Yasuhiro Kakihara1, Tetsuya Takiguchi1, Yasuo Ariki1, Yasushi Nakai2, Satoshi Takada3
1Graduate School of System Informatics, Kobe University, Kobe, Japan
2Graduate School of Education, University of Miyazaki, Miyazaki, Japan
3Graduate School of Health Sciences, Kobe University, Kobe, Japan
Correspondence to: Tetsuya Takiguchi, Graduate School of System Informatics, Kobe University, Kobe, Japan.
Email: |  |
Copyright © 2015 Scientific & Academic Publishing. All Rights Reserved.
Abstract
Recently, autistic spectrum disorders (ASD) have been the focus of much research. Anautistic spectrum disorder is a congenital cerebral dysfunction, and it is a type of developmental disease that causes difficulties incommunication, perceptual, cognitive, and linguistic functions. Since the symptoms of an autistic spectrum disorder are the result of a variety of causes, a fundamental, all-encompassing medical treatment is difficult. However, for an autistic spectrum disorder, early detection and suitable education can have a significant impact on future social prognosis. In this paper, for the purpose of early-age detection of ASD, an investigation of classification using pitch features is carried out for children with autism spectrum disorders and typically developing children, where statistics (percentiles, moment, maximum, minimum, and range) for static and dynamic pitch features are used for classification. Experimental results show 1) that a section division (the head, middle, and tail sections) of an utterance provides a better accuracy, compared with no section divisions of an utterance, and 2) the section that contributed to the classification of ASD was the head section of the utterance.
Keywords:
Children with autism spectrum disorders, Pitch features, Intonation
Cite this paper: Yasuhiro Kakihara, Tetsuya Takiguchi, Yasuo Ariki, Yasushi Nakai, Satoshi Takada, Investigation of Classification Using Pitch Features for Children with Autism Spectrum Disorders and Typically Developing Children, American Journal of Signal Processing, Vol. 5 No. 1, 2015, pp. 1-5. doi: 10.5923/j.ajsp.20150501.01.
1. Introduction
Many speech recognition technologies have been studied for adults to date. Recently, research has been carried out for children, elderly people and people with disabilities [1, 2, 3], and research related toautistic spectrum disorders (ASD) has also been focused on. Anautistic spectrum disorder is a congenital cerebral dysfunction, and it is a type of the developmental disease that causes difficulties incommunication, perceptual, cognitive, and linguistic functions [4-6]. Children with autisticspectrum disorders are diagnosed as having less than normal social interaction and linguistic skills, and have restricted interests and a stereotypical pattern of behavior [7-9]. It is estimated that the rate of autistic disorders, such as Asperger’s syndrome [10] and nonspecific pervasive developmental disorders, is between 1% and 2% of all children [11]. Since the symptoms of an autistic spectrum disorder are the result of a variety of causes, a fundamental, all-encompassing medical treatment is difficult. However, early detection and suitable education can have a significant impact on the future social prognosis of children with such disorders. Recent research has demonstrated that early-stage support, which specializes in autistic spectrum disorders (such as Picture Exchange Communication System [12]), is effective [8]. In the field of acoustic technology, however, there has been little research focused on discriminating between children with autismspectrum disorders and typically developing children.This paper reports the results of classification experiments carried out using pitch features for children with autism spectrum disorders, where statistics (percentiles, moment, maximum, minimum, and range) for static and dynamic pitch features are calculated, and a support vector machine (SVM) is used for classification. Experimental results show that a section division (the head, middle, and tail sections) of an utterance provides a better accuracy, compared with no section divisions of an utterance, and the section that contributed to the classification of ASD was the head section of the utterance.
