Essam El-Seidy , Maan T. Alabdullah , Shahd H. Alkaraz
Department of Mathematics, Faculty of Science, Ain Shams University, Cairo, Egypt
Correspondence to: Shahd H. Alkaraz , Department of Mathematics, Faculty of Science, Ain Shams University, Cairo, Egypt.
Email: |  |
Copyright © 2020 The Author(s). Published by Scientific & Academic Publishing.
This work is licensed under the Creative Commons Attribution International License (CC BY).
http://creativecommons.org/licenses/by/4.0/

Abstract
Initially, we provide basic knowledge of definitions and concepts related to the concept of matching in the graph. We are studying a model of games based on two players who take turns adding edges to
this process eventually produces a maximal matching of the graph. We call the first Maximizer and second player Minimizer. The first aims to get a final matching to be large while the second one wants to reduce it. Maximizer wins if he manages a maximal matching while Minimizer wins if he can prevent him from doing this. The matcher number
is the number of edges chosen when both players play optimally, while the matching number
is the number of maximum matching edges. In this research we study the relationship between
and
. And we also prove some results on types of graph.
Keywords:
Maximal matching, Maximum matching, Matching, Matcher game
Cite this paper: Essam El-Seidy , Maan T. Alabdullah , Shahd H. Alkaraz , When Sciences Overlaps: A Matching Game under a Graph Concept, American Journal of Mathematics and Statistics, Vol. 10 No. 2, 2020, pp. 55-61. doi: 10.5923/j.ajms.20201002.04.
1. Introduction
As the title suggests, this research is about games on graphs. At first sight, the topic may suggest lots of fun since games are widely recognized as amusing. But the reader should keep in mind that this is a collection of mathematical results and as such has a moderate fun impact.Matching in graphs has been an important topic and has a lot of applications for solving problems. It is used to solve Important problems. This can be generalized to solve any problem that can represented as a graph, we will discuss the general matching idea and Matching in graphs.The matcher game is related to the growing family of competition parameters or competitive optimization games on graphs and hypergraphs. For example, Phillips and Slater introduced a game where two players alternate adding vertices of
to an independent set until it becomes a maximal independent set. One player wants the final set to be large; the other wants it to be small. More recently, Cranston et al. [1] introduced a game where the players alternate adding edges of
to a matching until it becomes a maximal matching. Theirs is equivalent to the game of played on the line graph and is a very different game to ours. Probably the best-known such parameter is the game chromatic number, which was introduced by Brams for planar graphs (cf. [2]) and independently by Bodlaender [3] for general graphs, and for list colorings by Borowiecki et al. [4]. There is also work on domination [5,6], transversals in hypergraphs [7,8] and more.We denote the matching number of graphs
by
and define the lower matching number
as the minimum cardinality of a maximal matching of
.The main goal is to study the values of the game that fall between
and
. where we have two cases either Minimizer starts and the two values are equal and therefore the matching number is not interesting so our focus in this research is on the Max-start matcher number which we simply call the matcher number. There we show that, while for bipartite graphs the matcher number equals the matching number, in general
, and we characterize the graphs
for which
. We also investigate the relationship with the lower matching number, showing that
. We conclude with some sufficient conditions for
to be half the order.
