Mohamed Tahir
Department of Mathematics, College of Sciences, University of Sharjah
Correspondence to: Mohamed Tahir, Department of Mathematics, College of Sciences, University of Sharjah.
Email: |  |
Copyright © 2012 Scientific & Academic Publishing. All Rights Reserved.
Abstract
The problem addressed is that of sequentially estimating θ, the shape parameter of the type I Pareto distribution, subject to the loss function
where c is a known cost per observation and
is the maximum likelihood estimator of θ. We propose a stopping time t and provide a second-order asymptotic expansion, as c → 0, for the regret incurred by the sequential procedure
under the loss Lc. We also show that the point estimator
is asymptotically unbiased for θ.
Keywords:
Anscombe’s Theorem, Doob’s Maximal Inequality, Excess over the Stopping Boundary, Hölder’s Inequality, Regret, Shape Parameter, Stopping Time, Uniform Integrability
Cite this paper: Mohamed Tahir, Sequential Estimation of the Shape Parameter of the Pareto Distribution, American Journal of Mathematics and Statistics, Vol. 3 No. 6, 2013, pp. 332-336. doi: 10.5923/j.ajms.20130306.05.
1. Introduction
The Type I Pareto distribution is a continuous distribution with probability density function (p.d.f.) 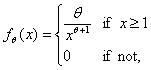 | (1) |
where θ is a positive number.The Pareto distribution was first formulated in the late 1800s by the Italian economist Vilfredo Pareto, who used this distribution to describe the allocation of wealth among individuals since it seemed to show rather well the way that a larger portion of the wealth of any society is owned by a smaller percentage of the people in that society. He also used it to describe the distribution of income. The Pareto distribution is not limited to describing wealth or income. It can be used as the distribution for insurance loss, oil reserve in a oil field, standardized price return on an individual stock, area burnt in a forest fire, etc…Let X1, , …, Xn denote independent observations to be taken sequentially up to a predetermined stage n from the Pareto distribution with p.d.f. (1). It is desired to estimate the parameter θ, subject to the loss function | (2) |
where c is a known cost per observation and
is the maximum likelihood estimator of θ. Since the log-likelihood function is
for observed values x1 > 1, …, xn > 1, of X1, , …, Xn, it follows that the maximum likelihood estimator of θ is
where
with Yi = lnXi, i = 1, …, n, and where the random variables Y1, …, Yn are independent with common distribution the Exponential distribution with mean
Expanding h(y) = 1/y about y = 1/θ and substituting
in the obtained expansion yields
for large n. It follows that the risk incurred by estimating θ by
under the loss (2) is
for large n. The approximate risk is minimized with respect to n by choosing n as the greatest integer less than or equal to  | (3) |
The minimum risk is
for sufficiently small c. Since n* depends on the unknown value of θ, there is no fixed-sample-size procedure that attains the minimum risk
Therefore, we propose to use the sequential procedure
which stops the sampling process after observing Y1, …, Yt and estimates θ by
, where | (4) |
with m being a positive integer. The performance of the procedure
is measured by its regret, which is defined as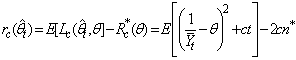 | (5) |
for c > 0.In this paper we propose a stopping time, t, based on (3), and provide a second-order asymptotic expansion, as c → 0, for the regret incurred by the sequential procedure
under the loss (2). We also show that the estimator
is asymptotically unbiased for the shape parameter θ.The problem of sequentially estimating the mean of a population was studied by many authors. Starr and Woodroofe[7] considered the case in which X1, X2, … are i.i.d. Normal random variables and showed that the regret of his procedure is O(1). Then, Woodroofe[10] showed that the regret is 0.5 + o(1) if m ≥ 4. Martinsek[8] extended Woodroofe’s[10] result to the nonparametric case. For the distribution-free case, Ghosh and Mukhopadhyay[3] and Chow and Yu[5] established asymptotic risk efficiency under certain conditions. Tahir[9] proposed a class of bias-reduction estimators of the mean of the one-parameter exponential family and provided an asymptotic second-order lower bound for the regret.
