Franco Caldas Degrazia, Bardo Bodmann
Mechanical Engineering Graduate Program (PROMEC), Federal University of Rio Grande do Sul – UFRGS, Porto Alegre/RS, Brazil
Correspondence to: Franco Caldas Degrazia, Mechanical Engineering Graduate Program (PROMEC), Federal University of Rio Grande do Sul – UFRGS, Porto Alegre/RS, Brazil.
Email: |  |
Copyright © 2018 The Author(s). Published by Scientific & Academic Publishing.
This work is licensed under the Creative Commons Attribution International License (CC BY).
http://creativecommons.org/licenses/by/4.0/

Abstract
The present work is part of the research project that aims to create an automated system for the evaluation of air pollution and odour for CMPC CELULOSE RIOGRANDENSE. Initially, three different ways of initializing the CALPUFF model (one of the models under evaluation to compose the system) were evaluated. The results when compared with observational data on air quality suggest the need for a validation of the boundary conditions and turbulent scale parameters in the hourly time representation when CALPUFF is the model being used. The choice was not random, but based on three quantities that impose physical affinity to micrometeorological data. The surface roughness, the albedo and the Bowen ratio. Changes in these quantities were imposed during pre-processing of AERMET, which established the quantities of interest in a simulation of dispersion of pollutants. After this alternative the results become more representative for the atmospheric dispersion process. The monthly and annual representation of the CALPUFF model is satisfactory without validation procedure.
Keywords:
Planetary boundary layer, Air quality system, AERMET, CALPUFF, Surface roughness, Albedo, Bowen ratio, Micrometeorological parameters
Cite this paper: Franco Caldas Degrazia, Bardo Bodmann, On an Air Quality and Odor Modeling System for CMPC CELULOSE RIOGRANDENSE, American Journal of Environmental Engineering, Vol. 8 No. 4, 2018, pp. 159-165. doi: 10.5923/j.ajee.20180804.13.
1. Introduction
Models of transport and dispersion of contaminants in the atmosphere are important tools for assessing anthropogenic influences on the environment. In most cases, atmospheric emissions can contribute to changes in air quality in the industrial, urban and rural environment [1]. In addition, some types of pollutants can also cause unpleasant odors when the atmospheric concentration exceeds the threshold of human perception. The present study is part of a research project of the atmospheric dispersion modeling group from the Federal University of Rio Grande do Sul (ADMG-UFRGS), in partnership with the Luiz Englert Foundation and financed by CMPC CELULOSE RIOGRANDENSE. The activities of the group consists in developing mathematical models, testing such models, and subsequently certifying them for widespread use by the scientific community as well as by society, such as environmental consulting firms and industries. The models currently under development contemplate different approaches to the natural phenomena of atmospheric dispersion. [2, 3]. The project aims to evaluate four types of atmospheric dispersion models, two Gaussian models, a deterministic and stochastic model and finally a Lagrangian particle model. The simulations will be used in order to evaluate the atmospheric dispersion in a real situation. Industrial atmospheric emission of CMPC CELULOSE RIOGRANDENSE will be studied. Continuous air quality monitoring will be used for the comparison between the different models and the actual physical phenomenon of atmospheric dispersion. The air quality station of CMPC CELULOSE RIOGRANDENSE (AQCMPC) will be used in this evaluation. In these station various atmospheric parameters are monitored such as: wind speed, wind direction, temperature, relative humidity, global and UV radiation, and atmospheric pressure. Air quality monitoring sensors permit to establish the atmospheric concentrations of ozone (O3), inhalable particulate matter (PM10), carbon monoxide (CO), sulfur dioxide (SO2), Nitrogen Oxide (NO), Nitrogen Dioxide (NO2), and Total Reduced Sulfur compounds (TRS). Among the compounds present in the TRS are hydrogen sulphide (mercaptanes), dimethylsulfide (C2H6S6), dimethyl disulfide (C2H6SO2), carbonyl sulfide (COS) and carbon disulfide (CS2). The air quality monitoring data from the municipality of Guaíba, Rio Grande do Sul, will be provided by CMPC CELULOSE RIOGRANDENSE. After evaluating all models, an autonomous air and odor quality system will be created with the best performance model, i.e. when the simulated results of atmospheric concentration are very correlated