1.1. Introduction of the Matching Game
In this research we introduce the matching game. This is played on undirected graph
by two players, called Maximizer and Minimizer, who take turns in constructing a matching of
. Each round, one player chooses a vertex
with at least one neighbor not previously chosen, and the other player chooses a vertex
not previously chosen that is a neighbor of
. This process continues until no more play is possible; that is, the edges
form a maximal matching of
. Maximizer wishes to maximize the number of edges in this matching, while Minimizer wishes to minimize it. The Max-start matcher number
of
is the number of edges in the matching when Maximizer starts and both players play optimally, while the Min-start matcher number
of
is the number of edges chosen when Minimizer starts and both players play optimally.For notation and graph-theory terminology not defined herein, we in general follow [9]. We denote the degree of a vertexin a graph
by
. The minimum degree among the vertices of
is denoted by
.The following are some important concepts:• A matching M: is a set of edges in
no two of which are adjacent.• A perfect matching M: if every vertex of
is incident to an edge of
.• Maximal matching: if no other edges of
can be added to
.• Maximum matching: are largest maximal matching.• Matching number
: is number of edges in the maximum matching of
.• Lower matching number
: is the minimum cardinality of a maximal matching of
.• If
is a matching in
, then a vertex is
-matched or covered by
if it is incident with an edge of
; otherwise, the vertex is
-unmatched.• A path or cycle is
-alternating of its edges are alternately in and not in
.• Max start matcher number
: is the number of edges in the matching when Maximizer starts and both players play optimally• Min start matcher number
: is the number of edges chosen when Minimizer starts and both players play optimally.The following figure shows the difference between Maximal matching and Maximum matching.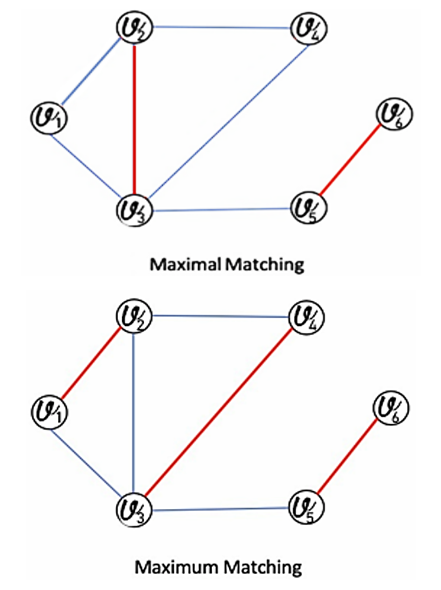 | Figure 1. The difference between Maximal matching and Maximum matching |
2. The Minimizer Starts the Game
We start by showing that the Min-start matcher number of a graph is precisely the matching number of the graph. We shall need the following trivial well-known preliminary lemma. Lemma 1. For every graph
, every non-isolated vertex is incident with an edge that belongs to some maximum matching of
[10]. In the following graph we note that every vertex is incident with an edge belong to a maximum matching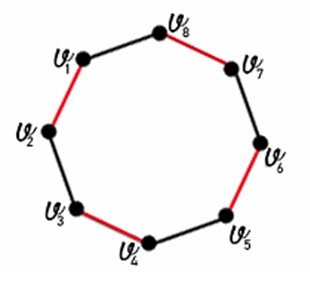 | Figure 2. Example of Lemma 1 |
Theorem 1.If
is a graph, then have
.Proof.We show that Maximizer has a strategy that guarantees that the Min-start matcher game always finishes with a maximum matching, implying that
. Suppose that Minimizer chooses vertex
By Lemma 1, the vertex
is incident with an edge, say
that belongs to some maximum matching,
say, In
. So, Maximizer chooses vertex
. Then let
. Note that
, while by induction we have
, then
and from it
and then
.
3. The Maximizer Starts the Game
For the remainder of the paper we study the Max-start matcher game. Here is an example:Lemma 2.For the path
with
it holds that
. For the cycle
with
it holds that
[10].For the cycle
and
, we find
and
as shown in Figure 3.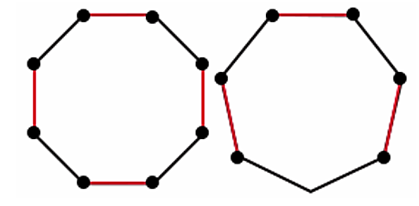 | Figure 3. Max start matcher number for the cycle  |
The above case is an example where the matcher number equals the matching number. For an example where the matcher and matching numbers are different is the following:Lemma 3. If
is a graph with minimum degree at least two that contains a unique maximum matching, then
.Proof.Let
be the unique maximum matching in
. By Lemma 1, every vertex is incident with
; that is,
is a perfect matching. Let
be the first vertex chosen by Maximizer. Since
has degree at least two, Minimizer can respond by choosing a vertex
such that
is not in
; thus, the maximal matching that is constructed will not be
. It follows that
.Definition: We define a vertex of a graph
as liberal if it is not isolated and every edge incident with
belongs to some maximum matching in
.An example in the following figure we call the vertexes
and
liberal vertex, while the vertexes
and
are not.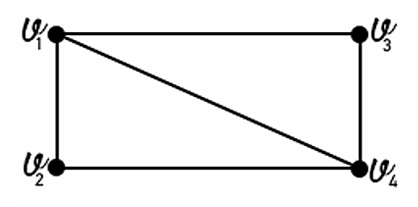 | Figure 4. Graph that contains liberal vertexes |
The usefulness of the idea can be expressed in the following observation. Recall that a family of graphs is hereditary if it is closed under vertex removal.Lemma 4.Let
be a hereditary graph family such that every nonempty graph in
has a liberal vertex. Then for all
it holds that
.Proof. The proof is by induction. If
has no edges, then
. If
is nonempty, then by assumption there is a liberal vertex in
, say
. Maximizer chooses this as his first move. Let
be the response of Minimizer and let 
. Note that
while by induction we have
since
, then
and from it
and then
.Note from Figure 4 for liberal vertex
we have
.For example, it is immediate that any end-vertex of a graph is liberal; thus trees/forests are examples of the above situation. We show next that isolate-free graphs without perfect matchings always have a liberal vertex (see [11]). Lemma 5.If
is a non-isolated vertex and there exists a maximum matching
where
is
-unmatched, then
is liberal.Proof. As in the proof of Lemma 1, every neighbor
of
is incident with an edge of
, say
, and
is a maximum matching in
containing
. That is,
is liberal.
