Chinedu Pascal Ezenkwu, Ogungbemi Emmanuel Oluropo, Bliss Stephen
Department of Electrical/Electronic and Computer Engineering, University of Uyo, Uyo, Nigeria
Correspondence to: Chinedu Pascal Ezenkwu, Department of Electrical/Electronic and Computer Engineering, University of Uyo, Uyo, Nigeria.
Email: |  |
Copyright © 2016 Scientific & Academic Publishing. All Rights Reserved.
This work is licensed under the Creative Commons Attribution International License (CC BY).
http://creativecommons.org/licenses/by/4.0/

Abstract
Improvements in web technologies have motivated a rapidly increasing research interests in online collaborative research frameworks. Such frameworks provide online networks of individuals from the academia, the industry, and the public sector for the purpose of research collaborations; and the use of collective intelligence in tackling societal problems. This paper aims at modelling a collaborative digital research ecosystem which leverages on a novel time-dependent matchmaker model in identifying and linking collaborators with similar research behaviours and interests. In the development of this model, it was considered that a researcher’s interest in a particular topic may change with time. Notably, this system will encourage formation of online research teams; enhance multidisciplinary researches; facilitate co-authoring among researchers regardless of geographical locations; easy identification of domain experts; ideation and so on.
Keywords:
Research, Collaboration, Co-authoring, Multidisciplinary research, Matchmaking, Web analytics, Digital Ecosystem
Cite this paper: Chinedu Pascal Ezenkwu, Ogungbemi Emmanuel Oluropo, Bliss Stephen, Modelling a Collaborative Digital Research Ecosystem Based on a Novel Time-Dependent Matchmaker Model, American Journal of Intelligent Systems, Vol. 6 No. 1, 2016, pp. 14-21. doi: 10.5923/j.ajis.20160601.02.
1. Introduction
Collaborative research involves two or more individuals coming together for the purpose of investigating some existing situations or problems; providing solutions to problems; exploring and analysing issues; constructing or creating procedures or systems; explaining new phenomena; generating new ideas or achieving a combination of the mentioned objectives. The advantages of collaborative research amongst others include the ability to solve problems cutting across disciplines; encouragement of interdisciplinary knowledge sharing; and optimal approach to problem solving. Communications among collaborators can occur via myriads of media such as telephone, face-to-face meetings, email, letter or website. Unlike other IT-based communication media; websites can as well serve as a melting pot where potential collaborators meet for research purpose. The advent of web 2.0 had encouraged User Generated Content (UGC) on the web. Consequently, this has encouraged smarter online informatics and applications such as social networks, ecommerce, eTourism, eLearning, virtual community, blog and blogs aggregator and so on. Digital Ecosystems can be viewed as digital counterparts of biological ecosystems, which are considered to be robust, self-organising and scalable architectures that can automatically solve complex, dynamic problems [1]. According to [2], “the Digital Ecosystem is the space formed by the convergence of the media, telecoms and IT industries. It consists of users, companies, governments and civil society, as well as the infrastructure that enables digital interactions.” In a Digital Ecosystem, the digital users are not just consumers of information and services but they equally have their own niche in the community. They can be contributors to online communities, creators and distributors of digital contents and services. Digital Ecosystems enable users to identify like-minded individuals for interaction and sharing of digital contents as well as gaining access to experts’ knowledge and advice [2]. In natural environment, opinions or words-of-mouth from experienced people have been the most influential source of information for decision making about goods and services. In the same vein, Digital Ecosystems have supported electronic words-of-mouth as a means of allowing opinions to crystallise. This has been achieved in different systems, especially in digital business ecosystems, through users’ reviews, ratings and so on. Apart from explicit reviews or ratings of services on these systems by the digital users, users’ interests or other relevant information can as well be obtained implicitly using web analytic tools. However, more robust users’ profiles can be realised by combining both the explicit and implicit strategies. Web analytic tools help in monitoring a user’s interests or preferences by keeping track of his/her click-throughs, page views and so on. However, this information is used in updating the user’s profile on the fly. A user’s experience on an online platform can be improved upon through correct recommendations of products or matchmaking of the user with other users of like-minds or related interests depending on the context. The thrust of this paper is to model a collaborative digital research ecosystem based on a novel time-dependent matchmaker model developed to link collaborators who have similarities in their research behaviours. This model is based on the fact that each collaborator’s research profile changes with respect to time. The remainder of this paper is organised as follows: The objectives of the research are highlighted in section 2. In section 3, reviews on related literatures are presented. In section 4, the system architecture design and modelling is elaborated. Section 5 details the development of the time-dependent matchmaker model while Section 6 presents the results and discussions. Finally, the Conclusion and areas of Future research are presented in section 7.
