Elem-Uche O.1, Omekara C. O.2, Okereke E. W.2, Madu C.1
1Department of Maths/Statistics, Akanu Ibiam Federal Polytechnic Unwana, Afikpo, Ebonyi State, Nigeria
2Department of Statistics, Michael Okpara University of Agriculture Umudike, Abia State, Nigeria
Correspondence to: Elem-Uche O., Department of Maths/Statistics, Akanu Ibiam Federal Polytechnic Unwana, Afikpo, Ebonyi State, Nigeria.
Email: |  |
Copyright © 2019 The Author(s). Published by Scientific & Academic Publishing.
This work is licensed under the Creative Commons Attribution International License (CC BY).
http://creativecommons.org/licenses/by/4.0/

Abstract
This paper gear to provide a vector error correction model VECM that establishes the relationship between real output between (GDP) and monetary policy transmission channel variables (credit, exchange rate and interest rate) for Nigeria. To achieve the objectives of this paper the vector error correction model VECM techniques was employed. The data used was sourced from Central Bank of Nigeria Statistical bulletin and it covers the period of thirty-seven years (1981 – 2017). The Augmented Dickey Fuller test was applied on the variables and it was observed that all the variables were non-stationary at level. The variables were differenced and their order of integration observed. Cointegration trace test performed showed that the monetary policy variable and its channels of transmission cointegrated. Based on the evidence of cointegration, the long term relationship was established. The VECM was also estimated and revealed that the variations observed in the real output in the short run were accounted by money supply, credit channel and exchange rate channel, and also that the values of real output adjusted speedily to the changes in money supply, credit channel, exchange rate channel and interest rate channel at long run. Hence, it was concluded that variations experienced by Nigerian real output are determined by money supply, credit channel and exchange rate channel in the short run, and money supply, credit channel, exchange rate channel and interest rate channel in the long run. Therefore, the VECM model for forecasting real output using monetary policy transmission channels in short term and long term was established.
Keywords:
Monetary policy, Real output, Transmission channels, Vector Error Correction Model
Cite this paper: Elem-Uche O., Omekara C. O., Okereke E. W., Madu C., Vector Error Correction Model for Forecasting Real Output Using Monetary Policy Transmission Channel Variables for Nigeria, American Journal of Mathematics and Statistics, Vol. 9 No. 2, 2019, pp. 92-99. doi: 10.5923/j.ajms.20190902.04.
1. Introduction
The real gross domestic product of a nation which refers to the real output is essentially a measure of the health and size of its economy (Usoro, 2018). As an indicator of the economic health, it is the value of a country’s overall output of goods and services at market prices excluding net income abroad. According to Usoro (2018), Nigerian real output is an aggregation of sectors that have significant contributions to the growth of the nation’s economy. These sectors include: agriculture, industries, building & construction, wholesale/retail trade, and services (CBN bulletin, 2017). It is vital to note that the growth of real output (GDP) of a nation is synonymous with the changes that take place in its economy. However, these changes which can be the success of the economy are largely dependent on the efficacy of monetary policy framework, and achieving this success requires some ability to peep into the near future. Consequently, decision makers must make forecasts to help them in decision making. To conduct these forecasts, most central banks take a number of variables into account (Feridun and Adebiyi, 2005).It is widely known that the central bank uses monetary policy to achieve the goals of macroeconomic management. This validates that monetary policy can be employed as a useful tool to control or influence the economy with a view to achieving set policy targets such as tackling unemployment, inflation, economic growth, etc. In this regard therefore, monetary policy plays an important role towards achieving the ultimate economic goals of sustainable growth, full employment, price stability and a healthy balance of payments. However, in the pursuit of these goals, the central bank always set intermediate goals for monetary policy. These are goals which relate to using interest rates, money supply, bank credits and the exchange rate to achieve the ultimate goals of monetary management, and these are regarded as channels through which monetary policy is transmitted to the macro economy with the aim of achieving on the ultimate objectives (CBN, 2008).Over the years Nigerian government has adopted various monetary policies through Central Bank of Nigeria in other to achieve success in growing her real output. Despite the increasing emphasis on manipulation of monetary policy, the problem surrounding her real output growth still persists. Such problems include high unemployment rate, low investment, high rate of inflation and unstable foreign exchange rate. These perceived problems are being claimed to have caused a fast decline in the real output of Nigeria.There is no consensus among economist as to whether government intervention through the use of monetary policy will bring about improvement in the growth of Nigerian economy (Nwoko et al., 2016). However, the Nigeria government in collaboration with its monetary authority still adopts certain policy to grow the economy, thus utilizing monetary policy without regarding its various transmission channels in manipulating the fluctuations experienced so far in the economy. These transmission channels are the routes through which monetary policy affects real output and macroeconomic activities, and are considered vital in