Alkreemawi Walaa Khazal1, 2, Wang Xiang Jun1
1School of Mathematics and Statistics, Huazhong University of Science and Technology, Wuhan, China
2Department of Mathematics, College of Science, Basra University, Basra, Iraq
Correspondence to: Alkreemawi Walaa Khazal, School of Mathematics and Statistics, Huazhong University of Science and Technology, Wuhan, China.
Email: |  |
Copyright © 2016 Scientific & Academic Publishing. All Rights Reserved.
This work is licensed under the Creative Commons Attribution International License (CC BY).
http://creativecommons.org/licenses/by/4.0/

Abstract
A stochastic differential equation (SDE) defines N independent stochastic processes
The drift term depends on the random variable
. The distribution of the random effect
depends on unknown parameters, which are to be estimated from continuous observation of the processes
. When the drift term is defined linearly on the random effect
(additive random effect) and
has a Gaussian mixture distribution, we obtain an expression of the exact likelihood. When the number of components is known, we prove the consistency of the maximum likelihood estimators
. The convergence of the EM algorithm described when the algorithm is used to compute
.
Keywords:
Maximum likelihood estimator, Mixed effects stochastic differential equations, Consistency, EM algorithm, Mixture distribution
Cite this paper: Alkreemawi Walaa Khazal, Wang Xiang Jun, Consistency of Estimators in Mixtures of Stochastic Differential Equations with Additive Random Effects, American Journal of Mathematics and Statistics, Vol. 6 No. 4, 2016, pp. 162-169. doi: 10.5923/j.ajms.20160604.04.
1. Introduction
A mixture model (MM) is beneficial for modeling data as output from one of several groups, clusters, or classes; the groups (clusters, classes) might be different from each other, but the observations within the same group are similar to each other. In this paper, we concentrate on the classification problem of longitudinal data modeled by a stochastic differential equation (SDE) with random effects that have a mixture of Gaussian distributions. Some researchers state that the classes are known, whereas other researchers state the opposite. Arribas-Gil et al. [1] and references therein assumed that the classes are known and deal with classification drawbacks of longitudinal data by using random effects models or mixed-effects models. Their aim is to establish a classification rule of longitudinal profiles (curves) into a number that enables dissimilar classes to predict the class of a new individual. Celeux et al. [6] and Delattre et al. [9] assumed that the numbers of classes are unknown. Celeux et al. [6] used maximum likelihood with the EM algorithm to estimate the random effects within a mixture of linear regression models that include random effects (see, Dempster, A. et. al. [10]), and they used Bayesian information criterion (BIC) to select the number of components. Delattreet al. [9] used maximum likelihood to estimate the random effects in SDE with multiplicative random effect in the drift and diffusion terms without random effects with the EM algorithm (Dempster, A. et. al. [10]). They also used BIC to select the number of components. Delattre et al. [9] studied SDEs with the following form: | (1) |
where
are
independent Wiener processes,
are
independently and identically distributed
random variables. The processes
are also independent on random variables
, and
is a known real value. The drift function
is a known function defined on
and the functions
. Each process
represents an individual, and the random variable
represents the random effect of individual i.Delattre et al. [2] considered the special case (multiple case) where
is linear in
; in other words,
, where
is a known real function, and
has a Gaussian mixture distribution.Here, we consider functional data modeled by a SDE with drift term
depending on random effects where
is linear in random effects
(addition case),
and diffusion term without random effects. We consider continuous observations
with a given
. Here,
are unknown parameters in the distribution of
from the
which will be estimated, but the estimation is not straightforward. Generally, the exact likelihood is not explicit. Maximum likelihood estimation in SDE with random effects has been studied in a few papers (Ditlevsen and De Gaetano, 2005 [11]; Donnet and Samson, 2008 [12]; Delattre et al. (2013) [8]; Alkreemawi et al. ([2], [3]); Alsukaini et al. ([4], [5]). In this paper, we assume that the random variables
have a common distribution with density
for all
, which is given by a mixture of Gaussian distributions; this mixture distribution models the classes. We aim to estimate unknown parameters and study the proportions.
is a density with respect to a dominant measure on
, where
is the real line, and
is the dimension.
with
as the proportions of the mixture
,
as the number of components in the mixture, and
and
a
as an invertible covariance matrix. Let
denote the true value of the parameter. M is the number of components and is known.
is set for the unknown parameters to be estimated. Our aim is to find estimators of the parameters
of the density of the random effects from the observations
. We focus on an additional case of linear random effects
in the drift term
. In addition, we prove and explain that the observations concerning exact likelihood are explicit. With M as the number of known components, we discuss the convergence of the EM algorithm, and the consistency of the exact maximum likelihood estimator is proven. The rest of this paper is organized as follows: Section 2 contains the notation and assumptions, and we present the formula of the exact likelihood. In Section 3, we describe the EM algorithm and discuss its convergence. In Section 4, the consistency of the exact maximum likelihood estimator is proved when the number of components is known.
