Charles Dwumfour Osei 1, Richard Tawiah 2, Yaw Osei-Boadu 3
1Department of Economics and Entrepreneurship Development, University for Development Studies, Tamale, Ghana
2Department of Mathematics, Kwame Nkrumah University of Science and Technology, Kumasi, Ghana
3Department of Mathematics Education, Valley View University, Techiman Campus, Ghana
Correspondence to: Richard Tawiah , Department of Mathematics, Kwame Nkrumah University of Science and Technology, Kumasi, Ghana.
Email: |  |
Copyright © 2014 Scientific & Academic Publishing. All Rights Reserved.
Abstract
This paper investigates the relationship between rural household poverty and farmers’ choice of child labour in the cocoa sector. In line with this objective, the multinomial logit model was fitted to data gathered from 150 farmers residing in different communities in the Sefwi Wiawso Municipality in Ghana. The model explained a moderate proportion of the total variability in the data (thus, Negelkerke Pseudo R – square= 0.634; Cox and Snell Pseudo R- Square= 0.572). Our results revealed that, farmers’ decision on child labour is as a response to the grinding poverty experienced in the rural areas. This study demonstrates that productivity of farm land and quality of house are useful proxies for wealth. The productivity of farm land positively affects farmers’ choice of farm work and schooling combination but negatively related to their choice of using children in exclusive farm work. Moreover, the quality of household negatively affects farmers’ choice of exclusive farm work but positively affect their choice of exclusive schooling. The study recommends that, any policy that is geared towards eliminating child labour should be channelled through improvement in the wealth of farmers such as productivity of farm land.
Keywords:
Child Labour, Cocoa, Multinomial Logit, Poverty, Ghana
Cite this paper: Charles Dwumfour Osei , Richard Tawiah , Yaw Osei-Boadu , Analysis of Rural Household Poverty and Farmers’ Decision on Child Labour Nexus Using Multinomial Logit Model, American Journal of Mathematics and Statistics, Vol. 4 No. 6, 2014, pp. 248-253. doi: 10.5923/j.ajms.20140406.02.
1. Introduction
Child labour occurs predominantly in developing countries but, its root cause remains debatable. This subject has received a growing attention globally in particular, due to its high potential risk of militating against the attainment of the Millennium Development Goal 2 which targets the achievement of universal basic education [1, 2]. It is reported that, at approximately 250 million children aged 5-14 years are involved in child labour worldwide [3]. Findings by Fluitman [4] indicate that, child labour is a norm in Asia and Africa accounting for more than 90% of children engaged in mining, farming, quarrying, fishing, manufacturing and many other economic activities worldwide. The author further reported that, even though there is a higher risk of child labour incidence in Africa, there is a greater number of children participating in the labour force in Asia than other parts of the world. Similar studies have also shown that, using children in economic activities is common in Africa [1]. Evidence moreover suggest that, about 30% of these child labourers are found in the sub-Saharan Africa and are engaged mostly in cocoa farming, fishing, trading (‘kayeye’), and quarrying [3]. Correspondingly, report by the Ghana Ministry of Manpower, Youth and Employment indicates that majority of children are employed on cocoa farms and are members of farm households [5]. In that apart, Ghana statistical service (GSS) in their report also revealed that about 60% of children in the rural areas in the western region of the country are engaged in agriculture mostly cocoa farming [6]. A study by Khanam [7] indicated that several factors such as education of farmers, sex of famers, farmers’ income and poverty account for the increase in child labour in cocoa farming and other economic activities. The study concluded that, the higher the farmer’s education, the more likelihood that his children attend school and reduces their participation in farm work.Many previous studies have tried to investigate the relationship between household poverty and farmers’ decision to exploit child labour in farming and many other economic activities with mixed conclusions. For example, studies by Neils-Hugo and Dorte [8], Edmonds [9] and Basu and Van [10] shown that household poverty is the major factor that influences farmers’ decisions on child labour exploitation in the rural areas in most developing countries. They argue that, poor households engage the labour of children in economic activities more than rich households especially in farming communities in most developing countries. However, these findings have been strongly contended and their validity critically questioned by many authors such as Kambhampati and