Mugotitsa B., Orwa G., Mung’atu J.
Department of Statistics and Actuarial Sciences, Jomo Kenyatta University of Agriculture and Technology, Nairobi, Kenya
Correspondence to: Mugotitsa B., Department of Statistics and Actuarial Sciences, Jomo Kenyatta University of Agriculture and Technology, Nairobi, Kenya.
Email: |  |
Copyright © 2018 The Author(s). Published by Scientific & Academic Publishing.
This work is licensed under the Creative Commons Attribution International License (CC BY).
http://creativecommons.org/licenses/by/4.0/

Abstract
The focus of this study is to fit a model that ranks commercial banks in Kenya, according to their specific financial status. The second focus of this study is to use dimensionally reduced financial distress determinants. Secondary data in audited bank financial statements from the Nairobi Stocks Exchange (NSE) report 2016/2017, and other statements available from bank websites used for the fitting of the model. In total, 17 banks were sampled. 10 explanatory variables were used to analyze the linkage between bank specific factors and economic factors majorly associated with bank fragility. The data obtained was cleaned, coded and the required statistical outputs generated using R for Windows statistical package. Principal component analysis (PCA) was employed in dimension reduction. A multiple logit regression model was fitted to the collected data. The most significant determinants of bank fragility were found to be bank size and profit before tax. Using the derived logit model, it was determined that Kenya Commercial Bank (KCB), Equity Bank and Cooperative Bank were the top three banks in terms of financial strength. Their probabilities of staying up were 1.000, 0.999 and 0.985 respectively. The bottoms two were Chase Bank (0.125) and Imperial Bank (0.037). These two were under receivership during this study.
Keywords:
Logit model, Principal component analysis, Bank fragility, Correlation analysis
Cite this paper: Mugotitsa B., Orwa G., Mung’atu J., Application of the Logit Hazard Model as an Approach for Ranking of Banks Fragility Status in Kenya, American Journal of Mathematics and Statistics, Vol. 8 No. 3, 2018, pp. 65-69. doi: 10.5923/j.ajms.20180803.02.
1. Introduction
BackgroundBanking system fragility has impaired the functioning of the payment system thus leading to various challenges including high interest rates, high inflation rates, bank fraud, banks being put under receivership and economic stagnation [2, 8, 12]. This research will point out the major determinants of banking system fragility in Kenya and the level of their influence to rank commercial banks under study, a topic which related studies largely overlooked in the current academic literature which mainly focus on stability of individual banks or individual countries banking systems [1, 18]. We refer to banking system fragility as a situation where there is present vulnerability of a financial system to a financial crisis. But the attainment of the banking benefits has been jeopardized because both private and commercial banks have been vulnerable to financial distress, with private banks being on the lead. Substantial numbers of banks have failed, mainly because of various fragility determinants. Poor banking sector quality has its roots in the informational problems which afflict financial markets, and which are at their most acute in developing countries, including Kenya. The field of business prediction comprises bankruptcy prediction, firm failure prediction and financial distress prediction [4]. As the name suggests, it involves developing models that attempt to predict the financial failure of a business before it actually happens [6, 17]. The vulnerability of the banking sector associated with a weak macroeconomic environment is characterized by a large government deficit, a large trade deficit, high inflation, excessively high real interest rates, depreciated exchange rate and slow GDP growth. These are among the main factors that increased the problems in the banking sector and led to the emergence of the banking crisis in Kenya [15]. Theoretical ModelsTheoretical models can be examined in three categories: the first, second and third-generation models [5]. The first-generation models focus on the role of weak fundamentals in the policy of government as a triggering factor of currency crises. The fiscal deficit, growth of money supply, current accounts balances and level of foreign exchange. This were the main features of the crisis in the early 1980s in Latin America [7, 13, 14]. The second-generation currency crises models offered no critical developments to fundamental macroeconomic variables; instead, it provided model of self-fulfilled speculative attacks in foreign exchange markets. In this model, market expectations directly influence macroeconomic policy decisions in a rational direction. During this crisis period in Africa countries inflation rates, government deficit and unemployment were low and credit was high. Hence, this crisis in Africa revealed the need for a new framework that integrates weaknesses in the banking sector into the early generation models. This new framework, which takes into account the weaknesses of the financial sector, is called the third-generation models or the term twin crises, which emphasize the occurrence of both banking and currency crises [20].