growing the economy. Therefore, it becomes pertinent to determine the monetary policy transmission channels that influence the economy (real output) in Nigeria. It is in this regard that we model real output using selected monetary policy transmission channel variables for Nigeria, to examine how they have actually affected the variations in Nigerian real output.In this paper, certain questions raised were:i. Is there any significant relationship between monetary policy transmission channels and real output in Nigeria?ii. Which of these monetary policy transmission channel variables influence the variations experienced by the Nigerian real output? andiii. What effect has money supply on real output in Nigeria?One of the tools that aim to provide good statistical representations of these relationship with real output is the Vector autoregressive VAR or/and Vector error correction VEC models. They are dynamic system equations that examine the inter-relationship between economic variables, using minimal assumptions about the underlying structure of the economy. They have been shown to produce reliable forecasts of economic variables, and to examine the effects of economic fluctuations. The application of VECM to study real output monetary policy channel variables is empirically useful, as it reveals the variables that significantly promote growth in a developing economy like Nigeria.Hence, the objective of this paper is to determine a Vector Error Correction model (VECM) that establishes the relationship between real output and monetary policy transmission channel variables, and can be used in forecasting real output in Nigeria.Furthermore, it well known that the growth performance of an economy could be as a result of the influence monetary policy channels have on the real output. Thus, this paper gears to provide information to Government and Non-Governmental Agencies on subject about monetary policy channel variables that can be used to forecast Nigerian real output; and to academia’s and researchers on the application of Vector Error Correction model. This paper covers a period of thirty-seven (years) from 1981 – 2017.
2. Literature Review
In this paper we mentioned the use of Vector Error Correction Model to model the real output using monetary policy transmission channel variables for Nigeria. It is important to note that apart from modeling real output, VECM techniques have been widely applied in analyzing and establishing relationship between economic variables. To mention but few scholars who applied VECM techniques to analyze their works, we have the following.Maysami and Koh (2000) applied Vector Error Correction Model to analyze the long term equilibrium between Singapore stock index and selected macroeconomic variables as well as among stock indices of Singapore, Japan and United States. The study concluded that the Singapore Stock Market is significantly and positively cointegrated with stock markets of Japan and the United States.Zou (2018) used Vector Error Correction Model to establish a short term and long term relationship between oil price fluctuation and carbon emission change and GDP fluctuation. The study concluded that oil prices impacts will have a great influence on GDP and carbon emission in the short term, but in the long term, the influence will tend to be gentle.Hammoudeh et al., (2014) utilized Vector Autoregressive and Vector Error Correction Model to analyze the short term dynamic influence of changes in oil prices, coal prices and carbon emission quota on carbon emission prices. One important found conclusion is that a positive impact of the crude oil price will produce a negative effect on the approved price of carbon emissions.Asari et al., (2011) analyzed the relationship between interest rate, inflation rate and exchange rate volatility in Malaysia covering the period between 1999 – 2009 using Vector Error Correction Model. The study showed that at long term relationship, interest rate moves positively while inflation rate moves negatively towards exchange rate volatility in Malaysia.Bernhard (2013), using unrestricted Vector autoregressive model estimated the relationship between the channels of monetary transmission mechanism in Nigeria and the selected macroeconomic aggregates. The study shows that the three channels of transmission (the interest rate, exchange rate and credit channels) were functional for inflation targeting.Omoke and Ugwuanyi (2010), applied Cointegration technique and Granger Causality test analysis to investigate the relationship between inflation and output. The study shows no existence of co integrating vector in the series used. Thus, the result suggested that monetary stability can contribute towards price stability in Nigerian economy since the change in price level is mainly caused by money supply and thus concluded that inflation in Nigeria is to a large extent a monetary phenomenon.Okoro (2013), employed Augmented Dickey Fuller (ADF) test, Cointegration test and Vector Error Correction Model (VECM) techniques, to examine the impact of monetary policy on Nigerian economic growth. The results show the existence of long term equilibrium relationship between monetary policy instruments and economic growth.Adefeso and Mobolaji (2010), employed Cointegration procedure to investigate the relationship between fiscal - monetary policy and GDP in Nigerian. The result shows that there is a long – run relationship between GDP, degree of openness, government expenditure and broad money supply (M2).Much research have not been done by researchers on the modeling (establishing of relationship) or analyzing of real output using monetary policy transmission channels, in other to determine monetary policy channels that have significant influence on the variations experienced by Nigerian real output. So this paper gears to cover the gap considering the period under study.