2. Pitch Features
Some analyses based on pitch features of ASD have been reported [13, 14], and in this paper, the effectiveness of pitch features is investigated for classification of children with autismspectrum disorder and typically developing children.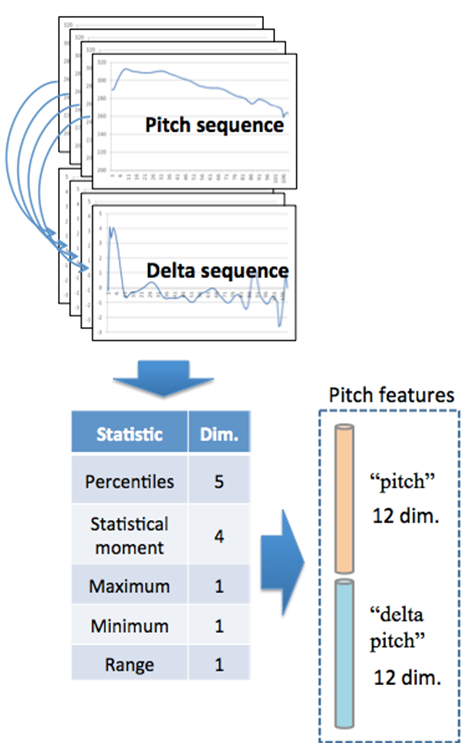 | Figure 1. Pitch features |
Figure 1 shows an overview of pitch feature calculation. In this paper, pitch features consist of 12statisticsfor the time sequence of static and dynamic (
) pitchas follows:• 25th percentile,
25th percentile• 50th percentile,
50th percentile• 75th percentile,
75th percentile• 25-50 percentile difference,
25-50 percentile difference• 50-75 percentile difference,
50-75 percentile difference• mean,
mean• standard deviation,
standard deviation• kurtosis,
kurtosis• skewness,
skewness• maximum,
maximum• minimum,
minimum• range (max. – min.),
rangeThe dynamic feature is calculated using the following regression formula [16]:
where
is the delta coefficient at time t calculated in terms of the corresponding static pitch features from
to
is the parameter related to time (in a delta window size), and
is the delta window size
in our experiment).An example of pitch features is shown in Figure 2, where the top figure shows the 50th percentile and the minimum of static pitch and the bottom one shows the mean and the
25th percentile.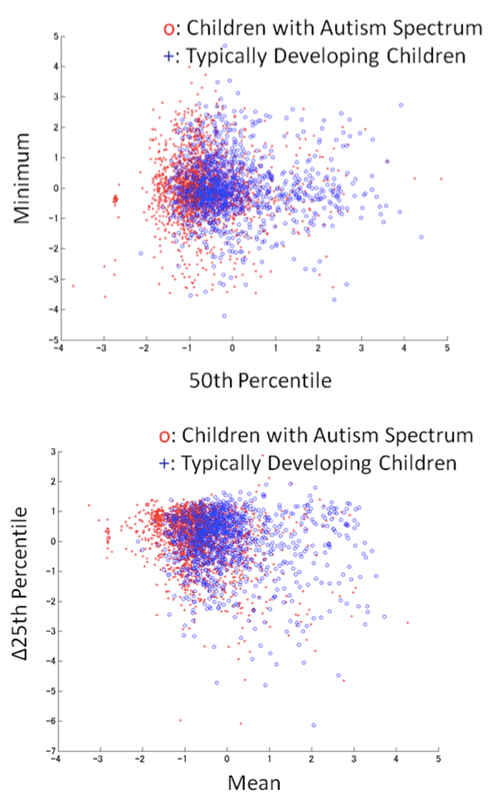 | Figure 2. An example of pitch features |
These pitch features are calculated in three steps:1. Extraction of the (static) pitch for every 10 msec.2. Calculation of the dynamic pitch from the static pitch.3. 12 statistics are calculated from the static and dynamic pitch.In this paper, STRAIGHT [15] is used for extraction of the (static) pitch. The pitch features are normalized to have zero mean and unit variance. Then, the normalized features are classified using a SVM.
3. Speech Corpus
In our experiments, we recorded the Japanese speech data of children with autismspectrum disorders and typically developing children because there is no common or commercial Japanese speech corpus of children with autism spectrum disorders [13]. The children ranged in age from kindergarteners through the fourth graders. This database consists 30 ASD children and 54 typically developing children. ASD participants were recruited from among the 4-to 9-year-old children who visited the Developmental Behavioral Pediatric Clinic of Kobe University Hospital between April and July, 2010, after approval from the Medical Ethics Committee of Kobe University Graduate School of Medicine was received. Each child uttered 50 kinds of words. Table 1 shows the condition of the Japanese speech corpus of children with autism spectrum disorders.Table 1. Japanese speech corpus for classification of children with autism spectrumdisorders and typically developing children 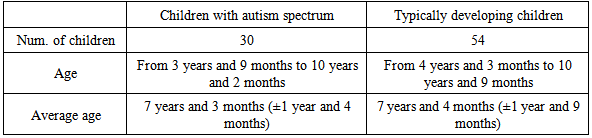 |
| |
|
4. Experiments
Three types of classification experiments were carried out using pitch features.