with the measured atmospheric concentration [4]. The first model to be evaluated called CALPUFF is a state-of-the-art software in the dispersion of contaminants in the atmosphere [5]. CALPUFF is a model for environmental regulation issues and is currently adopted in the state of Rio Grande do Sul as the tool to be used in activities of environmental regulation and air quality assessment. For this reason, it is essential to compare this model with other models under development.The second approach makes use of a deterministic model, in which the results are average values to be compared with the relevant legislation for air pollution. The stochastic behavior of atmospheric turbulence is not taken into account. More precisely, it is an attempt to simulate stochastic effects in a deterministic model for dispersion of pollutants in the atmospheric boundary layer by the use of a probability-weighted contour condition. That is, the escape of pollutants through the top of the boundary layer on one side and the surface boundary on the other side are modeled by probabilities to quantify the fraction of pollutant that returns to the boundary layer from above and the process of adsorption or deposition in the soil. These effects are represented by partially reflective contour conditions which together with diffusion and advection define the model under consideration. The consequences of the reflections have already been analyzed using the meteorological conditions and data from Hanford and Copenhagen experiments. A variety of simulations have shown that the partial reflection on the top of the boundary layer and on the soil, respectively, obtains the most significant correlations between the model and the data, suggesting that the contour effects are essential to model the dispersion processes in the atmospheric boundary layer [3].A second approach of the research group is to simulate the dispersion of pollutants through a deterministic/stochastic model. In this case, fluctuations in atmospheric concentration are expected and desired. It also consists of a robust environmental simulation tool, in which the presence of fluctuations helps to study and identify intervening variables in the atmospheric dispersion process. Thus, a new attempt with deterministic-stochastic structure can combine the advantages of models, while providing higher statistical moments properties that characterize stochasticity rather than suggesting additional models for each statistical moment. To this end, we study a deterministic model, more specifically the advection diffusion equation, which is based on the continuity equation, together with a Fickian-type closure. Since Fickian closure makes the model deterministic, a complex turbulence diffusivity was proposed that explains the presence of a phase and allows to interpreat the solution of the complex advection diffusion equation in terms of an amplitude that, after multiplication with its complex conjugate defines a probability density function of sesquilinear form. Such a procedure already exists, for example, the energy density in electrodynamics or the probability density function in quantum approaches. We performed a selection of simulations to explore the effects of the parameterization of the complex turbulent diffusion on the pattern of concentration distributions. These studies shall contribute to an understanding as to how to determine the dispersion coefficients from observational data [2].The last one is the stochastic Lagrangean particle model (LAMBDA) [67] that is efficient and fundamental tool in the investigation and study of the phenomena of turbulent diffusion in the planetary boundary layer (CLP). In a stochastic turbulent dispersion Lagrangean model the movement of fluid particles subject to speed fluctuations is simulated. Such particles passively follow the turbulent flow. In these types of model, to reproduce the effects of eddies, the velocities of the particles are subjected to a random forcing [8]. Thus, such dispersion models are based on the stochastic Langevin equation. This equation is derived assuming that velocity can be written as the sum of a deterministic term and a stochastic term. In this case, for each time step, the fluid particle moves due to the action of the average wind and the turbulent diffusion. This flow is characterized by certain initial conditions and physical constraints. Therefore, the motion of each particle is independent of the remaining ones. Thus, the concentration field, estimated from the spatial distribution of the particles, is interpreted as a mean performed on the total set of simulated particles [1, 9].
