Adikpe Iyata1, Omotunde Ayokunle2
1Software Development and Analysis, The Smarthub Technologies and Solutions, Abuja, Nigeria
2Department of Computer Science, Babcock University, Ogun State, Nigeria
Correspondence to: Adikpe Iyata, Software Development and Analysis, The Smarthub Technologies and Solutions, Abuja, Nigeria.
Email: |  |
Copyright © 2018 The Author(s). Published by Scientific & Academic Publishing.
This work is licensed under the Creative Commons Attribution International License (CC BY).
http://creativecommons.org/licenses/by/4.0/

Abstract
Operations Research (OR) is an interdisciplinary field that finds its roots in applied mathematics, automates its underlying mathematical processes using computer science systems and mechanisms, and produce results for enhancing managerial decision making. The profession has grown and evolved from the use of traditional mathematical techniques for optimization of processes through to a stage where advanced computer-based techniques such as modelling and simulation are being used to carry out OR studies. This growth has been engendered by two main factors – wide acceptance by academic institutions; and, the impact of Computer Science tools and programs used for carrying out activities during OR studies. No country develops beyond its growth in science and technology. This paper presents the role Computer Science has played in the development of OR and consequently the role of Computer Scientists in each phase of OR studies. The paper also reveals the future prospects of OR as research in the area of Artificial Intelligence continues to find relevance in OR (i.e., Artificial Operations Research [AOR]). The paper concludes by presenting key recommendations for immediate action by the Nigerian government, business owners, academia and OR teams in order to position the country as a producer and exporter of OR tools and techniques and not just a consumer of OR techniques.
Keywords:
Operations Research (OR), Computer Science, Computer Scientists, Artificial Operations Research
Cite this paper: Adikpe Iyata, Omotunde Ayokunle, Towards Fostering the Growth of Operations Research in Nigeria: The Role of Computer Scientists, American Journal of Operational Research, Vol. 8 No. 1, 2018, pp. 1-9. doi: 10.5923/j.ajor.20180801.01.
1. Introduction
Throughout the development of Operations Research, the solution of OR models has been a constant concern for Computer Technology [1]. Design of user interfaces, data/knowledge management in information systems and distributed information processing have all been significant drivers of OR growth [1]. Operational Research Society of America (ORSA), The Institute of Management Sciences (TIMS), Institute for Operations Research and the Management Sciences (INFORMS); all unanimously agree that: “Operations Research and Management Sciences are the disciplines that deal with the application of information technology for informed decision-making” [1]. This suggests that OR growth and development has been stimulated majorly by the contributions of computer science and information systems. Borrowing from the metaphor of the orchestra: the people are the businesses, organizations and corporations who need to be at their best possible performance levels (i.e., profit maximization or cost minimization); the instruments are the OR tools and techniques necessary for computation; the music is the continuous optimization and management of organizational processes; and, the conductor are the Computer Scientists. Thus, the music has to be produced using the instruments in a rhythm that is dictated by the guidance of the conductors for the people who need to listen to continuous ‘best’ music. In essence, optimization and management of organizational processes has to be done using OR tools and techniques as have been automated by Computer Scientists. This paper reveals the extents to which Computer Scientists can and may function within phases of operations research studies, the future prospects of operations research in the area of Artificial Operations Research and also presents the lead way for Nigeria to go in order to harness the potentials of OR as well as in advancing the profession.
2. What is Operations Research?
Operations research as a discipline has been found to have myriads of definitions. This may be credited to the fact that various disciplines view the practice (with respect to the underlying art and science) from different perspectives. This has been attributed to the broad nature of the discipline which may have rendered the adoption of a consensus on definition unrealistic [2]. From the perspective of health practitioners: “Operations Research is the search for knowledge on interventions, strategies or tools that can enhance the quality, effectiveness or coverage of programs in which the research is being conducted” [3]. This definition views operations research as a purposeful quest for knowledge to be applied to an existing system in order to make improvements to the defunct processes embodied by such a system.Managerial Sciences tend to adopt definitions that capture both the artistic and the scientific procedures undergone in operations research studies. Operations Research has been described as a science-informed approach to decision making which seeks to determine how best to design and coordinate a system usually under conditions where allocation of scarce resources is of interest [4]. The “Research” component of the term Operations Research suggests the application of well-known scientific methods such as: observation of a system, scenario or process and formulation of a problem; data collection; model formulation to abstract the essential components of such a system; hypothesizing that the model represents the stated problem; experimentation on the model (model solution) to verify if the hypothesis is true; and finally, understandable decision alternatives are extracted from the results of the experiments so managers can select for possible implementation [4].Others view OR as a consortium formed by the active participation of experts from various disciplines, applying scientific methods to problems akin to the control of organized systems with the aim of proffering solutions that serve the purpose of the organization as a whole [5]. The contributory dimension taken by this definition depicts OR as a ‘discipline of disciplines’ and not just one that is knotted to any one field of study. Experts from both the sciences and arts all play some role in OR studies.One may also perceive OR as a tool box of scientific and programmable rules that offer managers at all levels with measureable basis for decision-making [2]. This perspective in essence directly presents scientific processes as the goose that lays the eggs for sound decision making by managers of organizations.In adopting a definition for Operations Research through the lenses of computer scientists, it is imperative to state clearly who a Computer Scientist is, as well as defining Computer Science as a discipline. These have been issues of debate in the jury of the discipline of Computer Science as to what and who Computer Science and Computer Scientists are respectively.
