Mukesh K. Sharma, Sarbjit S. Brar, Harinder Kaur
Department of Statistics, Punjabi University, Patiala, India
Correspondence to: Sarbjit S. Brar, Department of Statistics, Punjabi University, Patiala, India.
Email: |  |
Copyright © 2016 Scientific & Academic Publishing. All Rights Reserved.
This work is licensed under the Creative Commons Attribution International License (CC BY).
http://creativecommons.org/licenses/by/4.0/

Abstract
For estimating the population mode using the known information of population mean
of the auxiliary variable, a class of estimators has been proposed by making use of new parametric relationship for mode developed by Sharma et.al. (2016). We have derived the asymptotic expression for the bias and MSE of any estimators of the proposed class and also obtain the minimum MSE in the proposed class. The results are also illustrated numerically.
Keywords:
Mode, Skewness, Auxiliary variable, Bias and Mean square error, Bivariate normal distribution
Cite this paper: Mukesh K. Sharma, Sarbjit S. Brar, Harinder Kaur, Class of Estimators of Population Mode Using New Parametric Relationship for Mode, American Journal of Mathematics and Statistics, Vol. 6 No. 3, 2016, pp. 103-107. doi: 10.5923/j.ajms.20160603.03.
1. Introduction
In many practical situations, the more appropriate measure of location is mode rather than mean and median. For example, it is useful in finding the ideal size as in studies relating to marketing, trade and industry and also to find the ideal size such as in the manufacturing of shoes or ready-made garments, etc. In addition, the mode is also useful when dealing with skewed distributions such as income, drugs, AIDS etc. The impact of the proposed research is expected to be useful for scientists in Sociology, Psychology, Demography, Business and Economics, where the mode value is routinely being used in practice. Sharma et al (2016) established the new parametric relationship for population mode
as
where
is unknown constant to be determined for the given population. They proposed three estimators of
under the different situations as
and
Where
and
are unknown constants, whose values are determined by minimizing the MSE’s of respective estimators
and
Here the estimator
uses no information on auxiliary variable
which is highly correlated with
whereas
and
uses the known information of
which are of ratio and product type estimators respectively.In the present paper, we propose a class of estimators of population mode using the new parametric relationship for population mode when the population mean
of the auxiliary variable is known. Asymptotic expression for the bias and MSE of any estimators of the proposed class and also their minimum values are obtained upto to terms of order
The results are also illustrated numerically.
2. Notations and Expectations
Let a simple random sample of size
is drawn from a population of size
by using simple random sampling without replacement (SRSWOR). Without loss of generality, we ignore the finite population correction (fpc) throughout the paper.Let
and
be the values of study and auxiliary variables for
unit in the population respectively and the corresponding small letters
and
denote the sample values.Taking,
Obviously
Defining,
For the given SRSWOR, we have the following expectations,
and up to terms of order 
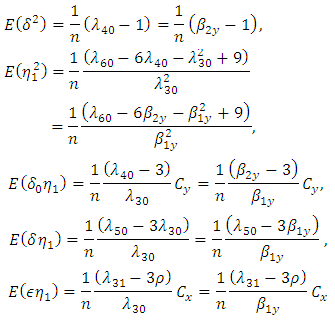
3. Proposed Class of Estimators
In this section, we generalize the mean per unit, ratio-type and product-type estimators of population mode
given by Sharma et al. (2016).Whatever be the sample chosen, let
assume values in a bounded closed convex subset
of the one-dimensional real space containing the value ‘1’. Let
be a function of
such that
and it satisfies the following conditions:(i) The function
is continuous and bounded in
(ii) The first and second order partial derivatives of
exist and are continuous and bounded in
We propose the class of estimator of the population mode
as 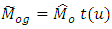 | (3.1) |
To find the biases and of estimators of class
we expand the function
about the value ‘1’ in second-order Taylor’s series, we get
where
and
After writing the above function in terms of
and then taking the expectations given in section 2, upto terms of order
we get, | (3.2) |
 | (3.3) |
where
Here
and
are unknown constants whose values are determined by minimizing
To obtain the minimum value of
we differentiate (3.3) w.r.t.
and
then equating to zero, we get,
and
Solving above quadratic equations for
and
we get two pairs of solutions
and
where,
Clearly, the only feasible pair of values is
Substituting the values of pair
in (3.3), we get,
We can construct the large number of estimators belonging to the proposed class
Here it should be noted that the efficient use of estimators of the proposed class
requires the optimum values of constants
and
which are further functions of unknown population parameters. However, if it is possible to guess accurately the values of such parameters either through past experience or through a pilot sample survey and the value of optimum constants so obtained by using these guessed values of parameters are close enough to the optimum values of constants, then the resulting estimators will be better than the convention estimators. Srivastava and Jhajj (1983) have shown that upto the first order of approximation, the estimators of the class with estimated values of optimum parameters obtained by their respective consistent estimators, attain the same optimum MSE of class based on optimum values upto the first order of approximation. Therefore, the presence of unknown population parameters in optimum values of constants will not create any problem for practical use of the proposed class 
3.1. Special Case of Bivariate Normal Population
Let
then we have 

