Janardan Mahanta1, Soma Chowdhury Biswas1, Manindra Kumar Roy2, M. Ataharul Islam3
1Department of Statistics, University of Chittagong
2Department of Statistics, Mawlana Bhasani Science and Technology University, Tangail
3Department of Applied Statistics, East West University, Dhaka, Bangladesh
Correspondence to: Janardan Mahanta, Department of Statistics, University of Chittagong.
Email: |  |
Copyright © 2015 Scientific & Academic Publishing. All Rights Reserved.
Abstract
Inferential Statistics is the main subject of statistics. Classical and non-classical are the two types of approaches of estimation. In Bayesian estimation selection of appropriate loss function and prior density are very important. Jeffery’s non-informative prior and squared error loss function were used. Lindley approximation was used to solve the Bayesian integral. The proposed procedure is applied to a longitudinal data on pregnancy complication in rural Bangladesh collected from the Bangladesh Institute of Research for Promotion of Essential and Reproductive Health and Technologies (BIRPERHT). In this study, we have conducted Markov model by maximum likelihood and Bayesian approach and compare them above the approaches. Bayesian approach of estimation found to be better than the classical approach in this particular case.
Keywords:
BSE, Credible interval, MLE, T.K.
Cite this paper: Janardan Mahanta, Soma Chowdhury Biswas, Manindra Kumar Roy, M. Ataharul Islam, A Comparison of Bayesian and Classical Approach for Estimating Markov Based Logistic Model, American Journal of Mathematics and Statistics, Vol. 5 No. 4, 2015, pp. 178-183. doi: 10.5923/j.ajms.20150504.02.
1. Introduction
Now-a-days Bayesian approach is widely used for decision-making. In this paper, we have applied Bayesian approach in Markov chain based logistic model. Markov chain models can be used in analyzing longitudinal data. There is several discrete time Markov chain models proposed for analyzing repeated categorical data over decades. To analyze the binary sequence of presence or absence of diseases, Muenz and Rubinstein [10] introduced a discrete time Markov chain for expressing the transition probabilities in terms of covariates. The technique suggests by them is applicable for first order Markov model. For analyzing sequences of ordinal data from relapsing and remitting of a disease, Albert [1] developed a finite Markov chain model. In addition, Albert and Waclawiw [2] developed a class of quasilikelihood models for a two state Markov chain with stationary transition probabilities for heterogeneous transitional data. Raftery [14], Raftery and Tavare [13] proposed a higher order Markov chain model with dependence on contribution of the past transitions. Islam and Chowdhury [8] applied a three state Markov model for analyzing covariate dependence, also Islam and Chowdhury [7] presented a higher order version of the covariate dependent Markov model. For analyzing repeated observations, there is a renewed interest in the development of multivariate models based on Markov chains. These models can be employed for analyzing data generated from meteorology, epidemiology and survival analysis, reliability, econometric analysis, biological concerns, etc. Muenz and Rubinstein [10] employed logistic regression models to analyze the transition probabilities from one state to another. The estimation for first-order Markov models is quite straight forward, but still there is serious lack of generalization in estimation and testing for models applicability for higher order Markov chains. Islam and Chowdhury [7] provided a further generalization for covariate dependent higher order models. Following Azzalini [3], Heagerty and Zeger [5] presented a class of marginalized transition models (MTM) and Heagerty [4] proposed a class of generalized MTMs to allow serial dependence of first or higher order. These models are computationally tedious and the form of serial dependence is quite restricted. Heagerty [4] provided derivatives for score and information computations. Muenz and Rubinstein [10] introduced a discrete time Markov chain for expressing the transition probabilities in terms of function of covariates for a binary sequence of presence or absence of a disease. Islam, Chowdhury and Singh [6] suggest covariate dependence in a higher order Markov models is examined. The proposed model and inference procedures are simple and the covariate dependence of the transition probabilities of any order can be examined without making the underlying model complex. Another advantage of the model lies in the fact that the estimation and test procedures for both the specific parameter of interest and the overall model remain easy for practical applications for any longitudinal data. A simple alternative is also proposed. Applications are illustrated using maternal morbidity during pregnancy. In this paper, an attempt is made to estimate the probability of being pregnancy complicacies of women according to the characteristics of the women and estimate the parameters by Bayesian approach and method of maximum likelihood approach. This characteristics or variables may often be related to each other. Higher difficulties occur to any miscarriage, economic status and age at marriage in this study.