3. What is Computer Science?
In a survey of some of the proposed definition for computer science by a sufficiently broad base of opinions, some coming from computer science professionals and others from arguably novices in the profession, it was suggested that Edsger Dijkstra’s definition of computer science as: “what (abstract) mechanisms we can conceive without getting lost in complexities of our making”; as being devoid of implementation concerns which the discipline typifies as well by the researcher’s opinion [6].The work also presented the definition put forward by the Computing Sciences Accreditation Board (CSAB) which describes computer science as “a discipline that involves the understanding and design of computers and computational processes” [6]. The researcher was of the judgment that this definition is accommodative of tasks that may not be akin to computer science [6].However, the researcher was of the opinion that it is preferable to describe what a computer scientist does in a bid to defining the science itself. It was at such compromise that the researcher accepted Dirk Siefkes’ description of a Computer Scientist as “one who discusses problems, describes solutions, designs and uses computers and formalisms” [6].From the forgoing, this study dares to infer a definition of computer science as the discussion of problems, description of solutions through applied principles of science with the use of appropriate data structures and tools, and, the design and use of computers in solving practical problems.This definition has been coined and found befitting enough to capture the essential characteristics of the discipline as a science that follows laid down scientific methods, and as well, addresses the issue of relegating the discipline to one of merely theoretical/conceptual relevance as Dijkstra’s definition may have suggested.It is on the premise of this definition of computer science and drawing from the interdisciplinary formation of OR teams that this study presents Operations Research as an interdisciplinary field that finds its roots in applied mathematics, automates its underlying mathematical processes using computer science systems and mechanisms, and produce results for enhancing managerial decision making.
4. History of Operations Research
Operations Research was born out of need for efficient resource allocation. During World War II, a group of scientists were tasked with carrying out research on military operations by the British and US military managements through the application of scientific approaches for dealing with resource allocation concerns to the various tactical and strategic problems. These were the fathers of Operations Research [7].The industrial revolution that succeeded the end of the war was characterized by steady growth of organizations in size, structure and operational complexities. As much as these were welcome developments that soared profits, it came along with challenges in coordinating operations of all facets of the organization in such a manner that would guarantee efficient allocation of resources to all components in ‘a best possible’ fashion. Another issue was maintaining a balance between the rate of growth in the individual components of the organization and the overall growth of the organization; ensuring that departmental goals do not overshadow organizational goals [7].These pressing needs in the early 1950’s consequently prompted the management of various organizations to seek more scientific solutions to their managerial decision-making problems by employing the services of Operations Research Scientists [8]. This laid the bedrock for the emergence of operations research as a discipline.A good number of umbrella professional bodies have been formed over the years including: Operational Research Society of America (ORSA), American Institute of Decision Sciences (AIDS), The Association of European Operational Research Societies (EURO), The Institute of Management Sciences (TIMS) now known as Institute For Operations Research and Management Sciences (INFORMS), International Federation of Operational Research Societies (IFORS); which is an organization made up of national Operations Research Societies all over the world [2], Institute For Operations Research of Nigeria (INFORN), and, the Institute of Operational Research and Management Science of Nigeria (IORMS). All these assist in maintaining both regional and international standards for the practice of the profession.