2. Objectives of Study
The work entailed in this paper is to achieve the under-listed objectives:● To model a collaborative digital research ecosystem that will serve as a melting pot for individuals from the academia, the industry, and the public sector;● To develop a time-dependent matchmaker model that will link like-minded collaborators based on their research behaviours.
3. Review of Related Literature
3.1. Collaborative Research
In [3], the authors examined the extent of research collaborations amongst agricultural engineers in Nigeria, using the Nigerian Institution of Agricultural Engineers (NIAE) proceedings between 2000 and 2010 with the exclusion of 2008 data, covering a period of 10 years. From the ten years data gathered, co-authored papers surpassed single author papers for each year which shows a sign of collaboration amongst researchers in that field. Reference [4] highlighted some challenges of collaborative research. The paper outlined factors contributing to these challenges to include the inability of the team members to work efficiently towards realising the goals within the stipulated time due to unequal drives to research amongst the team members. Another challenge mentioned by the authors is the inability to select a reliable technology or tool for collaboration. However, the authors reviewed some collaborative technologies that are motivated by the advent of web 2.0. The most common examples of such technologies as mentioned in the paper include Google Docs and Wikis.The authors of [5] presented a definition of Social Research Network sites (SRNs) along with four basic functionalities; these are identity and network management, communication, information management, and collaboration. The authors had in-depth interviews with the founders of ten SRNs. The paper suggested the first tentative taxonomy according to the purpose the founders of these SRNs had in mind and the basic functionalities they provide. The paper identified three types of SRNs providing one or a combination of the above-mentioned functionalities. They include research directory sites, research awareness sites and research collaboration sites. The research directory sites focus on the identification of researchers according to certain criteria, e.g. his research agenda or special competencies in a field, theory or method. In this type of SRN, the researchers mak;e their comprehensive profile visible and accessible to other researchers on the network, hence, supporting the functionalities of identity management and Communication. Example of such SRN is academia.edu. Another type of SRN presented by the authors is a research awareness sites, which allow researchers to maintain their profile, supply detailed information on their current works and interests, as well as following other users they are interested in to keep track of their activities. As such these sites offer the functionalities of identity and network management as well as information management. Example of such site is Research Gate. Finally, the authors presented the third SRN as research collaboration sites. This supports virtual research collaboration by facilitating joint research process. Example of such SRN is collabrx, which provides researchers with the opportunity to work synchronously on a shared scientific dataset and to develop data analysis scripts collaboratively.