3.1. Bipartite Graphs
We conclude below some properties to see the relation between
and
.Lemma 6. If
is an isolate-free bipartite graph, then each partite set contains a liberal vertex.Proof.Color red all edges of
that belong to no maximum matching. Let
be a fixed maximum matching of
, and color blue all edges of
. Thus, each vertex of
is incident with at most one blue edge.Let us say a path is happy if the edges alternate colors. Let
be a longest happy path in
. As in the proof of Lemma 5, an
-un matched vertex is not incident with any red edge. So if the first edge of
is red, vertex
must be incident with a blue edge; by the maximality of
, this blue edge must join
to a vertex of
; indeed, this blue edge must join
to
, since all other vertices of
are already incident with a blue edge. But then, if we take
and remove the (blue) edges of
and add the red edges of
, we get another maximum matching, contradicting the claim that the edges are red. It follows that both the first and last edge of the happy path
are colored blue. In particular this implies that
is even.Suppose that
is not liberal. That is, it is incident with at least one red edge. It follows by the maximality of
that
is a red edge for some
. Since
is bipartite, the cycle
is an even cycle whose edges alternate between blue and red edges. Replacing the (blue) edges of
that belong to
with the (red) edges of
not in
and leaving all other edges of
unchanged, produces a new maximum matching of
that contains some red edges, contradicting the definition of a red edge [10].And by this we have proven the vertex
is liberal and in the same way we prove that
is liberal, then every partite sets have liberal vertex.Theorem 2. If
is a bipartite graph, then
.Proof.It is produced from Lemmas 4 and 6 [10].An example of this, in the following figure we have 
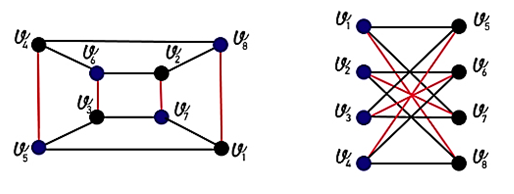 | Figure 5. Example of bipartite graph has  |
Theorem 3.If
is a complete multipartite graph, then
.Proof. Assume
is a complete multipartite graph with partite sets
where
. By Lemma 4 it is sufficient to show that if
is nonempty then it contains a liberal vertex. By Lemma 5 we may assume that
has a perfect matching, say
. We claim that every vertex
is liberal. For, suppose there is an edge
incident with
that is not in any perfect matching; say
. By symmetry, no edge between
and
is in a perfect matching. Since
is perfect, it contains edges incident with
and
; say
and
. If
and
are adjacent, then
is a perfect matching that contains the edge
, a contradiction. So, assume that
and
are in the same partite set, say
(as observed earlier,
and
).Since
there is a vertex,
say, in
that is not
-matched to a vertex of
. Hence, the vertex
is incident with an edge of
, say
, where
. But then
is a perfect matching that contains the edge
a contradiction. Thus, every vertex of
is liberal [10].An example of this, in the following figure the complete multipartite graph
we have 
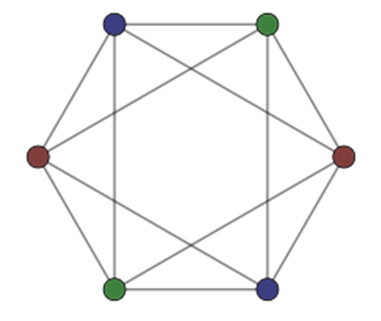 | Figure 6. Complete multipartite graph  |
4. Lower Bounds
We next consider lower bounds for the matcher game, especially in relation to the matching and lower matching numbers. We will need the following lemma.Lemma 7.If