5. Development Stages of Operations Research
Four phases of OR development (i.e., The Primitive -, Academic -, Maturing -, and Matured Phase of OR) have been identified over time since its inception [2, p. 2]. This paper proposes the Intelligence phase as the new phase of OR development which hinges on the intermarriage between OR and Artificial Intelligence (AI). Distinct characteristics of these phases are presented below:a. The Primitive Phase (1940 – 1960): characterized by high interest in practical application of scientific techniques to solving operational problems. Less amount of sophisticated computation was required, hence, mathematical processes and low-end computing devices were by and large sufficient for computation. A handful of Universities trained students on the use of OR techniques.b. The Academic Phase (Early 1960s): over 500 percent increase in the number of Universities that offered formal training in OR was witnessed in this stage. Aggressive development of theoretical framework for the discipline and less focus on practical application characterized this stage. A notable factor that spurred this rapid development was the availability of high speed computers. The presence of OR analyst as executives in various industries and organizations facilitated easier acceptance and implementations of recommendations from OR studies.c. Maturing Phase (the 1970s): this phase saw the drive towards establishing an equilibrium between theory and practice gain appreciable momentum. Managers had a better insight into what OR could and could not achieve; more fact based research as opposed to developing theoretical techniques; better integration of behavioural, functional and quantitative analysis; and, more attention paid to developing processes that could result in obtaining solutions to mutable problem circumstances were all milestones from this phase.d. The Matured Phase (21st Century): the use of software packages to lessen the burden of tedious mathematical computation changed the narrative of the practice for the better during this phase [2]. The use of modelling and simulation software packages are also instrumental in reducing the costs (e.g., time taken to manually compute and analyze data, collateral damage from implementation of solutions from poorly formulated models) associated with OR studies also helped stabilizing OR’s growth during this phase. OR was then tailored into the curriculum of students in management sciences and engineering across a good number of universities around the globe [2, p. 2].e. Intelligence phase: AI is a tool for aimed at achieving automated decision making. Formally, both fields had little or no crossroads in terms of collaboration, but, in more recent times, meeting points between both fields have been reached. It is essential to note that both disciplines are concerned with human problem solving and decision making even though they both approach these tasks from different perspectives and with the use of different methodologies and tools. Both fields leverage on similar tools such as graph, probability theory and heuristics [9].AI has a strong foundation in logic and emphasizes automatic theorem proving; whereas, OR is concerned with the mathematics of optimization and quantification through utility and value functions [10]. These foundational distinctions render both fields incapable of handling the complex nature of most real world decision problems completely as standalones. Therefore, the future of OR lies heavily on the extents to which both fields can intermarry for the purpose of leveraging on their individual strengths.AI techniques which provide richer and more flexible representation of real world problems would come in handy to alleviate some of the challenges faced during the use of OR techniques that are traditionally made up of rigid models with limited expressive power [9]. The research further identified OR techniques and processes such as: Duality, Problem Structure Formalization, Cutting Planes and Constraint Propagation; as having the potentials of being enhanced by AI techniques as the future of OR in handling complex real-world problems [9].Procedures embodied by both AI and OR are highly computer dependent [10] and are designed by Computer Scientists who are well abreast with the approaches employed by each of the fields in tackling decision-making and problem solving concerns. Therefore, in order for Nigeria to be a contributor to the growth of OR profession at the international scene, it has to place more focus in building human capacity that would be able to function in the coming era of OR evolution; which is, Artificial Operations Research (AOR).From the forgoing, it is noteworthy that the major drivers of reasonable and substantive growth in OR are arguably the wide acceptance of the discipline by higher institutions of learning as either full-time programs, part-time programs or mandatory courses taken within the curriculum of other academic disciplines. Also, the development of supporting software packages to ease the tedious underlying computations involved with OR studies has changed the narrative of the discipline significantly.