2. Material and Methods
This research attempted to identify the determinants that have more effect on the overall bank financial status and use this determinants to rank commercial banks in Kenya according to their financial fragility status. DataSecondary data in audited bank financial statements from the Nairobi Stocks Exchange (NSE) report 2016/2017 financial year, and other statements available from bank websites were used. 12 banks were part of the research, 10 of which were listed under NSE sampled. Each had a 5 year financial reporting period 2011-2016 except for Barclays which had the 2010 statement available. Out of these, 9 were private and 3 public (KCB, Coop Bank and NBK). Further, data from two recently failed banks, not listed in the NSE was obtained from the specific banks. 10 explanatory variables were used to analyse the linkage between bank specific factors and economic factors majorly associated with bank fragility. The data obtained was cleaned, coded and the required statistical outputs generated using R for Windows statistical package. Independent VariablesTable 1. Independent Variable Definitions 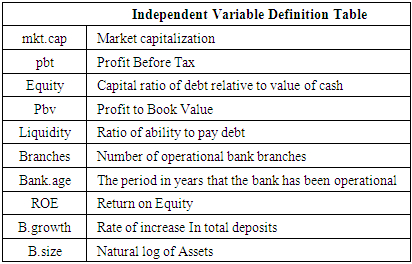 |
| |
|
Dependent VariableThe variable to be estimated was Bank status index S, which assisted in telling the state whether a bank is in a healthy financial status or not. This was analysed using the probability between 0 and 1 of the determinants. A bank with probability close to 0 is considered to have possible failure and that of probability close to 1, possible success.MethodologyBy use of Principal Component Analysis (PCA), a statistical procedure that uses a set of observations of possibly correlated variables and converts them into a set of values of linearly uncorrelated variables called principal components which are less than the number of original variables, the most significant variables were the successfully selected. The selected variables were then used to fit a logit hazard model to bank fragility [19]. This model is often used when the relationship between the discrete variable and the predictor variable is nonlinear. The aim was to fit a regression model whose response variable is binomial. That is, bank financial status.Akaike Information CriteriaAkaike Information Criteria (AIC) penalizes increasing number of coefficients in the model. In other words, it helps to avoid overfitting. Looking at the AIC metric of one model wouldn't really help. It is more useful in comparing models (model selection). So, I fitted several Logistic Regression models and compared their AIC. The model with the lowest AIC is relatively better. Several logistic models were built and their AIC compared. The model with the lowest AIC is relatively better. This is an important indicator of whether the model is of good fit.From the analysis, it was concluded that the best choice of a model that has significant coefficients and minimum AIC values is | (1) |
This had AIC = 23.308 with both independent variables having significant coefficients at 5% level of significance.Variance Inflation FactorAnother measure of the viability of variables in a model is the Variance Inflation Factor (VIF). An effort was made to compute the VIF for each independent variable in each model. Essentially, it's desired that for a model with good fit, the VIF be less than 5. All the models considered here pass this test. The best model in a set of many however is that with the least VIF. Here again the model with VIF 1.004 was preferred. The following output summarizes the outcomes.Table 2. Variance Inflation Factor 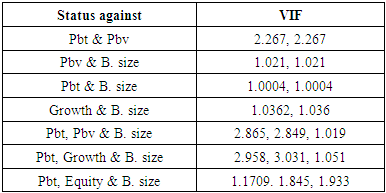 |
| |
|
The mean performance was then used to rank the individual banks under study according to their financial status over the years under consideration.
3. Results and Discussion
Correlation analysisCorrelation analysis between the dependent variable and independent ones was carried out with pairwise scatter plots generated for all main variables. Some of the variables were found not to be related to the rest of the variables and with bank status. These include number of branches, bank age, return on equity and liquidity ratio.The plots below (Figure 1 and Table 3) show the inter relationships between variables whose correlations were found to be strongest. The respective correlation analysis gave the following results.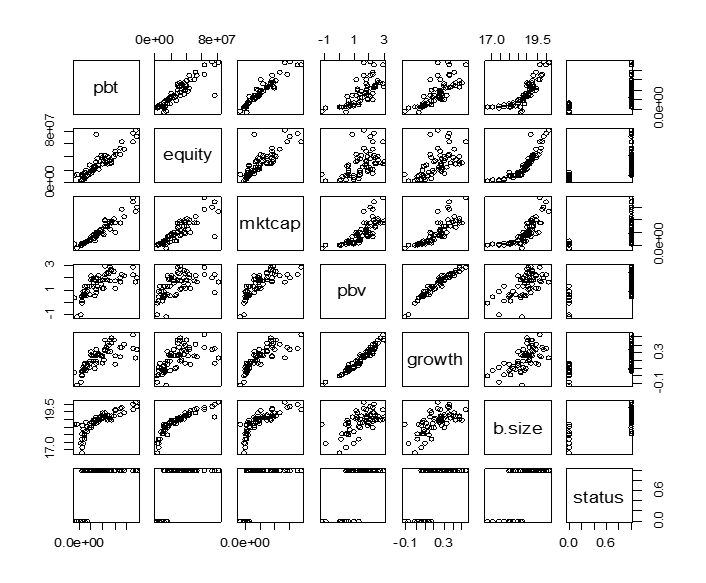 | Figure 1. Correlation Analysis |