is a graph and
is an independent set of vertices such that there is a matching that covers
, then
.Proof. Let
be the bipartite subgraph of
with vertex set
and edge-set the edges incident with
. By Lemma 6 there is a vertex
of
that is liberal in
. Maximizer chooses this vertex. Say Minimizer chooses
in response. Since
is liberal in
, the edge
is in a maximum matching
of
. The matching
covers
in
. Thus the result follows by induction. For example, the above lemma shows that if a graph
has a perfect matching, then
, here
is the independence number of
. We next use the above lemma to provide a lower bound on the matcher number in terms of the lower matching number.Lemma 8. If
is a graph, then
.Proof.Consider a maximum matching
and a minimum maximal matching
. Then the vertices of
form an independent set. Further, at most
of them are not covered by
, where
is the order of
. That is, there is a subset of
of at least
vertices, all of whose vertices are
-matched. By Lemma 7, it follows that
.For example, if the lower matching number is half the matching number, then the matcher and matching numbers are equal.As another consequence of Lemma 8 and the earlier trivial observation that
, we obtain the following lower bound:Theorem 4.If
is a graph then
.Proof. 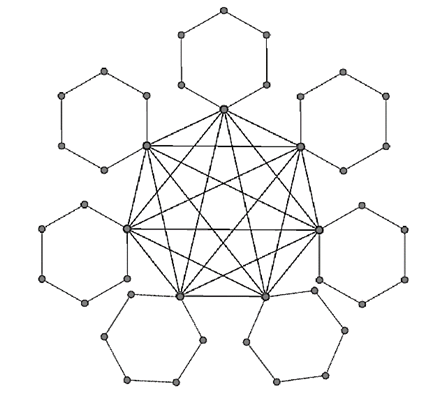 | Figure 7. Depiction of  |
As long as an edge remains, Max plays an edge belonging to a maximum matching; this reduces the matching number by 1. When
, the edge played by Min in response is incident to at most two edges of a maximum matching and hence reduces the matching number (of the residual graph) by at most 2. Hence a round reduces
by at most 3 while adding2 to the number of edges played. When
and Max starts, two more edges will be played.For graph
and integer
, define the generalized corona
as follows. For each vertex
of
introduce a disjoint k-cycle and identify one vertex of the cycle with
; we call the vertex
the root of the cycle. For example, here is a depiction of
.Lemma 9. If graph
has order
and
is odd, then
.Proof. In each copy of
color red both edges incident with the root and then every alternate edge. Note that every root is incident with exactly two red edges and every other vertex is incident with exactly one red edge. The strategy for Minimizer is to prefer red edges. That is, if possible, she chooses a neighbor connected by a red edge to Maximizer’s choice, otherwise she chooses any neighbor.We claim that Minimizer’s strategy means that every root will be matched to a vertex inside its copy of
Focus on some copy
, and let
be the set consisting of the root and its two neighbors in
. The strategy for Minimizer means that if Maximizer chooses a vertex in
, then so does Minimizer. Thus, Maximizer must be the first to choose a vertex in
; and when he does so, Minimizer chooses its neighbor in
. Thus the root is matched within
.It follows that at the end of the game, at least one vertex from each copy of
is unmatched. That is, the maximal matching obtained has size at most
.It follows that
is an example of equality in Theorem 4 whenever
has a perfect matching.