2. Model
Muenz and Rubinstein [10] in 1985 proposed a two state Markov chain to model a discrete time-binary sequence. The transition matrix of the chain is:
where
denotes the transition probabilities from 0 to 0 and
is the transition probability from 1 to 0. At each time point, a vector of length two contains the probability of outcome of interest and its complement.The first such vector (row) is P(1) = (p (1), 1 –p (1)), with the first element equal to the probability of the complement of the event of interest. The matrix M and initial vector P(1) suffice to characterize the Markov chain. After time point 1, the vector of occupation probabilities is:
Muenz and Rubinstein [10] proposed model the transition probabilities
and
by logistic regressions.
and
The vector X contains covariates and for the qth person in the study is equal to,
. There are two logistic regressions, one having parameter vector
and the other having parameter vector
. Large positive (negative) values of
and
yield large (small) transition probabilities. Since the transition matrix is a function of covariates, P (j) relates the occupation probabilities to the initial state for each pattern of covariates.For transition 0 to 0For binary random variable
with covariate
the joint density function is as
where,
and
are the number of transitions.
3. Bayesian Approach
In this study, we use the Bayesian paradigm to make inferences about parameters of the model [10] for pregnancy complication data.
3.1. Prior and Posterior Distribution
In Bayesian analysis prior and posterior distribution are important. Since the parameters of Markov model of
lies between
to
, then according to Jeffrey’s non-informative prior [15] of
are
. Where, I represent unit vector.Then the posterior density of
for the given sample is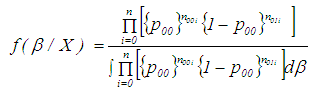
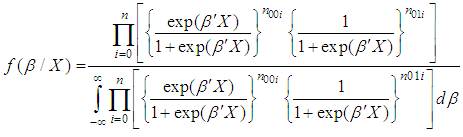
3.2. Bayes Estimators under Squared Error Loss Function
The squared error loss function is defined as
For squared error loss function, Bayes estimators are the mean of the posterior density [11]
The two integrals appear in the ratio cannot be solved to have a closed form. For evaluating them, we use the Lindley [12] approximation.We have,
where,
is the log likelihood and
is the logarithm prior distribution.Since,
then
or,
Then according to Lindley, I(X) can be approximately evaluated as
where,
is the functional form of the parameter
, which is expected to posterior density and
is the maximum likelihood [10] estimator of
.
Now we know that the likelihood function of binary dependence Markov model is
Therefore,
Taking log on both sides
where,
and
are the likelihood function of transition 0 to 0 and 1 to 0 respectively.Now considering
Differentiating both sides with respect to
, we have
Therefore, Bayes estimator under squared error loss function is
where
is the maximum likelihood [10] estimate of
.If
is large then Bayes estimator tends to maximum likelihood estimator.In addition, the same way we estimate the parameters of the transition 1 to 0.
4. Bayesian Credible Interval
If
is the posterior distribution given the sample, we may be interested in finding an interval such that
This interval is called
100% Bayesian credible [9] interval of
.In Bayesian analysis, credible interval becomes the counterpart of the classical confidence interval, also credible interval may be unique for all models. The Bayesian credible interval, on the other hand, has a direct probability interpretation
and is completely determined from the current observed data x and the prior distribution.
5. Numerical Analysis
The covariate dependent Markov model proposed in this paper is applied to the pregnancy complication data conducted from BIRPERHT data for the period November 1992 to December 1993. The data were collected using both cross-sectional and prospective study designs. A multistage sampling design was used for collecting the data for this study. Districts were selected randomly in the first stage, one district from each Division. Then Thanas were selected randomly in the second stage, one Thana from each of the selected Districts. At the third stage, two Unions were selected randomly from each selected Thana. The subjects comprised of pregnant women with less than 6 months duration in the selected Unions. All the selected pregnant women from the selected Unions were followed on regular basis (roughly at an interval of 1 month) throughout the pregnancy. Again, the subjects were followed at the time of delivery for a full- term pregnancy and 90 days after delivery or 90 days after any other pregnancy outcome. A total of 1059 pregnant women were interviewed in the follow-up component of the study. The following pregnancy complications are considered under the complications in this study: hemorrhage, fits, convulsion, edema, excessive vomiting, and cough or fever for more than three days. If hemorrhage, fits, convulsion, edema, excessive vomiting, and cough or fever for more than three days occurred to the respondents, we considered complications and was coded as 1, if no complications then coded as 0. The explanatory variables are: age at marriage (15 years or lower, more than 15 years), economic status (lower or upper), any miscarriage (yes, no). The number of transitions for the two-state Markov chain of first order is displayed in Table 1.Table 1. Transition counts of Markov chain for first orders of pregnancy complication of different flow-up |
| |
|
Estimate the Muenz-Rubinstein model using pregnancy complication data. In this study, we have used three covariates because of complexity to fit the model. Three highly significant covariates are used in our study. Bayesian approaches have been applied for estimating the parameter of the above model.