2. Experiment
The CMPC CELULOSE RIOGRANDENSE station conducts continuous assessments of air quality and meteorological data. Air quality observations utilize Thermo Scientific equipment, which follows international standards in air quality assessment, in accordance with the US Environmental Protection Agency (EPA). It is located in the municipality of Guaíba, RS, at the following geographical coordinates: latitude 30.113882 degrees to the south and longitude 51.332051 degrees to the west, approximately 2 kilometers from the CMPC. The Location was established throughout the environmental impact assessment, in agreement with the environment agency FEPAM. Since the station opperates in continuous mode, it was only necessary to establish the period of data to be used. Initial evaluations of the models were employed for the compound of interest TRS. It is a typical compound emitted into the atmosphere in the pulp production, and the pulp industry is the main contributor in the region. The comparison between models and the observational data of the station is adequate and simplified by the knowledge of a main source in the region.The evaluation comprises the period from January of 2016 to April of 2017, totalling 11184 hours of available observational data. First, the observations under suspicion were discarded, indicating malfunction. Secondly, preference was given to wind direction data from east to south. The air quality station is most influenced by the atmospheric emissions of CMPC CELULOSE RIOGRANDENSE in this quadrant of wind direction, which is also the predominant quadrant in the region. Figure 1 shows the study area of the research project.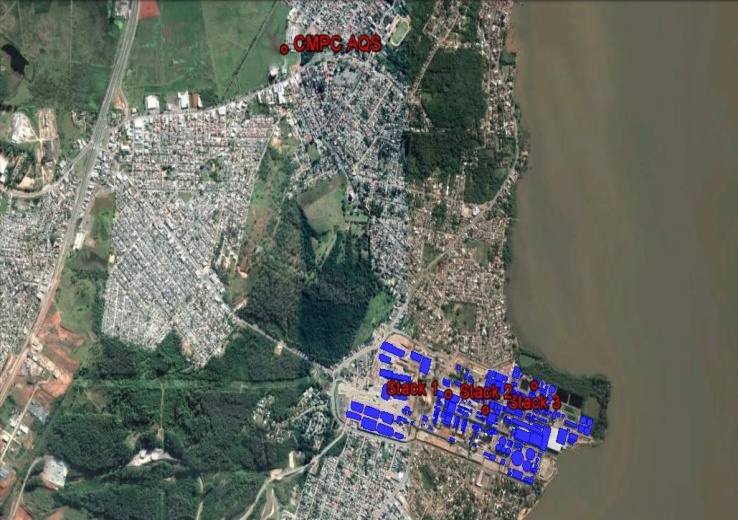 | Figure 1. Location of the CMPC air quality station (CMPCAQS), point sources and CMPC industrial buildings CMPC in blue |
3. Methodology
The analysed region comprises part of figure 1, in the locality of Guaíba, Rio Grande do Sul state in Brazil. The simulation included the location and amount of CMPC emissions of the tracer gas, the location of the air quality station and the surface and altitude meteorological stations. Topography was initially considered without elevations in this first approach. The performance of the CALPUFF model was established by comparing the surface concentrations and the results observed at the CMPCAQS. Hourly simulated concentration data were compared with hourly observations of TRS. Since it comprises a period of more than one year, the simulations encompassed a great variability of atmospheric stability. Micrometeorological variables were established by the AERMET pre-processor, incorporating data from the CMPCAQS station. Variables which are not measured at the CMPCAQS (cloud cover and ceiling height) were complemented by data from the Salgado Filho Airport meteorological station. All data were compiled into a single file referring to the surface data. Altitude data (radiosondes) were also used, from the Salgado Filho Airport, in Porto Alegre. The choice of the AERMET pre-processor was established based on the future need to compare different simulation methods. The ADMG-UFRGS produces computational codes with or without spatial variability of the atmospheric conditions, in the lateral and longitudinal axes. It is appropriate to compare the CALPUFF model in the non-spatial variability mode, because the necessity of comparison with the LAMBDA model, that does not consider spatial variability. For CALPUFF this does not necessarily result