6. Phases of OR and the Participation of Computer Scientists
Inasmuch as OR has been viewed more as a management science, the contribution of Computer Science has either not been fully appreciated or such potential is yet to be properly projected in literature. A careful examination of the various phases involved in OR studies with an objective view on where Computer Scientists can and may function has been taken below:Problem definitionLike every other science, the first step to solving a problem in OR is identifying the problem. But in the case of OR teams, problems are not just given to the team to solve however they see fit; rather, they are to perform detailed analysis of the problem and provide alternative courses of action to management for consideration and possible action [7, pp. 8-10]. In cases where the OR study is to be undertaken on demand by management, the problem at hand would be readily provided by management to the OR team; otherwise, expertise and experience on the part of the OR team is required to identify the processes within the organization that could be optimized. It is also essential for the OR team to fine-tune vaguely defined problems into structured formats with appropriate objectives, constraints on resources, interrelationships between the area being studied and other areas within the organization, and, the determination of the research time. For the delicacy of the problem definition phase, such tasks as highlighted above are arguably best handled by experienced OR analysts.Data Gathering and AnalysisThis entails sourcing for all possible data that may be needed as input for the mathematical model. Most often than not, OR teams spend considerably large amounts of time gathering data owing to reasons such as: unavailability of sought data because of no/poor record keeping pattern by the organization, sieving out of irrelevant data from the available data store, storage of data in wrong forms and the use of non-computerized data storage systems [7, p. 9]. Techniques and tools such as: data mining from large databases managed by organizations in order to make new meaning of existing data sets, and, using online simulation of data for the purpose of analysis [11] are used during this phase. Tools such as Common Online Data Analysis Platform (CODAP) [12] and NOVA On-line fire-growth simulation [13] require computer science expertise for the purpose of data analysis.Model FormulationA model is a simplification of the real world occurrence or phenomenon. It is a miniature of something or an example for emulation [14]. In this phase, the defined problem is translated into mathematical symbols and expressions. For business problems, the system of equations and expressions that describe the essence of the problem constitute the model [7, p. 11]. If the model fits any of the standard mathematical models (e.g., linear -, integer -, goal - programming) then an algorithmic approach is followed in obtaining a solution; otherwise, complex models that cannot be solved analytically and which do not readily fit available standard mathematical models could be solved using heuristic and simulation methods. Handling the design of simulation models, simulation experiments, execution of simulation runs and finally performing analysis on the result sets from the simulation runs are tasks that require high level of expertise and mastery of the use of computer simulation software packages and tools. The active engagement of computer scientists that have such proficiencies as part of the operations research team would lessen the burden of training team members that do not have these skillsets to carry out such tasks, and by implication, lessen the entire research time.For reason that OR is intended to assist managers in decision making, the mathematical models usually express decision alternatives as decision variables. The sought objective (E.g., cost minimization or profit maximization) is then expressed as a mathematical function of the decision variables and the resulting function is referred to as the objective