5.1. Comparison between Credible Interval and Confidence Interval
Confidence intervals for maximum likelihood estimators and credible intervals of Bayesian estimators under squared error loss function were calculated for Muenz-Rubinstein model and two types of transition are presented in the following table.For 0 to 0 transition Table 2. Confidence interval for maximum likelihood estimators 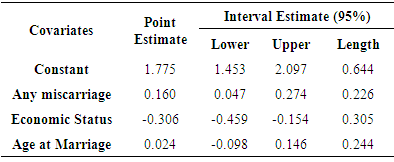 |
| |
|
Table 3. Credible interval for Bayesian estimator under squared error loss function 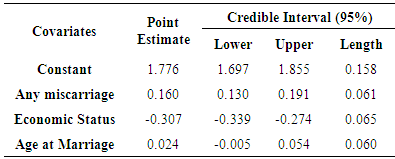 |
| |
|
From the above results, we have found that the length of Bayesian credible is lower than the length of confidence interval for all covariates. Moreover, 1 to 0 transitionsTable 4. Confidence interval for maximum likelihood estimators 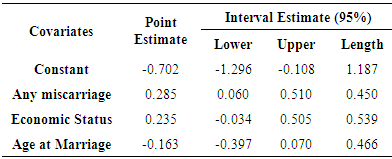 |
| |
|
Table 5. Credible interval for Bayesian estimator under squared error loss function 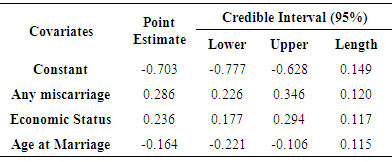 |
| |
|
Therefore, from the above tables it is also seen that, Baysian credible intervals are smaller length than confidence interval in 1 to 0 transitions. Therefore, we can say that Bayesian approach provides better result than usual method maximum approach in Muenz-Rubinstein model. In addition, economic status is negatively associated, any miscarriage and age at marriage are positively associate with pregnancy complication.All the numerical analysis was performed by R-Language (Version-2.10.0).
6. Conclusions
Markov chain is very important part in real world situation. Its application increasing day by day, in this study we applied pregnancy complication data and only three covariates were used because complexity of the model. Meanwhile different types of approach were used for estimating the parameters in this model. In Bayesian approach under squared error of loss function were used and compare with method of maximum likelihood and we have found that length of Bayesian credible interval is smaller than length of confidence interval for all covariates in two types of transitions. From the above analysis, we conclude that Bayes estimators under squared error loss function give better result in Muenz Rubinstein model. An application is included in this paper to illustrate the use of the proposed model for real life problems.
References
[1] | Albert, P. S. (1994). A Markov model for sequence of ordinal data from a relapsing remitting disease. Biometrics, 50, 51-60. |
[2] | Albert, P. S., & Waclawiw, M. A. (1998). A two state Markov chain for heterogeneous transitional data: A quasilikelihood approach. Statistics in Medicine, 17, 1481-1493. |
[3] | Azzalini, A. (1994). Logistic regression for autocorrelated data with application to repeated measures. Biometrika, 81, 767-775. |
[4] | Heagerty, P. J. (2002). Marginalized transition models and likelihood inference for longitudinal categorical data. Biometrics, 58, 342-351. |
[5] | Heagerty, P. J., & Zeger, S. L. (2000). Marginalized multi-level models and likelihood inference (with Discussion). Statistical Science, 15, 1-26. |
[6] | Islam, M. A., Chowdhury, R.I., & Singh, K. P. (2008). Covariate Dependent Markov Models for Analysis of Repeated Binary Outcomes. Journal of Modern Applied Statistical Methods, 6(2), 561-572. |
[7] | Islam, M. A., & Chowdhury, R. I. (2006). A higher-order Markov model for analyzing covariate dependence. Applied Mathematical Modelling, 30, 477-488. |
[8] | Islam, M. A., & Chowdhury, R. I. (2004). A three state Markov model for analyzing covariate dependence. International Journal of Statistical Sciences, 3, 241-249. |
[9] | Kazmi, S. M., Aslam, M., & Ali, S. (2012). Preference of prior for the class of life-tome distributions under different loss functions. Pak. J. Statist, 28(4), 467-484. |
[10] | Muenz, L. R., & Rubinstein, L. V. (1985). Markov models for covariate dependence of binary sequences. Biometrics, 41, 91-101. |
[11] | Podder, C. K., & Roy, M. K. (2003). Bayesian estimation of the parameter of Maxwell distribution under MLINEX loss function. Journal of Statistical Studies, 23, 11-16. |
[12] | Press, S. J. (1989). Bayesian Statistics: Principles, Models, and Applications. New York: John Wiley & Sons. |
[13] | Raftery, A., & Tavare, S. (1994). Estimating and modeling repeated patterns in higher order Markov chains with the mixture transition distribution model. Applied Statistics, 43(1), 179-199. |
[14] | Raftery, A. E. (1985). A model for higher order Markov chains. Journal of Royal Statistical Society B, 47, 1528-39. |
[15] | Smith, G. P. (n.d.). Expressing Prior Ignorance of a Probability Parameter. Notes, University of Missouri http://www.stats.org.uk/priors/noninformative/Smith.pdf on informative priors. |