El Hachloufi Mostafa, El Haddad Mohammed
Faculty of Law, Economics and Social Sciences, University of Mohamed IV, Rabat, Morocco
Correspondence to: El Hachloufi Mostafa, Faculty of Law, Economics and Social Sciences, University of Mohamed IV, Rabat, Morocco.
Email: |  |
Copyright © 2016 Scientific & Academic Publishing. All Rights Reserved.
This work is licensed under the Creative Commons Attribution International License (CC BY).
http://creativecommons.org/licenses/by/4.0/

Abstract
In this work we present a mathematical modeling approach to exchange rate risk using the stochastic model of Vasicek. The risk measure used in this modeling approach is the conditional value at risk CVaR. The objective of this approach is to provide a tool of decision for the exchange market managers.
Keywords:
Exchange rates, Risk, CVaR, Vasicek Stochastic model
Cite this paper: El Hachloufi Mostafa, El Haddad Mohammed, Modeling of Exchange Rate Risk, American Journal of Mathematics and Statistics, Vol. 6 No. 6, 2016, pp. 233-237. doi: 10.5923/j.ajms.20160606.02.
1. Introduction
The exchange rate is very important macroeconomic variable that plays a crucial role in international trade. Indeed, the change of the exchange rate affects the terms of trade, it allows modification and adjustment of prices in the different sectors of tradables and non-tradables.Thus, exchange rate fluctuations constitute an area of research that requires considerable effort at modeling. It aims to establish a model to study the convergence of the exchange rate towards equilibrium.In another, the modeling and the evaluation of exchange rate risk is a very important task for managers in the field of foreign trade.The organization of this work is as follows: In Section 1 we present the stochastic model Vasick. Then, value at risk conditional of exchange rate is presented in section 3.
2. Stochastic Model of Exchange Rate Vasicek
The exchange rate can be modeled by several stochastic models, among them there are stochastic processes that tend to return to a moderate level in more or less long term that are used to model the exchange rates of some currencies.In this context fits the stochastic process Vasick or the process of returning to the mean.Thereby, more the fluctuations of
is around to its mean of long term is low, more the volatility of
is low.The model of exchange rate of Vasicek is given by the following equation: | (1) |
Where: Ø
is the speed of exchange rate of return to the mean;Ø
is the average exchange rate.Ø
is the exchange rate volatility which is assumed independentØ
is a Brownian motion as
with
Thus we can develop this model as follows:
In other,
So we get:
So the exchange rate
according to this model can be expressed as follows: | (2) |
Knowing that
is a Brownian motion as
with
then
follows the normal law and thereafter variable
also follows the normal law.As the term
is not a random term then
is a random variable that follows the normal law.By using Iso isometry [2] it is found that:
Then the mean and variance are respectively:Ø
Ø
Thus the random variable follows the normal distribution with mean and variance respectively:
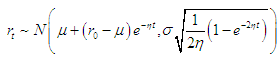 | (3) |
3. Modeling of Exchange Rate Risk
Value at risk VaR is a risk measure proposed by JP Morgan in 1994. It represents the maximum loss that may arise in a portfolio over
for a given level of probability
i.e : | (4) |
Where
with:Ø
: Portfolio value at beginning of the period.Ø
: Portfolio value by the end of the period.Value at Risk depends on three parameters:Ø The distribution of the change in portfolio valueØ The probability that the losses are less than the VaRØ The horizon for which VaR is calculated.VaR offers several advantages such as ease of comparison and interpretation.However, studies such as Szergõ [9] showed that VaR does not take into account the amount of losses exceeding the VaR. Thus VaR is not sub-additive, it means that diversification does not imply a reduced risk.To overcome the limitations of VaR, a new risk measure called the conditional VaR (CVaR), defined as the expected loss exceeding the VaR can be adopted.This is the average value of losses that exceed VaR. CVaR is expressed as follows: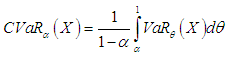 | (5) |
The nominal exchange rate (NER) is the amount of domestic currency per unit of currency of a foreign country. If TCN = 10.5 DH / EUR then it means that the buyer must pay to have a 10.5 DH /EURO. Thus it should be noted that exchange offices must display two NER: one for the purchase of currency and the other for sales.Exchange rate risk or currency risk occurs when a change in exchange rates negatively affect the cash flows of a company following a trade with the outside. The exchange rate risk measurement can be performed by VaR.The calculation of VaR to the exchange rate time horizon is given by the following equation:
as
Then
Then  | (6) |
Thus, in the case of normal distribution was in:
Put
then
Gold
where
with
and
are the mean and variance respectively
Let
it comes :
where
For calculating
we know successively:
It follows
then
where
hence the following result:
Replace
and
expressions in formula previous. So we get:
Thus  | (7) |
4. Numerical Application
In this part we will present an example of a numerical application of the previous formula of CVaR.
Consider a sample of 28 exchange rate DH / EUR of February month 2015 from which we calculate the CVaR The results obtained are given in the following table:
5. Conclusions
In this paper, we developed a mathematical formula to express the conditional value at risk CVaR exchange rate using the Vasick stochastic model.This formula allows to assess the risk of exchange rate, thereby providing a tool for decision support to the exchange market managers.
References
[1] | Abdelhak Zoglat, Elhadj Ezzahid, Elhaouari Bouziani and Younés Mouatassim, A Mean-reverting Model of the Short-term Interbank Interest Rate: the Moroccan Case, Journal of Finance and Investment Analysis, vol. 2, no.3, 2013, 55-67. |
[2] | Bernt Oksendal, Stochastic Differential Equations An Introduction with Applications, Springer, 2003. |
[3] | ÉGERT. B, LOMMATZSCH. K, LAHRÈCHE-RÉVIL. A, (2007) Real Exchange Rates in Small Open OECD and Transition Economies: Comparing Apples with Oranges?, William. |
[4] | M. Elhachloufi, Z. Guennoun, F. Hamza, Stocks Portfolio Optimization Using Classification and Genetic Algorithms, Vol 6, 2012, Applied Mathematical Sciences, no: 94, 4673 – 4684. |
[5] | M. Elhachloufi, Z. Guennoun, F. Hamza, 2012, Minimizing Risk Measure Semi-Variance Using Neural Networks and Genetic Algorithms, Journal of Computational Optimization in Economics and Finance, Volume 4, p:1-12. |
[6] | M. Elhachloufi, Z. Guennoun, F. Hamza , Issue 3 October 2013, Optimization of Stocks Portfolio Using Genetic Algorithms and Value at Risk, International Journal of Mathematics and Computation, Vol. 20, p:30-39. |
[7] | M. Elhachloufi, Z. Guennoun, F. Hamza, 2013, Optimization Stocks Portfolio Optimization using Neural Network and Genetic Algorithm, International Research Journal of Finance and Economics, Issue 104 , p:119-129. |
[8] | LAZAER (2010), Parité réelle du Dirham: Approche par le taux de change d’équilibre, Thèse de doctorat national à l’Université Med I-Oujda. |
[9] | Szergö, G. No more VaR,. Journal of Banking & Finance, 26:1247.1252 (2002). Rudd et Rosenbeg (1979), Realistic Portfolio Optimisation. TIMS Studies in the Management Science, 11, p.21-46. |