Vladimir Sadovoy1, 2, Arcady Silantyev3, Tatiana Shchedrina1, Elena Savelieva2
1North-Caucasian Federal University, Pyatigorsk, Russia
2Stavropol Institute of Cooperatives (branch) BUCEP, Stavropol, Russia
3Povolzhskiy State University of Telecommunications and Informatics, Stavropol, Russia
Correspondence to: Vladimir Sadovoy, North-Caucasian Federal University, Pyatigorsk, Russia.
Email: |  |
Copyright © 2014 Scientific & Academic Publishing. All Rights Reserved.
Abstract
On the basis of the analysis of the chemical and amino acid compositions of raw materials with the using of cluster analysis and neural networks, called Kohonen maps, developed a method of assessing prescription of regulatory requirements. The developed technique was tested in the analysis of prescription of meat products.
Keywords:
Food products, Regulatory requirements, Cluster analysis, Meat products, Chemical composition, Amino acid composition
Cite this paper: Vladimir Sadovoy, Arcady Silantyev, Tatiana Shchedrina, Elena Savelieva, The Assessment of Food Composition Conformity with the Recipes’ Requirements, Advances in Life Sciences, Vol. 4 No. 2, 2014, pp. 82-87. doi: 10.5923/j.als.20140402.08.
1. Introduction
In the recent years, food-processing companies have been producing foods that are often below the required quality standards. Adding various food additives as a way to boost revenue has become commonplace. The vast majority of formulations substitutelow-grade ingredients for higher quality materials, using additives of unverified composition from various manufacturers. Practice shows that such food products are usually of lower quality compared to those produced in compliance with the regulatory requirements. Quality issues are especially serious when it comes to such products as cooked sausage, semi-smoked sausage, and cooked-and-smoked sausage. Thus follows the importance of protecting the interests of both manufacturers and consumers in an equal fashion, balancing out the accepted risks of food consumption and the possibilities connected to the scientific, technological and economical level of development. It is necessary to provide also that the quality of the products delivered to the store counters meets the guaranteed standards.The current quality standards specify the requirements based on the microbiological and organoleptic (appearance, texture, appearance on the cut, smell, taste, color, shape, size) properties, as well as some physiochemical parameters (protein, fat and moisture content, and other indicators). These indicators, however, while important, do not allow the accurate identification of the product. Developing a method for quality assessment of food products determining whether they meet the regulatory requirements will protect consumers from substandard products.Research objective is working outa method of quality assessment for food products, to validate this method by analyzing cooked sausage products to confirm their compliance with regulatory requirements.
2. Materials and Methods
All statistical and mathematical modelling is implemented Statistic v. 10, Statistical Neural Networks v. 4, 10 and algorithmic language Pascal. The preliminary phase carried out an analysis to determine the chemical and amino acid composition of the raw materials used in sausage production: beef and lean pork (all grades), beef liver, fat, pork belly, pork skin, isolated and concentrated soy protein preparations, sodium case in ate, animal protein Cat-Pro 95, soy flour, chicken eggs, and wheat flour.Based on the amount of the analyzed components, the mixture model was created, and factor variability intervals were pre-set. In the planning matrix, the dimensionless characteristics were substituted for natural values. The planning metrics data was run through a program developed in Pascal to create a component array where the factor scores were calculated assuming that the sum of the raw material components in the formulation equaled 100%. The component array included 17,026 variations of mixture compositions. For each variation, solids, protein and fat (including calculations on a dry basis) content was calculated, as well as amino acid composition (based on essential amino acids and oxyproline), sum of essential amino acids (EAA), and tryptophan: oxyproline ratio. Oxyproline monitoring is necessary to determine the presence of connective tissue in meat products and gives the opportunity to determine whether the formulation meets the standard requirements regulating the quantities of graded lean meats and food additives in the product.Protein and fat content indicators (in % on a dry basis) determine the possibility of using various carbohydrate- protein supplements and help establish whether the formulation meets the regulatory requirements for quantitative graded pork meat content.Amino acid composition indicates the formulation ratio for raw materials containing protein fraction, as each of the ingredients used in the formulation has a specific set of amino acids.
