Kouji Tahata, Takuya Yoshimoto, Sadao Tomizawa
Department of Information Sciences, Tokyo University of Science, Noda City, Chiba, 278-8510, Japan
Correspondence to: Kouji Tahata, Department of Information Sciences, Tokyo University of Science, Noda City, Chiba, 278-8510, Japan.
Email: |  |
Copyright © 2012 Scientific & Academic Publishing. All Rights Reserved.
Abstract
For the analysis of square contingency tables, Tomizawa, Miyamoto and Ashihara (2003) considered a measure to represent the degree of departure from marginal homogeneity. The measure lies between 0 and 1, and it takes the minimum value when the marginal homogeneity holds and the maximum value when one of two symmetric cumulative probabilities for any category is zero. This paper proposes improvement of the measure so that the degree of departure from marginal homogeneity can attain the maximum value even when the cumulative probabilities are not zero. The proposed measure would be useful for representing the degree of departure from marginal homogeneity, especially when some asymmetry models hold as the extended marginal homogeneity model or the conditional symmetry model. Examples are given.
Keywords:
Kullback-Leibler information, Measure, Power-divergence, Shannon entropy
Cite this paper: Kouji Tahata, Takuya Yoshimoto, Sadao Tomizawa, Marginal Asymmetry Measure Based on Entropy for Square Contingency Tables with Ordered Categories, American Journal of Mathematics and Statistics, Vol. 3 No. 3, 2013, pp. 95-98. doi: 10.5923/j.ajms.20130303.01.
1. Introduction
Consider an
square contingency table with the same row and column classifications. Let
denote the probability that an observation will fall in the
th row and
th column of the table (
), and let
and
denote the row and column variables, respectively. The marginal homogeneity (MH) model is defined by
namely
where
and
(see, for example, Stuart, 1955; Bishop, Fienberg and Holland, 1975, p.293). Let
and
for
. By considering the difference between the
and
, the MH model also be expressed as
Namely, this states that the cumulative probability that an observation will fall in row category
or below and column category
or above is equal to the cumulative probability that the observation falls in column category
or below and row category
or above for
. When the MH model does not hold, we are interested in measuring the degree of departure from MH. For square contingency tables with ordered categories, Tomizawa, Miyamoto and Ashihara (2003) proposed the measure (denoted by
in Section 2) to represent the degree of departure from MH. The measure
ranges between
and
Also, (i)
if and only if the MH model holds, and (ii)
if and only if the degree of departure from MH is a maximum; that is,
(then
) or
(then
) for all
. However, for the analysis of square contingency tables, all cell probabilities
are positive in many cases. Thus, the measure
may be unsuitable for such data, because the measure
cannot attain the maximum value. So, we are now interested in the measure to represent the degree of departure from MH such that it can attain the maximum value even when each of cell probabilities
is not zero. Yamamoto, Masumura and Tomizawa (2011) considered such a measure for nominal square table. We are now interested in proposing such a measure for ordinal square table. The purpose of this paper is to consider an improvement of measure for square contingency tables with ordered categories when all cell probabilities
are positive.
2. Improved Measure for Marginal Homogeneity
Consider an
table with ordered categories. Assume that
are positive. Let
for
; and let
For a specified
which satisfies
and
for all
, consider a measure defined by
where
with
and the value at
is taken to be the limit as
. Thus,
where
with
Note that
is the diversity index proposed by Patil and Taillie (1982), which includes the Shannon entropy when
. When
, then
is identical to the measure
given by Tomizawa et al. (2003). Since
, the minimum value of
is 
and the maximum value of it is
or
(if
) when
for all
. So, when
cannot attain the value 1. The proposed measure
with
is modified by using modification coefficient
such that the measure
can attain the value
. If all
are positive, then
must be taken as
. Moreover, for each
and a fixed
, the measure
has characteristics that (i)
must lie between
and
, (ii)
if and only if the MH model holds, i.e.,
for all
and (iii)
if and only if the degree of departure from MH is the largest in the sense that
for all
. The measure also may be expressed as, for 
where
especially
Note that
is the power-divergence between
and
(Cressie and Read, 1984) which includes the Kullback-Leibler information when
.
3. Approximate Confidence Interval for Measure
Let
denote the observed frequency in the
th row and
th column of the table (
). Assume that a multinomial distribution applies to the
table. The sample version of
, is given by
with
replaced by
, where
and
. Using the delta method (Bishop et al., 1975, Sec. 14.6),
has asymptotically (as
) a normal distribution with mean zero and variance
where for
with
and the value of variance at
is taken to be the limit as
. Let
denote
with
replaced by
. Using this result, the estimated approximate confidence interval for the measure
is obtained.