Rajan [11], Dumas [12], Bhalotra and Heady [13] who in their studies also found that, poverty does not influence farmers decision to exploit child labour in their farm work activities. These contrasting findings and mixed conclusions though interesting, indicate that the link between poverty and farmers’ decision to patronize child labour exploitation seems weakly established. Findings on this subject of poverty and farmers’ decision on child labour may also have the likelihood of varying across regions, countries, and even continents.To the best of our knowledge, only few existing studies have tried to establish the link between poverty and farmers’ decision on child labour in Ghana. This study therefore employs the multinomial logit model to examine the link between poverty and farmers’ decision on child labour using more refined explanatory variables such as farm land productivity and house quality as proxies for farmers’ wealth. The study is conducted in the Sefwi Wiawso municipality in the Western region of Ghana. In many studies, to determine whether a household is poor or not depends on their stock of wealth. This is usually measured by households’ possession of physical assets, durable goods, livestock, farm land size, quality of house, household income and expenditure levels among others [14, 15, 13]. For example Nkamleu [15] in his studies in Cote d’ivoire, in testing the ‘’wealth paradox’’ found that, poverty can be a cause of child labour in the rural areas using house quality as proxy for wealth. The author concluded that, households with high quality houses are less likely to engage children in farm work. A study by Bhalotra and Heady [13] also found a positive relationship between household wealth and child labour in Ghana using land size as a proxy for household wealth in the rural communities.However, using land size as proxy for wealth may bias the conclusions for the reason that, a mere land size tells little about the quality of land and its wealth. Therefore, land size alone as proxy for wealth may be risky since it gives partial indication of farmers’ poverty level. It is also possible that farmers’ wealth may constitute non-farm sources in addition to their farm returns therefore the need for more refined variable such as productivity of farm land which tells the quality of the land. The remainder of the paper is organized as follows: section 2 consists of methodology; section 3 presents results and discussions whereas section 4 concludes the paper.
2. Methodology
2.1. Study Area
The study was carried out in the Sefwi Wiawso Municipality in the Western region of Ghana. The Municipality lies in the North-Eastern part of the Western Region of Ghana between latitudes 6N and 6.30N and longitudes 2.45W and 2.15W. It covers an area of 2634Km2 and has a total population of 139,200 with 69,447 females and 69753 being males [16]. The Municipality is within the forest ecological zone of Ghana which usually records a moderate rainfall of 1524mm-1780mm with temperatures 25℃ – 30℃. The major economic activity in the Municipality is agriculture where about 80% of the inhabitants of the Municipality are engaged in this sector. The Municipality has about 13365 cocoa farmers where 18% of them are females. The target population for this study consisted of the household heads who were cocoa farmers in the Municipality. The study employed multistage sampling procedure. In the first stage, a purposive sampling technique was used to select the Sefwi Wiawso Municipality on the basis of the evidence of its economic significance as the leading cocoa producing municipality in Ghana. The next stage was to select communities within the municipality for the study. The list of all the communities was collected from the Municipal planning office. A simple random sampling technique was then used to select five communities within the Municipality for the study. In selecting the respondents from the accessible population, random sampling technique was adopted to select 200 household heads to form the sample size for the study. The main instruments used to collect the data for the study was semi-structured questionnaires, where focus group discussion and personal observations were adopted to achieve the stated objectives. About 200 researcher-designed questionnaires were administered to respondents to collect information for the study. 150 answered questionnaires were retrieved representing 75% while 50 questionnaires could not be retrieved amounting to 25%. The data was first coded in SPSS and later imported into STATA for computational implementations.In this study, we used cocoa farm land productivity and House Quality as the major proxies for wealth and indicators for poverty in addition to commonly used explanatory variables such educational level of farmer, availability of sharecropper, number of children of farmer, sex of farmers.