Table 3. Correlation analysis 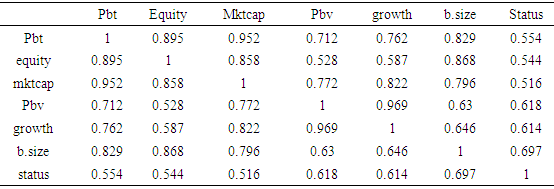 |
| |
|
From the correlation matrix (table 3), profit before tax (pbt) was highly correlated to market capitalization (mkt.cap), equity and bank size (bank.size). Further, price to book value (pbv), return on equity (roe) and growth are also highly correlated. It’s noted that liquidity, bank age and number of branches are sparsely correlated with the rest of the variables. The following variables have the same effect in the intended regression model. pbt and mktcap, growth and pbv, equity and b.size. This would lead to over-fitting (multicollinearity). We therefore select the variables pbt, b.size and growth to predict status. Profit before tax (pbt) is a reliably good measure of bank stability. We expect that good predictors of bank fragility be well aligned with pbt. Indeed, from the correlation matrix, pbt has the highest number of independent financial variables strongly related to it.Principal component analysisPrincipal component analysis (PCA) results were computed and presented as followsTable 4. A table of eigenvalues for the principal components 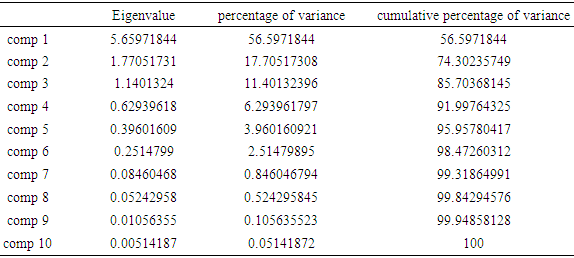 |
| |
|
Components with eigenvalues greater or equal to 1 are usually selected. Approximately 86% of the variance is explained by the first 3 components. The rest contribute less to the predictive power of the new variables (components). For a 95% explanatory power, the first 5 components are selected.Scree-plot A scree-plot is used to visualize the variations along the principal components. Principal components along the steep gradient are selected while those running asymptotically at the bottom has less explanatory power to the model. Three principle components can be extracted. Using the rotation matrix, we determine them as follows:The logit ModelA logistic regression is a statistical modeling method used to analyze multivariate problems [16]. It attempts to describe the complex relationship between a set of independent explanatory variables and a response variable. The logistic (logit) model assumes that the disturbance term
has a standard logistic distribution: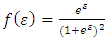 | (2) |
The model equation that was of best fit was: | (3) |
This gives the probability that the bank in question is in good financial health status given the two predictor variables, bank size and profit before tax.
4. Conclusions
The main objective of this study was to fit a logit model to bank fragility and use the model to rank commercial banks in under study. A successful attempt was made to fit the logistic regression model to the data, which could be used to predict bank status given a few variables. Finally, after testing the model with bank data, the model was verified. This was then used to rank the banks under consideration in terms of their financial status. The study was also set to perform PCA for dimensional reduction of data used in constructing the bank fragility model. This was carried out successfuly. It was noted that out of the ten initial variables, some were not significant contributors in the determination of bank fragility. These included bank age, number of branches, liquidity ratio, and Return on Equity.The scree plots (figure 2) together with the rotation matrix show the contributions of variables towards the important principal components. The first three contribute to 86% of variation caused by the selected variables. fewer variables were selected for modelling to avoid over-fitting, due to multicollinearity. Variables were discriminated logically and a lean and effective model fit.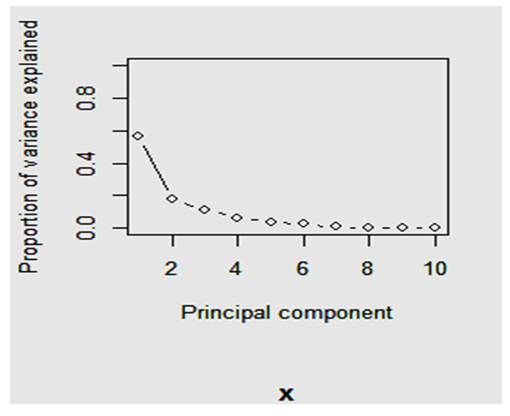 | Figure 2. Scree plot from PCA |
The best model fit had the independent variables as bank size and profit before tax. This gave the probability that the bank in question is in good financial health given the two predictor variables.Using the model, the best performing bank was KCB with status index 1.000. This was followed closely by Equity bank with 0.999 then Cooperative bank with 0.985. At the lower end was National Bank of Kenya (0.219) then Chase bank (0.125) and Imperial bank (0.037).