3. Results and Discussions
3.1. Development of Composition’s Model
Until recently a decision on optimization options were passed without special studies, only on the basis of the experience and common sense. However, the more complex became a system of requirements to the final product, the less valid approximate solutions and the greater significance of special methods to evaluate the advantages and disadvantages of each option.To validate the effectiveness of the developed method, a sample was manufactured using the formulation for top grade cooked sausage (kolbasavarenaya) “Stolichnaya”. Allowing a margin for error in experimental studies (accepted margin for process design compilations being 5%), chemical and amino acid compositions were calculated for the given product. On entrance variables of a matrix of planning and investigated indicators the architecture of a neural network is developed. The laboratory analysis results and calculated indicators were recorded in the database consisting of component array and functional indicators which calculated by means of a neural network that measure the given meat product’s compliance with the regulatory requirements (table 1, 2).Table 1. Array of prescription formulations1 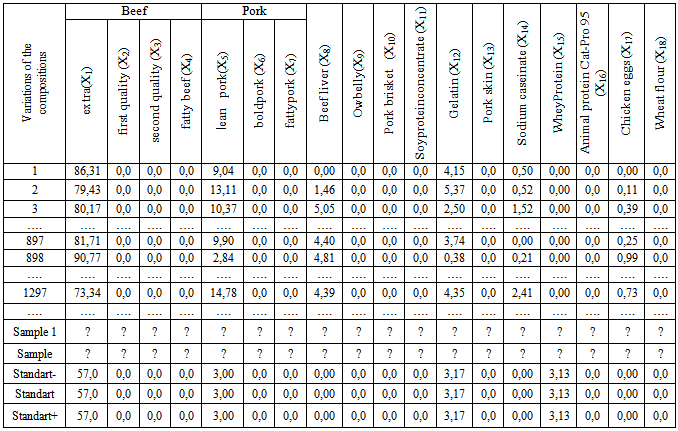 |
| |
|
Table 2. Chemical and amino acid content of prescription formulations2 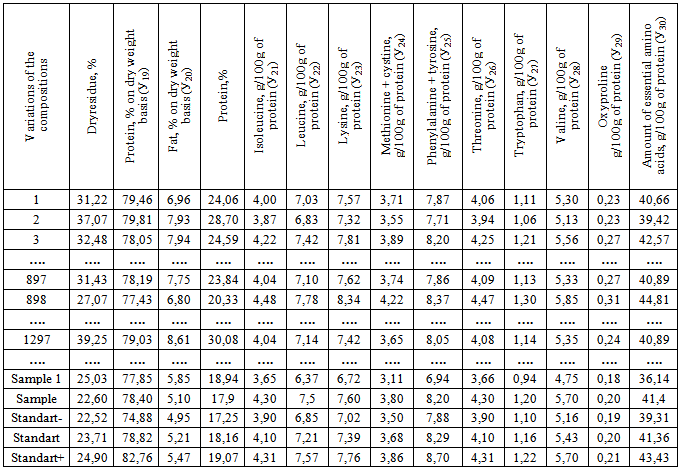 |
| |
|
In the array prescription compositions (table 1) dimensionless parameters were replaced with natural values. Using developed on the algorithmic language Pascal program based on the data of the planning matrix created factor is array of factors. Array included 17026 variants of composite structures. For each option was calculated (table 2) the content of dry matter, protein and fat (including in terms of dry substance), amino acids (essential amino acid and oxyproline), the amount of essential amino acids (NAC). Control of oxyproline is necessary to evaluate the availability of meat products connective tissue and provides the opportunity to determine the compliance of the formulation of a regulatory quantities of long trimmed meatand food additives. Indicators in the content of protein and fat (in % on a dry basis) characterize the possibility of using various protein-carbohydrate supplements and compliance recipe standards for the quantitative content of long trimmed pork.Amino acid composition specifies the ratio of the recipe of raw materials containing protein fraction, because each using ingredient has a characteristic set of amino acids.
