Ajay Kumar Chaudhary1, 2, Kanhaiya Jha1
1Deparmentof Mathematics, School of Science, Kathmandu University, Dhulikhel, Kavre, Nepal
2Department of Mathematics, Tri-Chandra Multiple Campus, Tribhuvan University, Kathmandu, Nepal
Correspondence to: Ajay Kumar Chaudhary, Deparmentof Mathematics, School of Science, Kathmandu University, Dhulikhel, Kavre, Nepal.
Email: |  |
Copyright © 2019 The Author(s). Published by Scientific & Academic Publishing.
This work is licensed under the Creative Commons Attribution International License (CC BY).
http://creativecommons.org/licenses/by/4.0/

Abstract
The classical Banach contraction principle in metric space is one of the fundamental results in metric space with wide applications. And the probabilistic metric space is one of the important generalizations of metric space introduced by Austrian mathematician Karl Menger in 1942. The purpose of this article is to describe different contraction conditions in Probabilistic Metric Space. Also, mention the generalized contraction conditions and interrelationships between contraction conditions.
Keywords:
Fixed Point, t-norm, Probabilistic metric space, Contraction condition
Cite this paper: Ajay Kumar Chaudhary, Kanhaiya Jha, Contraction Conditions in Probabilistic Metric Space, American Journal of Mathematics and Statistics, Vol. 9 No. 5, 2019, pp. 199-202. doi: 10.5923/j.ajms.20190905.03.
1. Introduction and Preliminaries
In mathematics, analysis plays an important role in the development of mathematics. Among several branches of analysis, functional analysis which deals with the study of several functions, come under Functional Analysis. It describes two types of functional analysis one is linear and another is non-linear functional analysis. Fixed point theory is one of the most important topics of non-linear functional analysis since 1960. It has wide applications to the numerous fields of mathematics as well as outside mathematics such as differential equations, integral equations, variational problems, optimization problems, game theory, graph theory, image and signal processing, economics, and many more.The notion of distance later known as metric space, introduced by M. Frechet in 1906, furnishes the common idealization of a large number of mathematical, physical and other scientific constructs in which the distance of a 'distance' appears. The objects under consideration may be most varied. They may be points, functions, sets, and even the subjective experiences of sensation. What matters is the possibility of associating a non-negative real number with each ordered pair of elements of a certain set, and that the number associated with pairs and triples of such elements satisfy certain conditions. However, in numerous instances in which the theory of metric spaces is applied, this very association of a single number with a pair of elements is, realistically speaking, an over idealization. This is so even in the measurement of an ordinary length, where the number given as the distance between two points is often not the result of a single measurement, but the average of a series of measurements. Indeed, in this and many similar situations, it is appropriate to look upon the distance concept as a statistical rather a determinate one. More precisely, instead of associating a number - the distance
- with every pair of elements
, one should associate a distribution function
any for any positive number
, interpret
as the probability that the distance from
to
less than
When this is done one obtains a generalization of the concept of metric space - a generalization which was first introduced by Austrian Mathematicians Karl Menger in 1942 and following him, is called a statistical metric space [8]. In this paper, we analyze the different contraction conditions in probabilistic metric space and their inter-relationships. Definition 1.1: Metric space is a pair
, where
is a non-empty set and
is a distance function or metric of the space defined by
, satisfies the following conditions:
Example 1.1: Let
be a non-empty set. For
we define
Then,
is discrete metric and the space
is discrete metric space.Definition 1.2: Let
be a map. Then, an element
is said to be fixed point of
if
Example 1.2: Let
, cubic equation.Then, it can be transferred to as
Here,
So, by definition
are fixed points of
.Definition 1.3: Let
be a metric space and let
be a mapping. Then,
is called contraction if there exists a fixed constant
such that
Example 1.3: Let
be defined by,
Then,
for all
. So,
is a contraction on
. But
is not continuous and thus not a contraction map.Definition 1.4: For the set
of real numbers, a function
is called a distribution function if(i)
is non-decreasing,(ii)
is left continuous, and(iii)
and
If
is a non-empty set,
is called probabilistic distance on
and