2. Notations and Assumptions
Consider the stochastic processes
, which are defined by (1). The processes
and the random variables
are defined on the probability space
. We use the assumptions (H1, H2, and H3) in Delattre et al. [9]. Consider the filtration
defined by
.H1. The functions
and
are Lipschitz continuous on
and
is Hölder continuous with exponent
on
.By (H1), for
, for all
, the stochastic differential equation  | (2) |
admits a unique solution process
adapted to the filtration
, Moreover, the stochastic differential equation (1) admits a unique strong solution adapted to
such that the joint process
is strong Markov and the conditional distribution of
given
is identical to the distribution of (2). (1) shows that the Markov property of
is straightforward as the two-dimensional SDE
The processes
are
(see Delattre et al. [8]; Genon-Catalot and Larédo [13], Alkreemawi et al. [2], [3]; Alsukaini et al. [4], [5]). To derive the likelihood function of our observations, under (H1), we introduce the distribution
on
given by (2), where
denotes the space of real continuous functions
defined on
endowed with the
associated with the topology of uniform convergence on
. On
, let
denote the joint distribution of
, and let
denote the marginal distribution of
on
. Also, we denote
,
(resp.
) the distribution
of
where
has a distribution
(resp. of
), with these notations | (3) |
H2. For all
,
We denote by
, with
, which is the canonical process of
. Under (H1)–(H2), based on Theorem 7.19 p. 294 in [14], the distributions
and
are equivalent. Through an analog approach of [9], the following results are used:
where
is the vector, and
is the
matrix  | (4) |
and
is the
matrix 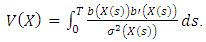 | (5) |
Thus, the density of
(the distribution of
on
) with respect to
is obtained as follows: | (6) |
The exact likelihood of
is | (7) |
Thus, for our situation (addition situation) of drift where
is linear in
,
, we have
and,  | (8) |
where  | (9) |
 | (10) |
We have to consider distributions for
such that the integral (8) can obtain a tractable formula for the exact likelihood. This is the case when
has a Gaussian distribution and the drift term
is linear in
,
(addition situation), as shown in Alkreemawi et al. [2]. This is also the case for the larger class of Gaussian mixtures. The required assumption is defined as follows:H3. The matrix
is positive definite
and
for all
.(H3) is not true when the functions
and
are not linearly independent. Thus, (H3) can ensure a well-defined dimension of the vector
.Proposition 2.1. Assume that
is a Gaussian mixture distribution, and set
,
,
,
,
. Under (H3), the matrices
are invertible
and
for all
. By setting
, we obtain,  | (11) |
where 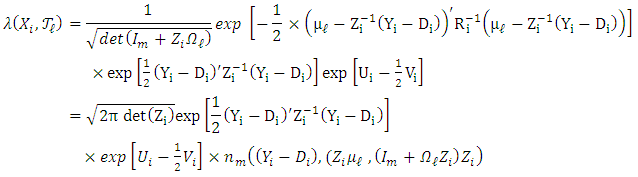 | (12) |
Here,
denotes the Gaussian density with mean
and covariance matrix
.Alkreemawi et al. [2] considered the formula for
(Proposition 3.1.1 and Lemma 4.2). The exact likelihood (7) is explicit. Hence, we can study the asymptotic properties of the exact MLE, which can be computed by using the EM algorithm instead of maximizing the likelihood.
3. Estimation Algorithm
In the situation of mixtures distributions with number of components M, M is known, rather than of solving the likelihood equation, we use the EM algorithm to find a stationary point of the log-likelihood. A Gaussian mixture model (GMM) is helpful for modeling
by using a mixture of distributions, which means that the population of individuals is grouped in M clusters. Formally, for the individual i, we (may) introduce a random variable
, with
and
. We assume that
are
and
independent of
. The concept of the EM algorithm was presented in Dempster et al. [10] which considered the data
as incomplete and introduced the unobserved variables
. Simply, in the algorithm, we can consider random variables
where
, for
; such values indicate that the density component drives the equation of subject i. For the complete data
, the logarithm likelihood function is explicitly given by  | (13) |
The EM algorithm is an iterative method in which the iteration alternates between performing an expectation (E) step, which is the computation of
where
is the conditional expectation given
computed with the distribution of the complete data under the value
of the parameter, and the maximization (M) step computes parameters that maximize the expected log-likelihood found on the (E) step
. In the (E) step, we compute
where
is the posterior probability | (14) |
In the EM algorithm, at iteration n, we want to maximize
with respect to
, where
is the current value of parameter
. We can maximize the terms that contain
and
separately. We introduce one Lagrange multiplier
to maximize with respect to
with the constraint
and solve the following equation:
And the classical solution:
Then, we maximize
, where the derivatives can be computed with respect to the components of
and
by using some results from matrix algebra. When taking the log of
, substituting it into
, and taking the derivative w.r.t.
