Akinrefon Adesupo A.1, Bamigbala Olateju A.1, Jibasen Danjuma1, Ike Emmanuel2
1Department of Statistics and Operations Research, Modibbo Adama University of Technology, Yola, Nigeria
2Department of Physics, Modibbo Adama University of Technology, Yola, Nigeria
Correspondence to: Bamigbala Olateju A., Department of Statistics and Operations Research, Modibbo Adama University of Technology, Yola, Nigeria.
Email: |  |
Copyright © 2017 Scientific & Academic Publishing. All Rights Reserved.
This work is licensed under the Creative Commons Attribution International License (CC BY).
http://creativecommons.org/licenses/by/4.0/

Abstract
The study examines the factors associated with diarrhea prevalence in Yola of Adamawa State. Using Poisson regression model, while Quasi-Poisson and Negative Binomial were used to handle over dispersion. Pearson Chi-Square and Deviance was used to check for the goodness of fit. Data was extracted from the medical records of Adamawa State Specialist Hospital and Valli Clinic Yola. The variables on which the data were collected included sex, age group, year and hospital type. AIC of model 3 was selected as the least with AIC of 355.29. This model examines the interaction between hospital type and year of occurrence independent of age of patient, year and their gender. We found that male children are more likely to be infected with diarrhea compare to females. The study also revealed that as the children advanced in age, the rate of been infected with diarrhea decreases. The hospital type indicates that more patients infected with diarrhea visited Specialist hospital than Valli Clinic. The rate of infection increases every year but reduce in 2015 compared to 2014.
Keywords:
Diarrhea, Chi-Square, Deviance, Poisson Regression, Negative Binomial, Odds
Cite this paper: Akinrefon Adesupo A., Bamigbala Olateju A., Jibasen Danjuma, Ike Emmanuel, Statistical Modelling of Diarrhea Prevalence among Infants in Adamawa State, Nigeria, American Journal of Mathematics and Statistics, Vol. 7 No. 1, 2017, pp. 27-31. doi: 10.5923/j.ajms.20170701.04.
1. Introduction
Diarrhea is the second leading cause of deaths among children younger than 5 years, with approximately 4 billion cases occurring each year in this age group (UNICEF/WHO, 2009). Tobin, Isah and Asogun (2014) studies diarrhea incidence and home management practice of a rural community in Edo State Nigeria and concluded that nursing mothers knowledge on diarrhea is on the decline and that diarrhea become more complicated with insufficient fluid intake. Desalegn, Kumie and Tefera (2011) stated that factors associated with diarrhea in children include Acute Respiratory infection (ARI), maternal history of recent diarrhea, maternal education, source of water, (e.g. obtaining water from storage container by dipping), availability of latrine facilities, living in a house with fewer number of rooms, not breast feeding, duration of breast feeding, and age of the child. Oyinloye, Jatau, Aminu and Ella (2016) survey the presence of norovirus and astrovirus antigen in Diarrhea stools of children in Borno State Nigeria. The study suggest that norovirus prevalence of 8% and astrovirus prevalence of 5% contribute significantly to diarrhea disease of childhood in Borno State, Nigeria. Furthermore, according to the sex stratification sample it revealed male susceptibility rate (6/130) to be greater than female susceptibility rate. Risk factors that predispose children to diarrhea include poor sanitation, poor social and economic status and malnutrition (Andu, Omilabu, Peenze and Steele, 2002). It was reported that in most South Asian countries, that poverty, high population density, low status of women, poor antenatal case, high rates of low birth weight, unfavorable child caring practices and poor access to health care are the underlying risk factors associated to the diarrhea which have contributed to the development of protein-energy malnutrition (PEM), (WHO, 2010). With the different roles played by factors that have been considered in previous studies on diarrhea, we carried out this study with a view to see how hospital-type and year of disease occurrence as a factor could be considered in diarrhea prevalence among infants of Adamawa State.
2. Methodology
Data for this work were obtained from the Adamawa State Specialist Hospital, Yola and Valli Clinic, Yola, Adamawa State Nigeria between 2013 to 2015. Data collected on infected patients includes age, gender, hospital type and year of occurrence.
