Subhash Kumar Yadav1, Sat Gupta2, S. S. Mishra3, Alok Kumar Shukla4
1Department of Mathematics and Statistics (A Centre of Excellence on Advanced Computing), Dr. RML Avadh University, Faizabad, U.P., India
2Department of Mathematics and Statistics, University of North Carolina at Greensboro, Greensboro, NC, USA
3Department of Mathematics and Statistics (A Centre of Excellence on Advanced Computing), Dr. R. M. L. Avadh University, Faizabad, U.P., India
4Department of Statistics, D.A-V. College, Kanpur, U.P., India
Correspondence to: S. S. Mishra, Department of Mathematics and Statistics (A Centre of Excellence on Advanced Computing), Dr. R. M. L. Avadh University, Faizabad, U.P., India.
Email: |  |
Copyright © 2016 Scientific & Academic Publishing. All Rights Reserved.
This work is licensed under the Creative Commons Attribution International License (CC BY).
http://creativecommons.org/licenses/by/4.0/

Abstract
In the present article, we have proposed a modified class of ratio and product type estimators of population mean using auxiliary information in two-phase sampling. The expressions for the Bias and Mean Squared Error of the proposed estimators have been obtained up to the first order of approximation. An efficiency comparison has been made with some of the other ratio and product estimators of population mean under two- phase sampling. A numerical study is also carried out to evaluate the performance of proposed and existing ratio and product estimators. It has been shown that the proposed estimators have smaller mean squared errors.
Keywords:
Ratio estimator, Product Estimator, Two-Phase Sampling, Mean Square Error
Cite this paper: Subhash Kumar Yadav, Sat Gupta, S. S. Mishra, Alok Kumar Shukla, Modified Ratio and Product Estimators for Estimating Population Mean in Two-Phase Sampling, American Journal of Operational Research, Vol. 6 No. 3, 2016, pp. 61-68. doi: 10.5923/j.ajor.20160603.02.
1. Introduction
It is well known that to estimate any parameter, a suitable estimator is the corresponding statistic. Thus for estimating population mean, sample mean is the most appropriate estimator. Although it is unbiased, it has a large amount of variation. Therefore we seek an estimator which may be biased but has smaller man squared error as compared to sample mean. This is achieved through the use of an auxiliary variable that has strong positive or negative correlation with the study variable. When there is strong positive correlation between the study variable and the auxiliary variable and the line of regression passes through origin, then the ratio type estimators are used for improved estimation of population mean. Product type estimators are used when there is strong negative correlation. The regression type estimators are used for the improved estimation of population mean when the line of regression does not pass through the origin.Cochran [2] utilized the positively correlated auxiliary variable and for the first time proposed the usual ratio estimator of population mean of the study variable. Later Robson [10] and Murthy [7] proposed the traditional product estimator independently, using negatively correlated auxiliary variable. Srivenkataramana [13] was the first to propose the dual to ratio type estimator for improved estimation of population mean of the study variable. Bahl and Tuteja [1] were the first to propose the exponential type ratio and product estimators of population mean using auxiliary information. In all of the estimators discussed above, the mean
of the auxiliary variable is assumed known. When mean
of auxiliary variable is not known, two-phase or double sampling, suggested by Neyman [9], is used. Kumar and Bahl [6] were the first to propose dual to ratio estimator of population mean in two-phase sampling. Singh and Choudhury [11] proposed the dual to product estimator of population mean in two-phase sampling. Exponential type ratio and product estimators of population mean in two-phase sampling have also been studied by Singh and Vishwakarma [12]. Corresponding dual estimators in two-phase sampling have been studies by Kalita and Singh [4]. Our main motivation in this study is to improve further the estimators by Kalita and Singh [4]. Let the finite population consist of N distinct and identifiable units under study. A random sample of size n is drawn using simple random sampling without replacement (SRSWOR) technique. Let