function. The values that the decision variables can assume are bound by certain restrictions owing to factors such as scarcity of resources and organizational and/or government policy restrictions on certain actions. These limits are expressed as inequalities or equations and are collectively called the constraints of the model. All constants within the model are referred to as the model parameters [7, pp. 10-11]. It is important to point out that standard mathematical models such as linear programming could be solved using computer programming languages. Standard algorithms for solving such models are to be followed in writing the computer programs. This is a process that can be effectively handled by computer programmers.The peculiarity of the model may necessitate the combination of the analytical, heuristic and the simulation models [15].Model SolutionThis phase has been perceived to be the simplest of all phases because it involves the use of well-defined optimization algorithms [15, p. 44]. It necessitates the development of a procedure (usually a computer-based procedure) for deriving solutions to the problem from the model [7, p. 14]. These procedures are executed using software packages and a search for ‘an optimal’ solution is carried out. The software packages used for executing these procedures are designed and developed by Computer Scientists who have an understanding of both the nature of the mathematical models usually used for OR studies and also the underlying procedures for solving these models.There are a couple of concerns on the optimality of solutions from models. Since models are miniature representations of real world problems, there is the risk of over simplification; that is, the risk of omitting important parameters of the problem from the formulated model [14]. In the event that an over simplified model is formulated, an optimal solution for the model may not be the best possible solution to the problem at hand. But if the model considerably abstracts most of the important parameters of the problem, then an optimal solution for the model may just as well be optimal for practical implementation on the real problem/system [7, p. 14].Another essential component of the model solution phase is carrying out Sensitivity Analysis. Sensitivity analysis helps in giving insights into the extents to which the proposed optimum solution remains valid when parameter values are perturbed. Also, when the parameters of the model cannot be estimated accurately, sensitivity analysis suffices in determining how the solution can perform under changing conditions [15, p. 44].Model ValidationModel validation seeks to answer the questions – have we solved the right problem? [14, p. 25]; and, does solution from the model reflect the behaviour of the real system under study? [15, p. 44]. This phase juxtaposes results gotten from solving the model with actual data from operating the existing system using similar parameter values or input conditions as has been observed in the real system. The goal here is to show that both the model and the solution are realistic enough for management to rely on for decision making [2, p. 5].In the event that the study is not carried out to optimize any existing system but rather to evolve a new system, no historical data would be available for comparing model results with. Consequently, the use of simulation as a technique for verifying the output of the mathematical model suffices. Simulation experiments must then be designed, simulation runs are executed on different sets of input conditions and analyses are carried out on the results from the simulations in order to have an idea of how such a system would eventually perform. Again, the services of Computer Scientists that are skilled in the science and art of translating mathematical model parameters into simulation experiment input conditions become invaluable.This process should be iterative as the sets of input conditions are varied at each experimental design. Below, Fig 1.0 illustrates this process: 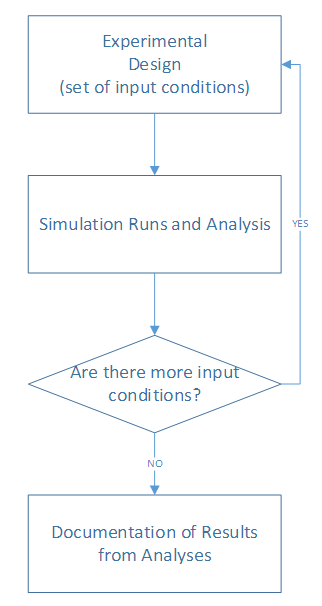 | Figure 1.0. Validation using simulation as a tool when a new system is being developed (Partly adopted from [14, p. 28]: “Model Development Lifecycle (Expanded) – a fundamentally iterative process”) |