2.2. Theoretical Model
Cocoa farmers may intuitively decide either to exploit the labour of their children on farming activities only, or allow children to combine farm work and school only or choose to enroll children in school only based on certain factors such as household wealth, education of farmers, farm ownership, number of children, educational level of farmer and age of farmer [17, 18]. Many studies in labour economics have used discrete choice models such as logistic regression models to examine the decision making involving choice of labour by participants in the labour market. Discrete choice models are statistical procedures that studies choices made by people among a finite set of alternatives. A discrete choice model specifies the probability that a person chooses a particular alternative, with the probability expressed as a function of observed variables that relate to the alternatives and the person [19]. Discrete choice models statistically relate the choice made by each person to the attributes of the person and the attributes of the alternatives available to the person [20]. In principle, choice of any decision- maker is made in relation to the perceived probability of the utility to be derived from a particular choice outcome.
2.3. The Random Utility Model
The discrete choice model is based on the principle that the decision-maker chooses the outcome that maximizes the utility. Let decision-maker
choose from a set of mutually exclusive alternatives,
The decision-maker obtains a certain level of utility from each alternative. It is not possible to observe utility gain, but some attributes of the alternatives as faced by the decision-maker can be observed. Hence, the utility is decomposed into deterministic
and random part
which is given by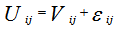 | (1) |
Since
is not observed, the decision maker’s choice cannot be predicted exactly. Instead, the probability of any particular outcome is derived. The unobserved term is treated as random with density
which captures impact of all unobserved factors that affect a person’s choice. In the analysis involving more than two choice set, parametric model known as multinomial logit can suitably be used in practice [21, 19].
2.4. Multinomial Logit Regression Model
Multinomial logit regression is a simple extension of binary logistic regression that allows for more than two choice categories of the dependent variable. Multinomial logit regression is flexible to handle in analysis because it does not assume normality, linearity, or homoscedasticity. Following Green [21] the multinomial logit model for multiple choice problem is specified as 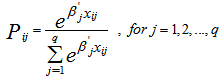 | (2) |
Our study introduced the multiple choice of farmers
into the framework of the multinomial logit model in Equation (2). We defined choice
for farmer as follows
To estimate the multinomial logit model in Equation (2) there is the need to normalize one category which is referred to as the “reference state”. Following our approach the first choice category “farm work and school only” was normalized. The normalized model is given by
In the expression, the alternative categories of outcomes are represented by
denotes the individual farmer,
denotes the vector parameter whilst
represents a vector of explanatory variables. The
vector contains exogenous factors including farmers’ characteristics such as, sex, education; household characteristics such as number of children, house quality, farm productivity and availability of farm sharecroppers. The coefficients in this model only tell the direction of effect but do not tell anything about the magnitude and the margins of the effect of the explanatory variable on the dependents variable. Therefore the easiest and better way to interpret the results is the use of the marginal effects which gives the magnitude and margins of effect on the dependents variable from the predicted probabilities [21]. The marginal effect is computed from the predicted probabilities of the categorical outcomes. The marginal effect of the multinomial logistic regression model is given, by taking the first order derivative of the equation 2. Then, the marginal effect of the multinomial logit model is given by | (3) |
where
represents probability weighted average of the
[22]. The log likelihood estimation used to evaluate the probability of categorical membership is given by Equation (4) 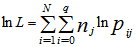 | (4) |
where
is the number of individuals who choose outcome
3. Results and Discussion
Table 1 presents the poverty associated characteristics that influence the farmers’ decision to either exploit the labour of their children in their farming activities or enroll them in school and the probabilities of farmers’ choice. Table 1. Descriptive Statistics of the Variables used in the analysis with total sample size (150) 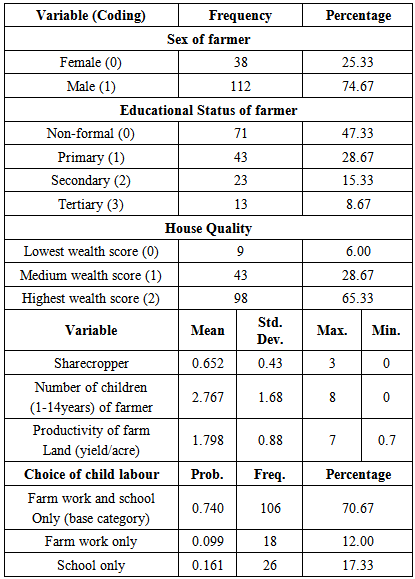 |