4.1. Odd Girth
We now consider a generalization of Theorem 4. Recall that the odd-girth of a nonbipartite graph
is the shortest length of an odd cycle in
.Theorem 5. If graph
has odd girth g, then
.Proof. The proof is by induction on the order. If there is an isolated vertex, then we can just discard it and induct; so, assume that
is isolated-free. If there is a liberal vertex
in
, then Maximizer chooses that vertex and we induct after Minimizer’s response. So, we may assume there is no liberal vertex. In particular, by Lemma 5, the graph has a perfect matching, say
.As usual we color red each edge that is in no perfect matching.Say Maximizer starts with vertex
and Minimizer responds with vertex
. If the edge
is in some perfect matching, then again, we can just induct on
. So we may assume that the edge
is red. Let
be the path
. Now the initial strategy of Maximizer will be to do the following as long as it is possible:choose the
-partner of one end of the current path,where by
-partner of vertex
we mean the vertex
such that
is in
. So, we obtain a series of
-alternating paths
such that each
has
vertices and starts and finishes with an edge not in M.If such a choice were always valid, then Maximizer would match off the entire graph, a contradiction of the fact that
is red. So, Maximizer becomes stuck somewhere; that is, he cannot choose the
-partner of either end of the current path since neither has an unchosen neighbor. Say we have path
with ends
and
. Let
be the
-partner of
and
the
-partner of
. Since Maximizer is stuck, all neighbors of
and
are on
.Suppose that
. By assumption, vertex
is not liberal and so is incident to a red edge, say
. If the edge
creates an even cycle with the path
, then we have a contradiction, since every edge in such an
-alternating cycle is in a perfect matching. So, the edge
must create an odd cycle with
. Similarly,
is joined by a red edge to some vertex
on
. that creates an odd cycle. By the odd-girth condition,
is closer to
than
is along the path
. See figure.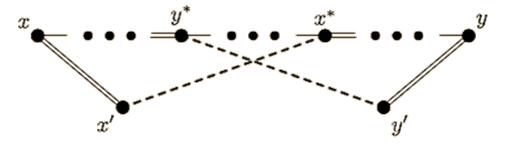 | Figure 8. -alternating cycle formed by  |
But then there is an
-alternating cycle formed by
. It follows that the edge
is in a perfect matching, a contradiction of the claim that it is red.That is, it must be the case that
. Then the remaining graph
has a perfect matching, and we can apply induction to
. The final matching is the union of a matching that has size
from
and a matching that has size at least
of
. The lower bound follows since 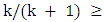
Note that for
odd, the generalized corona
has a perfect matching if and only if the graph
has a perfect matching. By Lemma 9, it follows that
is an example of equality in Theorem 5 for all odd
and graphs
with a perfect matching and girth at least
.Using the ideas in the above proof, one can characterize the graphs that achieve equality in Theorem 4.Theorem 6. If
is an isolate-free graph, then
if and only if it is isomorphic to
for some graph with a perfect matching.Proof. Suppose that
. Consider the proof of Theorem 5 for the case that
. To obtain equality, it is necessary that every vertex is incident with a red edge and that there is a perfect matching
. Further, in applying hisstrategy, Maximizer must get stuck with
. So we have an alternating path
with 4 vertices. Let B denote the subgraph induced by
. See figure.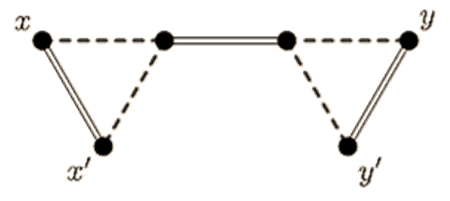 | Figure 9. Alternating path with 4 vertices |
Note that vertices
and
have degree 2. Since Maximizer can start with any vertex, it follows that every vertex has a neighbor of degree 2. In particular, the degree-2 vertices come in pairs.For equality, one needs that the graph
is also an example of equality. By induction, the graph
is isomorphic to
where
is a graph with a perfect matching. Since the degree-2 vertices form a paired dominating set, it follows that in
the only edges between B and
if any, are between the vertices that already have degree at least 3 in that subgraph. Thus, the original graph
has the desired structure. In general, it seems likely that the only graphs that achieve equality in Theorem 5 are the ones already noted; that is,
for
odd and
a graph with a perfect matching and girth at least
. As in the proof of Theorem 6, one can readily show that graphs with equality in Theorem 5 have
as a spanning subgraph, and by the odd-girth condition, the copies of
are induced. What seems harder to resolve is the absence or existence of other edges between the cycles.