, we have | (15) |
When the
are known and when the
are unknown, the maximum likelihood estimators of the parameters are given by the system.Proposition 3.1 The sequence
generated by the EM algorithm converges to a stationary point of the likelihood.Proof. We prove the convergence for
to avoid cumbersome details. We employ the results obtained by McLachlan and Krishnan [15]. As the following conditions are given:
Conditions 3, 4, 5, and 6 are verified by the regularity of the likelihood (see Proposition 4.2). In a standard Gaussian mixture, condition 2 is usually unverified (see McLachlan and Krishnan [15]. However, here, one has the following result (see (12)):
Where
. Therefore, the formula of
is a mixture of Gaussian distributions that consist of variances all bounded from below. This finding reveals condition 2.
4. Asymptotic Properties of MLE
This section aims to investigate theoretically the consistency and asymptotic normality of the exact maximum likelihood estimator of
when we assume that the number of components M is known. For simplicity’s sake, we consider only the case
. The parameter set
is given by
Now, we set
, but only
parameters need to be estimated. When necessary in notations, we set
. The MLE is defined as any solution of
where
is defined by (7)–(11). To prove the identifiability property, the following assumption is required as in Alkreemawi et al. [2]:(H4) Either the function
is constant or not constant, and under
, the random variable
admits a density
with respect to the Lebesgue measure on
, which is jointly continuous and positive on an open ball of
.When
is constant, this case is simple. For instance, let
. Then,
is deterministic, and under
. Under
is a mixture of Gaussian distributions with means
, variances
, and proportions
.The case where
is not constant. Under smoothness assumptions on functions
, assumption (H4) will be accomplished by using Malliavin calculus tools (see Alkreemawi et al. [2]). As mixture distributions are utilized, the identifiability of the entire parameter
can only be obtained in the following concept: | (16) |
Now, we can prove the following:Proposition 4.1. Under (H1)-(H2)-(H4),
implies that
.Proof. First, when
is not constant, we consider two parameters
and
, and aim to prove that
implies
. As
and
depend on
only through the statistics 
with a slight abuse of notation, we set
and | (17) |
Under (H4),
is the density of the distribution of
under
with respect to the density of
under
and
implies
a.e., hence, everywhere on
by the continuity assumption. We deduce that the following equality holds for all 
Let us set
and
We note that
. Thus, such quantities do not depend on
. After reducing to the same denominator, we obtain
The right-hand side is a function of
, whereas the left-hand side is a function of z only. This approach is possible only if
for all
. Therefore,  | (18) |
and the equality of the variances can be obtained by reordering the terms if required. Then, we have for
and a fixed z, | (19) |
Here,
indicates the Gaussian density with mean m and variance
. Analogously, by using the equality (18),
For all fixed
, we therefore have for all
,
Herein, the equality of two mixtures of Gaussian distributions with proportions
, expectations
and
, and the same set of known variances
are given. From the identifiability of Gaussian mixtures, we have the equality
and thus,
.Second, when
is constant, for instance, let
. As noted above, under
and
. Therefore,
is a Gaussian distribution. Under
,
has density
w. r. t. the Lebesgue measure on
. Specifically, the mixture of Gaussian densities can also be deduced based on the identifiability property of Gaussian mixtures.Proposition 4.2. Let
denote the expectation under
. The function
is
on
and 
for all
.The Fisher information matrix can be defined as
for all
. Proof. We use results proved in Alkreemawi el al. [2] (Section 3.1, Lemma 3.1.1, Proposition 3.1.2) to prove this proposition. For all
and all
,
is the distribution of
when
has a Gaussian distribution with parameters
. This idea implies that
for all
. Let
The random variable that has moments of any order under
is bounded, and the following relations hold: | (20) |
 | (21) |
 | (22) |
All derivatives of
are well defined. For
, we have
As for all
, the random variable above is
-integrable and
Moreover, as
, we have
Therefore, 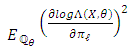
Higher order derivatives of
with respect to the
are nul:
Now we find the derivatives with respect to the parameters
. We have:
We know that: 
Consequently,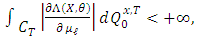
Now,
Thus,
Next, we have
Again, we know that this random variable is
-integrable with nul integral, thereby obtaining
Moreover,
This finding implies that
Now we look at second-order derivatives. The successive derivatives with respect to
with
are nul. We obtain
This random variable is integrable with respect to
with the nul integral. Thus,
We find that this random variable is integrable with respect to
, and computing the integral obtains
Next,
Thus, we conclude the proof analogously using (21) and (22).Proposition 4.3. Assume that
is invertible and (H1)–(H2). Then, an estimator
solves the likelihood estimating equation
with a probability tending to 1 and
in probability.Proof. For weak consistency following the standard steps, the uniformity condition needs to be proven. We prove that An open convex subset
of
exists, which contains
and functions
such that, on S,
.