4.1. Classification Results for Section Division of Utterances
In this subsection, as shown in Figure 3, an utterance is divided into three sections (the first (head), second (middle), and third (tail) sections), and 24th-dimension pitch features are calculated from each section.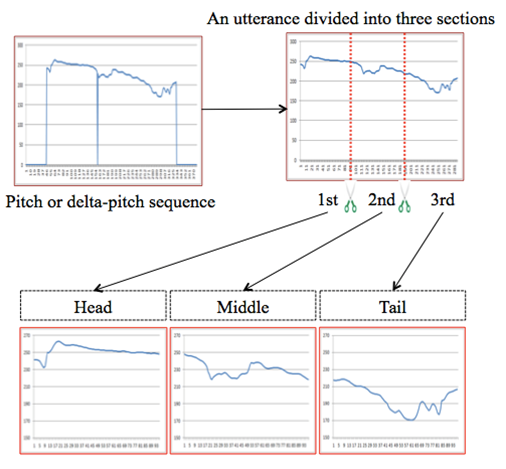 | Figure 3. Pitch interval divided into three sections |
Table 2 shows the classification results for 7 combinations of three sections, where the speech database is divided into 10 and 10-fold cross-validation is applied to our experiment in this subsection. The average over the ten results from the folds was computed.Table 2. Classification results for three divided sections (head, middle, and tail) of an utterance 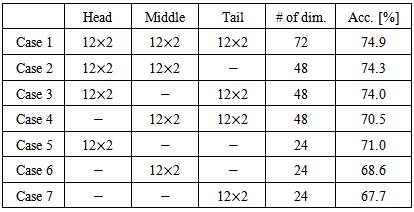 |
| |
|
The baseline (w/o division) of classification accuracy is 73.2%, where a 24th-dimension feature vector is calculated from the pitch and delta pitch sequences for each utterance. As shown in Table 2, the highest accuracy is obtained for Case 1, where an utterance is divided into three sections (the first (head), second (middle), and third (tail) sections), and 24th-dimension pitch features are calculated from each section (total number of dimensions: 243=72). In the case of two sections (from Case 2 to Case 4), the performance of Case 2 and Case 3 (which include the head section) is better than that of Case 4. In the case of one section (from Case 5 to Case 7), the performance of Case 1 (which includes the head section) is better than those of other cases. Therefore, the experimental results show that the head interval of the utterance greatly contributes to the classification of ASD. This may be due to a strain of the first lip movement when people with this disorder begin to speak.
4.2. Classification Results for Each Feature
In this subsection, each pitch feature is evaluated without section divisions. As shown in Table 3, pitch features are grouped into three categories: percentiles (5-dim. pitch features and their delta features), statistical moments (mean, standard deviation, kurtosis, skewness, and their delta features), and etc. (maximum, minimum, range, and their delta features).Table 3. Classification results for each feature 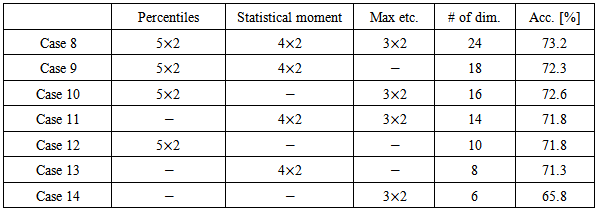 |
| |
|
The highest accuracy is obtained for Case 8, where a 24th-dimension feature vector is calculated. The experimental results show that all pitch features are useful for classifying children with autism spectrum disorders and typically developing children.
4.3. Classification Results for Each Word
In this subsection, a SVM is trained for each word, where ten words (/ashi/ (foot), /enpitsu/ (pencil), /inu/ (dog), /kirin/ (giraffe), /megane/ (glasses), /ringo/ (apple), /sakana/ (fish), /semi/ (cicada), /terebi/ (television), /tsukue/ (desk)) are used in this experiment (/Japanese/ (English)). The experiments were carried out based on leave-one-out cross-validation, where a 24th-dimension feature vector is calculated from the pitch and delta pitch sequences for each utterance (without section divisions).Figure 4 shows classification results for each word, where the word is sorted by decreasing accuracy order. The experimental results show that the words that obtained a high accuracy are /enpitsu/, /ashi/ and so on. On the other hand, the words that had a low accuracy are /kirin/, /inu/, and so on. The difference between the highest and lowest accuracies is 9.08%. In the future research, the relationship between the word and its classification accuracy will be investigated in detail.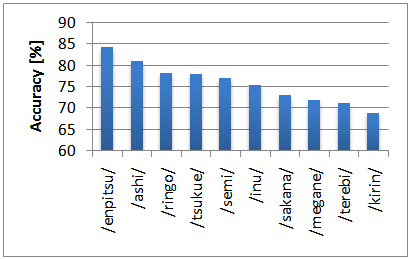 | Figure 4. Classification result for each word |
5. Conclusions
This paper described, for the purpose of early-age detection of autistic spectrum disorders (ASD), the results of classification experiments using pitch features carried out on children with autism spectrum disorders and typically developing children, from kindergarteners through the fourth graders. In our approach, an utterance is divided into three sections (the first (head), second (middle), and third (tail) sections), and 24th-dimension pitch features are calculated from each section. The experimental results show that the head interval of the utterance greatly contributes to the classification of ASD. This may be due to a strain of the first lip movement when those with ASD begin to say a word. In the future, the relationship between the text content (the word uttered by subjects) and its classification accuracy will be investigated in detail. Also, we will perform experiments that involve increasing the amount of speech data.