is usually denoted by
. We will denote by
the family of all distribution function on
and
on
Example 1.4: Let
is a maximal element for
then, distribution function
is defined by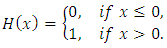
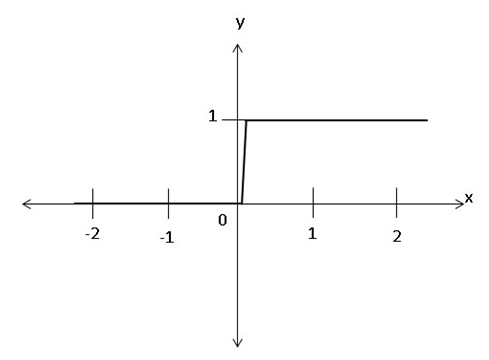 | Figure 1. Distribution Function |
Definition 1.5: [13] A probabilistic metric space (brief, PM-space) is an order pair
where
is a non-empty set and
is a function defined by 
(the set of all distribution functions) that is
associates a distribution function
with every pair
of points in
. The distribution function
is denoted by
whence the symbol
will represent the value of
at
And the function
are assumed to satisfy following conditions:(i)
; (ii)
, (iii)
for every
(iv) For every
and for every
The interpretation of
as the probability that the distance from
to
is less than
it is clear that PM condition (iii), (i) and (ii) are straight forward generalizations of the corresponding metric space conditions (i), (ii) and (iii). The PM condition (iv) is a 'minimal' generalization of the triangle inequality of metric space condition (iv). If it is certain that the distance of
and
is less than
and like wise certain that the distance of
and
is less than
, then it is certain that the distance of
and
is less than
The PM condition (iv) is always satisfied in metric spaces, where it reduces to the ordinary triangle inequality.Definition 1.6: [6] A mapping
is called a triangular norm (shortly t-norm) if for all
the following conditions are satisfied:
Example 1.5 of t-norms
The four basic standard t-norms are:(i) The minimum t-norm,
, is defined by
(ii) The product t-norm,
is defined by 
(iii) The Lukasiewicz t-norm,
, is defined by
(iv) The weakest t-norm, the drastic product,
, is defined by
With references to the point wise ordering, we have the following inequalities
Definition 1.7: [8] A Menger probabilistic metric space (briefly, Menger PM-space) is a triple
where
is a probabilistic metric space,
is a triangular norm and also satisfies the following conditions, for all
and 
. This is the extension of triangle inequality. This inequality is called Menger's triangle inequality.Example 1.6: Let 
and
where
then
is Menger Space.Definition 1.8: [4] Let
be a Menger Space and
be a continuous t-norm (1) A sequence
in
is said to be converge to a point
in
(written
) iff for every
and
there exists an integer
such that
for all
(2) A sequence
in
is called a Cauchy if for every
and
, there exists an integer
such that
for all
(3) A Menger space in which every Cauchy sequence is convergent is said to be Complete Menger Space.Banach Contraction Condition in Metric Space: The most basic fixed-point theorem is analysis known as the Banach Contraction Principle (BCP). It is due to S. Banach [1] and appeared in his Ph.D. thesis (1920, published in 1922). The BCP was first stated and proved by Banach for the Contraction maps in setting of complete normed linear spaces. At about the same time the concept of an abstract metric space was introduced by Hausdorff for the set valued mappings, which then provided the general framework for the principle for contraction mappings in a complete metric space. The BCP can be applied to mappings which are differentiable, or more generally, Lipschitz continuous.Theorem 1.1: Let
be a complete metric space, then each contraction map
has a unique fixed point.Example 1.7:
Obviously
is a Banach contraction and
where
denotes the fixed point of the mapping
.
2. Contraction Conditions in Probabilistic Metric Space
2.1. V.M. Seghal and A.T. Bharucha-Reid (B) Contraction Conditions in PM Space
The following definition of a contraction mapping was suggested and studied by V.M. Seghal and A.T. Bharucha-Reid in 1972, which is very natural probabilistic version of the notion of Banach contraction in metric space. Definition 2.1.1: [12] The following definition of a contraction mapping was suggested and studied by V.M. Seghal and A.T. Bharucha-Reid in 1972, which is very natural probabilistic version of the notion of Banach contraction in metric space.Let
be a probabilistic metric space. A mapping
is a contraction mapping (or a SB - Contraction mapping or B-contraction) on
if and only if there is a
such that | (2.1) |
where
and
It is also known as probabilistic k-contraction.The geometrical interpretation expression (2.1) is that the probability that the distance between the image points
being less than
is at least equal to the probability that the distance between
that is less than
Dentition 2.1.2: [2] Let
be a probabilistic metric space. A mapping
is a probabilistic q-contraction
if | (2.2) |
for every
and every
It is obvious that
is a probabilistic q-contraction if and only if for every
and every
the following implication holds | (2.3) |
The inequality (2.2) is a generalization of inequality.