3. Methodology
Data source The data used for this study is a secondary data and was sourced from Central Bank of Nigeria Statistical Bulletins and National Bureau of Statistics. The data sourced was arranged on annual basis and span from 1981 – 2017. The data include real output (proxy is GDP in Billion Naira), money supply (Billion Naira), interest rate (%), domestic credits (Billion Naira), and real effective exchange rate (N/US $1.00).Method of analysisThe data collected for the study was analyzed using Vector Error Correction Model VECM techniques. Vector Error Correction ModelGiven a VAR(p) of I(1) X’s (ignoring deterministic trends)
There always exists an error correction representation of the form (trick
)
Where,
and the
are functions of the
Specifically,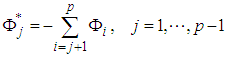
The characteristic polynomial is
Interpretation of
The
is referred to as the error correction mechanism. The error correction mechanism is the speed or degree of adjustment, that is, the rate at which the dependent variable
adjust to changes in the independent variables.Note, if
then there is no cointegration, that is, nonstationary of I(1) type vanishes by taking differences. If
has full rank, k, then the X’s cannot be I(1) but are stationary.
The interesting case is
as this is the case of cointegration. We write
Where the column of
contain the
cointegrating vectors, and the columns of
the
adjustment vectors.
Long term relationship in
There is an adjustment to the “equilibrium”
or long term relation described by the cointegrating relation.Setting
, we obtain the long run relation, i.e.
This may be written as
In this case,
, the number of equations of this system of linear equations which are different from zero is 
If the long run relation does not hold perfectly in
. There will be some deviation, an error,
The adjustment coefficients in multiplied by the “errors”
induce adjustment. They determine
so that the X’s move in the correct direction in order to bring the system back to “equilibrium”.Three distinguished cases for
are as follows:i.
ii.
iii.
Case 1:
In case of
it follows that:i.
, the null matrixii. There does not exist a linear combination of the I(1) VARS, which is stationary.iii. The X’s are not cointegrated.The EC form reduces to a stationary VAR(p-1) in difference
Case II:
The rank of
We factorize
in two
matrices
and 
, both
and
are 
The VEC form is then
In this case it follows that i. The X’s are integrated, I(1)ii. There are
eigenvalues
iii. The X’s are cointegrated. There are
linear combinations, which are stationary.iv. There are
linear independent cointegrating (column) vectors in
v. The
stationary linear combinations are
vi.
has
unit roots, so
common stochastic trends.Case III:
Full rank of
impliesi.
ii.