2.1. Poisson Regression Model
The Poisson regression is a member of a class of generalized linear models (GLM), which is an extension of traditional linear models where Poisson regression is often used to analyze count data. It can be used to model the number of occurrences of an event of interest or the rate of occurrence of an event of interest, as a function of some independent variables. For example, the rate of insurance claim, number of doctor visits, incidence of diseases, crime incidence, number of days a child is absent from school. Colony counts of bacteria, the number of people affected with HIV/AIDs, the number of deaths from fatal accident can be modelled using Poisson regression, (McCullagh and Nelder, 1989).In Poisson regression it is assumed that the dependent variable Y, number of occurrence of an event, has a Poisson distribution given the independent variables
Where
and
This is called the equidispersion property of the Poisson distribution. The log of the mean µ is assumed to be a linear function of the independent variables, that is,
where
or equivalently,
This is the model for analyzing normal count data.Sometimes, the response may be in the form of events of certain type that occur over time, space or some other index of size. When a response count Y has index (such as population size) equal to
the sample rate of occurrence is
. The expected value for rate is
Thus, for analysis rate data, the model can be written as,
This model has equivalent representation as,
The adjustment term –Int, on the left-hand side of the equation is called an offset.
2.2. Estimation of Parameters Using Maximum Likelihood Estimation (MLE)
Estimation of parameters in Poisson regression relies on maximum likelihood estimation (MLE) method. Maximum likelihood estimation seeks on answer question of what values of the regression coefficients are most likely to have given rise to the data. To discuss the maximum likelihood estimation for Poisson Regression. Let
be the mean for the
response, for i=1,2,…,n. Since the mean response is assumed to be a function of a set of explanatory variables.
the notation
is used to denote the function that relates the mean response
(the values of explanatory variables for case i) and β (the values of regression coefficients). Now consider the Poisson regression model in the following form: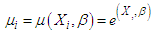 | (1) |
Then, from Poisson distribution,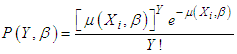 | (2) |
The likelihood function is given by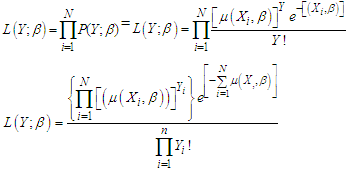 | (3) |
The next thing to do is taking natural log of the above likelihood function. Then, differentiate the equation with respect to β and equate the equation to zero. The log likelihood function is given as, | (4) |
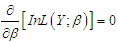 | (5) |
The solution to the set of Maximum Likelihood given above must generally be obtained by iteration procedure. This procedure will estimate the values of β. Maximum likelihood estimation produces Poisson parameters that are consistent, asymptotically normal and asymptotically efficient. (See Agreti P.147)
2.3. Overdispersion Test
In the Poisson regression analysis, there is an assumptions of equidispersion that the mean value of the variance must meet. However, this assumption is rarely fulfilled. Detecting over dispersion can be seen from the value of deviance/df or chi-square/df. If the value of the deviance/df or chi-square/df is greater than 1, it can be seen to be a case of overdispersion whereas if it is less than 1 then there is under dispersion.
2.4. Negative Binomial Regression Analysis
The negative binomial regression model is derived by rewriting Poisson regression model such that,
The negative Binomial regression distribution has the form.
Where
is a gamma function. This results in the likelihood function.
Maximum likelihood estimation is used to estimate parameters in negative binomial. In addition, the interpretation of regression coefficients for negative binomial regression is the same as for Poisson regression.