in a decrease in simulation quality. The lack of precision when CALMET model is used is well known, which is based on station meteorological data to generate the meteorological and micrometeorological fields [5, 10]. It is crucial to consider that once the micrometeorological quantities like friction velocity, convective velocity, Obukhov length and CLP height are established, these provide an initial condition or FIRST GUESS. No additional measures were taken to validate micrometeorology. For this reason, the adjustment or perception of the most representative atmospheric conditions for a given moment of simulation will be used by the CALPUFF model. Different values of micrometeorological variables, for a same instant of time, were attributed. The value of the ideal variable consisted of the one where the result of the CALPUFF model was better adjusted to the observed atmospheric concentration value. The choice was not random but based on three quantities that impose physical affinity to micrometeorological data. The surface roughness, the albedo and the Bowen ratio. Changes in these quantities were imposed during pre-processing of the AERMET, which established the quantities of interest in a simulation of pollutants dispersion. The validation or not of a micro variable was performed by the CALPUFF model, in the search for the ideal solution (best mathematical representation of the dispersion process). In this way the real micrometeorology (validated) consisted in the one, where the comparison between observed and simulated concentrations had the smallest difference. This methodology was quite efficient in the search for the contour conditions and characteristic scales of the atmospheric flow, given the large number of degrees of freedom.The fraction of solar radiation that reaches the surface and is reflected by the soil (albedo) ranged from 0.6 to 0.1. The amount of moisture present on the surface (Bowen ratio) ranged from 6 to 0.1. Surface roughness varied between 1.3 and 0.0001. The change in surface roughness is also necessary to impose changes in the behaviour of surface friction and to compensate to some extent the non-inclusion of the topography. Different roughness values are also related to the fact that in the simulation domain, changes in soil characteristics are very present. The presence of the Guaíba River, industrial buildings, residential buildings and trees, are some of the physical objects that impose changes in the behaviour of superficial friction. Therefore, in the continuous flow, several friction changes are present, at least in the same proportion of the number of obstacles present in the region. The search for the characteristic roughness is a parameterization that represents the infinite degrees of freedom of the flow. The same concept applies to the two other imposed variables. That is, albedo variability and Bowen ratio are to represent some physical characteristics of the flow.Initially, with only one land use characteristic for surface roughness, albedo and Bowen ratio, the simulated results were poorly correlated with the values observed at the CMPCAQS (Figure 2). 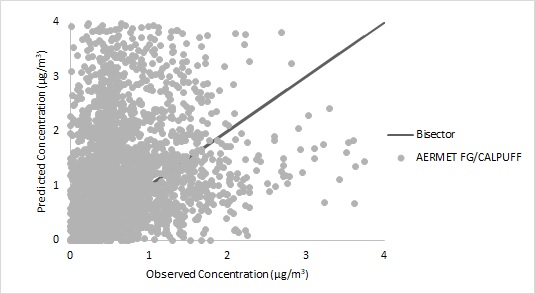 | Figure 2. Scatter diagram of modeling results of the AERMET FIRST GUESS/CALPUFF in comparison with observed concentrations |