has no unit root. That is,
is I(0).iii. There are
stochastic trendsAs consequence we model the relationship of the X’s in level, not in differences. There is no need to refer to the error correction representation.Test for cointegration If there exists a stationary linear combination of non-stationary random variables, the variables combined is said to be cointegrated. Given the specification of the deterministic term, we test for the rank m of
. There are 2 sequential tests: Trace test, and Maximum eigenvalue testTrace testH0:
; against HA:
The likelihood ratio statistic is
We start with
; that is
. There is no cointegration against
, there is at least one cointegration relationship etc. If
isLarge
then
is large Small
then
Maximum eigenvalue testH0:
; against HA:
The likelihood ratio statistic is
We start with
; that is
. There is no cointegration against
, that there is at least one cointegration relationship etc.The critical values of both test statistics are nonstandard and are obtained via Monte Carlo Simulation.ForecastingThe fitted VECM can be used for forecasting
. The forecast of
-step ahead) are obtained recursively as
Stationary test (Unit root test)If a time series has a unit root, the series is said to be non-stationary. Tests which can be used to check the stationary include: Partial autocorrelation function and Unit root tests.The unit root test has become widely popular over the past several years. Hence, we used the unit root test known as Augmented Dickey Fuller (ADF) test. The ADF test simply runs a regression of the first difference of the series against a first lagged value, constant and time trend as follow:Without intercept and trend:
With intercept:
With intercept and trend:
H0:
(there is a unit root or
is non-stationary) against H1:
(there is no unit root or
is stationary) If t* > ADF critical value, accept null hypothesis, i.e. Unit root exist.If t* < ADF critical value, reject null hypothesis, i.e. unit root does not exist.Where, t* is the t-statistic critical value at various significant levels.Evaluation of fitted modelEvery statistical model relies on certain assumptions, and their properties have been proved based on the existence of these assumptions. Hence if a particular statistical model is used, then one must test these assumptions held true for that model. The fitted model was evaluated based on econometric criteria. It aims at investigating whether the assumptions of the VECM model are met. To achieve this, the following tests were considered:i. Test for residuals autocorrelationii. Test for normality (Jarque-Bera, 1980)
4. Results
Unit root testTable 1. Augmented Dickey Fuller unit root test 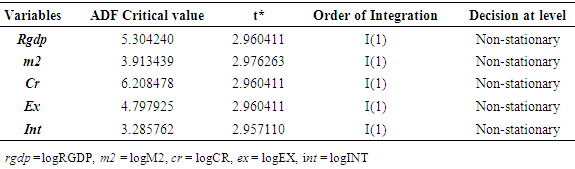 |
| |
|
The Augmented Dickey Fuller unit root test result on Table 1 showed that all the variables are non –stationary. The non – stationary variables were differenced to make the data stationary, and the order of integration was observed. Thus, Table 1 shows that the variables have the same order of integration I(1) (i.e. is stationary at first differencing). Co-integration testAll the variables as Table 1 showed exhibit unit root at level but after differencing, they all became stationary. The differencing was applied to avoid having a spurious regression. Since the differenced variables are stationary, there could be co-integration between the variables.This test enables one to know if some of the variables in the model are cointegrated and as well shows if there is long term relationship existing between the set of the variables. The number of the lag used in the co-integration test is based on the information criterion used in the underlying model. The cointegration trace test of real output (rgdp), money supply (m2), credit channel (cr), exchange rate channel (ex) and interest rate channel (int) is