3.2. Matchmaking in an Online Community
According to [6] “Matchmaking is an umbrella which covers the actual match, whereby two or more parties who are in need of each other’s expertise are brought together to build up relationships. The term also covers ideation, which is considered part of, or the result of, matchmaking. Knowledge sharing is also to be found under this umbrella.” Reference [7] posits that” Matchmaking is the process of searching the space of possible matches between demands and supplies.” Matchmaking is facilitated by a matchmaker who understands the parties involved and provides them with the right framework to meet and collaborate. Over the years, several researches have been carried out on how to automate matchmaking in Digital Ecosystems. Reference [7] presents a conceptual framework of matchmaking in B2B e-marketplaces environment. The author proposed a four step process together with the proposed methods for every step for solving the matching problem: (i.) analyse the requirements and proposals and create matches using the bipartite graph matching method; (ii.) modelling the matches (constructing buyer and seller satisfaction function and then the mathematical model), (iii.) implementation and (iv.) optimization of the matches using multi-objective genetic algorithm and finding the Pareto optimal solution set from feasible solutions.In [8] the authors presented a matchmaking strategy developed for Ghost Recon Online, a team-focused First Person Shooter from Ubisoft using a Neural network model. The paper provided solutions to the problems of (i) finding the best team combinations from a pool of players waiting for a match, and (ii) continuously updating the model in real time as new data is being collected.Reference [9] proposed a friend recommendation algorithm for a Social Bookmarking System, based on low computational effort heuristics that allow real time applications. Experimental results showed that, when users tag in the same way and are also interested in the same content, they can be recommended as friends. The proposed algorithm produces better results, in contrast to policies that use only tags and do not consider content.In Reference [10] an Adaptive Match-Making System (AMMS) that reflects the users’ explicit and implicit preferences was presented. Initially users assign weights to their attributes of interests based on their level of importance; the system automatically adjusts the weight of each attribute by analyzing the users’ previous behaviors and as such updating the users record on the system. A logistic regression model was used to find out the user’s implicit weights and reflects them in proportion to the accuracy of the resulting model.