3.2. Assessment of the Conformity of the Composition
The array data (table 2) were clusted using Kohonen neural networks [1, 2] to determine the recipes’ compliance of manufactured sample with the requirements of developed technical documentation (Fig. 1, 2).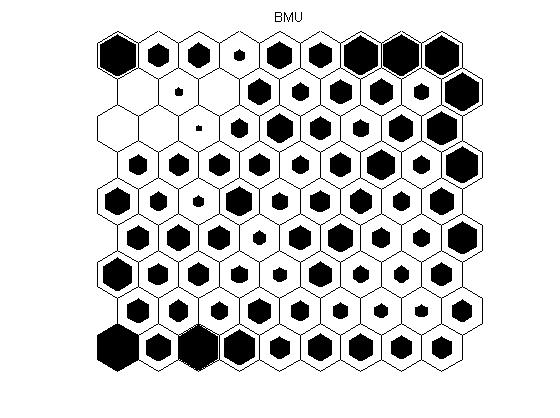 | Figure 1. Parameters of the neural network maps |
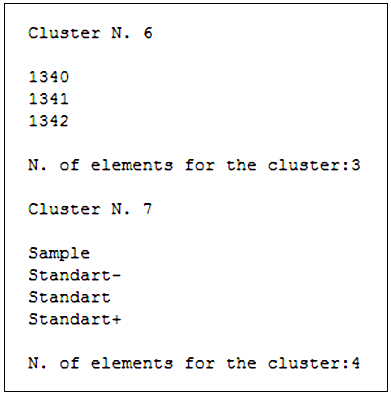 | Figure 2. Fragment of listing clustering results |
Aself-organizing map (SOM) orself-organizing feature map is a type of artificial neural network that is trained using unsupervised learning to produce a low-dimensional (typically two-dimensional), discretized representation of the input space of the training samples, called amap. Self-organizing maps are different from other artificial neural networks in the sense that they use a neighborhood function to preserve the topological properties of the input space. This makes SOMs useful for visualizing low - dimensional views of high-dimensional data, akin to multidimensional scaling. The model was first described as an artificial neural network by the Finnish professor Teuvo Kohonen, and is sometimes called aKohonen mapornetwork. The principal difference of neural networks, called Kohonen maps is uncontrolled study’s using. In this case training set consists only of the values of the input variables [3, 4].We can say that such network learns to understand the structure of the data. These networks are capable of solving the classification problem without any teacher i.e. clustering.Parameters of the neural network maps (fig.1) indicate uneven filling the cluster zones (neurons), characterized by the intensity of staining cells (some of the neurons do not include variants). At the same time, analysis of the listing clustering showed that the cluster №7 (Cluster №7, fig. 2) are full of examples, Sample, standart-, Standart, Standart+. This suggests that in the analyzed dataset not found options with similar composition of ingredients for the recipe sausage, and composition of the sample fully complies with the requirements of technical documentation (excluding the organoleptic and microbiological parameters) [5, 6].For the final evaluation of the effectiveness of the developed technique was made in the laboratory prototype of the cooked sausage recipe “Stolichnaya” (sample), another sample was purchased in store (sample 1). In both samples to determine the chemical and amino acid compositions.Laboratory results were added to the database, consisting of the factor of the array (Х1 –Х18) and functional indicators (У19 – У30) characterizing the compliance of a compounding this meat products regulatory requirements.The array data were subjected to cluster analysisby means of Kohonen neural networks. The learning set used in this method consists only of input variables values. As a result, a cluster of 61 composition variations was found. Figure 3 shows the analyzed cluster, with the computational data interval for the “Stolichnaya” sausage formulation (allowing a margin for error) displayed between the “standart” indicators. The results of chemical and amino acid composition analysis of the test sample are marked “sample” [7, 8].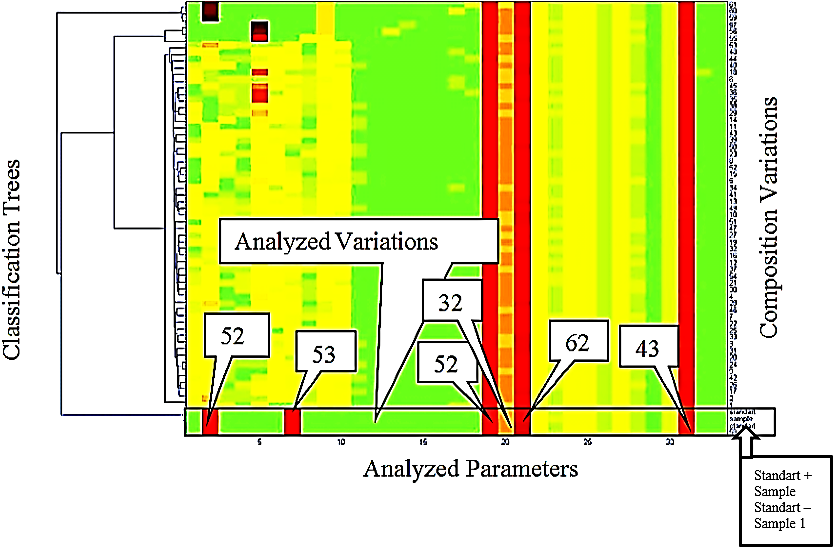 | Figure 3. Results of Analyzed Variations Clustering3 |