3. Results
The number of infants infected with diarrhea was modelled using Poisson regression and the results are presented in the Table 1. The Table contains various models considered and their Akaike Information Criterion (AIC).Table 1. The Poisson Regression Models for the Number of Infants with Diarrhea from 2013 to 2015 with their AIC’s 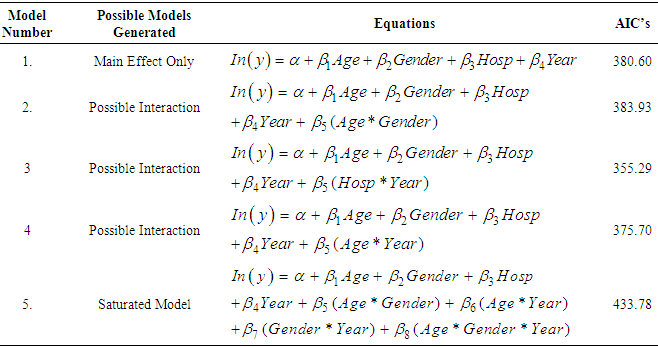 |
| |
|
The Table 1 shows that the best model which fit the infants infected with diarrhea from 2013 to 2015 is model 3 because it has the smallest AIC. The model 3 examines the interaction between hospital type and year of occurrence is independent of age of patient and their gender. Table 2 shows the results for the selected model (interaction between hospital type and year of occurrence is independent of age of patient and their gender) using Poisson Regression Model. From the table, babies between 1-3 years and 4-5 years are 0.7058 and 0.2424 times as likely to experience diarrhea incidence as those below 1 years. Also, Specialist hospital seem to record more cases of babies admitted for diarrhea cases than Valli clinic. These incidence seem to be on the increase between 2013 and 2015.Table 2. The Parameter estimate of Selected Poisson Regression Model for number of infants Infected with Diarrhea from 2013 to 2015 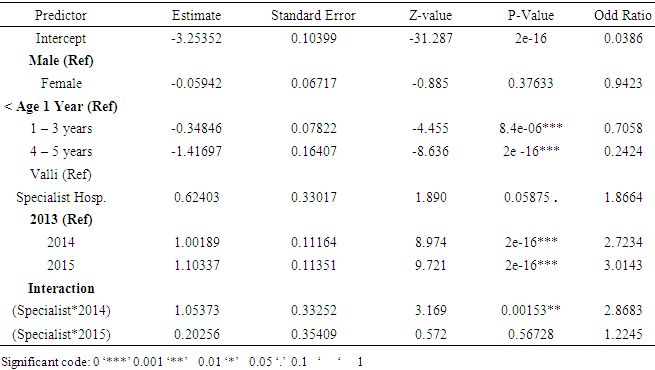 |
| |
|
To check the goodness of fit of the model, the following hypotheses are required.
the model has a good fit versus
the model has lack of fitTable 3 shows the Pearson Chi-square of 251.7801 and deviance of 190.8718 both on 27 degree of freedom following the critical value of chi-square distribution
with 27 degree of freedom with 40.113 at 5% level of significance which is Pearson Chi-Square and deviance value are greater than the critical value of 40.113. However, the assumption of equal variance to the mean in Poisson distribution has been violated since the dispersion parameter is not approximately equal to 1. The dispersion parameter of the above model is 9.3252 which is far greater than 1, an indication of over dispersion in the data. This means that the parameters of the model have been overestimated and the standard errors have been under estimated which will not give a true reflection of model which could provide appropriate mean number of infants with diarrhea from 2013 to 2015. To address this error, Quasipoisson model and Negative Binomial regression was used to modify the model to nullify the effect of overdispersion in the data and the result is shown in the Table 4.Table 3. Summary Result of Infants infected with Diarrhea (Categorical) Using Poisson Regression 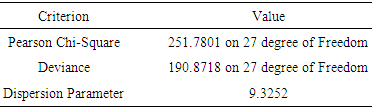 |
| |
|
Table 4 shows that Quasipoisson model maintain the same dispersion parameter with Poisson regression model but changes the standard error and reduced number of significant value compare to Poisson regression model as shown in table 4. It is clear that the Negative Binomial Regression model is actually the best model which fit the numbers of infant infected with diarrhea because the dispersion parameter has reduced from 9.325263 which was given by the Poisson Regression model and Quasipoisson model to 1.2969. Also the Pearson Chi-Square of Negative Binomial Regression is given as 35.0153 compared to the critical value of 40.113 on 27 degree of freedom, which indicate that Negative Binomial Regression Model has a good fit.Table 4. The Parameter estimate of Using Quasipoisson Model for numbers of infant infected diarrhea 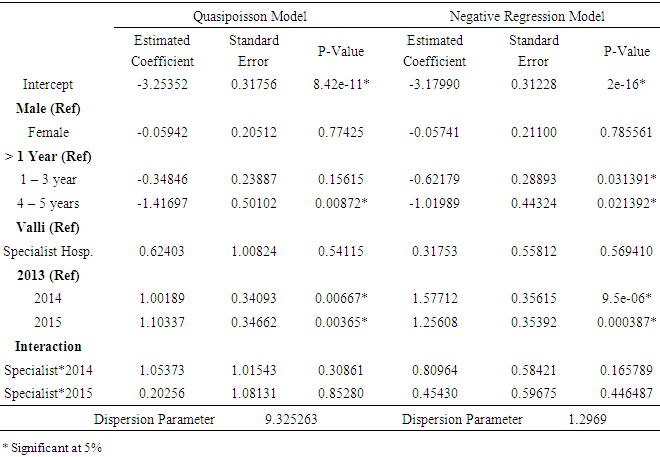 |