4. System Architecture Design and Modelling
The system architecture is presented in Figure 1. The proposed model assumes the three tier web architecture. The System intelligence is controlled by the web analytics script, matchmaker script, keyword mining script and the communication script that controls the transportation of data between the database and the web interface. The web analytic script monitors each user’s click-throughs, page navigations and page views. Based on the keywords or tags associated with the pages or papers a user clicks, downloads or reads, the user’s interest profile is updated. The matchmaker algorithm computes the similarities among users based on their domains of interests. The to and fro of data between the database and user interface or other scripts is controlled by the application control script via the web server. The activities that the users of the system can perform are equally presented in the user interface part of the architecture.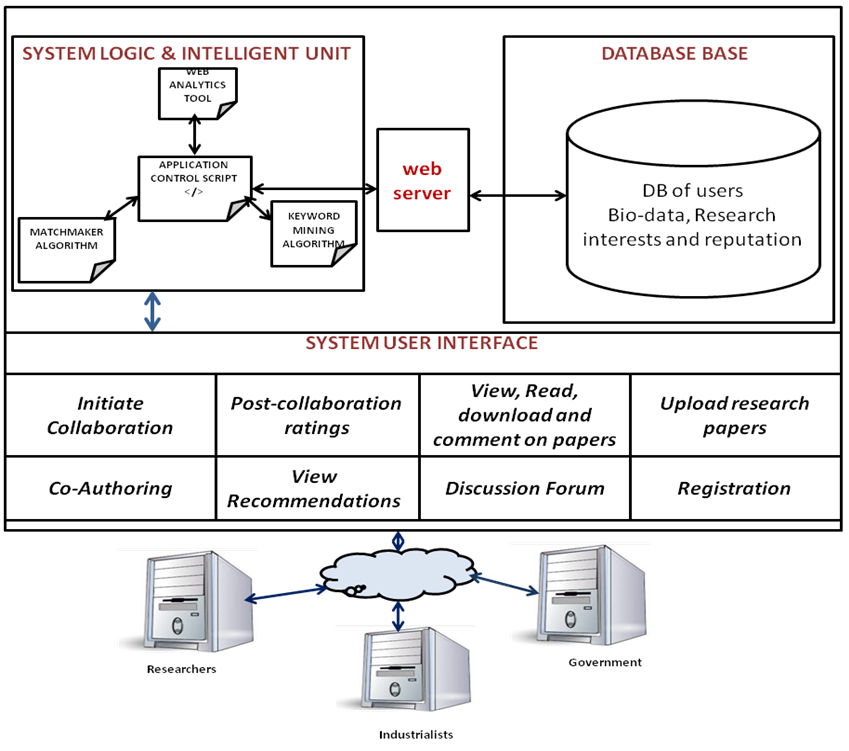 | Figure 1. System Architecture |
The use case description of the proposed system is presented in figure 2. Every user of the system must be registered in order to use the system. The registration process must involve the provision of the user’s bio-data and research profile information consisting of areas of research interest. The user may as well upload his/her research papers. A discussion forum is provided for synchronous communication amongst the users. Users post questions which are visible to other users. Moreover, to help users in identifying potential collaborators, the system uses the matchmaker algorithm developed in this paper to recommend potential collaborators to each user. The users can view the recommended collaborators; check their reputations, access their research profiles and publications so as to decide whether to initiate collaboration or not. Each user updates his/her engagement status to enable other users know if s/he is free for collaboration or not. Users, especially industrialists or civil servants, may present some incentives to motivate researchers in solving their problems. Potential collaborators who had agreed to co-author a paper or book may use a built-in wiki in the ecosystem for synchronous and asynchronous co-authoring or they may decide to use any of the available free co-authoring tools such as Google docs, SharePoint, etc. At the end of collaborations, the collaborators are prompted to rate one another and to also update their engagement statuses. 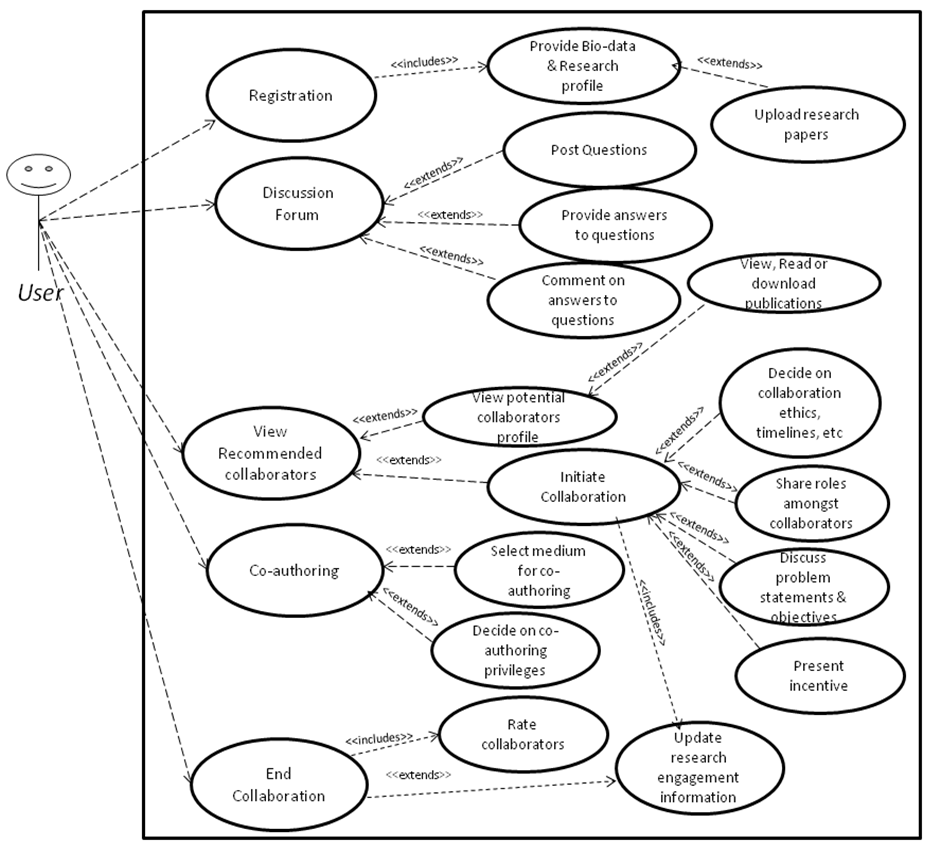 | Figure 2. Use case diagram describing system functionalities |
5. Development of the Time-dependent Matchmaker Model