presented below. The cointegration test result above shows that the null hypothesis of no cointegration was rejected since the trace test indicated one cointegrating equation at the 5% significance level. From the result, it could be inferred that a long term relationship or cointegration exists among the variables (real output, money supply, credit channel, exchange rate channel and interest rate channel).Based on this evidence, the vector error correction model VEC Model is estimated to account for the short term dynamics (relationship).Vector Error Correction Model (VECM)The long run model Table 2. Cointegration trace test 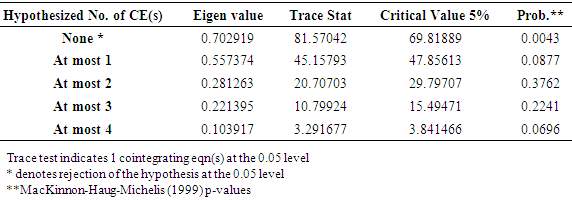 |
| |
|
The result of the cointegration test on Table 2 shows the existence of long term relationship among the variables. The cointegrating equation was chosen based on log likelihood ratio. If the log likelihood ratio is positively signed, we chose the equation with the lowest log likelihood ratio and if negative signed, we chose the highest log likelihood ratio at absolute term. All the cointegrating equations were observed to be positively signed, so the lowest log likelihood ratio chosen. The lowest log likelihood ratio had the corresponding co-integrating equation result stated below.Table 3. Long term relationship 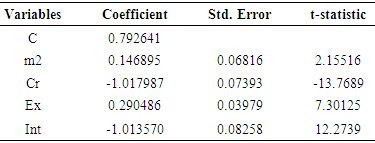 |
| |
|
Table 3 which presents the long term relationship equation showed that all the variables are significantly influencing real output in Nigeria. However, if all variables are held constant, it shows that real output (RGDP) will be maintaining 0.792641 units in the long term. The coefficient of money supply (m2) is 0.146895. This coefficient which is positively signed indicates that money supply and real output are directly related, and that a unit increase in money supply will tend to influences the growth of real output by 0.146895 units in Nigeria. Also, the coefficient of exchange rate (Ex) which is 0.290486 reveals that exchange rate and real output are directly related, and that a unit increase in exchange rate will tend to influence real output growth by 0.290486 units. Credit channel (Cr) has a coefficient of -1.017987. It can be deduced that credit channel has an inversely relationship with real output. This implies that as credit channel increases by a unit; it influences real output to decrease by 1.017987 units. Also, Interest rate (Int) has inverse relationship with real output with negative coefficient of -1.013570. This implies that as interest rate increases by a unit, real output will be influenced to decrease by 1.013570 units.Short run modelSince, it has been ascertained that the variables exhibit unit root (non-stationary) at their levels but stationary after differencing, and there is also existence of cointegration (a long term relationship) between the variables, we now generate the vector error correction model which will give the proportion of disequilibrium error that is accumulated in the previous period, corrected in the current period (speed of adjustment). An over-parameterized vector error correction model is then estimated by setting the lag length long enough to ensure that the dynamics of the model have not been constrained by a too short lag length. The result of the estimated vector error correction model VECM is given as below.Table 4. Over-parameterized Vector error correction model 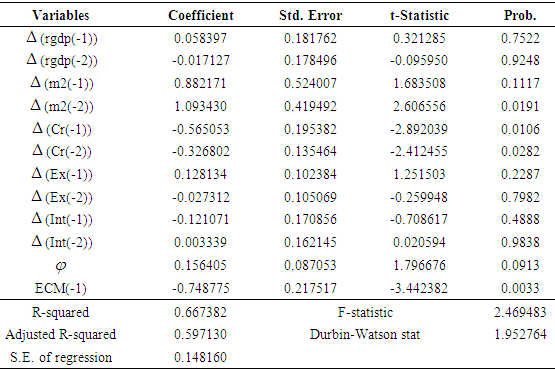 |
| |
|