Any form of anomalies in output from the model are spotted and debugged in this phase.ImplementationAll solutions derived from the validated model are translated into comprehensible operating instructions for management to take necessary action based on their judgment. The OR team is not responsible for implementing solutions derived from OR studies but rather the top level management is [16]. The OR team is therefore saddled with the responsibility of ensuring that management is carried along all through the course of the study in order to readily gain their support in implementing whatever solutions the study may have produced.The results, and, the decisions made based on the results provide feedback to the original model [2, p. 5]. This loop structure suggests that OR studies are continuous and iterative rather than a one-stop-solution affair.DocumentationAlthough many authors tend to either fasten this phase to the Review and Maintenance phase or even exclude it entirely from the OR phase cycle, this research considers it an equally crucial phase in any OR study and should be treated with no levity. It involves clear recording of the methodology and results obtained at all phases of the study [7, p. 20]. Some of the reasons why this should be done are: For reproducibility: In the event that a similar study is to be conducted, the OR team can easily refer back to the previous study that has been documented as a guide. For extensibility: As the need may arise to advance the study or to optimize the current system to cater for new problems, a properly documented OR study provides a healthy base for understanding the allowable limits for modification of the current model through information gathered during previous sensitivity analysis. For contingency: A change in the team formation due to a couple of unforeseen factors such as the demise of a key team member or introduction of new members to the team; change in organizational management, introducing decision makers that may not have been part and parcel of the study from the onset; or any other eventuality as the case may be can prove deleterious to the current study or its future prospects. A well-documented study would help new team members seamlessly fit in to specific team roles upon careful review of such records. Also, the OR team can easily familiarize new management with the direction and prospects of the study when proper documentation has been maintained.Documentations may be maintained locally for the organization using computer storage devices, hard copy prints or on cloud storage platforms. Although all methods have their advantages and pitfalls, it is the duty of the Documentation Master to decide on the best possible media for keeping documentation. Computer Scientists who are grounded in database design and maintenance, cyber security and data science can efficiently perform such functions as deciding the best, secure and appropriate media for documentation.Review and MaintenanceThe performance of the model has to be closely monitored to ensure it still serves the purpose for which it was implemented. Since organizational problems do not remain constant, solutions as well do not remain eternally optimal. Hence, it is imperative that the OR team revisits the model as changes are observed in the system environment over time [7, p. 20] [2, p. 5].The figures (i.e., flowcharts), 1.1 and 1.2 represent the flow of the Operations Research phases and the possible contribution of Computer Scientists at each phase respectively.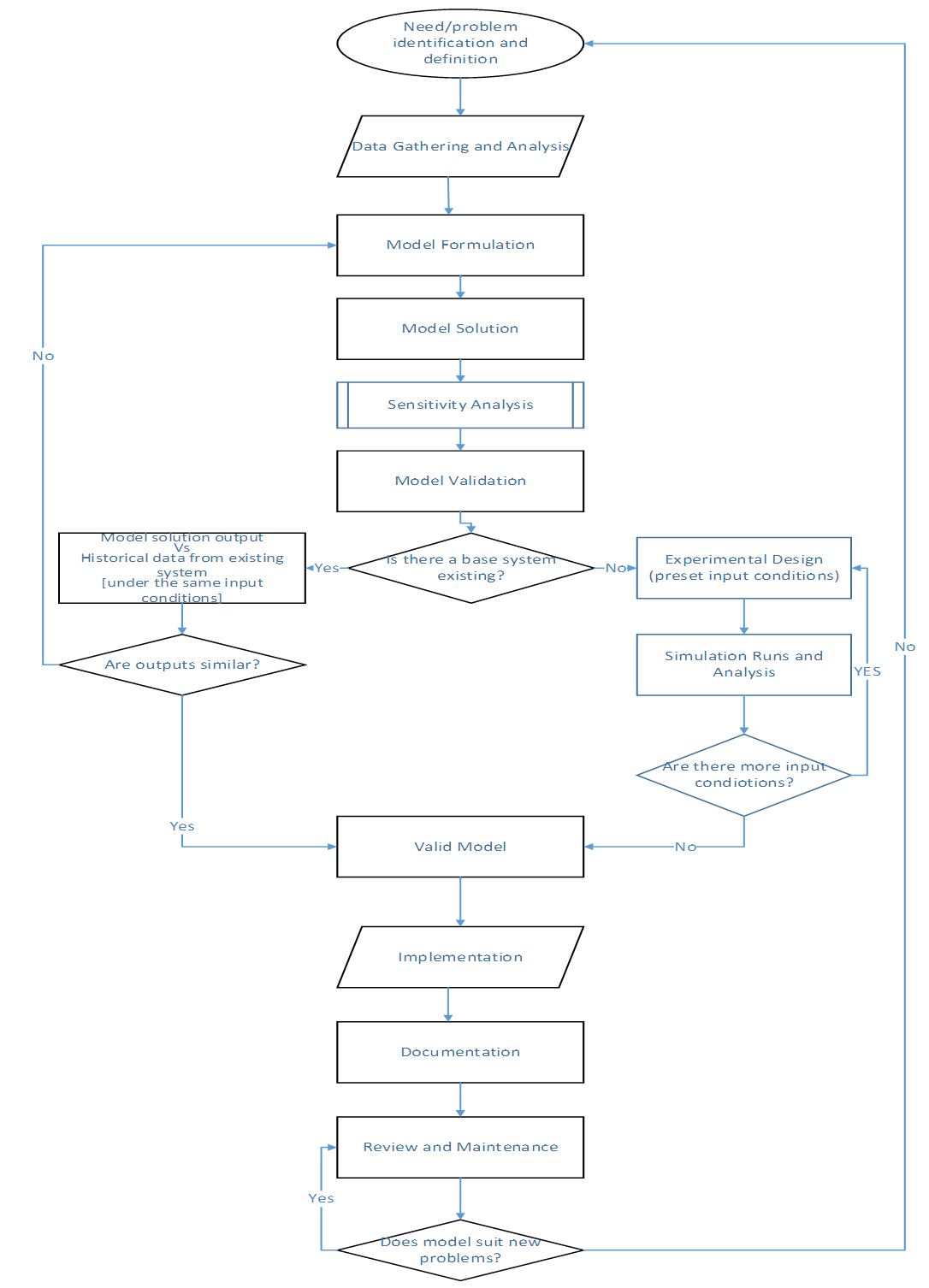 | Figure 1.1. Flowchart representing the phase-transition structure of Operations Research studies (Partly adopted from [14, p. 28]) |
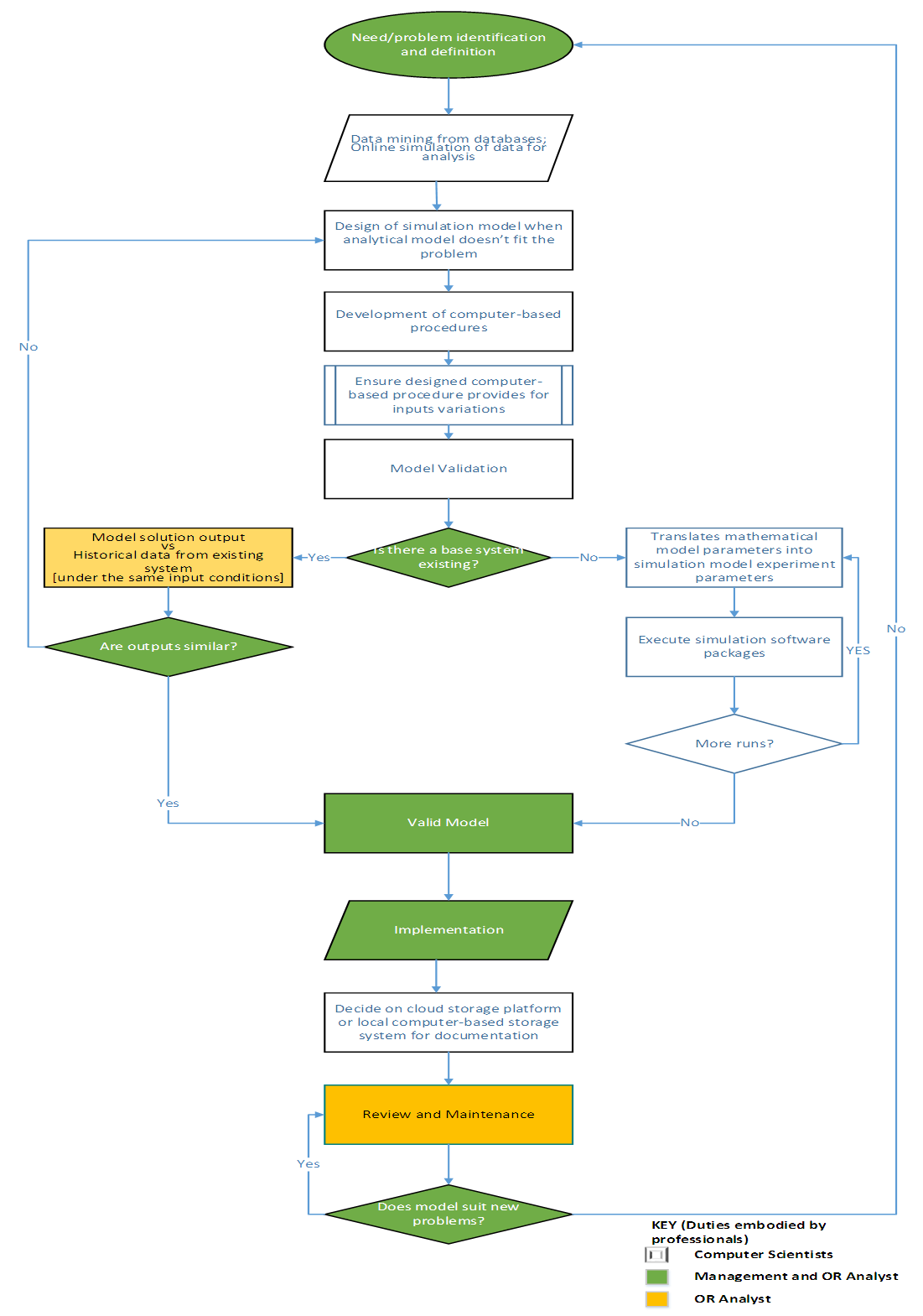 | Figure 1.2. Illustration of key roles occupied by professionals in Operations Research studies |