5.1. Conceptualisation and Modelling of the Time-dependent Matchmaker model
Definition: A matchmaker model is a mathematical function that takes a set of system users U and a target user ut as inputs and returns a set of users Um, such that
and there is at least a common interest between ut and each user belonging to Um . Figure 3 shows a conceptual graph for developing the proposed matchmaker model.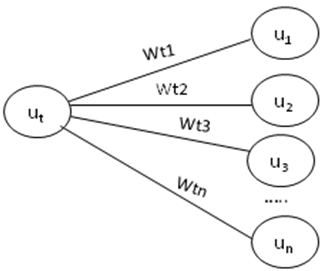 | Figure 3. Conceptual graph for developing the matchmaking model |
uj is the jth member of U = {u1,u2,u3,u4,…,un}, where, |U| = n. wtj is the similarity score between the target user ut and user uj , 
 | (1) |
IFi is a vector of interest factors of all topics T that user i has shown interest in.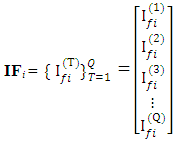 | (2) |
Ifi(T) is the Interest factor of user i in topic T and Q is the total number of topics that user i has shown interest in. Ifi(T) is calculated using equation (3)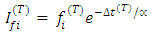 | (3) |
is the interest rating of user i in topic T or the number of times user i showed interest in topic T and
is the time difference between the time of computing
the current time
and the last time
when user i showed interest in topic T.  | (4) |
is a positive number that controls how fast
decreases as
increases. Figure 4 shows how
varies with
at different values of
. From figure (4), the larger the value of
, the more gradual the Interest factor
falls as
increases. 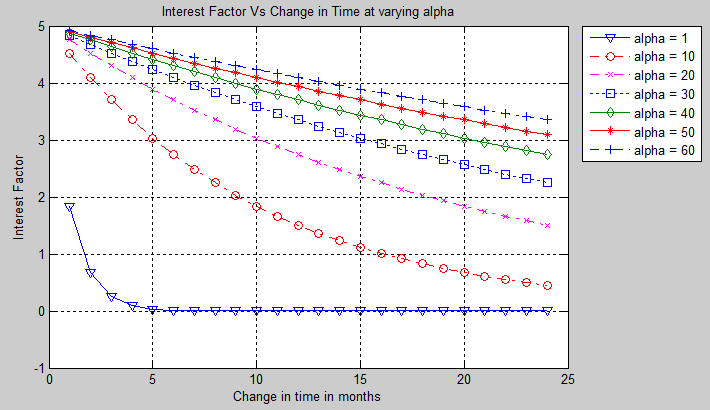 | Figure 4. Effect of on how of varies with |
5.2. Step by Step Procedure for Implementing the Time-dependent Matchmaker Model
For the sake of simplicity, the application of the proposed model in matchmaking a target user ut and any user uj is considered. Figure 5 presents a simplified conceptual graph for ut and uj .  | Figure 5. Simplified Conceptual graph for Matchmaking Two Users |
The steps for matchmaking ut and uj are as follows:Step 1: Compute each user’s interest factor in each topic. For example, Table 1 shows how to compute the interest factor in each topic for user ut.Table 1. A table of user t Interest factors 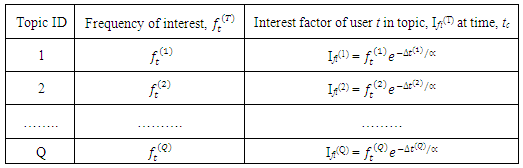 |
| |
|
Step 2: Build the Vectors of Interest Factors IF for both ut and uj.The vectors of Interest Factors for ut and uj,
and
respectively.Step 3: Calculate the similarity wtj between ut and uj using IFt and IFj from step 2 above. wtj = sim (IFt,IFj)The analysis above over-simplifies the matchmaking process. In an online community, the model is to identify a set of users in the ecosystem that have the best similarities with the target user. To achieve this, the users are sorted with respect to their wtj. The users with the smallest values of wtj are recommended to the target user.
