations) between these sequences. Once the distance matrix is obtained, one can choose the appropriate clustering method to obtain the phylogenetic tree. Unfortunately quality of the multiple alignment obtained usually vary with the alignment parameters (gap opening and extension penalties) and often an expert manipulation step is required to obtain a reasonable alignment and thus areasonable distance matrix.In this work, we propose to use Relative complexity Measure method to find the distances between the sequences without needing to align them. We also clustered the DNA sequences based on their physico-chemical properties to see which of the clustering methods, if any, yielded to a reasonable phylogenetic tree. The innovative concepts brought to this domain within this work are:
1.1. Physico-chemical Properties of DNA
DNA is a molecule that can store genetic information, have this information expressed, and have the information precisely duplicated. Information, in its most restricted technical meaning, is an ordered sequence of symbols, whether that sequence is chain of bases or computer 0’s and 1’s. The DNA is a double-stranded anti-parallel deoxyribose nucleic acid sequences in a double-helix and composed of four different types of bases: Adenine (A), Thymine (T), Guanine (G) and Cytosine (C) (A wider description can be found in[3].) Bases differ in each other by their physico-chemical properties. In this way, they represent different symbols in the sequence.DNA has physical and chemical properties which depend on the distribution of nucleotides A, T, G, C. Nucleotides have different physico-chemical properties according to their molecular structure. The effect of difference in physico-chemical properties between amino acids plays a significant role in determining the rate of codon substitution [4]. Nucleotides are grouped according to physico-chemical properties. A frequently used grouping property is having either a Purine or Pyrimidine base. Purine bases are A and G, whereas Pyrimidine bases are C and T (Figure 1). Another property is Hydrogen bonding between two complementary bases. Triple Hydrogen bonds formed between G and C while A and T form two bonds. Third property, bases A and C are 6-amino bases, whereas G and T are 6-keto bases[5].
1.2. Relative Complexity Measure Method
Relative Complexity Measure is a recently introduced phylogeny construction method that does not require an alignment procedure, and does not consider an evolutionary assumption – which are said to be the odds of current phylogeny programs[6]. In the recent works[8,9], RCM has found to be successful in constructing phylogenies of several taxonomic groups based on molecular sequences from different parts of genome. RCM computes organism relatedness based on the overall complexity of the sequences. However, the measure of complexity critically depends on the alphabet used to describe the sequence[9]. By using different physico-chemical properties of DNA we can group nucleotides and represent them as reduced alphabets. In this work, we propose to compare effects of physico-chemical features of DNA on phylogeny construction by RCM method using three reduced alphabets.
2. Materials and Method
2.1. Molecular Sequences
The most common clinically important Candida species were chosen for the phylogenetic analyse of sequences. 396-bp region of the mitochondrial cytochrome b gene has been obtained from[10]. Selected Candida species are C. albicans, C. glabrata, C. parapsilosis, C. tropicalis, C. krusei, C. lusitaniae, C. dubliniensis and an outgroup Filobasidiella neoformans were used as operational taxonomic units. Table 1 lists all sequences used in this study with their GenBank accession numbers. Candida data is also used in the study of utilization of RCM method in[7]. Information on sequencing, fungal strains, strain origins and some other information of used sequences can be reached from Yokoyama et al[10].
2.2. Reduced Alphabets
Three reduced alphabets have been generated according to the physico-chemical properties of DNA (Table 2). Sequence compositional complexity profiles are here decomposed into partial profiles using the branching property of the Shannon entropy[11]. Thus, a regular DNA sequence composed of ACGT’s would be converted into a sequence of 0’s and 1’s.Table 2 lists the reduced alphabets used in this study and grouping of the bases. A sample outputs for a simple sequence were tabulated in Table 3 with respect to corresponding reducing alphabet.