| |
|
Out of 150 respondents used in the study, 106 chose to combine their children’s education with cocoa farm work (probability=0.740) representing 70.67%.Also 18 farmers exclusively choose to engage children in cocoa farm work (probability=0.099) representing 12% while 26 farmers allowed their children to participate in school only (probability=161) representing 17.33%. In terms of gender from Table1, male headed households were the majority with 74.67% while female headed household were 25.33%. The reason for the difference can be attributed to the fact that, in the study area, women have limited right to farm land. The table indicates that, cocoa farming in the area was predominantly people with non- formal education representing 47.33% while only few had tertiary education amounting to 8.67%. Majority of the farmers lived in high quality houses (Table 1) categorized as indicator for high wealth score by Living Standard Surveys representing 65.33%. These houses have concrete block walls with iron and aluminium roofing. From the table, only 6% of the respondents were residents in low quality houses with lowest wealth score. These houses are houses with weak mud walls and thatched roofing. Results from the table shows that, the average sharecropper of the respondent was approximately 0.7 while the average number of children of farmers was approximately three (3). Results presented in Table 1 indicate that, the average farm land productivity calculated by yield/acre was approximately 1.798.The model summary as presented in Table 2 shows a log likelihood ratio value of 254.28 and a chi-square of value of 102.31(p-value=0.002). This means that the model is good in predicting the choice of child labour by participant cocoa farmers. Also the Negelkerke Pseudo R - square from Table 2 is 0.634 out of 1.00 for total variation explained in the data used in the model. The Cox and Snell Pseudo R- Square is again given as 0.572 out of 0.750 for the total variation explained in the data used in the model. This implies that, the model explains a greater proportion of the variation in the data used for the study. Table 2. Summary Statistics of the Multinomial Logit for Farmer’s Choice of Child Labour in Cocoa Farming |
| |
|
The multinomial logistic regression results presented in Table 3 show that, productivity of farm land, educational status of farmer, house quality, and availability of sharecropper statistically affect the probability of farmer’s choice for farm work with schooling, farm work only or school only.Table 3. Multinomial Logit Regression of Farmer’s Choice of Child Labour in Cocoa Farming 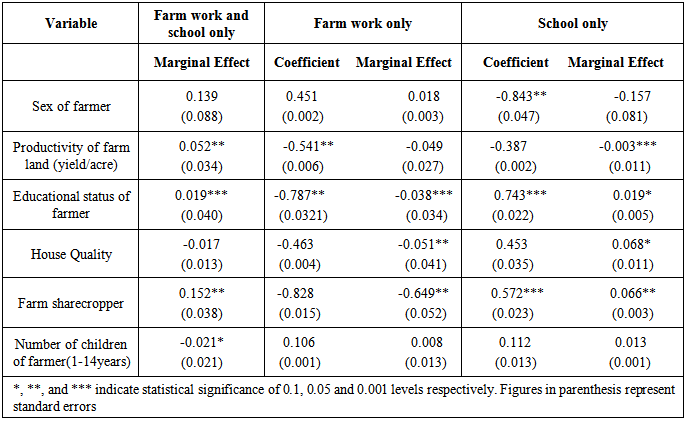 |
| |
|
Results from the table shows that, farm land productivity positively affect the probability of farmer’s choice for farm work with schooling but negatively affect the probability that a farmer chooses farm work only or school only in his decision to use child labour. The results indicate that, a unit increase in the productivity of farm land increases the probability that a farmer combines chooses schooling with farm work activities by 5.2%. The table also indicates that, Farm land productivity is negatively related to child participation in farm work only and school only. For example, an increase in farm land productivity by a unit point reduces the likelihood that a farmer’s child participates in farm work only by 4.9% and that of exclusive schooling by 0.3% compared to the choice of farm work and schooling combination. This implies that, as farmers’ wealth increases, they turn to combine their children’s schooling with farm work whilst reducing their participation in farm work only and exclusive schooling. This may be as a result of increase in marginal returns to child labour on the cocoa farm land and reduction in the opportunity cost of child labour participation in farm work. This is consistent with the finding by Nkamleu [15] who established that, farm land productivity increases child labour but decreases children’s exclusive participation in schooling option.Farmer’s educational level negatively affect the probability that a farmer chooses farm work only but positively affect the probability of framer’s choice for school only compared to farm work and school choice combination. The findings from the table show that, a unit increase in a farmer’s educational status reduces the probability of the farmer’s choice for farm work only by 3.8% while increasing the likelihood of the farmer’s choice for schooling only by1.9% compared to that of farm work and schooling combination alternative. Intuitively, this implies that, the higher a farmer achieves more formal education, the greater the likelihood that he/she chooses to combine the child’s schooling with farm work or allow the child to specialize in schooling. This result confirms that of Khanam [7] who argued that, increase in framer’s level of education increases his likelihood