if
is odd. Also,
and
Using these values, we get,
4. Numerical Illustrations
To illustrate the result numerically, we have made computations for 12 populations taken from literature by using Microsoft Excel 2010.The source of the populations, the nature of the variables, the values of
and
are listed in Table 1.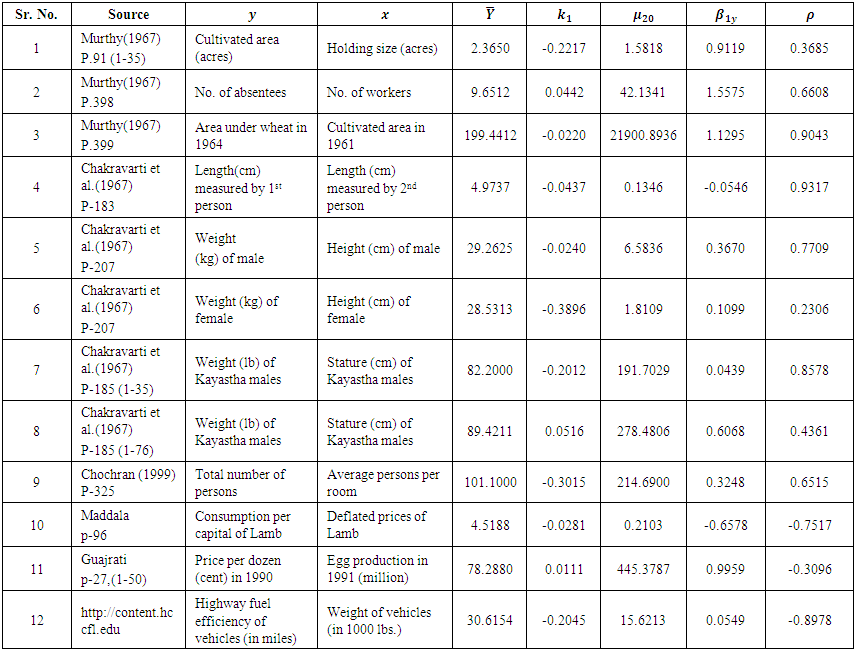 | Table 1. Description of populations |
The efficiencies of proposed estimators are given in Table 2.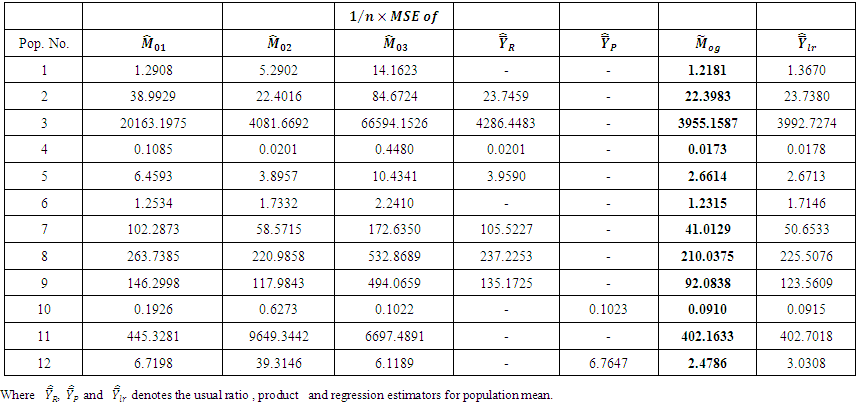 | Table 2. MSE’s of up to terms of  |
From Table 2, it has been clarified that upto terms of order
is less than
and
which implies that the proposed estimator is more efficient than the existing ones. Further,
is also smaller than 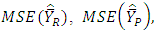
which shows that at optimum value of
the proposed class estimators is more stable than the
and
5. Conclusions
A general class of estimators of population mode has been proposed and the lower bound for the MSE has been obtained for the class of estimators. Further, the empirical study shows that
is less than 

which implies that the optimum estimator is more stable than
and 
References
[1] | Chakravarty, I. M., Laha, R. G., & Roy, J. (1967). Handbook of Methods of Applied Statistics: Techniques of Computation, Descriptive Methods, and Statistical Inference. John Wiley & Sons. |
[2] | Cochran, W. G. (1999). Sampling Techniques (Vol.3). John Wiley & Sons. |
[3] | Gujarati, D. N. (2004). Basic Econometrics. Mc. Graw Hills Pub. Co, New York. |
[4] | Kuk, A. Y., & Mak, T. K. (1989). Median estimation in the presence of auxiliary information. Journal of the Royal Statistical Society. Series B (Methodological), 261-269. |
[5] | Maddala, G. S., & Lahiri, K. (1992). Introduction to econometrics (Vol. 2). New York: Macmillan. |
[6] | Sedory, S. A., & Singh, S. (2014). Estimation of mode using auxiliary information. Communications in Statistics- Simulation and Computation, 43(10), 2390-2402. |
[7] | Sharma, M.K., Brar, S.S. & Kaur, H. (2016). Estimators of population mode using new parametric relationship mode. International Journal of Probability and Statistics, 5(1), 18-24. |
[8] | Srivastava, S.K. & Jhajj, H.S. (1983). A class of estimators of the population mean using multi-auxiliary information. Cal. Stat. Assoc. Bull., 32, 47-56. |
[9] | Murthy, M. N. (1967). Sampling theory and methods. Sampling theory and methods. |