As changes in the physical properties of the atmosphere and soil were imposed, the correlation between observed and simulated increased. On the other hand, the simulation with CALMET meteorological pre-processing had the same bad correlation of the initial condition with a single soil characteristic established by AERMET. 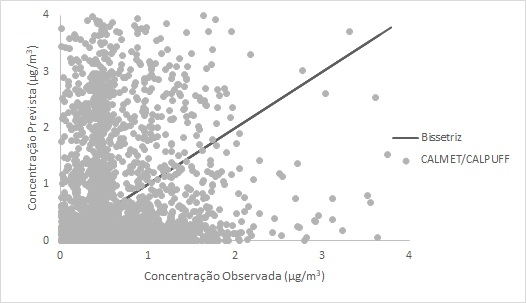 | Figure 3. Scatter diagram of modeling results of the CALMET/CALPUFF in comparison with observed concentrations |
Again, distortions of the micrometeorological fields of the CALMET are verified, when initialized from the meteorological station data, even with the inclusion of topography and land use varying spatially.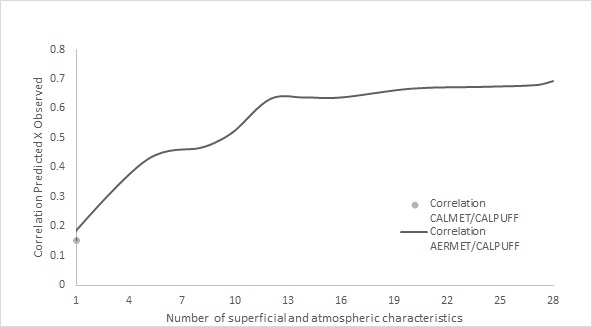 | Figure 4. Correlation in function of the increase of the superficial and atmospheric characteristics considered in the simulations |
Figure 4 shows the evolution of the correlation between predicted atmospheric concentrations compared to the observed concentrations. As the number of contour conditions and turbulent scales increases, with the previous air and soil characteristics presented, there is a larger affinity between observed and predicted results. In the FIRST GUESS there is a large lack of affinity between the signals, as indicated by the low correlation value. However, in the last trial, which counted a total of twenty-eight different boundary conditions and turbulent scales (BCTS), the correlation turned satisfactory. The spreading diagrams also present the same findings of better agreement in the final condition.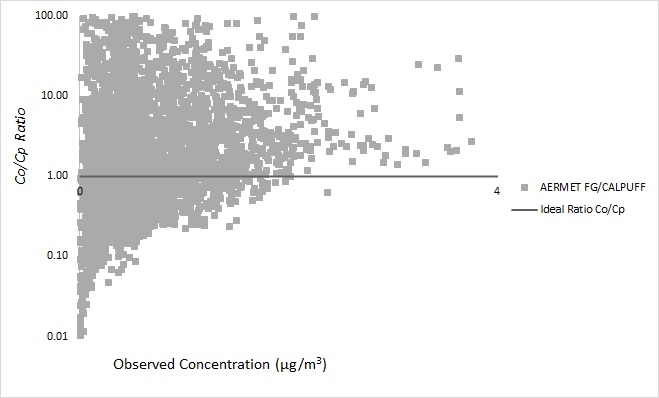 | Figure 5. Ratio between observed and predicted concentration AERMET FIRST GUESS/CALPUFF |
From the predicted results (Figure 5 and 6), it was possible to establish the ratio between observed and predicted concentrations. This evaluation allows us to understand that, initially, the distribution of the solutions is not centred at unity. It also presents a sparser behaviour, especially with underprediction. This behaviour implies in the existence of a physical lack of memory of the FIRST GUESS flow or the inability to represent the infinity of degrees of freedom. 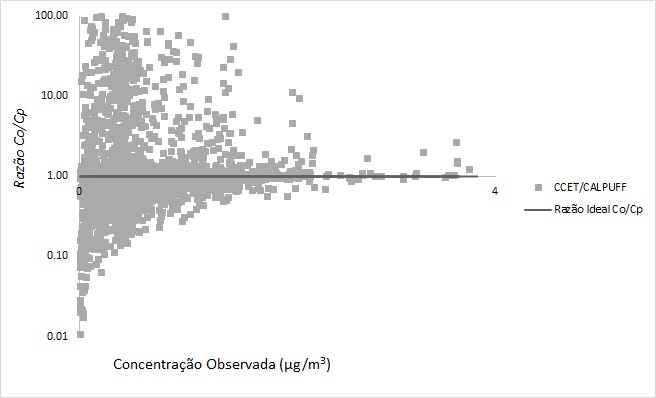 | Figure 6. Ratio between observed and predicted concentration AERMET CCET/CALPUFF in comparison with observed concentrations |
When the BCTSs were validated in the least error solutions, the distribution approached unity and was effectively less sparse in the super and underprediction.The validated BCTS simulations allow reconfiguring the solution in the most appropriate way of representing the natural phenomena. It is important to note that the CALMET pre-processor, initialized from the meteorological station data, has the same inability as the FIRST GUESS of the AERMET preprocessor, even considering the topography, land use and terrain roughness.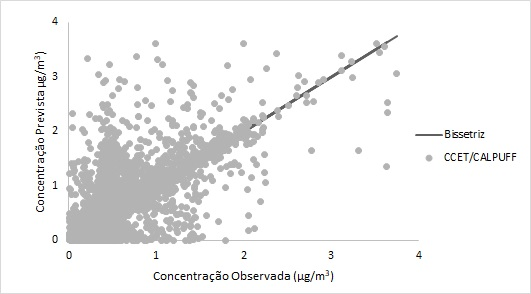 | Figure 7. Scatter diagram of modeling results of the AERMET CCET/CALPUFF in comparison with observed concentrations |
A linear regression also was evaluated to identify the deviation of the ideal situation and is presented in Figure 8. 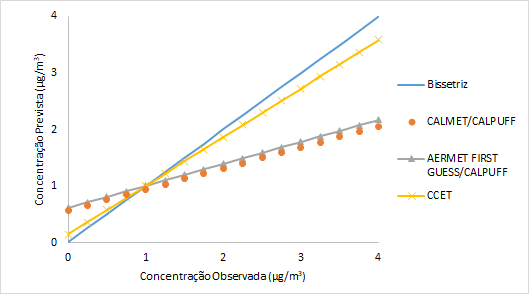 | Figure 8. Linear regression for the three alternatives simulated |
This analysis helps to determine how the model correlates with the expected data (the ideal case). The quantity that measures the deviation is
and is defined as [11]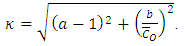 | (1) |
In the ideal case