and
respectively be the population means of the study and the auxiliary variables, and
and
be the respective sample means. When
is not known, double sampling or two-phase sampling is used to estimate the population mean of the study variable y. Under double sampling scheme the following procedure is used for the selection of the required sample:Case I: A large sample
of size
is drawn from the population by SRSWOR and the observations are taken only on the auxiliary variable x to estimate the population mean
of the auxiliary variable.Case II: A sample
of size
is drawn either from
or directly from the population of size
to observe both the study variable and the auxiliary variable. The most suitable estimator for the population mean is the corresponding sample mean given by | (1.1) |
The variance of
up to the first order of approximation, is given by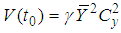 | (1.2) |
where,
Cochran [2] proposed the classical ratio type estimator of population mean utilizing the auxiliary information under simple random sampling as | (1.3) |
Kumar and Bahl [6] proposed the usual ratio estimator of population mean in two- phase sampling as | (1.4) |
where
is an unbiased estimator of population mean
of the auxiliary variable based on the sample
of size
The mean squared error of
up to the first order of approximation, for Case-I and Case-II respectively are,  | (1.5) |
 | (1.6) |
where,
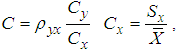
and
Singh and Choudhury [11] proposed the dual to product estimator of population mean in two- phase sampling as | (1.7) |
The mean squared error of
up to the first order of approximation for both the case-I and Case-II respectively are, | (1.8) |
 | (1.9) |
Singh and Vishwakarma [12] proposed the exponential type ratio and product estimators of population mean of study variable in two-phase sampling respectively as,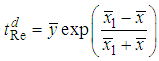 | (1.10) |
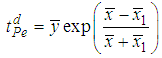 | (1.11) |
The mean squared errors of both the estimators
and
up to the first order of approximation for both the Case-I and Case-II respectively are, | (1.12) |
 | (1.13) |
 | (1.14) |
 | (1.15) |
Kumar and Bahl [6] proposed the following dual to ratio estimator of population mean under two-phase sampling as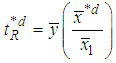 | (1.16) |
The mean squared error of
up to the first order of approximation for Case-I and Case-II respectively are, | (1.17) |
 | (1.18) |
where,
Singh and Choudhury [11] proposed the following dual to product estimator of population mean under two-phase sampling as,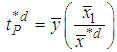 | (1.19) |
The mean squared error of
up to the first order of approximation for Case-I and Case-II respectively are, | (1.20) |
 | (1.21) |
Kalita and Singh [4] proposed the following exponential dual to ratio and exponential dual to product estimator in two-phase sampling respectively as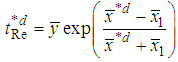 | (1.22) |
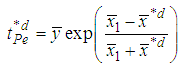 | (1.23) |
The mean squared errors of both the estimators
and
up to the first order of approximation for both the Case-I and Case-II respectively are, | (1.24) |
 | (1.25) |
 | (1.26) |
 | (1.27) |
In the present study, we have proposed the generalized exponential dual to ratio and product-type estimators in double sampling. The large sample properties have been studied up to the first order of approximation.
2. Proposed Estimators
Using the estimators of Kalita and Singh [4], we propose two generalized estimators of population mean as exponential dual to ratio and exponential dual to product-type estimators respectively, as given below: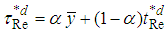 | (2a) |
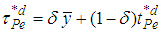 | (2b) |
where,
and
are the characterizing scalars which are obtained by minimizing the mean squared errors of the proposed estimators.The Bias and MSE of the proposed estimators are obtained for the following two cases.Case I: When the second phase sample of size
is a subsample of the first phase sample of size
Case II: When the second phase sample of size