ACKNOWLEDGEMENTS
I acknowledge the Nairobi Stock Exchange Directorate for making available the Banks Financial Statemnts and the Individual banks database where the individual statements of financial records were also accessed.
References
[1] | Alves, K., Matias B., & Kalatiz A. (2009). Survival Analysis of Private Banks in Brazil. Banking Failure Prediction Model. / Ecomod printers. |
[2] | Angela, S.E. (2014). Indicators of Banking System Stability Index in Nigeria. Banking Fragility in Nigeria. Journal of Applied Statistics, 49-77. |
[3] | Borio, L., & Lowe, P. (2002). Bank for International Settlements, Asset Prices, Financial and Monetary Stability. Early Warning Indicators for Financial Distress in Switzerland. Journal of BLE, 1-39. |
[4] | Caputo, P., & Partovi, H., (2004). Analysis of Efficient Portfolio Problem using Principal Component Analysis. Economics Bulletin, vol.7 (3), 1-10. |
[5] | Curry, E., Elmer, P., & Curry, T. (2004). Traditional Models That Predict Bank Failure. Can the Equity Markets Help Predict Bank Failure? American Journal of Theoretical and Applied Statistics, 1-39. |
[6] | Daniel, M. (1997). Early warning of bank failure: A logit regression approach. Journal of Banking & Finance, vol.1, 249-276, https://doi.org/10.1016/0378-4266(77)90022-X. |
[7] | Davis, Z. (1993). Effects of Credit Risk in Banks to Property Loss. Bankruptcy as A Result Of Credit Risk. Bank of England Working Papers, vol.8, 1-26. |
[8] | Demirguc, K., & Levine, R. (2008). Finance, Financial Sector Policies and Long-Run Growth. Commission on Growth and Development. Vol. 11, 1-68. |
[9] | Espahbodi, P. (1991). Identification of Problem facing Banks and Binary Choice Models. Journal of Banking and Finance. Vol.15, 53-71. |
[10] | Espahbodi, P., & Hamer, M. (1996). On the Robustness of Results of Adoption Debt Choice Studies: Case of Pension Accounting. Kluwer Academic Publishers, Vol.6, 63-77. |
[11] | Fox M., Lash T., & Greenland S. (2005). A Method to Automate Probabilistic Sensitivity Analyses of Misclassified Binary Variables. International Journal of Epidemiology. 1370-1376. |
[12] | Gertler, M., & Bernanke, B. (1989). Agency Costs, Net Worth and Business Fluctuations. The American Economic Association. Vol.79, 14-31. |
[13] | Gonzalez, H., Ceyla, P., & Billings, R. (1997). Determinants of Banking System Fragility: A case Study of Mexico. International monetary fund, vol.44, 295-314. |
[14] | Gunsel, N. (2010). Determinants of the Timing of Bank Failure in North Cyrus. Journal of Risk Finance. Vol.11, 89-106. |
[15] | Gwaya, O., & Mungai, J. (2015). The Effect of Mergers and Acquisitions on Financial Performance of Banks: A Survey of Commercial Banks in Kenya. International Journal of Research and Development, vol.4, 101-113. |
[16] | Hayden, E., & Halling, M. (2006). Two Step Survival Time Analysis. A Discrete Logit Time Model with Survival Time Variables That Allow Time-Varying Explanatory Variables. Vol.33, 1-36. |
[17] | Henebry, K. (1997). Test of Temporal Stability of Proportional Hazard Models for Predicting Bank Failure. Journal of Financial and Strategic Decisions. Vol.10, 1-12. |
[18] | Hermosillo, G. (1996). Modeling bank fragility and Systemic Sources of Fragility. Journal of Finance. 1-42. |
[19] | Hermosillo, G., Ceyla, P., & Pazarba, B. (1997). Determinants of Banking System Fragility. IMF Staff Papers. Vol.44, 295-314. |
[20] | Jan, W., Zake, P., & Boadu, V. (2016). Developing a Model for Determining Corporate Bank Performance after Bank Acquisition. International Journal of Science, 1-33. |
[21] | Jordan, C., & Jokil, H. (1996). Evaluating the Financial Health of Midwestern Banks. Journal of Banking and Finance, 511-531. |