6. Results and Discussions
Data for the evaluation of the model were collected using questionnaire issued to twenty (20) Computer Engineering and Computer science researchers. A sample of the questionnaire is shown in appendix I. With the questionnaire, each researcher rated his/her research interest in each area on a 0-6 point scale. 0 means no research interest while 6 means extreme research interest. 3 is the midpoint. Furthermore, the researchers provided the last time in months when they researched in each of the topics. If a researcher has never researched in a topic at all, such researcher is to choose 1000 months ago for that topic. The large time interval will reduce his/her interest factor in that topic. The responses from the researchers are provided in the dataset of appendix II. For purpose of analysis, researcher with ID #4 was chosen as the target user and using the model ID #4 was linked to other researchers from the dataset who have similar research behaviour.As shown in section 5, the table of users’ interest factors for each topic was computed (as shown in appendix III). In the experiment,
was set to 10. Using the interest factors for each user in the topics, the similarities between the target user and other users were computed. Different similarity measures such as Manhattan distance, Euclidean distance, hamming distance, cosine similarity, etc can be used. In this experiment, the Euclidean distance was adopted as the similarity measure. Given two vectors X = {x1, x2, ……., xn} and Y = {y1,y2,……..,yn}, the Euclidean distance between X and Y is given by  | (6) |
 | (7) |
Thus, for all j belonging to the list of researchers, wtj is computed using Euclidean distance as the similarity measure. The smaller the Euclidean distance of the target user from any user, the higher the similarity between them and the higher the possibility of matchmaking them.The first five users that are recommended to the target user in the order of their increasing Euclidean distances are: #11 with wtj of 0; #16 with wtj of 1.1351; #10 with wtj of 1.5304; #18 with wtj of 1.8819 and #2 with wtj of 2.3039. Comparing the above recommendations with the information in the dataset in appendix I, it can be proven that researchers #11, #16,#10,#18 and #2 have similar research behaviours as researcher #4(the target user). Researcher #4 has no interest in AI and has never researched in it before; he is extremely interested in CN and has researched in it one month ago; his interest in SE is average and he had researched in it two years ago; finally, he has no interest in DSA and has never researched in it. The recommended researchers have considerable research interest in CN and have researched in it at most four months ago. Interestingly, none of them had shown interest in any other research topic recently just like the target researcher. Looking at the dataset, one may doubt the performance of the model for not considering #6, #7, #3, #14, #15, #17, and #19, which are very interested in CN and have equally done a research in it recently. But checking their records thoroughly will reveal that these researchers have equally shown high interests in some topics that contradict #4’s interest and they have also worked on those topics in recent times. Thus, the model considered only those researchers who had shown similar research patterns as the target researcher for matchmaking in terms of time and interests in research topics. Moreover, this point could be more lucid from appendix IV in which the interest factors are expressed to a scale of 0 to 100 to reflect the strength of the model in choosing the right researchers for matchmaking. This is achieved by performing min-max normalization on the elements in each column of the table in appendix III and multiplying the result by 100.Each
belonging to a column vector X is converted to a scale of 0 to 100 by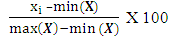 | (8) |
7. Conclusions and Future Research
In this paper, a novel time-dependent matchmaker model has been developed to link collaborators with similar research behaviours in a digital collaborative research ecosystem. In the development of the model, it was considered that a researcher’s interest in a particular topic may change with time. Through this model, researchers who have similar research behaviours based on their current research interests can be easily linked on the system for research purpose. Moreover, the system architecture showing different activities a user can perform on the system was presented. The aims of this paper are to enhance collaborative researches; facilitate co-authoring among researchers; ease the process of identifying experts; encourage multidisciplinary researches and so on through the use of a collaborative digital research ecosystem. However, future research should study the dependences of the model performance on - different values of
; the choice of similarity measures and the number of research topics considered for matchmaking.