should be zero. The model data around the straight line can be analyzed through the correlation coefficient close to 1.On the other hand when the average monthly atmospheric concentration of TRS was compared to the CALPUFF simulation with AERMET initialization in the FIRST GUESS option, the results suggest a satisfactory agreement between CALPUFF and observed data (Figure 9).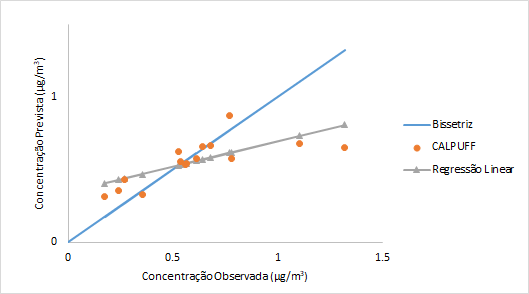 | Figure 9. Scatter diagram diagram for monthly averages |
Table 1. Location and characteristics of CMPC atmospheric sources  |
| |
|
Table 2. Models comparison with linear regression for monthly averages  |
| |
|
Table 3. Models comparison with linear regression for hourly averages  |
| |
|
4. Conclusions
The present study is part of a research project of the ADMG-UFRGS, in partnership with the Luiz Englert Foundation and financed by CMPC CELULOSE RIOGRANDENSE. The project aims to evaluate four types of atmospheric dispersion models, two Gaussian models, a deterministic and stochastic model and finally a Lagrangian particles model. The simulations will be used in order to evaluate the atmospheric dispersion in real situations of the industrial atmospheric emission of CMPC CELULOSE RIOGRANDENSE. After the evaluation of the four models, an operational air quality forecast system will be created. The tool that presents the best performance will be the one chosen to compose the system.The initial results for the CALPUFF dispersion model indicate a need for initial adjustment of boundary conditions and micrometeorological parameters. In the two initial approaches to meteorological pre-processing, AERMET FIRTS GUESS and CALMET, have led to high uncertainties in CALPUFF simulations. In this way, it was necessary to create a previous routine of validation (search) of turbulent parameters and of the appropriate or representative boundary conditions of the atmospheric flow at the locality of Guaíba. Once the CCET is established, it is expected to be able to compare the natural phenomenon in a more representative way. The other models will then be tested with the CCET established in this study, allowing to identify the model that will show the best performance in comparison with observed air quality data.Table 4. Models comparison with linear regression 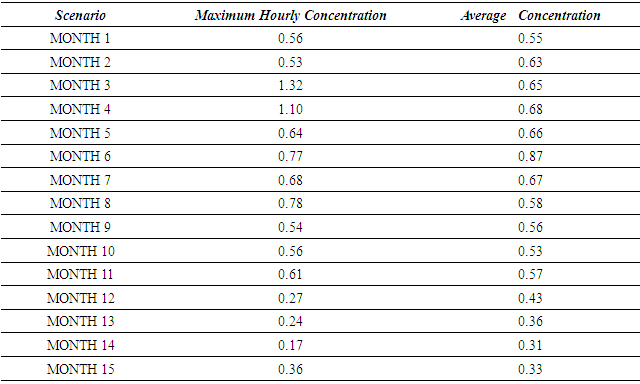 |
| |
|
Table 5. Models comparison with linear regression 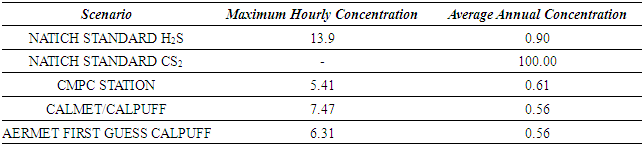 |
| |
|
The air quality and odor perception in the surroundings of CELULOSE RIOGRANDENSE were evaluated based on the atmospheric concentrations of compounds contained in the TRS. The following table presents the results of observed and simulated surface atmospheric concentrations. The reference adopted is in the National Air Toxics Information Clearinghouse - Environment Protection Agency (NATICH) [12], since Brazilian legislation does not establish air quality standards and odor for TRS. The participation of each compound was considered as total.In environmental licensing activities, AERMET and CALMET processors are often employed. The use of these two tools, with CALPUFF, enables a satisfactory representation of the monthly and annual average. In the temporal representation, hour by hour, the previous validation of the CCET is necessary. As the system is designed to reproduce the real time situation, the long-term average values are not sufficient. The AERMET\ FIRST GUESS and CALMET are not representative in this time resolution. The correction can be used from the presented technique for the boundary conditions. In the present work, 28 combinations of soil and atmosphere characteristics were simulated, which allowed for a greater temporal agreement in the short term.