7. The Way Forward for OR in Nigeria
Operations Research is renowned for its immense contributions to the most developed countries and organizations of the world. Cost savings, profits maximization and process optimization in organizations and in government projects are praiseworthy. However, the Nigerian business environment is yet to fully appreciate the extents to which the use of OR techniques can influence profiteering [2, p. 1]. Of greatest concern is the inattention to the very drivers of substantive development of the discipline – computer science contribution through development of software packages used at various stages of OR studies and the integration of OR into the academic programs of higher institutions of learning as full-time programs as obtainable in other parts of the world. Even though operations research has been tailored into the curriculum of business students in Nigerian Universities [2, p. 1], such a feat only positions the profession in Nigeria at a stationary spot of consuming already made OR techniques and not as a producer of relevant tools/software packages for carrying out activities during OR studies. It is therefore imperative that the following recommendations be given due attention by all stakeholders involved:
8. Recommendations
In a bid to foster sustainable growth in Operations Research in Nigeria, this paper presents the following lead ways for government, organizations, industry experts and OR teams operating within the Nigerian business environment:§ Operations research should not just be included in the syllabus of academic programs in the management sciences, but also to those of Computer Science and Mathematics departments in Universities. This would not only engender the advancement of the profession but also position the country as a producer of new OR techniques and software packages for use in carrying out OR studies in Nigeria and beyond.§ The Nigerian Government has recently been able to foresee the need to achieve effective industrialization through enhancement of the country’s science and technological innovation base through the establishment of two new agencies: The National Agency for Desertification and Erosion Research (NADER) and the National Agency for Research in Robotics and Artificial Intelligence (NARRAI) [17]. The Minister of Science and Technology, Dr Ogbonaya Onu has identified manufacturing, health care delivery and transportation as some of the critical areas that research in Robotics and Artificial Intelligence would assist in fast-tracking economic growth and industrialization in [17].However, in order for the country to fully appreciate the benefits highlighted in the Intelligence Phase of OR, it needs to integrate a special unit for Operations Research and Computational Science Studies within the National Agency for Research in Robotics and Artificial Intelligence (NARRAI). This unit shall carry out such responsibilities as research on implementable models for health care delivery, security logistics control, power sector operations management, etc.This agency should be saddled with the responsibilities of carrying out periodic checks on the mode of operations in various areas of the public sector with a view to optimizing processes where the need arises.§ Institutions of higher learning (especially the Polytechnics and Universities) should remodel existing postgraduate programs in the fields of mathematics and statistics to accommodate more computational sciences. Engineering and Computer Science departments should also consider having specialized courses and programs in the areas of Computational Operations Research in both undergraduate and postgraduate levels. This would be a combined investment in the students and institution towards producing ‘Industry-relevant’ research outputs.§ When Operations Research is taught in schools, more emphasis should be placed on developing new procedures that can fit problems peculiar to the Nigerian business environment. For those in the field of Computer Science and Mathematics, course work in operations research should ensure that the students automate various mathematical procedures involved in the various phases of operations research studies using computer programming languages and tools.