4. Examples
Consider the data in Table 1, taken from Andersen (1997, p.226). These data show the forecasts for production and prices for the coming three year periods given by experts in July 1956 and the actual production figures for production and prices in May 1959 given from Danish factories. For these data, the cell probabilities
are theoretically positive (not zero). Thus, it may be irrelevance to use the measure
with
. So we should use the measure
with
(for example,
) so that the measure can attain the maximum value 1. Table 1. Results from the forecasts for production and prices and the actual production figures for production and prices (Andersen, 1997, p.226) 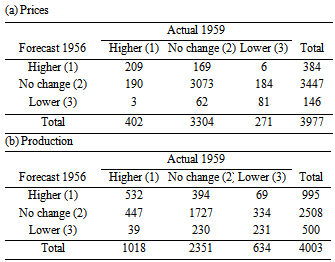 |
| |
|
Table 2. When , the estimates of , estimated approximate standard error for , and approximate 95% confidence interval for , applied to Tables 1a and 1b. 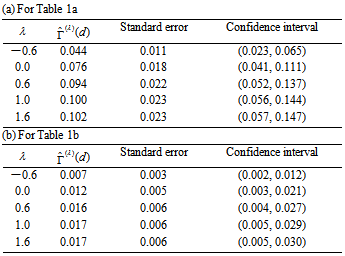 |
| |
|
If we set
and
, the estimated measure
is
for Table 1a and
for Table 1b from Tables 2a and 2b. Thus, (i) for Table 1a, the degree of departure from MH is estimated to be
percent of the maximum degree of departure from MH and (ii) for Table 1b, it is estimated to be
percent of the maximum. Furthermore, we see from Tables 2a and 2b that the degree of departure from MH is greater for Table 1a than for Table 1b because the values in the confidence intervals for
are greater for Table 1a than for Table 1b.
5. Discussion
Consider the extended MH (EMH) model defined by
also see Tahata and Tomizawa (2008). A special case of EMH model obtained by putting
is the MH model. When the EMH model holds, the proposed measure
is expressed as 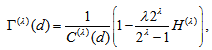 | (1) |
where
For
fixed and
fixed,
increases as
increases (or as
decreases). Especially, when
,
is identical to
proposed by Tomizawa et al. (2003). When the EMH model holds,
approaches 1 as
approaches infinity or zero. However, when the EMH model holds,
cannot attain 1 because then
and
, namely there is not the structure of
being the condition of
. The measure
with
can attain the maximum value 1 even if
and
for all
. Therefore, the measure
with
rather than
may be appropriate when the EMH model holds. Also since the probabilities
are positive (not zero), the measure
with
rather than
would be appropriate to represent the degree of departure from the MH toward the structure of maximum departure from MH which can be defined actually.The conditional symmetry (CS) model (McCullagh, 1978) is defined by
A special case of this model obtained by putting
is the symmetry model (Bowker, 1948). If the symmetry model holds, then the MH model holds. Also if the CS model holds, then the EMH model holds. Therefore when the CS model holds, the measure
is expressed by with
replaced by
. Thus by the similar reason, when the CS model holds, the measure
with
rather than
would be appropriate.
References
[1] | E. B. Andersen, Introduction to the Statistical Analysis of Categorical Data. Berlin, Germany: Springer, 1997. |
[2] | Y. M. M. Bishop, S. E. Fienberg and P. W. Holland, Discrete Multivariate Analysis: Theory and Practice. Cambridge, Massachusetts, U.S.: The MIT Press, 1975. |
[3] | Bowker, A. H., 1948, A test for symmetry in contingency tables. Journal of the American Statistical Association, 43, 572-574. |
[4] | Cressie, N. and Read, T. R. C., 1984, Multinomial goodness-of-fit tests. Journal of the Royal Statistical Society, Series B, 46, 440-464. |
[5] | McCullagh, P., 1978, A class of parametric models for the analysis of square contingency tables with ordered categories. Biometrika, 65, 413-418. |
[6] | Patil, G. P. and Taillie, C., 1982, Diversity as a concept and its measurement. Journal of the American Statistical Association, 77, 548-561. |
[7] | Stuart, A., 1955, A test for homogeneity of the marginal distributions in a two-way classification. Biometrika, 42, 412-416. |
[8] | Tahata, K. and Tomizawa, S., 2008, Generalized marginal homogeneity model and its relation to marginal equimoments for square contingency tables with ordered categories. Advances in Data Analysis and Classification, 2, 295-311. |
[9] | Tomizawa, S., Miyamoto, N. and Ashihara, N., 2003, Measure of departure from marginal homogeneity for square contingency tables having ordered categories. Behaviormetrika, 30, 173-193. |
[10] | Yamamoto, K., Masumura, K. and Tomizawa, S., 2011, Improved measures of departure from marginal homogeneity for square contingency tables with nominal categories. Oriental Journal of Statistical Methods, Theory and Applications, 1, 29-40. |