2.3. Phylogenetic Analysis
Phylogenetic analyses of molecular sequences were performed by using Relative Complexity Measure method. Method was explained in Otu and Sayood in detail[6]. Small modifications on RCM allowed us to analyze more than one sequence at a time. RCM can search for occurrence of a subsequence within the complementary string, but in current situation, it will create history for more than one sequence synchronous. 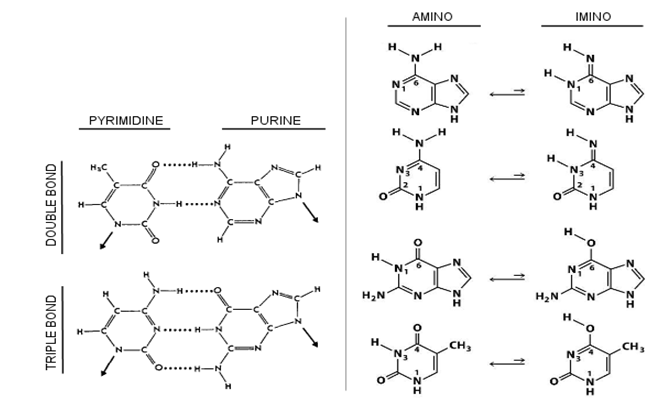 | Figure 1. Physico-chemical properties of nucleotides. Bonding and Purine/Pyrimidine structure are at the left side. Keto/Amino groups are at the right side of the Figure |
Table 1. Fungal strains and accession numbers of cytochrome b genes sequenced in this study |
| Species | Accession no. | Candida albicans | AB044909 | Candida albicans | AB044910 | Candida albicans | AB044911 | Candida albicans | AB044918 | Candida albicans | AB044919 | Candida dubliniensis | AB044912 | Candida dubliniensis | AB044913 | Candida dubliniensis | AB044914 | Candida glabrata | AB044920 | Candida glabrata | AB044921 | Candida glabrata | AB044922 | Candida glabrata | AB044915 | Candida krusei | AB044924 | Candida krusei | AB044923 | Candida krusei | AB044925 | Candida lusitaniae | AB044926 | Candida lusitaniae | AB044927 | Candida parapsilosis | AB044928 | Candida parapsilosis | AB044929 | Candida parapsilosis | AB044916 | Candida tropicalis | AB044930 | Candida tropicalis | AB044917 | Candida tropicalis | AB044931 | Candida tropicalis | AB044932 | Candida tropicalis | AB044933 | Filobasidiella neoformans | AB040656 |
|
|
Table 2. Reduced alphabets, abbreviation and groupings |
| Grouping factor | Code | Groups | Puine-Pyrimidine | A | {A, G} {T, C} | Complementary pairing | 1 | {A, T} {G, C} | Keto-Amino bases | X | {A, C} {G, T} |
|
|
Table 3. A sample sequence and corresponding outputs |
| Alphabet | Output | Original sequence | AGTGGTCACCTGATCAGTGGTCACCTGATC | Purine-Pyrimidine | 001001101110011001001101110011 | Complementary pairing | 010110101101001010110101101001 | Tautomeric form | 011111000011010011111000011010 |
|
|
We have used Unweighted Pair-Group Method with Arithmetic mean (UPGMA) tree construction method in NEIGHBOR routine of Phylogeny Inference Package (PHYLIP) version 3.69[12]. Resulting phylogenies were drawn by using FigTree version 1.3.1. Distances among produced phylogenies were calculated by TreeDist routine of PHYLIP with Symmetric distance option. Symmetric distance is used to calculate topology distances among phylogenies, not the branch length distances.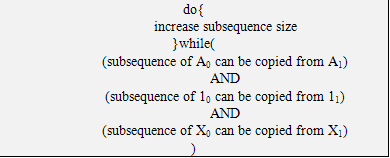 | Figure 2. Pseudo-code that describes how to combine A1X sequence from reduced alphabets |
Phylogeny names are abbreviated as ORG = original sequence, A = Purine/Pyrimidine grouping, 1 = double/triple bond grouping, X = Keto/Amin grouping. Other abbreviations are combinations of them with ‘AND’ or ‘OR’. As an example, A1X distance is generated using all sequences and while computing distance if any of A or 1 or X stops reading from history, all stop and increase distance by 1 (Figure 2).For 1X', sequences 1 and X were used and RCM distance score calculated by copying from history until all sequences stop copying.