To test for the speed of adjustment using the short term dynamism of error correction mechanism (ECM), Table 4 shows that the coefficient ECM(-1) is negatively signed and highly significant. The negative sign justifies its significance. This means that it will be effective to correct any deviations from the long term equilibrium. The coefficient of ECM is -0.748775, indicating that, the speed of adjustment to long run equilibrium is 74.9% when any past deviation will be corrected in the present period. This implies that the present value of real output adjust speedily to changes in money supply, credit channel, exchange rate channel and interest rate channel. Table 4 also shows that the coefficient of determination is 0.667382, indicating that the variables accounted for 66.7% of total variations in real output in Nigeria. However, there is a need to simplify the model into a more interpretable and certainly more parsimonious model. The parsimonious model would be built by estimating the equations of only those variables found to be significant in the over-parameterized model.Table 5. Parsimonious vector error correction model 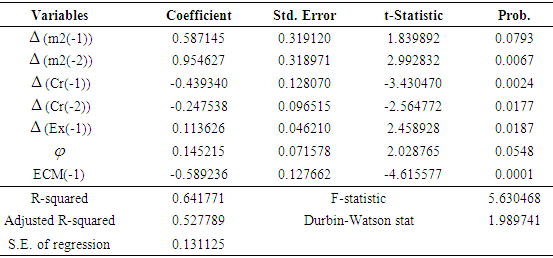 |
| |
|
From the Table 5 above, it shows that the coefficient of ECM is -0.589236. The ECM is significant with the appropriate negative sign. The coefficient of ECM in the parsimonious model indicates that the speed of adjustment of any past deviation to long term equilibrium is 58.96%. This shows that present value of the dependent variable adjust more slowly to changes in the independent variables than what was obtained in the over-parameterized model. The result of the parsimonious model also revealed that all variables are significant. Their significance was determined by taking into consideration their probability value. The corresponding probability value of each variable considered must be less than 10%. The coefficient of determination (0.641771) revealed that the variables accounted for 64.18% of variations in the real output.Hence, from the VEC model, it can be deduced that changes influencing output were determined by money supply, credit channel and exchange rate channel in the short term, and money supply, credit, exchange rate and interest rate in the long term.Diagnostic checkingTable 6. Breusch-Godfrey L-M test 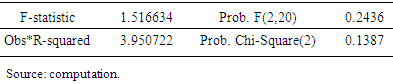 |
| |
|
The result of Table 6 is the Breusch-Godfrey Langrange Multiplier (LM) test for autocorrelation of the residuals. The result shows that probability value is greater than 0.05 (5% level of significance), indicating that there is no presence of autocorrelation in the residuals.Table 7. Descriptive summary of the residuals 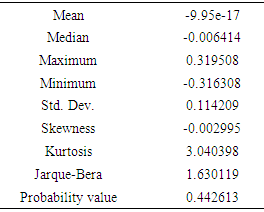 |
| |
|
Table 7 shows the descriptive summary of the residuals. The summary shows that the mean is zero, skewness is zero, kurtosis is approximately 3 and the probability value of the Jarque-Bera value is greater than 0.05 (5% level of significance). This implies that the residuals (error terms) is normally distributed.Forecast errorThe real output forecast for the long term model and short term model were carried out as shown in figures below. The statistical measures such as RMSE, MAE and MAPE etc. used to determine how closely a forecast tracks its actual data were also outline in the figures. 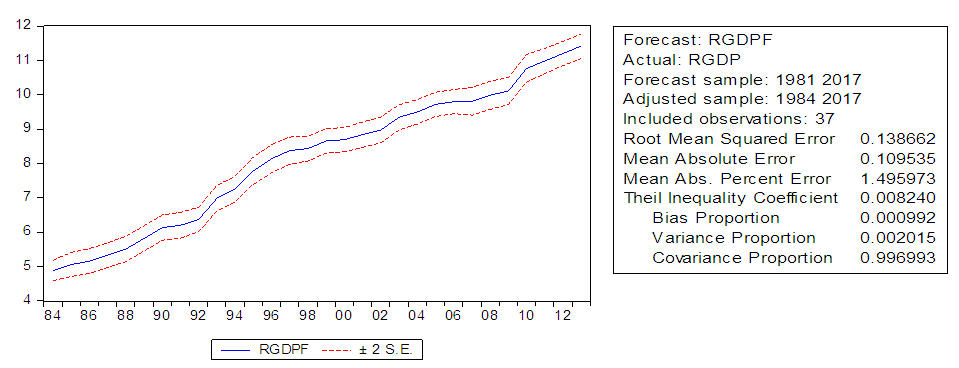 | Figure 1. Forecast for the long term model |
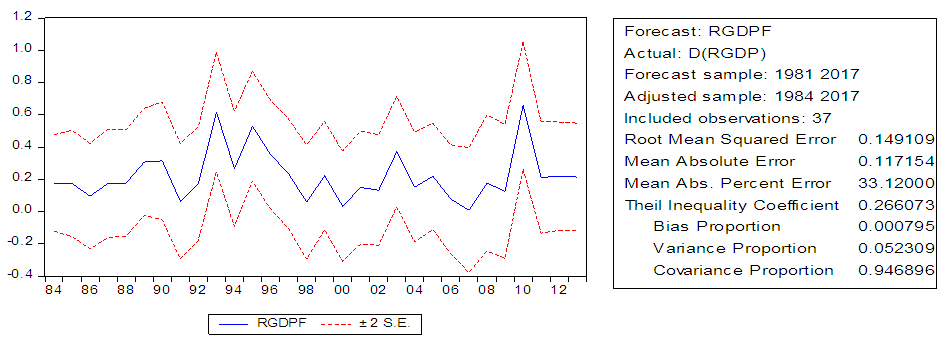 | Figure 2. Forecast for the short term model |
5. Conclusions
Based on the results, it could be concluded that the vector error correction model VECM generated can produce a good fit or forecast for the Nigerian real output. Also, an increase in money supply has a significant influence on real output in Nigeria positively. The changes experienced in Nigeria’s real output are from variations in money supply, credit and exchange rate channels at short term dynamics, and from money supply, credit channel, exchange rate channel and interest rate channel at long term dynamics. Finally, the significance of the monetary policy channels showed that their influence on the real output could aid in promoting and stabilization Nigeria’s economy as desired.