to allow children to specialize in schooling but increases their withdrawal from farm work participation.The findings from Table 3 also reveal that, House Quality affect the probability that a farmer chooses to use the child in farm work only but positively affect the probability of the farmer’s choice for school only compared to that of farm work and schooling combination choice. The results from the table suggests that, as House Quality increases by a unit point, the likelihood that a farmer withdraws the child from exclusive farm work participation increases by 5.1% but increases the likelihood of choosing to enroll the child in school only by 6.8% compared to the choice of farm work with schooling participation. Similarly studies such as Nkamleu [15] found that farmers choosing school only is more likely as the quality of their house improves.The availability of sharecropper negatively affect the probability that a farmer uses the child in farm work only but positively affect the probability of a farmer chooses school only. The results from the table show that a unit increase in the number of sharecroppers available to a household reduces the probability that a farmer chooses farm work only (64.9%) and increase the probability of choosing school only by 6.6%.
4. Conclusions
This current study revisited the link between household poverty and decision of cocoa farmers to employ child labour. Motivated by this, the study employed multinomial logit model to examine poverty and farmers’ decision on child labour participation nexus in a probabilistic framework. The study revealed that, in deciding on child labour participation, the most likely decision of farmer is to combine the child’s schooling with farm work (70.67%), followed by exclusive schooling (17.33%) and farm work only (12%). The study demonstrated that, farm land productivity and house quality are most significant and robust indicators used as proxy for wealth in the cocoa farming communities. We found that, as the wealth of farmers improves (reduction in poverty level), their children are withdrawn from exclusive participation in farm work whilst increasing their likelihood to allow their children to attend school only. This study also shown that, improvements in framers’ wealth directed through productivity of farm land even though reduces children’s participation in exclusive farm work and exclusive schooling, there is a greater probability of increasing children’s participation in farm work with schooling. This may be as a result of increase in marginal returns to child labour on the cocoa farm. The findings also, shown that, House quality as proxy for wealth was the only single and most significant indicator that accommodated the traditionally held assumption that, improvement in the wealth of households reduces child labour in the agrarian communities. It showed a stronger negative relationship between wealth and the likelihood of children’s participation in farm work. The study moreover, indicated that, other factors such as farmer’s education, availability of sharecropper, and number of children of a household influence farmer’s choice of child labour participation in farming activities. In particular, as farmer’s educational level increase, his choice of enrolling the children in exclusive schooling increases whilst the availability of sharecroppers reduces child labour participation in farm work activities. The study therefore concludes that, using standard of living conditions (household quality) as proxy for wealth confirms that poverty might be accountable for child labour in the case of agrarian rural communities especially among cocoa farmers all other things being equal. However, if farm land productivity is used as proxy for wealth, poverty produces mixed marginal effects on child labour in that it reduces likelihood of farmers using their children in farm work only and exclusive schooling only but increases the probability of a farmer combining their children’s schooling with farm work. From these conclusions, it is recommended that, Governments, policy makers and developmental partners should pay much attention to improve the wealth of farmers in the rural areas in eliminating child labour in those areas. This implies that, any policy that is geared towards eliminating child labour should be channeled through improvement in the wealth of farmers such as productivity of farm land. In doing this, the opportunity cost of child labour participation in farming activities such as reduced costs of education, provision of scholarships, bursary, school feeding allowances, and child’s conditional cash transfers should be increased to make the policy successful. Also farmers should be sensitized on the implications of child labour whilst assisting them with less expensive technology as substitute for child labour in farming, and providing them with credit facilities, and other farming implements.