2. Asymptotic Expansion for the Regret of the Sequential Procedure 
Theorem 1: Let t be as in (4) with m ≥ 1, then
as c → 0.Proof: The stopping time t can be rewritten as | (6) |
Furthermore, let
for i = 1, 2, … and let
Then,
with g(u) = u + 1. Since g is a convex function and
where U += max {U, 0}, it follows from Proposition 5 and Theorem 1 of[2] that
as c → 0, where ρ denotes the asymptotic mean of the excess over the stopping boundary, Bc = tZt – n*. Moreover,
where
The theorem follows.Expanding h(y) = 1/y about
and substituting
in the obtained expansion yields | (7) |
where Wt is a random variable lying between
and
Lemma 1: Let t be as in (4) and let p > 1. Then, (i)
w.p.1 as c → 0.(ii)
is uniformly integrable;(iii)
is uniformly integrable if m > p.Proof. Assertion (i) holds by Proposition 2 of Aras and Woodroofe[2]. For the second assertion,
since
by the definition of t. Thus, Assertion (ii) holds since
By Doob’s maximal inequality (see[4]). To establish (iii), observe that
on {t > m}, by the definition of t; so that
where I{.} denotes the indicator function. This implies that
by the cr-inequality (see Loève[6]). Since
and
for some A >0, by Doob’s maximal inequality, Assertion (ii) follows.Lemma 2: Let p > 1. If m > p, then,(i)
is uniformly integrable and(ii)
is uniformly integrable.Proof. The first assertion follows by using Assertion (i) of Lemma 1 and Theorem 2 of[3]. The second assertion follows since
and
for some constant B > 0, by Doob’s maximal inequality.Theorem 2: Let
be as in (5). Then,
as c → 0.Proof. It follows from (5) that 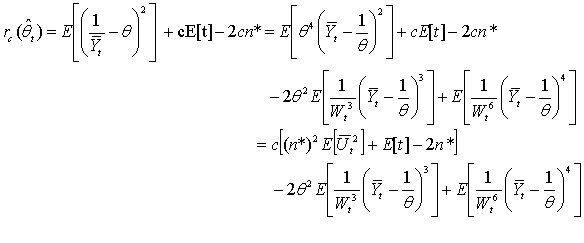 | (8) |
for c > 0. Next, by Corollary 1 of Aras and Woodroofe[2], | (9) |
as c → 0. Now, let 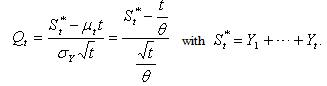 | (10) |
and let Z denote a random variable having the Standard Normal distribution. Then, 
 | (11) |
as c → 0, by Assertion (i) of Lemma 1, the fact that Qt → Z in distribution as c → 0 by Anscombe’s theorem (see[1]) and the fact that Wt → 1/θ w.p.1 as c → 0. The uniform integrability of
follows from Assertions (ii) of Lemmas 1 and 2 since
Now take the limit as c → 0 in (8) and use (9) and (11) to complete the proof of the theorem.Lemma 3: Let t be defined by (4). If m > 4, then
as c → 0.Proof: It follows from (7) that | (12) |
where Wt is a random variable lying between
and µY =
Next,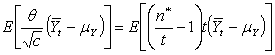 | (13) |
by (3) and Wald’s lemma. Moreover, it follows from (6) that
say, where
denotes the excess over the stopping boundary and Qt is as in (10). Now,
as c → 0, by Anscombe’s theorem and the fact that
is uniformly integrable. Also, by Hölder’s inequality,
as c → 0, by Proposition 7 of[2] and Lemmas 1 and 2.Using these results in (13) yields | (14) |
For the second term in (12), observe that | (15) |
by the fact that Wt → 1/θ w.p.1 as c → 0, Assertions (i) and (ii) of Lemma 1, Anscombe’s theorem and the uniform integrability of
. The lemma follows by using (14) and (15) in (12).
3. Conclusions
1- We have proposed a sequential procedure for estimating the shape parameter of the type I Pareto distribution and provided a second-order asymptotic expansion for the regret. The procedure can be used for the data representing the losses for insurance policyholders or the insured values of homes. It specifies how many policyholders or homes should be selected and provides an estimate of θ based on this number.2- Since the asymptotic regret is positive for small values of the cost of sampling, it would be interesting to find a sequential procedure whose asymptotic regret is negative.
References
[1] | Anscombe, F., 1952, Large sample theory of sequential estimation, Proeedings Cambridge Philos. Soc., 48, 600-607. |
[2] | Aras, G. and Woodroofe, M., 1993, Asymptotic expansions for the moments of a randomly stopped average, Annals of Statistics, 21, 503-519. |
[3] | Chow, Y. S., Hsiung, C. A., and Lai, T. L., 1979, Extended renewal theory and Moment convergence in Anscombe’s theorem, the Annals of Probability, 7, 304-318. |
[4] | Doob, J.L., 1953, Stochastic Processes, Wiley, New York |
[5] | Ghosh, M. and Mukhopadhyay, N.,1979, Sequential point estimation of the mean when the distribution is unspecified, Commun. Statist.-Theory & Methods, A8, 637-652. |
[6] | Loève, M., 1977, Probability Theory, 4th Ed., Springer-Verlag, New York. |
[7] | Starr, N. and Woodroofe, M., 1969, Remarks on sequential point estimation, Proc. Nat. Acad. Sci., U.S.A., 63, 285-288. |
[8] | Martinsek, A.T., 1983, Second order approximation to the risk of a sequential Procedure, Annals of Statistics, 11, 827-836. |
[9] | Tahir, M., 1989, An asymptotic lower bound for the local minimax regret in sequential point estimation, Annals of Statistics, 17, 1335-1346 |
[10] | Woodroofe, M., 1977, Second order approximations for sequential point and interval estimation, Annals of Statistics, 5, 984-995. |