Within the analyzed range, there were found four composition variations with the same color scheme, and thus similar in quality to the predicted indicators (standart, sample, standart, sample 1). If the analyzed compositions are selected into separate group (Figure 4), then the diagram overlapping of the analyzed samples shows that the selected variations are identical. Despite the visual matching of the analyzed variations, the composition sample 1 is located outside the “standart” range.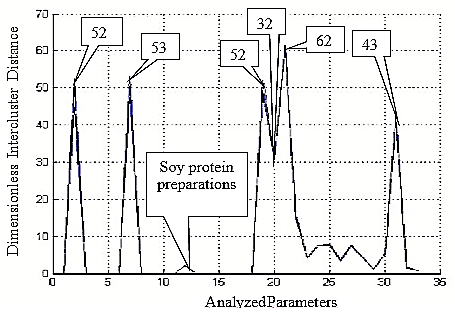 | Figure 4. Qualitative Characteristics Evaluation of the Analyzed Compositions |
This is due to an insignificant deviation of the analyzed parameters from the regulatory requirements. The analysis of the factor indicators of the sample 1 revealed the presence of an insignificant amount of soy protein preparations (analyzed indicators 12, 13 in Figure 4).Kohonen networks, it is possible to say, learns to understand the data structure. These networks are distinguished by their ability to validate not just numerical, but also textual values, which makes it reasonable to create a separate cluster for each kind of meat products using the presented method (similar to the cluster shown in Figure 3), introducing an additional column characterizing each composition variant to the table data. The analysis gives a Kohonen map which would provide a qualitative validation of the analyzed sample. Figure 5 presents Kohonen map review of the conformity of meat recipes regulatory requirements.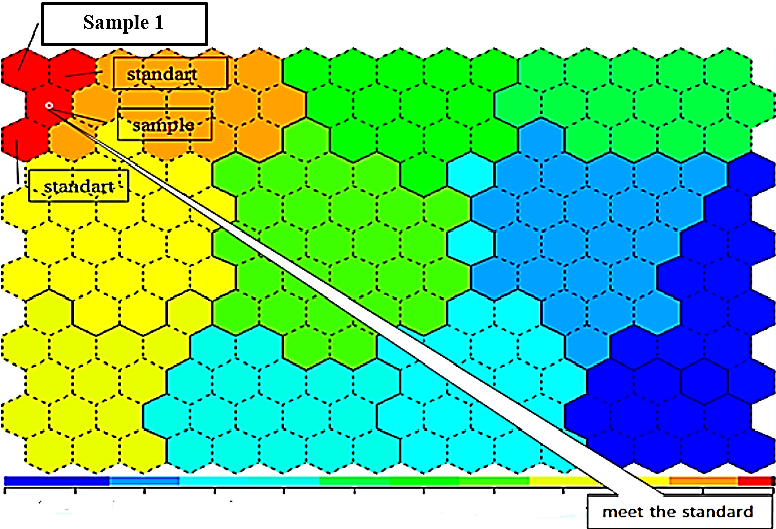 | Figure 5. Kohonen map review of the conformity of meat recipes regulatory requirements |
If you select the tabular data string in any of the investigated options, a circle appears at Kohonenmap, and on the scale of the X-axis a text, describing such composition [9, 10]. For example, it may be is close to the standard, but includes soy protein instead of beef etc. In this example, the investigated sample corresponded to regulatory requirements and on the scale of the axis «X» appears «compliance». Sample 1 appears to contain insignificant amount of soy protein, close to the standard. The terminology used in the program can be more specific, i.e. you can specify the grade of meat and quantitative content of protein supplements. Recent development of new modern devices gives the opportunity to carry out experimental trials to quickly determine chemical and amino acid profile of food products. Combining the existing quality assessment methods and the suggested framework will make effective tool for controlling conformity with standard requirements for both meat products and other foods as well.