5. Dense Graphs
We consider some dense graphs and show that their matcher number is half their order. It is well-known that if the minimum degree is at least half the order, and the order is even, then the graph has a perfect matching. (For example, this follows from Dirac’s theorem [12].)It follows from Theorem 3 that graphs of even order with minimum degree at least
have matcher number
.(Since such minimum degree implies the graph is complete multipartite.) Here is a tiny improvement on this.Lemma 10. If graph
has even order
and minimum degree
, then
.Proof. The strategy for Maximizer is simply to choose any vertex of minimum degree.Consider the situation when four vertices remain. The current graph, say
, has minimum degree at least 1. Suppose it does not have a perfect matching. Then
is (isomorphic to) the star with three edges. Let
denote the set of the three end-vertices in
. Now, go back to the graph, say
, with six vertices remaining. That graph
has minimum degree at least 3. So all three vertices of
are adjacent in
to the two vertices matched in
; this means that in
each vertex in
has degree 3 while the vertices matched each have degree at least 4. This is a contradiction of Maximizer’s strategy.Thus, it follows that
has a perfect matching. It is easily checked that the strategy of choosing a vertex of minimum degree ensures that the resultant maximal matching is perfect.One can improve the above lemma slightly. Computer search shows that all graphs of order 8 and minimum degree at least 4 have matcher number 4. It follows that if graph
has even order
and minimum degree at least
, then the matcher number is
. (Maximizer plays arbitrarily until eight vertices remain, and then uses the optimal strategy.)Lemma 11. If two graphs have the same order m, then the matcher number of their join is
Proof. The vertex set of the join can be partitioned into sets
and
of size m such that every edge between
and
is present. If both
and
are independent sets, then the graph is
and we are done. So, assume there is some edge
with both ends in
.Then Maximizer starts by choosing any vertex in
. If Minimizer takes a vertex of
, then the result follows by induction (as the remaining graph is the join of two graphs of order
). So, assume that she chooses a vertex of
.If only two vertices of
remain, then we are done, since they are joined by edge
and every other vertex has been matched. So, assume more than two vertices remain in
. Then Maximizer chooses a vertex in
but avoiding both ends of
. If Minimizer chooses a vertex in
, we are back to having equal number of vertices in the two sets, and so can induct.So, assume that she chooses a vertex of
. But then Maximizer chooses another vertex in
, avoiding the ends of e, and the argument repeats.
6. Conclusions
We provided bounds on the matcher games. It seems that the Max-start matcher game might be interesting for special families of graphs. For example, rooks' graphs or cartesian products in general seem worthy of consideration, as do cubic graphs or chordal graphs. In another direction, a natural question is the complexity of the game.
References
[1] | D.W. Cranston, W.B. Kinnersley, S. O, D.B. West, Game matching number of graphs, Discrete Appl. Math. 161 (2013) 1828–1836. |
[2] | M. Gardner, Mathematical games, Sci. Am. 244 (1981) 18–26. |
[3] | H.L. Bodlaender, On the complexity of some coloring games, Internat. J. Found. Comput. Sci. 2 (1991) 133–147. |
[4] | M. Borowiecki, E. Sidorowicz, Zs. Tuza, Game list colouring of graphs, Electron. J. Combin. 14 (2007) #R26 11 pp. |
[5] | B. Brešar, S. Klavžar, D.F. Rall, Domination game and an imagination strategy, SIAM J. Discrete Math. 24 (2010) 979–991. |
[6] | M.A. Henning, S. Klavžar, D.F. Rall, The 4/5 upper bound on the game total domination number, Combinatorica 37 (2017) 223–251. |
[7] | Cs. Bujtás, M.A. Henning, Z. Tuza, Transversal game on hypergraphs and the 34-conjecture on the total domination game, SIAM J. Discrete Math. 30(2016) 1830–1847. |
[8] | C. Bujtás, M.A. Henning, Z. Tuza, Bounds on the game transversal number in hypergraphs, European J. Combin. 59 (2017) 34–50. |
[9] | M.A. Henning, A. Yeo, Total Domination in Graphs, in: Springer Monographs in Mathematics, Springer, ISBN: 978-1-4614-6524-9, 2013. |
[10] | W. Goddard, M.A. Henning, The matcher game played in graphs, Discrete Applied Mathematics, 237 (2018) 82-88. |
[11] | M.D. Plummer, Extending matchings in graphs: a survey, Discrete Math. 127 (1994) 277–292. |
[12] | G.A. Dirac, Some theorems on abstract graphs, Proc. Lond. Math. Soc. (3) 2 (1952) 69–81. |