Appendix
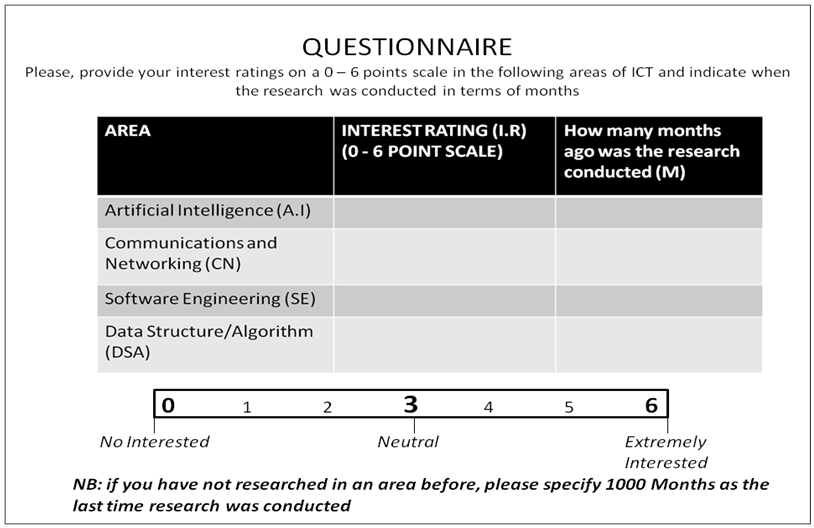 | Appendix I: Sample of the Questionnaire |
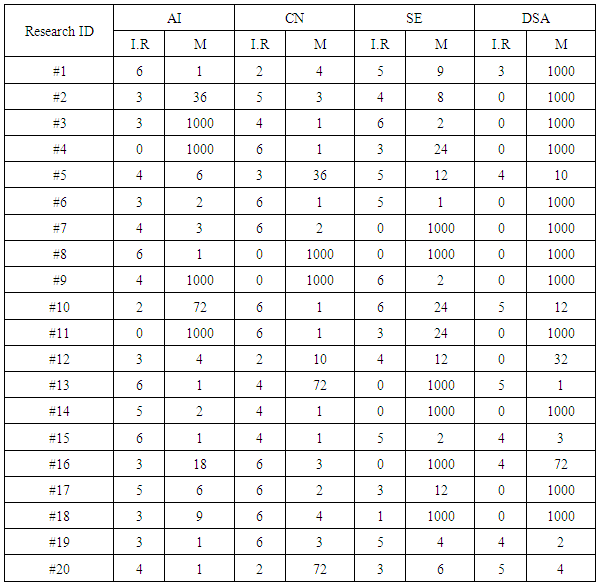 | Appendix II: Table Showing the Responses of the Selected Researchers |
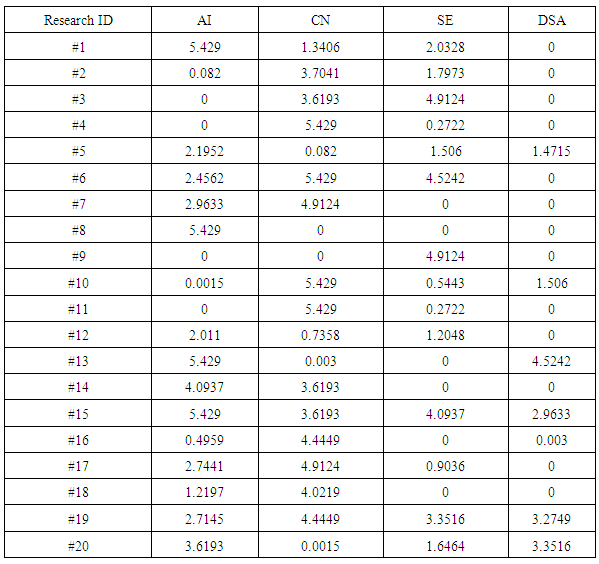 | Appendix III: The Table of Users’ Interest Factors in Each Topic |
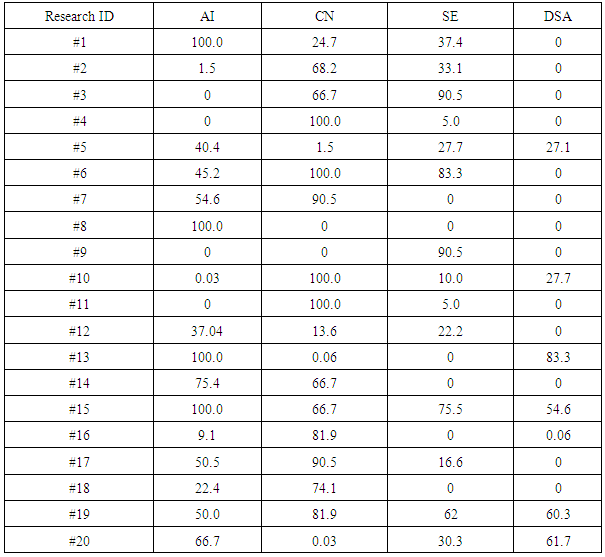 | Appendix IV: The Table of Users’ Interest Factors in Each Topic Expressed on a Scale of 0 to 100 |
References
[1] | Gerard, B, (2009), Digital Ecosystems (Doctoral Thesis, Department of Electrical and Electronic Engineering, Imperial College London). Retrieved from http://arxiv.org/pdf/0909.3423.pdf on December, 2015. |
[2] | World Economic Forum (2007). Digital Ecosystem Convergence between IT, Telecoms, Media and Entertainment: Scenarios to 2015. Retrieved from http://www3.weforum.org/docs/WEF_DigitalEcosystem_Scenario2015_ExecutiveSummary_2010.pdf on December, 2015. |
[3] | Oyeniyi, J. O. and Olaifa, T. P, (2012), Collaborative strength and pattern of authorship among agricultural engineers in Nigeria: A case study of the 2000 – 2010 NIAE proceedings. International Journal of Library and Information Science Vol. 4(6), pp. 115-120. Retrieved from http://www.academicjournals.org/Ijlis on December, 2015. |
[4] | Atkins, A.T., (2012), Collaborating Online: Digital Strategies for Group Work. Retrieved from http://www.parlorpress.com/pdf/atkins--collaborating-online.pdf on December, 2015. |