are set as positive numbers such that
, and
is assumed to belong to
where
. We have to study
Therefore, we have, for
distinct indexes
As
,
We use
again to bound the other third-order derivatives. Then, in the derivatives, random variables appear
for different values of n. We now bound
by an r. v. independent of
and have moments of any order under
. We have
Thus,
Now, in the same method
For all
, this finding implies that
Consequently,
The proof of Proposition 4.3 is complete.
5. Conclusions
In stochastic differential equations based random effects model framework. We considered the addition case in the drift where
is linear in
, where
has a mixture of Gaussian. We obtain an expression of the exact likelihood. When the number of components is known, we prove the consistency of the maximum likelihood estimators (MLEs). Properties of the EM algorithm are described when the algorithm is used to compute MLE.
ACKNOWLEDGEMENTS
The Author is grateful to Dr. Wang Xiang jun of School of Mathematics and Statistics, Huazhong University of Science and Technology, Wuhan, Hubei, 430074 P.R. China. Also, to Mr. Alsukaini M. S. of the department of Mathematics, College of Science, Basra University, Basra, Iraq for their constructive comments.
References
[1] | Arribas-Gil, A., De la Cruz, R., Lebarbier, E. and Meza, C. (2015). Classification of longitudinal data through a semiparametric mixed-effects model based on lasso-type estimators. Biometrics 71, 333–343. |
[2] | Alkreemawi W. K., Alsukaini M. S. and Wang X. J. “On Parameters Estimation in Stochastic Differential Equations with Additive Random Effects,” Journal of Advances in Mathematics, Vol. 11, no.3, 5018 – 5028, 2015. |
[3] | Alkreemawi W. K., Alsukaini M. S. and Wang X. J. “Asymptotic Properties of MLE in Stochastic Differential Equations with Random Effects in the Drift Coefficient,” International Journal of Engineering, Science and Mathematics (IJESM), Vol. 5, Issue. 1, 1 – 14, 2016. |
[4] | Alsukaini M. S., Alkreemawi W. K. and Wang X. J., “Asymptotic Properties of MLE in Stochastic Differential Equations with Random Effects in the Diffusion Coefficient,” International Journal of Contemporary Mathematical Sciences, Vol. 10, no. 6, 275 – 286, 2015. |
[5] | Alsukaini M. S., Alkreemawi W. K. and Wang X. J., “ Maximum likelihood Estimation for Stochastic Differential Equations with two Random Effects in the Diffusion Coefficient,” Journal of Advances in Mathematics, Vol. 11, no.10, 5697 – 5704, 2016. |
[6] | Celeux, G., Martin, O. and Lavergne, C. (2005). Mixture of linear mixed models application to repeated data clustering. Statistical Modelling 5, 243–267. |
[7] | Comte, F., Genon-Catalot, V. and Samson, A. (2013). Nonparametric estimation for stochastic differential equations with random effects. Stoch. Proc. Appl. 123, 2522–2551. |
[8] | Delattre M., Genon Catalot V. and Samson A., “Maximum Likelihood Estimation for Stochastic Differential Equations with Random Effects,” Scandinavian Journal of Statistics, 40, 322-343, 2013. |
[9] | Delattre M., Genon Catalot V. and Samson A., “Mixtures of stochastic differential equations with random effects: application to data clustering,” Journal of statistical planning and inference, Publication MAP5-2015-36. |
[10] | Dempster, A., Laird, N. and Rubin, D. (1977). Maximum likelihood from incomplete data via the EM algorithm. Jr. R. Stat. Soc. B 39, 1–38. |
[11] | Ditlevsen, S. and De Gaetano, A. (2005). Stochastic vs. deterministic uptake of dodecanedioic acid by isolated rat livers. Bull. Math. Biol. 67, 547–561. |
[12] | Donnet, S. and Samson, A. (2008). Parametric infer ence for mixed models defined by stochastic differential equations. ESAIM P&S 12, 196–218. |
[13] | Genon-Catalot, V. and Larédo, C. (2015). Estimation for stochastic differential equations with mixed effects. Hal-00807258 V2. |
[14] | Lipster, R. and Shiryaev, A. (2001). Statistics of random processes I: general theory. Springer. |
[15] | McLachlan, G. and Krishnan, T. (2008). The EM Algorithm and Extensions. |