3. Results and Discussion
Table 4. Matrix of Topology Distances between Tree Pairs 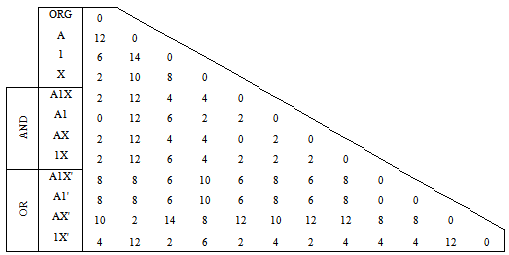 |
| |
|
The three different reduced alphabets had been extracted by using physico-chemical properties of DNA sequences from Candida species. Phylogenetic distance matrices for each set of reduced alphabet sequence were calculated by relative complexity measure method. Since UPGMA was accepted as the best method for constructing phylogenetic trees based on cytochrome b sequences of fungi[13], phylogenetic trees were calculated by UPGMA technique. Graphical representations of the resulting phylogenies were plotted by FigTree in Figure 3. A distance matrix has been created by topology distance scores of each phylogeny pairs as indicated in Table 4. Figure 4 is the graphical representation of the topology distance data matrix.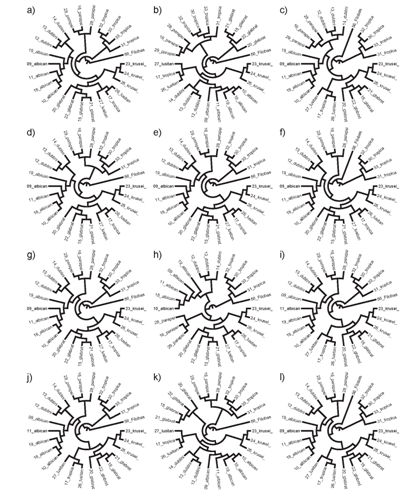 | Figure 3. Graphical representation of different sequences based on three reduced alphabets. (a) Original phylogeny, (b) phylogeny [A] based on purine/pyrimidine grouping, (c) phylogeny [1] based on triple/double bonding grouping, (d) phylogeny [X] based on keto/amino structure grouping, (e) phylogeny based on A and 1 and X, (f) phylogeny based on A and 1, (g) phylogeny based on A and X, (h) phylogeny based on 1 and X, (i) phylogeny based on A or 1 or X, (j) phylogeny based on A or 1, (k) phylogeny based on A or X, (l) phylogeny based on 1 or X.Phylogenies containing X also have acceptable topologies since they are all showing 2 TreeDist distance from the original phylogeny. Sequence X has completely different information than the 1 and A. The information content of the sequence is arising from transversion of bases. However, phylogenies produced by involvement of X are much more similar to original phylogeny than the two other sequences |
All the phylogenies are successfully clustered similar groups under same cluster except C. tropicalis AB044917. However, it is marked in Bastola et al[7] as there might be a problem with this sequence. According to the topology distances matrix and its graphical representation, A1 is found to have the same topology as original topology, X, 1X, AX and A1X follow next in order. Combination of sequences A and 1 is expected to be the same as original nucleotide sequence and it is the closest phylogeny to ORG as in Figure 4 and Table 4. As a general opinion, combinations of sequences have distant topology, especially in the use of or statement. As a group combination - where or statement used - gives worst results, A and 1 are located separately among them. When A and 1 are separately used, they contain half of the information content of original sequence. However, RCM does not deal with transition/transversion ratio, instead it uses relative complexity. 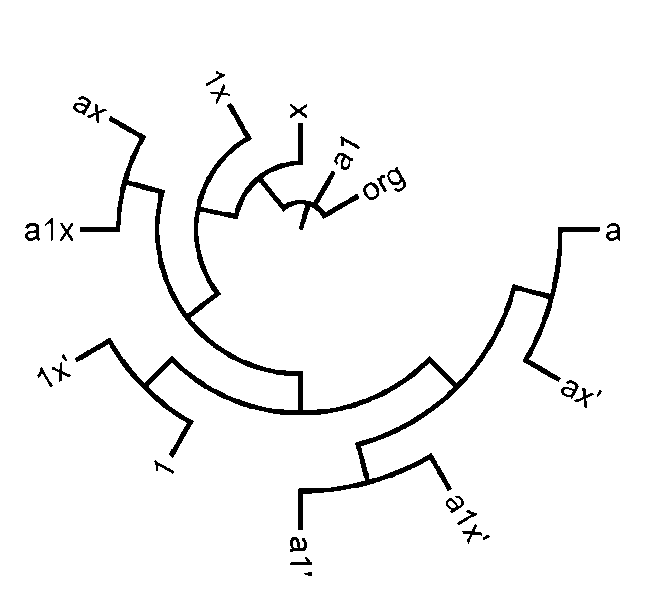 | Figure 4. Supertree of produced phylogenies based on topology distance |
4. Conclusions
Utilization of reduced alphabets based on physico-chemical properties of DNA or amino acid sequences have been assessed by bioinformaticians and molecular biologists for analysing binding domains[14], promoter prediction[15, 16], gene finding[17], Nonsynonymous Mutations in the Protein-Coding Genes[18] and many others. However, effect of physico-chemical properties on phylogeny construction was not very well covered within these studies. In this study, we are able to understand that, reduced alphabets of DNA sequences by physico-chemical properties are to be useful in phylogeny construction. Our results have shown that combinations of reduced alphabet sequences by and give closer results to true phylogeny. Especially, combination of A (purine/pyrimidine grouping) and 1 (double/triple bonding) has given closest solution together. On the other hand, X (keto/amin grouping) gives next best solution by only itself, and fails when combined with another sequence. Nevertheless, some results were unexpected, since the changing structure of DNA. In future work, we will consider different types of sequences from various sizes and different locations from genome while selecting molecular source. Utilization of reduced alphabets of DNA by other types of phylogeny analysis methods and use of reduced alphabets of protein sequences are also among the planned future works.