6. Recommendations
Based on the findings, we recommend that several measures could be implemented in the short term to strengthen the interest rate channel. The interest rate should be emphasized on in order to stimulate the real output. Also, monetary policy transmission through the credit channel and exchange rate channel should continue to be strengthened by tightening creditworthiness standards; strengthening accounting standards, bankruptcy laws, corporate governance, and credit rights; improving bank credit assessment capabilities; and strengthening the judicial system to improve banks’ ability to enforce on collateral. In addition to ensure the effectiveness of the credit channels, the monetary authority should maintain a low and stable inflationary level. Finally, the monetary policy channel variables can be used to forecast the performance of real output in Nigeria.
References
[1] | Adefeso, H. & Mobolaji, H. (2010). The fiscal–monetary policy and economic growth in Nigeria: Further Empirical Evidence. Pakistan Journal of Social Sciences, 7 (2), 137-142. |
[2] | Asari, F. H., Baharuddin, N. S., Jusoh, N. Mohamadu, Z., Shamsudin, N. & Josoff, K. (2011). A Vector Error Correction Model (VECM) approach in explaining the relationship between interest rate, inflation towards exchange rate volatility in Malaysia. World Applied Sciences Journal, 12, 49-56. |
[3] | Bernhard, O. I. (2013). Monetary transmission mechanism in Nigeria: Causality test. Mediterranean Journal of Social Sciences, 4(13), 377-388. |
[4] | CBN (2008). Central Bank of Nigeria (CBN), Monetary Policy Department Series 1, 2008. CBN/MPD/Series/01/2008. |
[5] | CBN (2017). Statistical Bulletin; 2017. |
[6] | Feridun, M. & Adebiyi, M. A. (2005). Forecasting Inflation in developing economies: The case of Nigeria. International Journal of Applied Econometrics and Quantitative Studies. 2-4. |
[7] | Gujarati, D. N. & Porter D. C. (2009). Basic Econometrics (5th Edition). New York: McTATA McGraw Hill Book Company. |
[8] | Hammoudeh, S. Nguyen, D. K. & Sousa, R. M. (2014). Energy prices and CO2 emission allowance prices: A Quantile regression approach. Energy Policy, 70, 201 – 206. |
[9] | Jarque, C. M. & Bera, A. K. (1980). Efficient tests for normality, homoscedasticity and serial independence of regression residuals. Economics Letter, 6 (93): 255 – 259. |
[10] | Koutsoyinannis, A. (1977). Theory of econometrics. London: Macmillan Press Ltd. |
[11] | Maysami, R. C. & Koh, T. S. (2000). A vector Error Correction Model of the Singapore Stock Market. International Review of Economics and Finance, 9, 79 – 96. |
[12] | Nwoko, N. M., Ihemeje, J. C., Anumadu, E. (2016). The Impact of Monetary Policy in theconomic Growth of Nigeria. An International Multi-disciplinary Journal, Ethiopia, 10(3), 192-206. |
[13] | Okoro, A. S. (2013). Impact of monetary policy on Nigeria Economic Growth. Prime Journal of Social Sciences, 2(2), 195-199. |
[14] | Omoke, P.C. & Ugwuanyi, C. U. (2010). Money, price and output: A causality test for Nigeria. American Journal of Scientific Research, (8), 78-87. |
[15] | Usoro, A. E. (2018). Modelling of Nigeria gross domestic product using seasonal and bilinear autoregressive integrated moving average models. Journal of Statistical and Econometric Methods, 7(2), 1-21. |
[16] | Zou, X. (2018). Vector Error Correction Model analysis of carbon emissions, GDP and International crude oil prices. Discrete Dynamics in Nature and Society. http://dio.org/10.1155/2018/5350308. |