is drawn independently of the first phase sample of size
Case ITo study the large sample properties of the proposed class of estimators, we define
and
such that
and
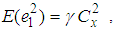
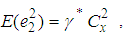
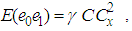

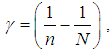
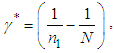
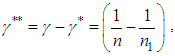
and
The expression for the bias of proposed estimator up to the first order of approximation is,  | (2a.1) |
The mean squared error of proposed estimator up to the first order of approximation is, | (2a.2) |
The optimum value of the characterizing scalar
is obtained by minimizing MSE in (2a.2) using the method of maxima-minima as,  | (2a.3) |
Where
and
The value of the bias of the proposed estimator, for this optimum value of
in (2a.3) is given by,  | (2a.4) |
Minimum value of the MSE of the proposed estimator is obtained by putting the optimum value of
in (2a.7) and thus the minimum MSE is given as, | (2a.5) |
Similarly, the Bias and MSE of proposed product type estimator in equation (2b), the minimum value of bias of the proposed estimator is obtained by putting optimum value of
as, | (2b.1) |
Minimum value of the MSE of the proposed estimator is obtained by putting the optimum value of
and thus the minimum MSE is given as,  | (2b.2) |
Where
and
Case IITo study the large sample properties of the proposed class of estimators, we define
and
such that
and 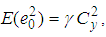


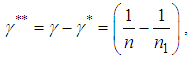
and
Similarly, Bias and MSE of proposed estimators in equation (2a) and (2b), The minimum value of bias and MSE of the proposed estimator is obtained by putting optimum values of characterizing scalars
and
are respectively as, | (2a.11) |
 | (2a.12) |
Where
and
 | (2b.3) |
 | (2b.4) |
where
and 
3. Efficiency Comparison
Case-Ia. Comparison of proposed exponential ratio type estimator with other estimators:From (1.2) and (2a.10), we have
If,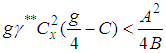 | (3.1) |
From (1.5) and (2a.10), we have
If, 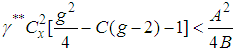 | (3.2) |
From (1.12) and (2a.10), we have
If, 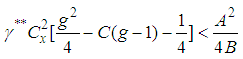 | (3.3) |
From (1.17) and (2a.10), we have
If, 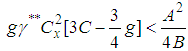 | (3.4) |
From (1.24) and (2a.10), we have 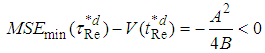 | (3.5) |
b. Comparison of proposed exponential product type estimator with other estimators: From (1.2) and (2b.2), we have
If, 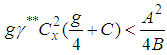 | (3.6) |
From (1.8) and (2b.2), we have
If, 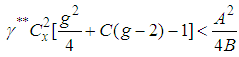 | (3.7) |
From (1.14) and (2b.2), we have
If, 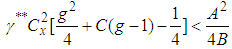 | (3.8) |
From (1.20) and (2b.2), we have
If,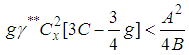 | (3.9) |
From (1.24) and (2a.9), we have 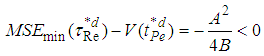 | (3.10) |
Case-IIc. Comparison of proposed exponential ratio type estimatorFrom (1.2) and (2a.12), we have
If, 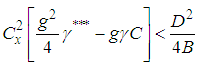 | (3.11) |
From (1.5) and (2a.12), we have
If, 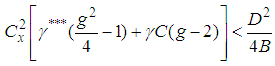 | (3.12) |
From (1.12) and (2a.12), we have
If, 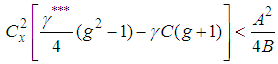 | (3.13) |
From (1.17) and (2a.12), we have
If, 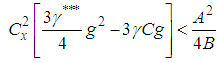 | (3.14) |
From (1.24) and (2a.12), we have 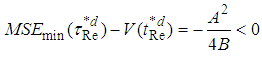 | (3.15) |
d. Comparison of proposed exponential product type estimatorFrom (1.2) and (2b.2), we have
If, 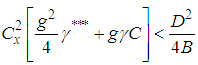 | (3.16) |
From (1.8) and (2b.2), we have
If, 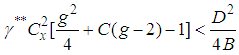 | (3.17) |
From (1.14) and (2b.2), we have
If, 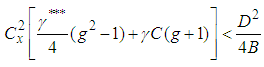 | (3.18) |
From (1.20) and (2b.2), we have
If, 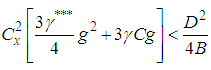 | (3.19) |
From (1.24) and (2a.9), we have 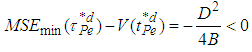 | (3.20) |
4. Numerical Study
To examine the performances of the proposed and existing estimators of population mean in two-phase sampling scheme, we have considered the following four populations: Population I: Source: Murthy [8]

Population II: Source: Kadilar and Cingi [5]
Population III: Source: Johnston [3]
Mean January temperature,