| |
|
3.1. Interpretation of Coefficient
From table 4, it is noted that the risk factors age categorized into 1-3 years, age 5-4 years, year 2014 and 2015 are significant at α=5% with their respective significance values equal to 0.031391, 0.021392, 9.5e-06 and 0.000387. The odd that female children will suffer from diarrhea is about
times that of males, indicating that the males are more likely to suffer from diarrhea than female. Children under 1 year seem to be the most hit from diarrhea than those between 1-3 years and 4-5 years which are
and
times as likely to have diarrhea as those under 1 year. Clearly, the chance of being affected with diarrhea reduces as the babies advance in age. For Valli Clinic compared to Specialist hospital has odds of
, which indicate that patient infected with diarrhea visited Specialist Hospital 37% more than Valli Clinic. For year 2013 compared to 2014 and 2015 recording odds of
and
which are greater than that of 2013, indicating that patient with diarrhea increased in 2014 and 2015 but there was a slight reduction in 2015 compared to that of 2014.
4. Conclusions
The Poisson Regression did not accurately fit the data on diarrhea due to the overdispersion effect. The Negative Binomial Model give a better fit. From this, it can be said that diarrhea is still a prevalent disease amongst children below 5 years. Younger babies suffer more. In the year 2013-2015, Adamawa State has recorded high incidence and prevalence of the disease among infants; as such diarrhea is still a critical morbidity issue in the State especially among male children.
References
[1] | Andu, R., Omilabu, S.A., Peenze, I. and Steele, D. (2002). Viral diarrhea in young children in two districts of Africa. Central African Journal for Medicine, 48: 59- 63. |
[2] | Agresti A. (2002). “Categorical Data Analysis” 2nd Edition, John Wiley and Sons, Inc, Publication. |
[3] | Desalegn, M., Kumie, A. and Tefera, W. (2011) Predictors of under-five childhood diarrhea: Mecha District, West Gojjam, Ethiopia. Ethiopian Journal of Health Development, 25, 174-232. |
[4] | McCullagh, P. and Nelder J.A. (1989). Generalized Linear Models. 2nd Edition. London: Chapman & Hall. |
[5] | Oyinloye, S.O., Jatau, E.D., Aminu, M. and Ella, E.E. (2016). A Preliminary Survey of Norovirus and Astrovirus Antigen in Diarrheic Stools of Children in Borno State Nigeria. Global Journal of Medical Research: C. Microbiology and Pathology. Volume 16 issue 1 version 1.0 year 2016. Global Journal Inc. (USA) |
[6] | Tobin, E.A., Isah, E.C. and Asogun, D.A. (2014). Care Giver Knowledge about Chilldhood Diarrheal Managemenr in a Rural Community in South-South Nigeria. International Journal of Community Research. http://www.arpjournals.com. |
[7] | UNICEF/WHO (2009). Why are children still dying and what can be done. Vol. 11. UNICEF/WHO Press, New York, USA. http://www.unicef.org/health/index_51412.html. |