where
and
is a metric space. In order to prove that (2.3) implies (2.2) recall that every metric space
is also a Menger space
, if
is defined in the following way: | (2.4) |
Suppose that
is such that (2.3) holds and prove that (2.2) is satisfied i.e.,that for every
we have
If
then
and (2.3) implies
which means that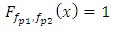
2.2. Hick’s Contraction (C) in PM Space
Definition 2.2.1: [7] T.L. Hicks in 1996, defined the following C-contraction mapping in PM space.Let
be a probabilistic metric space and
. The mapping
is called Hicks C-contraction (or, C-contraction) if there exists
such that the following implication holds for every
: and for every 
Definition 2.2.2: [9] D.Mihet in 2005, introduced the weak- hicks contraction in PM Space as follows:Let
be a nonempty set and
be a probabilistic distance on
. A mapping
is said to be weak - Hicks contraction (w-H contraction) if there exists
such that, for all 

Example 2.2.1: Let
and
It is known ([10], [11]) that
is a complete Menger space under the triangular norm
. Also, it can easily be seen that the mapping
,
is a w-H contraction for every 
2.3. Generalization of Bharucha (B)-Contraction
As a generalization of the notion of a probabilistic B-contraction, we shall introduce the notion of a probabilistic (m,k) - B-contraction where
and
Definition 2.3.1: [6] If
is a PM - space,
and
, a function
is called probabilistic (m,k)-B-contraction if for any
there is an
with
such that for every 
If
and
then a probabilistic 
is a probabilistic B-contraction.As a generalization of C-contraction, we haveDefinition 2.3.2: [6] If
is a PM - space,
and
, a function
is called a (m,k)-C-contraction if for any
there is an
with
such that for every 
If
and
then a probabilistic
is a probabilistic C-contraction.
2.4. Probabilistic G-contraction Mapping
Definition 2.5.1: [5] g-contraction mapping is the generalization of Hick’s C-contraction in Probabilistic Metric Space. Let
be two mappings defined on a Menger space
with values into itself and let us suppose that
is bijective. The mapping
is called a probabilistic g-contraction with a constant
if
and
impies 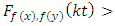
The notion of g-contraction is justified because the images of two points
under the function
are nearer than images of the same points under the function 
3. Conclusions [3]
The Probabilistic g-contraction is Hicks C-contraction when g = I, an identity mapping. Since H-contraction need not be B-contraction. So, Probabilistic g-contraction need not be B-contraction. Moreover, C-contraction is an extension of Banach contraction in Probabilistic Metric Space.It is clear that(i) (m-k) contraction
C-contraction
B-contraction
Banach contraction(ii) g-contraction
C-contraction
B-contraction(iii) C-contraction
(w-H) contraction
References
[1] | Banach S (1922) Sur les operations dans les ensembles abstraits et leur applications aux equations integral. Fund. Math. 3, 133-181. |
[2] | Carrisi J (1976) Fixed Point theorem for mapping satisfying inwardness conditions. Trans. Amer Math. Soc. 215, 241-251. |
[3] | Chaudhary AK (2018) A Study on Contraction conditions in Probabilistic Metric Space. MPhil Dissertation, Kathmandu University, School of Science, Dhulikhel, Kavre, Nepal. |
[4] | Chauhan S and Pant BD (2011) A Fixed Point Theorem in Menger Space, Kathmandu University Journal of Science. Engineering and Technology, 7, 9-17. |
[5] | Golet I (2004) On Contractions in Probabilistic Metric Space, Radovi Mathematic Ki., 13, 87-92. |
[6] | Hadzic O and. Pap E (2010) Probabilistic Fixed Point Theory in Probabilistic Metric Space. Kluwer Academic Publisher, London. 536. |
[7] | Hicks TL (1996) Fixed Point Theory in Probabilistic Metric Space ii. Math Japonica, 44, 487-493. |
[8] | Menger K (1942) Statistical Matrices, Proceedings of National Academy of Sciences of USA, 28, 535-537. |
[9] | Mihet D (2005) Weak Hicks Contractions, Fixed Point Theory 6(1)71-78. |
[10] | Radu V (1985) On Some Fixed-Point theorems in PM space, Lecture Notes on Maths 1233, 125-133. |
[11] | Radu V (2002) Some Remarks on Probabilistic contractions of fuzzy menger spaces, 8th international conference, on applied mathematics and computer science, Cluj-Napoca Maths 11, 125-131. |
[12] | Sehgal VM and Bharucha-Reid AT (1972) Fixed Point contraction mapping in Probabilistic Metric Space. Math System Theory, 6, 97-102. |
[13] | Sklar A and Schweizer B (2005) Probabilistic Metric space. Dover Publications, INC, Mineola, New York. |