References
[1] | Griffin, H. G. and A. M. Griffin, 1994, Computer Analysis of Sequence Data, Methods in Molecular Biology, 24, 1-8 |
[2] | Hennig, W., 1966, Phylogenetic Systematics, University of Illinois Press, Urbana |
[3] | Watson, J. D. and F. H. Crick, 1953, Molecular structure of nucleic acids; a structure for deoxyribose nucleic acid, Nature, 171, 737-8. |
[4] | Xia, X., 2000, Data analysis in molecular biology and evolution, Kluwer Academic, Boston |
[5] | Lowdin, P. O., 1985, Advances in Quantum-Chemistry - Preface, Advances in Quantum Chemistry, 17, R9-R10 |
[6] | Out, H. H. and K. Sayood, 2003, A new sequence distance measure for phylogenetic tree construction, Bioinformatics, 19, 2122-2130 |
[7] | Bastola, D. R., H. H. Otu, S. E. Doukas, K. Sayood, S. H. Hinrichs, and P. C. Iwen, 2004, Utilization of the relative complexity measure to construct a phylogenetic tree for fungi, Mycological Research, 108, 117-125 |
[8] | Bakış, Y., H. Otu, U. Sezerman, N. Taşçı, and N. Bilgin, 2008, Constructing Robust Phylogenetic Trees for Galanthus by Using Relative Complexity Measure Method and A New Bootstrap Model, in Health Informatics and Bioinformatics '08 Conference, İstanbul |
[9] | Bernaola-Galvan, P., J. L. Oliver, and R. Roman-Roldan, 1999, Decomposition of DNA sequence complexity, Physical Review Letters, 83, 3336-3339 |
[10] | Yokoyama, K., S. K. Biswas, M. Miyaji, and K. Nishimura, 2000, Identification and phylogenetic relationship of the most common pathogenic Candida species inferred from mitochondrial cytochrome b gene sequences, J Clin Microbiol, 38, 4503-4510 |
[11] | Cover, T. M. and J. A. Thomas, 1991, Elements of Information Theory, Wiley, New York |
[12] | Felsenstein, J., 2009, PHYLIP Home Page [Online]. Available: http://evolution.genetics.washington.edu/phylip.html |
[13] | Wang, L., K. Yokoyama, M. Miyaji, and K. Nishimura, 1998, The identification and phylogenetic relationship of pathogenic species of Aspergillus based on the mitochondrial cytochrome b gene, Medical Mycology, 36, 153-164 |
[14] | Huang H. L., I. C. Lin, Y. F. Liou, C. T. Tsai, K. T. Hsu, W. L. Huang, S. J. Ho, S. Y. Ho, 2011, Predicting and analyzing DNA-binding domains using a systematic approach to identifying a set of informative physicochemical and biochemical properties, BMC Bioinformatics, 12(Suppl 1):S47 |
[15] | Uren, P., R. M. Cameron-Jones and A. Sale, 2006, Promoter Prediction Using Physico-Chemical Properties of DNA, Lecture Notes in Computer Science, 4216 (2006), 21-31 |
[16] | Abeel, T., Y Saeys, P. Rouzé and Y. Peer, 2008, ProSOM: core promoter prediction based on unsupervised clustering of DNA physical profiles, Bioinformatics, 24 (13), i24-i31 |
[17] | Singhal, P., B. Jayaram, S. B. Dixit, and D. L. Beveridgey, 2008, Prokaryotic Gene Finding Based on Physicochemical Characteristics of Codons Calculated from Molecular Dynamics Simulations, Biophysical Journal, 94, 4173–4183 |
[18] | Moilanen, J. S. and Kari Majamaa, 2003, Phylogenetic Network and Physicochemical Properties of Nonsynonymous Mutations in the Protein-Coding Genes of Human Mitochondrial DNA, Molecular Biology and Evolution, 20(8), 1195–1210 |