References
[1] | Owusu,V., Addo, G. K. (2008). A Empirical analysis on the determinants of child labour in cocoa production. A paper presntted at PEGNet Conference, 2008 in Accra, Ghana. |
[2] | UNICEF (2007). child labour, education and policy options, Division of polcy and planning. Working paper, NY, USA. |
[3] | International Labour Organization (2008). Tackling child labour from commitment to action. International programme on thte Elimination of Child Labour. ISBN978- 92-2-126374-6. |
[4] | Fluitman, F., (2001). “Working but not well’’. Notes on the nature and extent of employment problems in sub-Saharan Africa. Occasional paper. International training centre of international labour organization in Turin, Italy. |
[5] | Ministry of Manpower, Youth and Employment (2008). Pilot survey in cocoa production in Ghana: National program for elimination of child labour in Agriculture. Annual report manual. |
[6] | Ghana Statistical Service (2003). Report on child labour in Agriculture in Ghana. |
[7] | Khanam, R., (2006). Child labour and school attaendance: Evidence from Bangladesh. MPRA paper no.6990. |
[8] | Neils–Hugo, B. and Dorte, V (2000). Link between poverty and child labour: the Ghana experience. Research report presented in world Bank summit in October, 4, 2000. |
[9] | Edmonds, E., (2005). Does child labour decline with improving economic standards? Journal of human resources 40(1):77-99. |
[10] | Basu, K., and and Van, P. H., (1998). ‘’The economics of child labour’’. American Economic Review 88(3):412-427. |
[11] | Kambhampati, V., and Rajan, R., (2005). Economic growth, a panacea for child labour. World development 34(3):426-445. |
[12] | Dumas, C. (2007). Why do parents make their children work? A test of the poverty hypothesis in rural areas of Burkina Faso. Oxford Economic papers 59(4): 301-329. |
[13] | Bhalotra, S. and Heady C. (2003). Child labour, the wealth paradox’’. World Economic Review 17(2):197-227. |
[14] | Akarro R. R. J., and Mtweve N. A. (2011). poverty and associated with child labour in Njonbe District in Tanzania. The Case of Igima Ward. Current Journal of Social Scciences 3(3):199-206. |
[15] | Nkamleu, G. B. (2006). Poverty and child farm labour in Africa: wealth paradox or Bad orthodox. African journal of economic policy 13(1): 1-24. |
[16] | Ghana Statistical Service (2010). Report on 2010 national population and housing census in Ghana. |
[17] | Ray, R., (2000). Analysis of child labour in Peru and Pakistan: a comparative study. Journal of popualtaion Economic 13(1):3-19. |
[18] | Andvig, J. C. (2001). Family controlled child labour in sub Saharan Africa. A survey research. The world Bank Social Protection Discussion Paper No. 0122. |
[19] | Maddala, G. S. (2001). Introduction to Econometrics. John willey and sons, Ltd. Chichester, U.K. |
[20] | Pakes, A., and Berry S., (2007). “The pure characteristics of descrete choice model of diiferentiated products’’ International Economic Review. |
[21] | Green, W., H. (2008). Econometric Analysis (6th edition). New Jersy; Pearson Education Inc. Available athttp://mpra.ub.unimuenchen.de/66990 Accessed: 15/06/2014. |
[22] | McFadden, D., and K. Train, (2000). “Mixed MNL models for Discrete Respoonse’’. Journal of applied econometric, 35(1):319-333. |