References
[1] | R. Aihara, R. Takashima, T. Takiguchi, and Y. Ariki, “A preliminary demonstration of exemplar-based voice conversion for articulation disorders using an individuality-preserving dictionary,” EURASIP Journal on Audio, Speech, and Music Processing, 2014:5, doi:10.1186/ 1687-4722-2014-5, 10 pages, 2014. |
[2] | T. Yoshioka, T. Takiguchi, and Y. Ariki, “Robust Feature Extraction to Utterance Fluctuation of Articulation Disorders Based on Random Projection,” in Proc. 4th Workshop on Speech and Language Processing for Assistive Technologies, pp. 129-133, 2013. |
[3] | T. Nakashika, T. Yoshioka, T. Takiguchi, Y. Ariki, S. Duffner, and C. Garcia, “Dysarthric Speech Recognition Using a Convolutive Bottleneck Network,” IEEE International Conf. on Signal Proc. (ICSP), pp. 505-509, 2014. |
[4] | L. Wing, “Asperger’s syndrome: a clinical account,” Psychological Medicine, pp. 115-129, 1981. |
[5] | L. Wing, “Past and future of research on Asperger syndrome,” Asperger syndrome, pp. 418-432, 2000. |
[6] | E. B. Caronna, J. M. Milunsky, and H. Tager-Flusberg, “Autism spectrum disorders: clinical and research frontiers,” Archives of Disease in Childfood, vol. 93, no. 6, pp. 518-523, 2008. |
[7] | M. Rutter, “Incidence of autism spectrum disorders: Changes over time and their meaning,” Acta Paediatrica, vol. 94, no. 1, pp. 2-15, 2005. |
[8] | C. Plauche, J. Scott, M. Myers et al., “Management of children with autism spectrum disorders,” Pediatrics, vol. 120, no. 5, pp. 1162-1182, 2007. |
[9] | S. J. Rogers and L. A. Vismara, “Evidence-based comprehensive treatments for early autism,” Journal of Clinical Child & Adolescent Psychology, vol. 37, no. 1, pp. 8-38, 2008. |
[10] | A. Klin, D. Pauls, R. Schultz, and F. Volkmar, “Three diagnostic approaches to Asperger syndrome: implications for research,” Journal of Autism and Developmental Disorders, vol. 35, no. 2, pp. 221-234, 2005. |
[11] | H. Honda, Y. Shimizu, and M. Rutter, “No effect of MMR withdrawal on the incidence of autism: a total population study,” Journal of Child Psychology and Psychiatry, vol. 46, no. 6, pp. 572-579, 2005. |
[12] | A. Bondy and L. Frost, A Pictures’s Worth: PECS and Other Visual Communication Strategies in Autism. Topics in Autism, ERIC, 2012. |
[13] | Y. Nakai, R. Takashima, T. Takiguchi, and S. Takada, “Speech Intonation in Children with Autism Spectrum Disorder,” Brain and Development, Vol. 36, Issue 6, pp. 516-522, 2014. |
[14] | L. D. Shriberg, R. Paul, J. L. McSweeny, A. Klin, D. J. Cohen, and F. R. Volkmar, “Speech and Prosody Characteristics of Adolescents and Adults with High-Functioning Autism and Asperger Syndrome,” Journal of Speech, Language and Hearing Research, vol. 44, no. 5, p. 1097, 2001. |
[15] | H. Kawahara, I. Masuda-Katsuse, and A. deCheveigne, “Restructuring Speech Representations Using a Pitch-Adaptive Time-Frequency Smoothing and an Instantaneous-Frequency-Based F0 Extraction: Possible Role of a Repetitive structure in sounds,” Speech Communication, vol. 27, no. 3, pp. 187-207, 1999. |
[16] | HTK Book (Hidden Markov Model Toolkit), http://htk.eng.cam.ac.jp/docs/docs.shtml. |