4. Conclusions
As a result of the analytical and empirical research paired with the existing methods of quality assessment of food products, the framework for defining how the formulations of the analyzed products match the standard requirements is proposed.
Notes
1. Contents of the components in the formulation given in kg per 100 kg of the basic raw material.The structure of meat raw materials is resulted according to standards of the Russian Federation.2. The chemical composition of the test sample formulation (Sample), obtained as a result of the experiment, recalculated without added fiber and moisture in preparing the stuffing.3. The range of analyzed parameters includes components of the compositions in accordance to their ordinal number in table 1 (1 – 18) and functional indicators set in order of their position in table 2 (left to right): 19, 20 – protein and fat content in % on solids basis; 21 – 29 amino acid composition by essential amino acids and oxyproline (g/100g protein); 30 – EAA sum (g/100g protein); 31 – connective tissue composition indicator (tryptophan: oxyproline).
References
[1] | Borovikov, V., 2001, “Statistic: the art of computer’s data analysis. Professionals.” SPb., Petersburg,p.656. |
[2] | Neural networks: STATISTICA Neural Networks: Trans. from English, Moscow, Hot line, Telecom, 2001.p.182. |
[3] | Kohonen, Teuvo, 1982, "Self-Organized Formation of Topologically Correct Feature Maps", J.Biological Cybernetics 43 (1), 59–69. |
[4] | Martirosyan, V.V., 2013,A method for preparing an extract of biologically active substances”, J. Storage and processing of agricultural, Moscow, 3, 13-14. |
[5] | Brykalov, A.V.Yakuba, Y.F., Pilipenko, N.Y., Belik, E.V., P.Richomme, and Ph. Simoneau, “Modern methods of isolation and research of natural and biologically active substances and microorganisms”, Krasnodar, KSAU, 2013, p.116. |
[6] | Brykalov, A.V., and Pilipenko, N.Y., 2011, “Biological active components and there using in reception of drinks from the dairy whey”, J. Proceedings of The Kuban State Agrarian University, 6(33), Krasnodar, KSAU, 79 – 81. |
[7] | Khamitsaeva, A.S. and Britaev, B.B., 2010, “Enrichment recipes bread product plant bioactive components”, J. The commodities of food products, Krasnodar, 7, 45-48. |
[8] | Khamitsaeva, A.S., Kolodyaznay, V.S., and Каirov, V.R., 2010, “Scientific-practical bases of the use of bioactive regional plants in production of sausage and bread”, Vladikavkaz, Mountain state agrarian university, p.140. |
[9] | Limareva, N.S., Donchenko, L.V., 2014, “Innovative drinks based on vegetable juices functional purpose”, (Scientific journal KubGAU) Polythematic Network electronic scientific journal of the Kuban State Agrarian University, 01(095), 242 – 253, [Online]. Available:http://ej.kubagro.ru/2014/01/pdf/12.pdf. |
[10] | Martirosyan, V.V., Zhirkova, E.V., Malkina, V.D., and Nikolaeva, S.V., 2007, “Working out of a composit mix for manufacture of pasta”, J. Storage and processing of agricultural, Moscow, 4, 66-69. |