§ Nigerian Universities should introduce Operations Research as a standalone program to be offered by students on a full-time basis and consequent accreditation granted to such programs by the National Universities Commission (NUC).§ Operations Research teams should leverage on the available software tools and engage the expertise of Computer Scientists in carrying out such tasks as recognized in fig 1.2.§ IORMS, INFORN and other umbrella bodies should create platforms for undergraduate students in Nigerian Universities from diverse disciplines to interact with industry experts and gain insights into what contributions they could make to the profession [2, p. 12]. This would spur up interest of students to toll the line of Operations Research as a career path. Annual quiz and debate competitions should be organized for students during operations research conferences to create more awareness for students about the profession.§ Managers and business owners should employ the services of Operations Research teams to offer better informed advice on the best possible decisions that could be made in order to maximize profits and save costs.
References
[1] | "Optimization and Operations Research," in Optimization and Operations Research, vol. 1, Cologne, Cologne: Encyclopedia of Life Support Systems (EOLSS), p. 8. |
[2] | O. S. Ighomereho, "Making Use of Operations Research Techniques in Nigerian Business Organizations," IOSR Journal of Business and Management (IOSR-JBM), vol. 7, no. 4, pp. 1-13, January - February 2013. |
[3] | Operational Research Unit, OCB Luxembourg, "Operational Research Definition, Purpose & Procedures (A Policy Framework)," Medecins Sans Frontieres-Brussels, Medical department (Operational research) Brussels Operational Center, Luxembourg, 2010. |
[4] | M. Kulej, Operations Research, J. Mercik, Ed., Wrocław University of Technology, Wrocław: PRINTPAP Łódź, 2011. |
[5] | P. O. Ekoko, Basic Operations Research in Science and Social Science, Benin City: United City Press, 1999. |
[6] | J. W. McGuffee, "Defining Computer Science," ACM SIGCSE Bulletin, pp. 74-76, June 2000. |
[7] | S. F. Hillier and J. G. Lieberman, Introduction to Operations Research, 7th ed., K. Kevin, M. M. Eric and L. Maja, Eds., New York: The McGraw-Hill Companies, Inc., 2001. |
[8] | P. O. Ekoko, "Basic Operations Research in Science and Social Science," Benin City, United City Press, 1999. |
[9] | P. G. Carla, "Artificial Intelligence and Operations Research: Challenges and Opportunities in Planning and Scheduling," Air Force Research Laboratory, New York, 2001. |
[10] | E. B. Donald and C. W. I. Chelsea, Eds., Operations Research and Artificial Intelligence: The Integration of Problem-Solving Strategies, Charlottesville, Virginia: Kluwer Academic Publishers, 1990, pp. 1-4. |
[11] | D. Tara, "How to Conduct Operational Research," Hearst Newspapers, LLC, 2017. [Online]. Available: http://smallbusiness.chron.com/conduct-operational-research-5054.html. [Accessed 16 December 2017]. |
[12] | The Concord Consortium, "Common Online Data Analysis Platform," 2017. [Online]. Available: https://codap.concord.org/. [Accessed 17 December 2017]. |
[13] | R. Groleau, "Wildfire Simulator," 2002. [Online]. Available: http://www.pbs.org/wgbh/nova/fire/simu_text.html. [Accessed 17 December 2017]. |
[14] | E. Onuiri and O. Awodele, General Introduction to Modelling and Simulation, 2013. |
[15] | A. T. Hamdy, Operations Research (An Introduction), 9th ed., vol. 13, S. Holly and R. keri, Eds., New Jersey: Prentice Hall, Pearson Education Inc., 2011, p. 44. |
[16] | A. O. Adebayo, Introductory Operational Research: Notes and Worked Examples on Basic Concepts of Certain Topics, Mic 'N' Dell Ventures, 2015. |
[17] | Federal Ministry of Science and Technology, Federal Republic of Nigeria, "FG to Establish Two New Agencies," Federal Ministry of Science and Technology, Nigeria, Abuja, 2018. |