Date of flowering of a particular summer species (number of days from January 1) 
Population IV: Source: Johnston [3]
Percentage of hives affected by disease,
Date of flowering of a particular summer species (number of days from January 1) 
Table-1 and Table-2 below present the mean squared errors of different ratio type and product type estimators respectively for both the cases. Table 1. Mean squared error of different ratio estimators 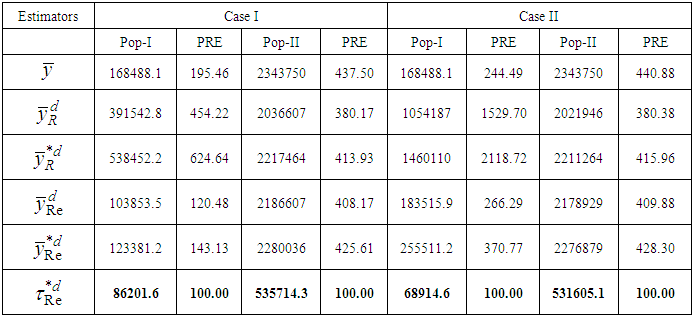 |
| |
|
Table 2. Mean squared error of different product estimators 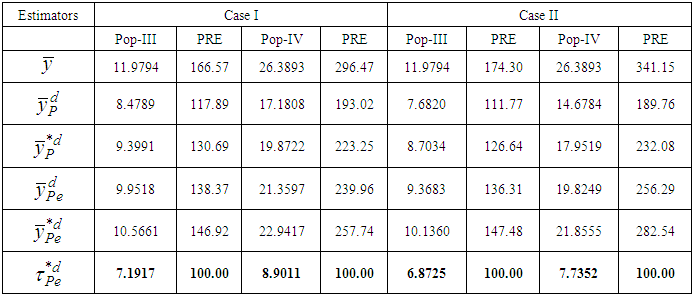 |
| |
|
5. Conclusions
In the present manuscript we have proposed two exponential ratio and exponential product type class of estimators. The biases and the mean squared errors of both the estimators have been obtained up to the first order of approximation. The optimum values of the characterizing scalars which minimize the corresponding mean squared errors have been obtained and corresponding minimum mean squared errors of these estimators have been obtained. The various conditions under which both the estimators perform better than the other ratio and product type estimators under two-phase sampling scheme have been given. A numerical study is also carried out to evaluate the performances of various ratio and product estimators along with the proposed estimators under two-phase sampling using four populations. In the first two populations, the study variable and the auxiliary variable are positively correlated. There is negative correlation in the other two populations. From Table-1 and Table-2, we see that the mean squared error of the proposed estimators
and
respectively are smaller than the other estimators discussed here. Hence the proposed estimators may be preferred over the existing estimators.
Appendix
Using approximations, the proposed estimator may be written as,
Retaining the terms up to the first order of approximation, we have
Subtracting
on both sides of above equation, we have
Taking expectation on both sides in above equation, we have
Putting the values of different expectations, we have bias of the proposed class of estimators as,
Squaring on both sides of (*), simplifying and retaining the terms up to the first order of approximation, we have,
Taking expectations both the sides of above equation, we have the mean squared error of the proposed class of estimators up to the first order of approximation as,
Putting the values of different expectations and simplifying, we have,
References
[1] | Bahl, S., & Tuteja, R. K. (1991). Ratio and Product type exponential estimator, Information and optimization Sciences, 12, 159-163. |
[2] | Cochran, W. G. (1977). Sampling techniques, New-York: John Wiley and Sons |
[3] | Johnston, J. (1972): Econometric methods, (2nd ed.), McGraw-Hill, Tokyo. |
[4] | Kalita, D. and Singh, B. K. (2013). Exponential dual to Ratio and dual to Product-type Estimators for Finite Population Mean in Double Sampling, Elixir Statistics, 59, 15458-15470. |
[5] | Kadilar, C., Cingi, H. (2006). New ratio estimators using correlation coefficient, Interstat, 4, 1–11. |
[6] | Kumar, M., and Bahl, S. (2006). Class of dual to ratio estimators for double sampling, Statistical Papers, 47, 319-326. |
[7] | Murthy, M. N. (1964). Product method of estimation, Sankhya, 26, 69-74. |
[8] | Murthy, M. N. (1967). Sampling theory and methods, Calcutta, India: Statistical Publishing Society. |
[9] | Neyman, J.(1938) Contribution to the theory of sampling human population, Journal of the American Statistical Association, 33, 101-116. |
[10] | Robson, D.S., (1957), Application of Multivariate polykays to the theory of unbiased ratio-type estimation, Journal of the American Statistical Association, 52, 511-522. |
[11] | Singh, B.K., Choudhury. S. (2012). Dual to Product Estimator for Estimating Population Mean in Double Sampling, International Journal of Statistics and Systems, 7,1, 31-39. |
[12] | Singh, H. P. and Vishwakarma, G. K. (2007). Modified exponential ratio and Product estimators for finite population mean in double sampling. Austrian Journal of Statistics, 36, 3, 217-225. |
[13] | Srivenkataramana, T. (1980). A dual to ratio estimator in sample surveys, Biometrika, 67, 1, 199-204. |