ACKNOWLEDGEMENTS
The authors thank CMPC CELULOSE RIOGRANDENSE for the total financial support of this work.
References
[1] | F. C Degrazia, M. T. Vilhena, G. A. Degrazia, B. E. J. Boadmann. Comparisons between line source modeling with calpuff and lagrangian particle model lambda. Ciência e Natura, 2015. |
[2] | D. L. Gisch, B. E. J. Bodmann, M. T. M. B. Vilhena, A genuine solution of the diffusion advection equation sesqui-linear way to multi-source problem. American Journal of Envi-ronmental Engineering, v. 6(4A), p. 160–163, 2016. |
[3] | J. F. Loeck, B. E. J. Bodmann, M. T. M. B. Vilhena, On a model for pollutant dispersion in the atmosphere with partially reflective boundary conditions. American Journal of Environmental Engineering, v. 6(4A), p. 28–39, 2016. |
[4] | F. C. Degrazia, H. F. C. Velho, R. R. Cintra, J. P. S. Barbosa, M. R. Moraes, Sistema de previsão da qualidade do ar para o vale do paraíba. Ciência e Natura, v. 29, p. 293-296, 2007. |
[5] | Environmental Protection Agency. Documentation of the Evaluation of CALPUFF and Other Long Range Transport Models Using Tracer Field Experiment Data. [S.l.]: ENVIRON International Corporation, 2012. |
[6] | E. Ferrero, D. Anfossi, Comparison of PDFs, closures schemes and turbulence parameterisations in Lagrangian Stochastic Models. International Journal of Environment and Pollution, 9, p. 384-410. 1998a. |
[7] | E. Ferrero, D. Anfossi, Sensitivity analysis of Lagrangian Stochastic models for CBL with different PDF's and turbulence parameterisations. In: S.E. Gryning, N. Chaumerliac, (Eds.), Air Pollution Modelling and its Applications XII, Vol. 22. Plenum Press, New York, p. 673-680, 1998b. |
[8] | H. Rodean. American Meteorological Society. 1996. |
[9] | D. L. Gisch, B. E. J. Bodmann, M. T. M. B. Vilhena, A genuine solution of the diffusion advection equation sesqui-linear way to multi-source problem. American Journal of Envi-ronmental Engineering, v. 6(4A), p. 160–163, 2016. |
[10] | F. C Degrazia, M. T. Vilhena, G. A. Degrazia, B. E. J. Boadmann. The Influence of Decorrelation Time Scales in the CALPUFF Modeling System under Neutral Conditions. American Journal of Environmental Engineering, v. 5(1A), p. 125-132, 2015. |
[11] | K. B Mello, Simulação da Dispersão de Poluentes na Camada Limite Planetária através da Solução da Equação de Langevin pelo Método de Decomposição, Programa de Pós Graduação de Engenharia Mecânica, Universidade Federal do Rio Grande do Sul, 2010. |
[12] | Environmental Protection Agency. National Air Toxics Information Clearinghouse: NATICH Data Base Report on State and Local Agency Air Toxics Activities - Final Report: Fog. North Carolina, 1990. Disponível em: <https://0x9.me/czYND>. Acesso em: Acessado em 16 set 2017. |