[5] | Bullinger, A.C., Hallerstede, S.H., Renken, U., Soeldner, J. & Moeslein, K.M., (2010). Towards Research Collaboration – a Taxonomy of Social Research Network Sites. Proceedings of the Sixteenth Americas Conference on Information Systems, Lima, Peru. |
[6] | Danish Agency for Science, Technology and Innovation, (2012), Inspirational Catalogue: Matchmaking, knowledge sharing and Idea Creation. Retrieved from http://www.clusterexcellencedenmark.dk/Admin/Public/Download.aspx?file=Files%2FFiler%2Fpublikationer%2FInspirational_catalogue.pdf on December, 2015. |
[7] | Fekete, Z. A., (2010). Matchmaking Framework for B2B E-Marketplaces. Informatica Economică vol. 14, no. 4. Retrieved from http://revistaie.ase.ro/content/56/16%20-%20Zoltan%20Alpar.pdf on December, 2015. |
[8] | Delalleau, O., Contal, E., Thibodeau-Laufer, E., Ferrari, R. C., Bengio, Y., and Zhang, F., (2012). Beyond skill rating: Advanced matchmaking in Ghost Recon Online. IEEE Trans. Comput. Intell. AI Games, vol. 4, no. 3, pp. 167–177. |
[9] | Matteo, M., Ludovico, B., and Salvatore, C., (2013). Producing friend recommendations in a social bookmarking system by mining users content. The Third International Conference on Advances in Information Mining and Management, pages 59–64. |
[10] | Park, Y.J. (2013). An Adaptive Match-Making System reflecting the explicit and implicit preferences of users. Expert Systems with Applications, 40(4), 1196–1204. |
[11] | Overbeek, S. J., van Bommel, P., & (Erik) Proper, H. A. (2011). Statics and dynamics of cognitive and qualitative matchmaking in task fulfillment. Information Sciences, 181(1), 129–149. |